Bicubic G1 interpolation of arbitrary quad meshes using a 4-split
基于LIG的红外视频小目标检测方法

0 引言
and gradientꎬLIG) 目标检测方法ꎬLIG 算法表现出了良好
红外视频包含大量背景杂波和空气流动等引起的噪
点ꎬ因此从远距离检测红外小目标具有一定难度ꎮ 目前ꎬ
红外图像的小目标检测已成为视觉检测领域的研究热点ꎮ
文献[1] 利用局部对比度检测红外小目标的方法耗时且
有时会增强目标和杂波ꎮ 为了提高性能ꎬ文献[ 2] 引入了
which verifies the effectiveness of the proposed meted videoꎻ small target detectionꎻ LIGꎻ real-time tracking
目标检测性能ꎮ 其次ꎬ引入了低强度与梯度( low intensity
多尺度对比度度量方法ꎮ 文献[ 3- 4] 引入红外拼接图像
的性能和计算速度 [5] ꎬ并且引入连通分量分析与扩张方
法ꎬ从而降低了误检ꎮ 最后ꎬ为了进一步提高整体检测性
能ꎬ在本文的框架中加入了简单在线和实时跟踪( simple
online and realtime trackingꎬ SORT) 目标关联算法ꎬ进一步
super - resolution moduleꎬ unsupervised target detectorꎬ connected area analysis module and track correlation moduleꎬ is
established. The proposed methodꎬ composed of video resoluton increaseꎬ local gradient small together detection and multi frame
Bile acids, obesity, and the metabolic syndrome

4Bile acids,obesity,and the metabolic syndromeHuijuan Ma,MD,Adult Endocrinologist a ,Mary Elizabeth Patti,MD,Investigator andAdult Endocrinologist b ,*aDepartment of Endocrinology and Metabolism,Hebei General Hospital,Shijiazhuang,Hebei 050051,China b Research Division,Joslin Diabetes Center,and Harvard Medical School,Boston,MA 02215,USAKeywords:Bile acidsFXRTGR5ObesityInsulin resistance a b s t r a c tBile acids are increasingly recognized as key regulators of systemic metabolism.While bile acids have long been known to play important and direct roles in nutrient absorption,bile acids also serve as signalling molecules.Bile acid interactions with the nuclear hormone receptor farnesoid X receptor (FXR)and themembrane receptor G-protein-coupled bile acid receptor 5(TGR5)can regulate incretin hormone and fibroblast growth factor 19(FGF19)secretion,cholesterol metabolism,and systemic energyexpenditure.Bile acid levels and distribution are altered in type 2diabetes and increased following bariatric procedures,in parallelwith reduced body weight and improved insulin sensitivity andglycaemic control.Thus,modulation of bile acid levels andsignalling,using bile acid binding resins,TGR5agonists,and FXRagonists,may serve as a potent therapeutic approach for thetreatment of obesity,type 2diabetes,and other components of themetabolic syndrome in humans.©2014Elsevier Ltd.All rights reserved.IntroductionBile is a mixture of bile acids (BAs),cholesterol,phosphatidylcholine,and bilirubin.Of these,BAs are essential constituents and play critical roles in regulation of metabolism in both humans and animal *Corresponding author.Joslin Diabetes Center,1Joslin Place,Boston,MA 02215,USA.Tel.:þ16173091966;fax:þ16173092593.E-mail address:Mary.Elizabeth.Patti@ (M.E.Patti).Contents lists available at ScienceDirectBest Practice &Research ClinicalGastroenterology/10.1016/j.bpg.2014.07.0041521-6918/©2014Elsevier Ltd.All rights reserved.Best Practice &Research Clinical Gastroenterology 28(2014)573e 583models.Bile acids have long been recognized to aid in the absorption of fat and fat-soluble vitamins and modulate cholesterol levels.However,recent data indicate that bile acids also play an important role in glucose and lipid homeostasis by activating both the nuclear receptor LXR and the cell surface receptor G protein-coupled bile acid receptor 5(TGR5)[1e 3].Moreover,modulation of plasma bile acid levels and the total bile acid pool can affect glycaemic control,body weight,and insulin sensitivity[4e 6].In this review,we will focus on the relation between bile acids and regulation of systemic meta-bolism and the potential for bile acids as a therapeutic approach for obesity,insulin resistance,type 2diabetes (T2D),and other components of the metabolic syndrome.Bile acid synthesis and regulationBile acid synthesisBAs are amphipathic molecules with a steroid backbone which are synthesized from cholesterol in hepatocytes.It is estimated that about half of the 800mg of cholesterol synthesized daily is used for bile acid synthesis,totalling about 200e 600mg daily in humans [7].Bile acids are synthesized from cholesterol through two dominant pathways:the classic pathway and the alternative pathway (Fig.1).In the classic (or neutral)pathway,CYP7A1catalyses the initial and rate-limiting step converting cholesterol into 7a -hydroxycholesterol,with CYP8B1subsequently regulating synthesis of 12a -hydroxysterols including cholic acid (CA).In the alternative (or acidic)pathway,CYP27A1first hydroxylates the cholesterol side chain,converting cholesterol into 27-hydroxycholesterol,which is then 7a -hydroxylated by CYP7B1prior to CYP8B1action.In humans,the classical pathway produces the primary BA cholic acid (CA)and chenodeoxycholic acid (CDCA)in Fig.1.Bile acid synthesis pathway.Cholesterol is converted to two primary bile acids in human liver,CA and CDCA.Key regulatory enzymes in these pathways include CYP7A1,CYP8B1,CYP27A1,and CYP7B1.CYP7A1initiates the classic (neutral)biosynthetic pathway,while CYP27A1initiates the alternative (acidic)pathway in liver and macrophages.CA and CDCA can be conjugated with glycine (G)and taurine (T).In the intestine,conjugated CA and CDCA are deconjugated and then dehydroxylated at the 7a -position to the secondary bile acids DCA and LCA,respectively.H.Ma,M.E.Patti /Best Practice &Research Clinical Gastroenterology 28(2014)573e 583574H.Ma,M.E.Patti/Best Practice&Research Clinical Gastroenterology28(2014)573e583575 roughly equal amounts,whereas the alternative pathway produces mainly CDCA[8].Most bile acids are conjugated with either glycine or taurine,with a3:1predominance of glycine over taurine[3,5,9].Synthesized BA are stored in the gallbladder and secreted into the duodenum in response to feeding,contributing to digestion of lipids and lipid-soluble vitamins.The primary BA CA and CDCA can be dehydroxylated at the7a position by gut microbiota to produce the secondary BAs,predominantly deoxycholic acid(DCA)and lithocholic acid(LCA)[10,11].Thus,bile acid levels and relative composition can be modulated by gut microbiota populations[12].In the terminal ileum,BAs are efficiently absorbed by both active transport and passive diffusion, transported back to the liver via the portal vein,taken up at the sinusoidal membrane of hepatocytes, and secreted into bile again.Each BA molecule may complete4e12cycles of this enterohepatic cir-culation per day[1,13].This process is highly efficient,as only about5%of bile acids are lost in feces [3,14].This process is similar in mice,although different bile acid species dominate.CDCA is efficiently converted into muricholic acid(MCA),and BAs are conjugated to taurine.Given that different BA have different structures,hydrophobicity,and affinities for membrane and nuclear receptors,interindividual differences in BA pool composition resulting from differential regulation of the complex BA synthesis pathway may have functional consequences for systemic metabolism.Regulation of Bile Acid SynthesisBA are potent regulators of their own synthesis,serving to limit excessive accumulation of BA in the circulation via multiple redundant pathways.Feeding bile acids to rats strongly reduces CYP7A1 enzyme activity and bile acid synthesis[8,15].This self-regulation of BA synthesis involves activation of the nuclear receptor FXR(farnesoid X receptor,official gene name NR1H4)[16].FXR knockout mice have increased BA synthesis and Cyp7a1expression,verifying the central role for FXR in mediating bile acid inhibition of Cyp7a1[17].FXR has both direct effects on Cyp7a1expression as well as indirect effects,mediated by induction of small heterodimer partner(SHP)which in turn inhibits trans-activation of CYP7A1and CYP8B1by the transcription factors Hepatocyte Nuclear Factor4a(HNF4a) and liver-related homolog-1(LRH-1)at the bile acid response element.FXR also increases BA conju-gation and upregulates expression of several genes which promote bile acid efflux from hepatocytes into the bile.An additional feedback repression mechanism involves FXR regulation of several indi-vidualfibroblast growth factors(FGFs).For example,bile acid activation of FXR results in secretion of FGF-19from hepatocytes and enterocytes.In turn,FGF-19can bind tofibroblast growth factor receptor 4(FGFR4)receptors on hepatocytes,leading to suppression of CYP7A1expression and bile acid syn-thesis via a SHP-independent,but c-Jun N-terminal kinase(JNK)dependent mechanism[18].Consis-tent with this mechanism,FGFR4knockout mice have increased expression of CYP7A1,in parallel with increased fecal bile acids and bile acid pool size[19].Other nuclear receptors,such as the pregnane X receptor(PXR)[20]and vitamin D receptor(VDR)[21],can also regulate BA synthesis by suppressing CYP7A1.Moreover,activation of the nuclear receptor ROR a can modulate12a-hydroxylase(CYP8B1) expression[22,23].BA differ markedly in their potency to activate FXR.The hydrophobic bile acid CDCA is the most potent BA ligand of FXR,followed by lithocholic acid(LCA),deoxycholic acid(DCA),and CA;by contrast, the hydrophilic bile acids ursodeoxycholic acid(UDCA)and muricholic acid(MCA)do not activate FXR[24].BA are also regulated in response to other elements of systemic metabolism.Early studies showed that the bile acid pool size is increased in insulin-deficient diabetic rats,with a3-fold increase in the cholic acid pool[25].Conversely,insulin treatment reduces bile acid pool size,inhibits CYP7A1and CYP8B1activity,and alters bile acid composition[26].CYP7A1can also be regulated by steroid hor-mones,activated protein kinase C,and proinflammatory cytokines[8].Bile acids and regulation of systemic metabolismFXR and TGR5signalling mechanisms appear to dominate for BA effects on regulation of glucose, lipid,and energy metabolism[1,27e30].As noted above,BA are natural ligands for FXR a,a nuclearreceptor highly expressed in liver,intestine,kidney and adrenal glands [17,27].BAs can also activate TGR5,a membrane-bound G protein-coupled BA receptor.TGR5is expressed in many organs and tissues,with high expression in macrophages/monocytes,placenta,gallbladder,liver and intestine[31,32].BA stimulation of energy expenditure in brown adipose tissue (BAT)and skeletal muscle ap-pears to be mediated via TGR5[2].Bile acids and lipid metabolismBAs exert an important regulatory role in cholesterol and triglyceride (TG)metabolism.Increased bile acid synthesis increases utilization of cholesterol as substrate.Bile acid synthesis rates are correlated with serum triglyceride levels in hyperlipidemic patients [33].Bile acid sequestrants or other interruptions in enterohepatic circulation also increase both bile acid and VLDL triglyceride synthesis.Conversely,CDCA-mediated increases in the BA pool size leads to inhibition of BA synthesis and reduced serum triglycerides in hyperlipidemic patients [34].These effects of BA to modulate TG metabolism are likely mediated via several distinct mechanisms,predominantly BA activation of FXR [35,36].FXR alters the transcription of several genes involved in fatty acid and triglyceride synthesis and lipoprotein metabolism.In mice,administration of FXR ago-nists [GW4064,6a-ethyl-chenodeoxycholic acid]reduces plasma triglyceride and cholesterol levels[37e 41]via repression of the lipogenic genes sterol-regulatory-element-binding protein-1c (SREBP1c)and fatty acid synthase (FAS)in liver [36].FXR also induces expression of peroxisome proliferator activated receptor (PPAR)a ,a nuclear receptor that promotes lipid oxidation [42],and of pyruvate dehydrogenase kinase,isoenzyme 4(PDK4),leading to inhibition of pyruvate dehydrogenase and increased fatty acid oxidation [43].Additional FXR target genes include the apolipoproteins A-V,C-III,apoE,syndecan-1,and the VLDL receptor [1,44].Conversely,FXR-null mice have higher serum TG levels and increased synthesis of apolipoprotein (apo)B-containing lipoproteins [17].Thus,bile acids play central roles in lipid metabolism and in the control of TG levels,in part via FXR and downstream transcriptional targets.Additional effects of BA on lipid metabolism may be independent of FXR.For example,the bile acid tauroursodeoxycholic acid (TUDCA)also acts as a chaperone,modulating endoplasmic reticulum stress[45].TUDCA reduces adipogenesis in human adipocyte stem cells [46].Similarly,in another study,UDCA (but not TUDCA)profoundly inhibits adipogenesis,in parallel with activation of extracellular regulated protein kinases 1and 2(ERK 1/2)[47].Bile acids and glucose metabolismBile acids are also implicated in regulation of glucose metabolism [48e 50].Increasing hepatic bile acid synthesis can inhibit gluconeogenesis and stimulate glycolysis.Effects on glycogen metabolism appear complex [51].Some studies have shown bile acids stimulate glycogen phosphorylase (GP)and glycogen breakdown to glucose-1-P [52],while other data indicate bile acids also activate glycogen synthesis (GS)[53].Additional effects of BA on glucose metabolism and insulin action may also be mediated via reductions in endoplasmic reticulum (ER)stress,a key mediator of insulin resistance [45].In addition to direct effects,many of the bene ficial effects of BA on glucose metabolism are mediated via FXR.FXR is an important regulator of glucose metabolism,as demonstrated by reduced plasma glucose and reduced hepatic glycogen levels in FXR-null mice [49,50,54].Conversely,activation of FXR is associated with increased phosphoenolpyruvate carboxykinase (PEPCK)and glucose-6-phosphatase expression and glucose output from primary rat hepatocytes.However,in vivo pharmacologic stimulation of FXR in two mouse models of obesity and T2D (db/db or KKA(y)mice)causes inhibition of gluconeogenesis,hypoglycemia,and increased insulin sensitivity [49,50].Thus,FXR may have a dominant effect to inhibit gluconeogenesis in diabetes,perhaps via inhibition of PEPCK by SHP-dependent inhibition of HNF4a and FoxO1[55].Activation of FXR can also stimulate the insulin/Akt pathway,promoting glycogen synthesis in liver [55],GLUT2activation in pancreatic b -cells [56],and improving insulin resistance in obese ob/ob mice [57].In this context,inhibition of gluconeogenesis,improved insulin action,and stimulation of glycogen syn-thesis may synergize to improve plasma glucose,insulin secretion,insulin sensitivity,and glucoseH.Ma,M.E.Patti /Best Practice &Research Clinical Gastroenterology 28(2014)573e 583576H.Ma,M.E.Patti/Best Practice&Research Clinical Gastroenterology28(2014)573e583577 tolerance.In parallel,however,GW4064increases susceptibility to high fat diet-induced obesity and diabetes[50,58].These complex data highlight the importance of the specific context in which FXR is activated.Beneficial effects of BA on glucose metabolism may also be mediated via TGR5.TGR5is expressed in many organs and tissues,with highest expression in macrophages/monocytes,placenta,gallbladder, liver and intestine[32].Activation of TGR5in enterocytes can stimulate the secretion of the incretin hormone glucagon-like peptide GLP1,promoting glucose-dependent insulin secretion[59,60].More-over,BA stimulation of cell-surface TGR5on neurons may also modulate GLP-1secretion[61].In pe-ripheral tissues,TGR5activation may increase activation of the type2deiodinase,resulting in increased active thyroid hormone,mitochondrial oxidative capacity,and energy expenditure[2].A recent human genetic study demonstrates that a single nucleotide polymorphism(SNP)at the TGR5 locus(rs3731859)is associated with BMI,waist circumference,intramyocellular lipids,and fasting GLP-1levels[62].Furthermore,TGR5-null mice have a25%reduction in bile acid pool size,and female Tgr5 null mice show increased weight gain and fat accumulation when fed a high fat diet[63].Further support for the regulatory role of TGR5in glucose homeostasis comes from thefinding that TGR5 agonists decrease blood glucose in animals[64].Bile acids in humansIn healthy individuals,BA levelsfluctuate with cycles of fasting and refeeding.BA robustly increase in response to an oral glucose load[65].Such responses are increased in insulin sensitive individuals and are blunted in individuals with prediabetes[55],suggesting dysregulation of bile acid pools and/or intestinal regulation in insulin resistance.The majority of studies have analysed bile acid levels and species distribution in the fasting state; many of these demonstrate alterations in insulin resistance and diabetes.For example,one study demonstrated1.6-fold increases in deoxycholic acid(DCA)in T2D[66].Similarly,Haeusler et al. demonstrated that12a e hydroxylated species(sum of CA,DCA,and their conjugates)are significantly higher in patients with T2D[67].Furthermore,ratios of12-hydroxylated/non e12-hydroxylated BAs are associated with key features of IR,including higher insulin,glucose,and triglyceride(TG)levels and lower HDL cholesterol[67].Brufau and colleagues showed that individuals with T2D had higher cholic acid(CA)synthesis rates and enlarged DCA pool size[68].Our group recently reported that concen-trations of total taurine-conjugated BA were higher in T2D and intermediate in individuals with impaired glucose tolerance[69].Interestingly,total taurine-BA were positively associated with fasting and post-load glucose levels,fasting insulin,and HOMA-IR.However,insulin-mediated glycaemic improvement in T2D patients did not change fasting serum total BA,or BA composition,suggesting dysregulation of BA levels is not directly linked to glycaemic burden but possible due to other aspects of insulin resistance or the metabolic syndrome.Bile acids are increased following bariatric surgeryBariatric surgery is increasingly recognized as a robust method to not only reduce body weight,but also to reduce glycaemia and medication requirement(so called diabetes‘remission’)[70].Bariatric surgical procedures include Roux-en-Y gastric bypass(RYGB),vertical sleeve gastrectomy(VSG), laparoscopic adjustable gastric banding(LAGB)and biliopancreatic diversion(BPD).In this context,it is interesting that several studies have demonstrated that bile acids are markedly increased following bariatric surgery[5,6,71,72].Interestingly,total bile acids in post-bypass patients are correlated with improvement in several key metabolic parameters;bile acids are inversely correlated with post-prandial glucose,triglycerides,and positively correlated with adiponectin and peak GLP1levels following a mixed meal test.These intriguing data suggest increased serum bile acid levels could contribute to improvements in insulin sensitivity,incretin secretion,and postprandial glycaemia in response to bariatric surgery[6].Despite these intriguing data,it remains uncertain whether increased BA are absolutely essential for metabolic improvements following bariatric surgery,particularly during the early postoperative period.For example,longitudinal studies in humans demonstrate that increases in BA are not detecteduntil 1year postoperatively [73],despite improved glucose levels and reduced hepatic glucose output within one week of surgery [74,75].By contrast,increases in both fasting and postprandial BA are also observed as early as 14days following VSG in rodents [76,77].In addition,both RYGB and VSG can alter the distribution of bile acid species [8][78].By contrast,circulating BA do not change signi ficantly after LAGB [4,73,79],and could contribute to reduced ef ficacy of this procedure for long-term resolution of T2D as compared with RYGB or VSG.Importantly,increased BA action,via FXR-mediated effects on both gut microbiome and transcriptional pathways,appears to be required to achieve the metabolic effects of surgery,at least in rodent models [77].Intriguingly,direct modulation of small intestinal anatomy can alter bile acid levels and composi-tion.Mid-to-distal small intestinal resection,with preservation of the terminal ileum,increases bile acid levels [80].Similarly,interposition of the ileum into more proximal segments of gut also increases bile acid levels [81].Nonsurgical approaches may also be effective;for example,endoluminal sleeves,which allow luminal contents to bypass the duodenal mucosa,also increase bile acids in rodents and improve glucose metabolism [82].While there are many unanswered questions,these data suggest that modulation of segmental intestinal anatomy,and/or changes in intestinal flora which result from these modi fications,may improve systemic metabolism via BA-dependent mechanisms.Modulating bile acids as a potential therapeutic approach for obesity and T2DThe close links between plasma levels of bile acids and a host of key metabolic parameters raise the possibility that modulation of BA could be used as a therapeutic approach for the treatment of metabolic diseases.Dietary supplementation with cholic acid (CA)increases energy expenditure,reducing weight gain during high-fat feeding [83].While the precise mechanism remains uncertain,BA increase expression and activity of the type 2iodothyronine deiodinase (D2)via a TGR5-cAMP-mediated pathway,thus increasing active thyroid hormone (T3)availability and energy expenditure in tissues critical for thermogenesis,such as BAT [10].Similarly,CDCA has been shown to increase energy expenditure by induction of UCP1and activation of thermogenesis in BAT in mice [84].Bile acid sequestrants,which increase the CA pool size (while reducing CDCA and DCA pools),also reduce glucose,hemoglobin A1c,and cholesterol levels in patients with type 2diabetes [85e 87].Thus,therapeutic strategies which increase circulating BA levels or modulate relative distribution of active bile acid species could be an effective approach to improving systemic metabolism.Bile acid-binding resinsBile acid binding resins (BABR)(cholestyramine,colestipol,colestimide,and colesevelam)are positively charged nondigestible resins that bind to bile acids in the intestine to form an insoluble complex that is excreted in the feces.BABR have been successfully employed for the treatment of hypercholesterolaemia for many years.Recently,the second-generation BABR colestimide and cole-sevelam were found to improve glycaemic control in patients with T2D [86,88e 91].Several possible mechanisms have been proposed for these effects.BABR not only increase plasma BA but also change the composition of the BA pool [92].These changes in BA may promote increased energy expenditure,as observed in rodents [93].In addition,BABRs stimulate the expression of proglucagon and release of GLP1,thus improving incretin-mediated insulin secretion and reducing plasma glucose [94].This effect of colesevelam was achieved through regulation of FXR/FGF19and TGR5/GLP-1signalling pathways[89].Colestimide also decreases postprandial plasma glucose and increases GLP-1secretion in patients with T2D [85].Despite these intriguing possibilities,there is not a clear relationship between BA metabolism and improvements in glycaemia,and changes in FGF19or energy expenditure are not consistently observed in human studies [68,95].Nevertheless,BA sequestrants regulate glucose ho-meostasis,potentially at least in part by modulating FXR-and TGR5-mediated pathways.FXR agonistsSince FXR modulates many of the metabolic effects of bile acids,activation of FXR could be another approach to treat metabolic disease [96].Treatment with the FXR ligand GW4064signi ficantlyH.Ma,M.E.Patti /Best Practice &Research Clinical Gastroenterology 28(2014)573e 583578H.Ma,M.E.Patti/Best Practice&Research Clinical Gastroenterology28(2014)573e583579 decreases plasma glucose,triglycerides,and cholesterol in both wild-type and diabetic db/db mice [50].6-ECDCA,another FXR agonist,can decrease glucose,cholesterol,free fatty acid,and triglyceride levels in Zucker fa/fa rats[54]by enhancing glucose disposal,reducing body weight,in parallel with reduced expression of PEPCK and glucose-6-phosphates(G6Pase)in ob/ob mice.Interestingly,these effects of FXR agonists are achieved despite decreased bile acid levels,largely through direct activation of hepatic FXR-SHP and intestinal FXR-FGF15/19pathways[96].These data again highlight the importance of FXR pathways in mediating metabolic effects of BA.TGR5agonistsThe cell surface receptor TGR5represents another novel pharmacological target for the treat-ment of the metabolic syndrome and related disorders[97,98].TGR5can be activated by either synthetic ligands[10,80],or by BA in a dose-dependent manner,with potency of activation depending on specific BA species:lithocholic acid>deoxycholic acid>chenodeoxycholic acid >cholic acid.In rodents,synthetic TGR5agonists decrease plasma glucose and insulin levels and protect against weight gain induced by a high-fat diet[64].The expression and activity of the type2 deiodinase and energy expenditure can be increased with incubation of skeletal muscle with a synthetic TGR5agonist[10].Therapeutic modulation of BA via manipulation of the intestinal tract and its residentflora As noted above,bariatric surgery is associated with increased plasma BA levels and alterated composition of BA species.In turn,increased BA may contribute to enhanced FXR and TGR5acti-vation,leading to reduced hepatic glucose production,increased GLP1secretion,and increased systemic energy expenditure.In support of this concept,recent data implicate FXR activity as a critical mediator of the beneficial effects of bariatric surgery in rodents[85].Given the important role of the gut microbiome in modulating bile acid pool size,composition,and enterohepatic recirculation,it is intriguing to consider whether modification of the gut microbiome could pro-mote BA-mediated beneficial changes in metabolism.This could potentially be achieved by altered dietary fatty acid composition[99]or with probiotics which modulate gutflora[100,101].For example,one recent study demonstrated that probiotics could increase BA deconjugation,increase fecal BA excretion,and increase hepatic BA synthesis in an FGF-dependent mechanism[101].These intriguing data provide hope that BA action on the gut microbiome and systemic metabolism could be harnessed to yield beneficial effects similar to those observed after bariatric surgery,but in the absence of invasive surgery.This line of investigation will be an important question for future studies.SummaryTaken together,data from both humans and preclinical animal models demonstrate that BA are important signalling molecules which contribute to regulation of whole-body glucose and lipid metabolism and body weight.Such effects of BA are largely mediated by the nuclear receptor FXR and the G protein-coupled receptor TGR5.Future research employing proteomic,metabolomic,and lip-idomic approaches are likely to help in identifying bile acid-related biomarkers which may be useful for predicting and assessing response to BA-related therapy for human obesity and metabolic syn-drome.Moreover,novel approaches to altering biliaryflow and enterohepatic recirculation,plasma BA levels,composition of BA pools,and downstream effectors of BA signalling pathways should be pur-sued,as they may be effective strategies for the management of obesity,insulin resistance,type2 diabetes,and other components of the metabolic syndrome.Conflict of interestNone.AcknowledgementsThe authors gratefully acknowledge grant support from Hebei Health Department of Scienti fic Research Fund (to HM),P30DK036836DRC (Joslin Diabetes Center),and the American Diabetes As-sociation (to MEP).References[1]Houten SM,Watanabe M,Auwerx J.Endocrine functions of bile acids.EMBO J 2006;25:1419e 25.[2]Watanabe M,Houten SM,Mataki C,Christoffolete MA,Kim BW,Sato H,et al.Bile acids induce energy expenditure bypromoting intracellular thyroid hormone activation.Nature 2006;439:484e 9.[3]Chiang JY.Bile acid metabolism and pr Physiol 2013;3:1191e 212.[4]Kohli R,Bradley D,Setchell KD,Eagon JC,Abumrad N,Klein S.Weight loss induced by Roux-en-Y gastric bypass but notlaparoscopic adjustable gastric banding increases circulating bile acids.J Clin Endocrinol Metab 2013;98:E708e 12.[5]Simonen M,Dali-Youcef N,Kaminska D,Venesmaa S,Kakela P,Paakkonen M,et al.Conjugated bile acids associate withaltered rates of glucose and lipid oxidation after Roux-en-Y gastric bypass.Obes Surg 2012;22:1473e 80.[6]Patti ME,Houten SM,Bianco AC,Bernier R,Larsen PR,Holst JJ,et al.Serum bile acids are higher in humans with priorgastric bypass:potential contribution to improved glucose and lipid metabolism.Obesity 2009;17:1671e 7.[7]Chiang JY.Bile acids:regulation of synthesis.J Lipid Res 2009;50:1955e 66.[8]Chiang JY.Regulation of bile acid synthesis.Front Biosci 1998;3:d176e 93.[9]Deo AK,Bandiera SM.Biotransformation of lithocholic acid by rat hepatic microsomes:metabolite analysis by liquidchromatography/mass spectrometry.Drug Metab Dispos 2008;36:442e 51.[10]Russell DW.Fifty years of advances in bile acid synthesis and metabolism.J Lipid Res 2009;(Suppl.50):S120e 5.[11]Hofmann AF,Hagey LR.Bile acids:chemistry,pathochemistry,biology,pathobiology,and therapeutics.Cellular andmolecular life sciences.CMLS 2008;65:2461e 83.[12]Sayin SI,Wahlstrom A,Felin J,Jantti S,Marschall HU,Bamberg K,et al.Gut microbiota regulates bile acid metabolism byreducing the levels of tauro-beta-muricholic acid,a naturally occurring FXR antagonist.Cell Metab 2013;17:225e 35.[13]Moschetta A,Xu F,Hagey LR,van Berge-Henegouwen GP,van Erpecum KJ,Brouwers JF,et al.A phylogenetic survey ofbiliary lipids in vertebrates.J lipid Res 2005;46:2221e 32.[14]Hofmann AF,Borgstroem B.The intraluminal phase of fat digestion in man:the lipid content of the micellar and oilphases of intestinal content obtained during fat digestion and absorption.J Clin Invest 1964;43:247e 57.H.Ma,M.E.Patti /Best Practice &Research Clinical Gastroenterology 28(2014)573e 583580。
基于注意力融合网络的视频超分辨率重建
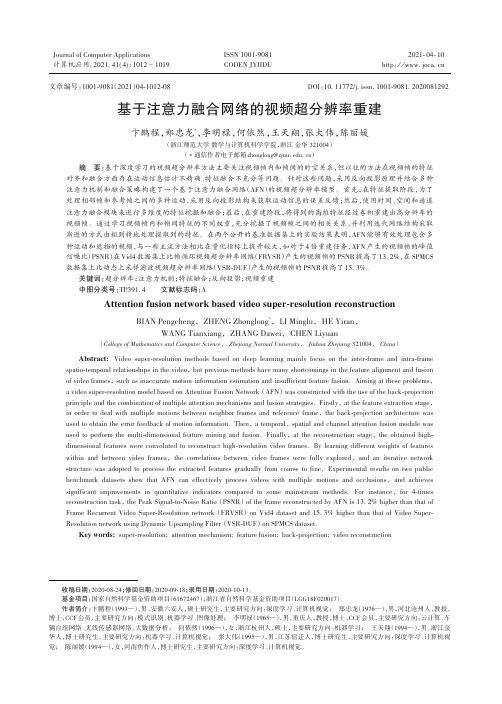
2021⁃04⁃10计算机应用,Journal of Computer Applications2021,41(4):1012-1019ISSN 1001⁃9081CODEN JYIIDU http ://基于注意力融合网络的视频超分辨率重建卞鹏程,郑忠龙*,李明禄,何依然,王天翔,张大伟,陈丽媛(浙江师范大学数学与计算机科学学院,浙江金华321004)(∗通信作者电子邮箱zhonglong@ )摘要:基于深度学习的视频超分辨率方法主要关注视频帧内和帧间的时空关系,但以往的方法在视频帧的特征对齐和融合方面存在运动信息估计不精确、特征融合不充分等问题。
针对这些问题,采用反向投影原理并结合多种注意力机制和融合策略构建了一个基于注意力融合网络(AFN )的视频超分辨率模型。
首先,在特征提取阶段,为了处理相邻帧和参考帧之间的多种运动,采用反向投影结构来获取运动信息的误差反馈;然后,使用时间、空间和通道注意力融合模块来进行多维度的特征挖掘和融合;最后,在重建阶段,将得到的高维特征经过卷积重建出高分辨率的视频帧。
通过学习视频帧内和帧间特征的不同权重,充分挖掘了视频帧之间的相关关系,并利用迭代网络结构采取渐进的方式由粗到精地处理提取到的特征。
在两个公开的基准数据集上的实验结果表明,AFN 能够有效处理包含多种运动和遮挡的视频,与一些主流方法相比在量化指标上提升较大,如对于4倍重建任务,AFN 产生的视频帧的峰值信噪比(PSNR )在Vid4数据集上比帧循环视频超分辨率网络(FRVSR )产生的视频帧的PSNR 提高了13.2%,在SPMCS 数据集上比动态上采样滤波视频超分辨率网络(VSR -DUF )产生的视频帧的PSNR 提高了15.3%。
关键词:超分辨率;注意力机制;特征融合;反向投影;视频重建中图分类号:TP391.4文献标志码:AAttention fusion network based video super -resolution reconstructionBIAN Pengcheng ,ZHENG Zhonglong *,LI Minglu ,HE Yiran ,WANG Tianxiang ,ZHANG Dawei ,CHEN Liyuan(College of Mathematics and Computer Science ,Zhejiang Normal University ,Jinhua Zhejiang 321004,China )Abstract:Video super -resolution methods based on deep learning mainly focus on the inter -frame and intra -frame spatio -temporal relationships in the video ,but previous methods have many shortcomings in the feature alignment and fusion of video frames ,such as inaccurate motion information estimation and insufficient feature fusion.Aiming at these problems ,a video super -resolution model based on Attention Fusion Network (AFN )was constructed with the use of the back -projection principle and the combination of multiple attention mechanisms and fusion strategies.Firstly ,at the feature extraction stage ,in order to deal with multiple motions between neighbor frames and reference frame ,the back -projection architecture was used to obtain the error feedback of motion information.Then ,a temporal ,spatial and channel attention fusion module was used to perform the multi -dimensional feature mining and fusion.Finally ,at the reconstruction stage ,the obtained high -dimensional features were convoluted to reconstruct high -resolution video frames.By learning different weights of features within and between video frames ,the correlations between video frames were fully explored ,and an iterative network structure was adopted to process the extracted features gradually from coarse to fine.Experimental results on two public benchmark datasets show that AFN can effectively process videos with multiple motions and occlusions ,and achieves significant improvements in quantitative indicators compared to some mainstream methods.For instance ,for 4-times reconstruction task ,the Peak Signal -to -Noise Ratio (PSNR )of the frame reconstructed by AFN is 13.2%higher than that of Frame Recurrent Video Super -Resolution network (FRVSR )on Vid4dataset and 15.3%higher than that of Video Super -Resolution network using Dynamic Upsampling Filter (VSR -DUF )on SPMCS dataset.Key words:super -resolution;attention mechanism;feature fusion;back -projection;video reconstruction文章编号:1001-9081(2021)04-1012-08DOI :10.11772/j.issn.1001-9081.2020081292收稿日期:2020⁃08⁃24;修回日期:2020⁃09⁃18;录用日期:2020⁃10⁃13。
Empirical processes of dependent random variables
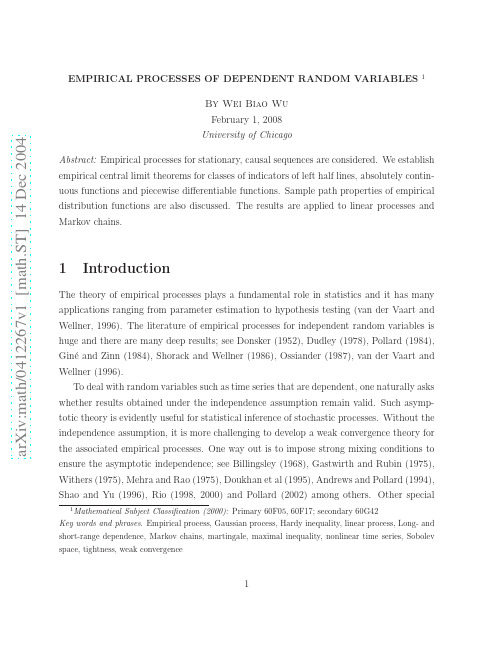
2
Preliminaries
n i=1
from R to R. The centered G -indexed empirical process is given by (P n − P )g = 1 n
n
the marginal and empirical distribution functions. Let G be a class of measurabrocesses that have been discussed include linear processes and Gaussian processes; see Dehling and Taqqu (1989) and Cs¨ org˝ o and Mielniczuk (1996) for long and short-range dependent subordinated Gaussian processes and Ho and Hsing (1996) and Wu (2003a) for long-range dependent linear processes. A collection of recent results is presented in Dehling, Mikosch and Sorensen (2002). In that collection Dedecker and Louhichi (2002) made an important generalization of Ossiander’s (1987) result. Here we investigate the empirical central limit problem for dependent random variables from another angle that avoids strong mixing conditions. In particular, we apply a martingale method and establish a weak convergence theory for stationary, causal processes. Our results are comparable with the theory for independent random variables in that the imposed moment conditions are optimal or almost optimal. We show that, if the process is short-range dependent in a certain sense, then the limiting behavior is similar to that of iid random variables in that the limiting distribution is a Gaussian process and the norming √ sequence is n. For long-range dependent linear processes, one needs to apply asymptotic √ expansions to obtain n-norming limit theorems (Section 6.2.2). The paper is structured as follows. In Section 2 we introduce some mathematical preliminaries necessary for the weak convergence theory and illustrate the essence of our approach. Two types of empirical central limit theorems are established. Empirical processes indexed by indicators of left half lines, absolutely continuous functions, and piecewise differentiable functions are discussed in Sections 3, 4 and 5 respectively. Applications to linear processes and iterated random functions are made in Section 6. Section 7 presents some integral and maximal inequalities that may be of independent interest. Some proofs are given in Sections 8 and 9.
Secrets of Optical Flow Estimation and Their Principles
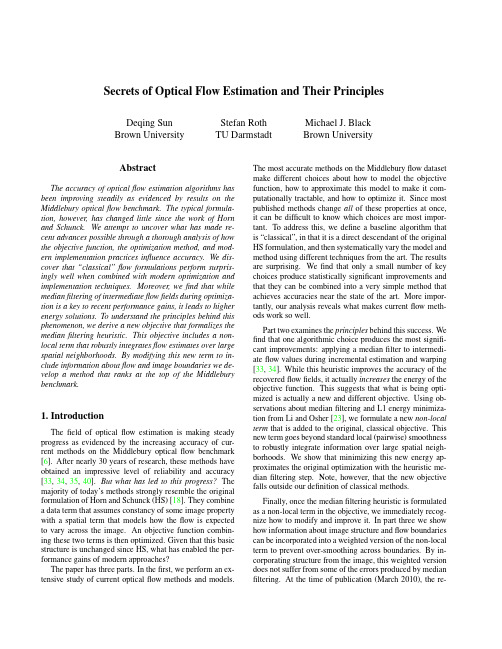
Secrets of Optical Flow Estimation and Their PrinciplesDeqing Sun Brown UniversityStefan RothTU DarmstadtMichael J.BlackBrown UniversityAbstractThe accuracy of opticalflow estimation algorithms has been improving steadily as evidenced by results on the Middlebury opticalflow benchmark.The typical formula-tion,however,has changed little since the work of Horn and Schunck.We attempt to uncover what has made re-cent advances possible through a thorough analysis of how the objective function,the optimization method,and mod-ern implementation practices influence accuracy.We dis-cover that“classical”flow formulations perform surpris-ingly well when combined with modern optimization and implementation techniques.Moreover,wefind that while medianfiltering of intermediateflowfields during optimiza-tion is a key to recent performance gains,it leads to higher energy solutions.To understand the principles behind this phenomenon,we derive a new objective that formalizes the medianfiltering heuristic.This objective includes a non-local term that robustly integratesflow estimates over large spatial neighborhoods.By modifying this new term to in-clude information aboutflow and image boundaries we de-velop a method that ranks at the top of the Middlebury benchmark.1.IntroductionThefield of opticalflow estimation is making steady progress as evidenced by the increasing accuracy of cur-rent methods on the Middlebury opticalflow benchmark [6].After nearly30years of research,these methods have obtained an impressive level of reliability and accuracy [33,34,35,40].But what has led to this progress?The majority of today’s methods strongly resemble the original formulation of Horn and Schunck(HS)[18].They combine a data term that assumes constancy of some image property with a spatial term that models how theflow is expected to vary across the image.An objective function combin-ing these two terms is then optimized.Given that this basic structure is unchanged since HS,what has enabled the per-formance gains of modern approaches?The paper has three parts.In thefirst,we perform an ex-tensive study of current opticalflow methods and models.The most accurate methods on the Middleburyflow dataset make different choices about how to model the objective function,how to approximate this model to make it com-putationally tractable,and how to optimize it.Since most published methods change all of these properties at once, it can be difficult to know which choices are most impor-tant.To address this,we define a baseline algorithm that is“classical”,in that it is a direct descendant of the original HS formulation,and then systematically vary the model and method using different techniques from the art.The results are surprising.Wefind that only a small number of key choices produce statistically significant improvements and that they can be combined into a very simple method that achieves accuracies near the state of the art.More impor-tantly,our analysis reveals what makes currentflow meth-ods work so well.Part two examines the principles behind this success.We find that one algorithmic choice produces the most signifi-cant improvements:applying a medianfilter to intermedi-ateflow values during incremental estimation and warping [33,34].While this heuristic improves the accuracy of the recoveredflowfields,it actually increases the energy of the objective function.This suggests that what is being opti-mized is actually a new and different ing ob-servations about medianfiltering and L1energy minimiza-tion from Li and Osher[23],we formulate a new non-local term that is added to the original,classical objective.This new term goes beyond standard local(pairwise)smoothness to robustly integrate information over large spatial neigh-borhoods.We show that minimizing this new energy ap-proximates the original optimization with the heuristic me-dianfiltering step.Note,however,that the new objective falls outside our definition of classical methods.Finally,once the medianfiltering heuristic is formulated as a non-local term in the objective,we immediately recog-nize how to modify and improve it.In part three we show how information about image structure andflow boundaries can be incorporated into a weighted version of the non-local term to prevent over-smoothing across boundaries.By in-corporating structure from the image,this weighted version does not suffer from some of the errors produced by median filtering.At the time of publication(March2010),the re-sulting approach is ranked1st in both angular and end-point errors in the Middlebury evaluation.In summary,the contributions of this paper are to(1)an-alyze currentflow models and methods to understand which design choices matter;(2)formulate and compare several classical objectives descended from HS using modern meth-ods;(3)formalize one of the key heuristics and derive a new objective function that includes a non-local term;(4)mod-ify this new objective to produce a state-of-the-art method. In doing this,we provide a“recipe”for others studying op-ticalflow that can guide their design choices.Finally,to en-able comparison and further innovation,we provide a public M ATLAB implementation[1].2.Previous WorkIt is important to separately analyze the contributions of the objective function that defines the problem(the model) and the optimization algorithm and implementation used to minimize it(the method).The HS formulation,for example, has long been thought to be highly inaccurate.Barron et al.[7]reported an average angular error(AAE)of~30degrees on the“Yosemite”sequence.This confounds the objective function with the particular optimization method proposed by Horn and Schunck1.When optimized with today’s meth-ods,the HS objective achieves surprisingly competitive re-sults despite the expected over-smoothing and sensitivity to outliers.Models:The global formulation of opticalflow intro-duced by Horn and Schunck[18]relies on both brightness constancy and spatial smoothness assumptions,but suffers from the fact that the quadratic formulation is not robust to outliers.Black and Anandan[10]addressed this by re-placing the quadratic error function with a robust formula-tion.Subsequently,many different robust functions have been explored[12,22,31]and it remains unclear which is best.We refer to all these spatially-discrete formulations derived from HS as“classical.”We systematically explore variations in the formulation and optimization of these ap-proaches.The surprise is that the classical model,appropri-ately implemented,remains very competitive.There are many formulations beyond the classical ones that we do not consider here.Significant ones use oriented smoothness[25,31,33,40],rigidity constraints[32,33], or image segmentation[9,21,41,37].While they deserve similar careful consideration,we expect many of our con-clusions to carry forward.Note that one can select among a set of models for a given sequence[4],instead offinding a “best”model for all the sequences.Methods:Many of the implementation details that are thought to be important date back to the early days of op-1They noted that the correct way to optimize their objective is by solv-ing a system of linear equations as is common today.This was impractical on the computers of the day so they used a heuristic method.ticalflow.Current best practices include coarse-to-fine es-timation to deal with large motions[8,13],texture decom-position[32,34]or high-orderfilter constancy[3,12,16, 22,40]to reduce the influence of lighting changes,bicubic interpolation-based warping[22,34],temporal averaging of image derivatives[17,34],graduated non-convexity[11]to minimize non-convex energies[10,31],and medianfilter-ing after each incremental estimation step to remove outliers [34].This medianfiltering heuristic is of particular interest as it makes non-robust methods more robust and improves the accuracy of all methods we tested.The effect on the objec-tive function and the underlying reason for its success have not previously been analyzed.Least median squares estima-tion can be used to robustly reject outliers inflow estimation [5],but previous work has focused on the data term.Related to medianfiltering,and our new non-local term, is the use of bilateralfiltering to prevent smoothing across motion boundaries[36].The approach separates a varia-tional method into twofiltering update stages,and replaces the original anisotropic diffusion process with multi-cue driven bilateralfiltering.As with medianfiltering,the bi-lateralfiltering step changes the original energy function.Models that are formulated with an L1robust penalty are often coupled with specialized total variation(TV)op-timization methods[39].Here we focus on generic opti-mization methods that can apply to any model andfind they perform as well as reported results for specialized methods.Despite recent algorithmic advances,there is a lack of publicly available,easy to use,and accurateflow estimation software.The GPU4Vision project[2]has made a substan-tial effort to change this and provides executablefiles for several accurate methods[32,33,34,35].The dependence on the GPU and the lack of source code are limitations.We hope that our public M ATLAB code will not only help in un-derstanding the“secrets”of opticalflow,but also let others exploit opticalflow as a useful tool in computer vision and relatedfields.3.Classical ModelsWe write the“classical”opticalflow objective function in its spatially discrete form asE(u,v)=∑i,j{ρD(I1(i,j)−I2(i+u i,j,j+v i,j))(1)+λ[ρS(u i,j−u i+1,j)+ρS(u i,j−u i,j+1)+ρS(v i,j−v i+1,j)+ρS(v i,j−v i,j+1)]}, where u and v are the horizontal and vertical components of the opticalflowfield to be estimated from images I1and I2,λis a regularization parameter,andρD andρS are the data and spatial penalty functions.We consider three different penalty functions:(1)the quadratic HS penaltyρ(x)=x2;(2)the Charbonnier penaltyρ(x)=√x2+ 2[13],a dif-ferentiable variant of the L1norm,the most robust convexfunction;and(3)the Lorentzianρ(x)=log(1+x22σ2),whichis a non-convex robust penalty used in[10].Note that this classical model is related to a standard pairwise Markov randomfield(MRF)based on a4-neighborhood.In the remainder of this section we define a baseline method using several techniques from the literature.This is not the“best”method,but includes modern techniques and will be used for comparison.We only briefly describe the main choices,which are explored in more detail in the following section and the cited references,especially[30].Quantitative results are presented throughout the remain-der of the text.In all cases we report the average end-point error(EPE)on the Middlebury training and test sets,de-pending on the experiment.Given the extensive nature of the evaluation,only average results are presented in the main body,while the details for each individual sequence are given in[30].3.1.Baseline methodsTo gain robustness against lighting changes,we follow [34]and apply the Rudin-Osher-Fatemi(ROF)structure texture decomposition method[28]to pre-process the in-put sequences and linearly combine the texture and struc-ture components(in the proportion20:1).The parameters are set according to[34].Optimization is performed using a standard incremental multi-resolution technique(e.g.[10,13])to estimateflow fields with large displacements.The opticalflow estimated at a coarse level is used to warp the second image toward thefirst at the nextfiner level,and aflow increment is cal-culated between thefirst image and the warped second im-age.The standard deviation of the Gaussian anti-aliasingfilter is set to be1√2d ,where d denotes the downsamplingfactor.Each level is recursively downsampled from its near-est lower level.In building the pyramid,the downsampling factor is not critical as pointed out in the next section and here we use the settings in[31],which uses a factor of0.8 in thefinal stages of the optimization.We adaptively de-termine the number of pyramid levels so that the top level has a width or height of around20to30pixels.At each pyramid level,we perform10warping steps to compute the flow increment.At each warping step,we linearize the data term,whichinvolves computing terms of the type∂∂x I2(i+u k i,j,j+v k i,j),where∂/∂x denotes the partial derivative in the horizon-tal direction,u k and v k denote the currentflow estimate at iteration k.As suggested in[34],we compute the deriva-tives of the second image using the5-point derivativefilter1 12[−180−81],and warp the second image and its deriva-tives toward thefirst using the currentflow estimate by bicu-bic interpolation.We then compute the spatial derivatives ofAvg.Rank Avg.EPEClassic-C14.90.408HS24.60.501Classic-L19.80.530HS[31]35.10.872BA(Classic-L)[31]30.90.746Adaptive[33]11.50.401Complementary OF[40]10.10.485Table1.Models.Average rank and end-point error(EPE)on the Middlebury test set using different penalty functions.Two current methods are included for comparison.thefirst image,average with the warped derivatives of the second image(c.f.[17]),and use this in place of∂I2∂x.For pixels moving out of the image boundaries,we set both their corresponding temporal and spatial derivatives to zero.Af-ter each warping step,we apply a5×5medianfilter to the newly computedflowfield to remove outliers[34].For the Charbonnier(Classic-C)and Lorentzian (Classic-L)penalty function,we use a graduated non-convexity(GNC)scheme[11]as described in[31]that lin-early combines a quadratic objective with a robust objective in varying proportions,from fully quadratic to fully robust. Unlike[31],a single regularization weightλis used for both the quadratic and the robust objective functions.3.2.Baseline resultsThe regularization parameterλis selected among a set of candidate values to achieve the best average end-point error (EPE)on the Middlebury training set.For the Charbonnier penalty function,the candidate set is[1,3,5,8,10]and 5is optimal.The Charbonnier penalty uses =0.001for both the data and the spatial term in Eq.(1).The Lorentzian usesσ=1.5for the data term,andσ=0.03for the spa-tial term.These parameters arefixed throughout the exper-iments,except where mentioned.Table1summarizes the EPE results of the basic model with three different penalty functions on the Middlebury test set,along with the two top performers at the time of publication(considering only published papers).The clas-sic formulations with two non-quadratic penalty functions (Classic-C)and(Classic-L)achieve competitive results de-spite their simplicity.The baseline optimization of HS and BA(Classic-L)results in significantly better accuracy than previously reported for these models[31].Note that the analysis also holds for the training set(Table2).At the time of publication,Classic-C ranks13th in av-erage EPE and15th in AAE in the Middlebury benchmark despite its simplicity,and it serves as the baseline below.It is worth noting that the spatially discrete MRF formulation taken here is competitive with variational methods such as [33].Moreover,our baseline implementation of HS has a lower average EPE than many more sophisticated methods.Avg.EPE significance p-value Classic-C0.298——HS0.38410.0078Classic-L0.31910.0078Classic-C-brightness0.28800.9453HS-brightness0.38710.0078Classic-L-brightness0.32500.2969Gradient0.30500.4609Table2.Pre-Processing.Average end-point error(EPE)on the Middlebury training set for the baseline method(Classic-C)using different pre-processing techniques.Significance is always with respect to Classic-C.4.Secrets ExploredWe evaluate a range of variations from the baseline ap-proach that have appeared in the literature,in order to illu-minate which may be of importance.This analysis is per-formed on the Middlebury training set by changing only one property at a time.Statistical significance is determined using a Wilcoxon signed rank test between each modified method and the baseline Classic-C;a p value less than0.05 indicates a significant difference.Pre-Processing.For each method,we optimize the regu-larization parameterλfor the training sequences and report the results in Table2.The baseline uses a non-linear pre-filtering of the images to reduce the influence of illumina-tion changes[34].Table2shows the effect of removing this and using a standard brightness constancy model(*-brightness).Classic-C-brightness actually achieves lower EPE on the training set than Classic-C but significantly lower accuracy on the test set:Classic-C-brightness= 0.726,HS-brightness=0.759,and Classic-L-brightness =0.603–see Table1for comparison.This disparity sug-gests overfitting is more severe for the brightness constancy assumption.Gradient only imposes constancy of the gra-dient vector at each pixel as proposed in[12](i.e.it robustly penalizes Euclidean distance between image gradients)and has similar performance in both training and test sets(c.f. Table8).See[30]for results of more alternatives. Secrets:Some form of imagefiltering is useful but simple derivative constancy is nearly as good as the more sophisti-cated texture decomposition method.Coarse-to-fine estimation and GNC.We vary the number of warping steps per pyramid level andfind that3warping steps gives similar results as using10(Table3).For the GNC scheme,[31]uses a downsampling factor of0.8for non-convex optimization.A downsampling factor of0.5 (Down-0.5),however,has nearly identical performance Removing the GNC step for the Charbonnier penalty function(w/o GNC)results in higher EPE on most se-quences and higher energy on all sequences(Table4).This suggests that the GNC method is helpful even for the con-vex Charbonnier penalty function due to the nonlinearity ofAvg.EPE significance p-value Classic-C0.298——3warping steps0.30400.9688Down-0.50.2980 1.0000w/o GNC0.35400.1094Bilinear0.30200.1016w/o TA VG0.30600.1562Central derivativefilter0.30000.72667-point derivativefilter[13]0.30200.3125Bicubic-II0.29010.0391GC-0.45(λ=3)0.29210.0156GC-0.25(λ=0.7)0.2980 1.0000MF3×30.30500.1016MF7×70.30500.56252×MF0.3000 1.00005×MF0.30500.6875w/o MF0.35210.0078Classic++0.28510.0078 Table3.Model and Methods.Average end-point error(EPE)on the Middlebury training set for the baseline method(Classic-C) using different algorithm and modelingchoices.Figure1.Different penalty functions for the spatial terms:Char-bonnier( =0.001),generalized Charbonnier(a=0.45and a=0.25),and Lorentzian(σ=0.03).the data term.Secrets:The downsampling factor does not matter when using a convex penalty;a standard factor of0.5isfine. Some form of GNC is useful even for a convex robust penalty like Charbonnier because of the nonlinear data term. Interpolation method and derivatives.Wefind that bicu-bic interpolation is more accurate than bilinear(Table3, Bilinear),as already reported in previous work[34].Re-moving temporal averaging of the gradients(w/o TA VG), using Central differencefilters,or using a7-point deriva-tivefilter[13]all reduce accuracy compared to the base-line,but not significantly.The M ATLAB built-in function interp2is based on cubic convolution approximation[20]. The spline-based interpolation scheme[26]is consistently better(Bicubic-II).See[30]for more discussions. Secrets:Use spline-based bicubic interpolation with a5-pointfilter.Temporal averaging of the derivatives is proba-bly worthwhile for a small computational expense. Penalty functions.Wefind that the convex Charbonnier penalty performs better than the more robust,non-convex Lorentzian on both the training and test sets.One reason might be that non-convex functions are more difficult to op-timize,causing the optimization scheme tofind a poor local(a)With medianfiltering(b)Without medianfilteringFigure2.Estimatedflowfields on sequence“RubberWhale”using Classic-C with and without(w/o MF)the medianfiltering step. Color coding as in[6].(a)(w/MF)energy502,387and(b)(w/o MF)energy449,290.The medianfiltering step helps reach a so-lution free from outliers but with a higher energy.optimum.We investigate a generalized Charbonnier penalty functionρ(x)=(x2+ 2)a that is equal to the Charbon-nier penalty when a=0.5,and non-convex when a<0.5 (see Figure1).We optimize the regularization parameterλagain.Wefind a slightly non-convex penalty with a=0.45 (GC-0.45)performs consistently better than the Charbon-nier penalty,whereas more non-convex penalties(GC-0.25 with a=0.25)show no improvement.Secrets:The less-robust Charbonnier is preferable to the Lorentzian and a slightly non-convex penalty function(GC-0.45)is better still.Medianfiltering.The baseline5×5medianfilter(MF 5×5)is better than both MF3×3[34]and MF7×7but the difference is not significant(Table3).When we perform5×5medianfiltering twice(2×MF)orfive times(5×MF)per warping step,the results are worse.Finally,removing the medianfiltering step(w/o MF)makes the computedflow significantly less accurate with larger outliers as shown in Table3and Figure2.Secrets:Medianfiltering the intermediateflow results once after every warping iteration is the single most important secret;5×5is a goodfilter size.4.1.Best PracticesCombining the analysis above into a single approach means modifying the baseline to use the slightly non-convex generalized Charbonnier and the spline-based bicu-bic interpolation.This leads to a statistically significant improvement over the baseline(Table3,Classic++).This method is directly descended from HS and BA,yet updated with the current best optimization practices known to us. This simple method ranks9th in EPE and12th in AAE on the Middlebury test set.5.Models Underlying Median FilteringOur analysis reveals the practical importance of median filtering during optimization to denoise theflowfield.We ask whether there is a principle underlying this heuristic?One interesting observation is thatflowfields obtained with medianfiltering have substantially higher energy than those without(Table4and Figure2).If the medianfilter is helping to optimize the objective,it should lead to lower energies.Higher energies and more accurate estimates sug-gest that incorporating medianfiltering changes the objec-tive function being optimized.The insight that follows from this is that the medianfil-tering heuristic is related to the minimization of an objective function that differs from the classical one.In particular the optimization of Eq.(1),with interleaved medianfiltering, approximately minimizesE A(u,v,ˆu,ˆv)=(2)∑i,j{ρD(I1(i,j)−I2(i+u i,j,j+v i,j))+λ[ρS(u i,j−u i+1,j)+ρS(u i,j−u i,j+1)+ρS(v i,j−v i+1,j)+ρS(v i,j−v i,j+1)]}+λ2(||u−ˆu||2+||v−ˆv||2)+∑i,j∑(i ,j )∈N i,jλ3(|ˆu i,j−ˆu i ,j |+|ˆv i,j−ˆv i ,j |),whereˆu andˆv denote an auxiliaryflowfield,N i,j is the set of neighbors of pixel(i,j)in a possibly large area andλ2 andλ3are scalar weights.The term in braces is the same as theflow energy from Eq.(1),while the last term is new. This non-local term[14,15]imposes a particular smooth-ness assumption within a specified region of the auxiliary flowfieldˆu,ˆv2.Here we take this term to be a5×5rectan-gular region to match the size of the medianfilter in Classic-C.A third(coupling)term encouragesˆu,ˆv and u,v to be the same(c.f.[33,39]).The connection to medianfiltering(as a denoising method)derives from the fact that there is a direct relation-ship between the median and L1minimization.Consider a simplified version of Eq.(2)with just the coupling and non-local terms,where E(ˆu)=λ2||u−ˆu||2+∑i,j∑(i ,j )∈N i,jλ3|ˆu i,j−ˆu i ,j |.(3)While minimizing this is similar to medianfiltering u,there are two differences.First,the non-local term minimizes the L1distance between the central value and allflow values in its neighborhood except itself.Second,Eq.(3)incorpo-rates information about the data term through the coupling equation;medianfiltering theflow ignores the data term.The formal connection between Eq.(3)and medianfil-tering3is provided by Li and Osher[23]who show that min-2Bruhn et al.[13]also integrated information over a local region in a global method but did so for the data term.3Hsiao et al.[19]established the connection in a slightly different way.Classic-C 0.5890.7480.8660.502 1.816 2.317 1.126 1.424w/o GNC 0.5930.7500.8700.506 1.845 2.518 1.142 1.465w/o MF0.5170.7010.6680.449 1.418 1.830 1.066 1.395Table 4.Eq.(1)energy (×106)for the optical flow fields computed on the Middlebury training set .Note that Classic-C uses graduated non-convexity (GNC),which reduces the energy,and median filtering,which increases it.imizing Eq.(3)is related to a different median computationˆu (k +1)i,j=median (Neighbors (k )∪Data)(4)where Neighbors (k )={ˆu (k )i ,j }for (i ,j )∈N i,j and ˆu (0)=u as well as Data ={u i,j ,u i,j ±λ3λ2,u i,j±2λ3λ2···,u i,j ±|N i,j |λ32λ2},where |N i,j |denotes the (even)number of neighbors of (i,j ).Note that the set of “data”values is balanced with an equal number of elements on either side of the value u i,j and that information about the data term is included through u i,j .Repeated application of Eq.(4)converges rapidly [23].Observe that,as λ3/λ2increases,the weighted data val-ues on either side of u i,j move away from the values of Neighbors and cancel each other out.As this happens,Eq.(4)approximates the median at the first iterationˆu (1)i,j ≈median (Neighbors (0)∪{u i,j }).(5)Eq.(2)thus combines the original objective with an ap-proximation to the median,the influence of which is con-trolled by λ3/λ2.Note in practice the weight λ2on thecoupling term is usually small or is steadily increased from small values [34,39].We optimize the new objective (2)by alternately minimizingE O (u ,v )=∑i,jρD (I 1(i,j )−I 2(i +u i,j ,j +v i,j ))+λ[ρS (u i,j −u i +1,j )+ρS (u i,j −u i,j +1)+ρS (v i,j −v i +1,j )+ρS (v i,j −v i,j +1)]+λ2(||u −ˆu ||2+||v −ˆv ||2)(6)andE M (ˆu ,ˆv )=λ2(||u −ˆu ||2+||v −ˆv ||2)(7)+∑i,j ∑(i ,j )∈N i,jλ3(|ˆu i,j −ˆu i ,j |+|ˆv i,j −ˆv i ,j |).Note that an alternative formulation would drop the cou-pling term and impose the non-local term directly on u and v .We find that optimization of the coupled set of equations is superior in terms of EPE performance.The alternating optimization strategy first holds ˆu ,ˆv fixed and minimizes Eq.(6)w.r.t.u ,v .Then,with u ,v fixed,we minimize Eq.(7)w.r.t.ˆu ,ˆv .Note that Eqs.(3)andAvg.EPE significancep -value Classic-C0.298——Classic-C-A0.30500.8125Table 5.Average end-point error (EPE)on the Middlebury train-ing set is shown for the new model with alternating optimization (Classic-C-A ).(7)can be minimized by repeated application of Eq.(4);weuse this approach with 5iterations.We perform 10steps of alternating optimizations at every pyramid level and change λ2logarithmically from 10−4to 102.During the first and second GNC stages,we set u ,v to be ˆu ,ˆv after every warp-ing step (this step helps reach solutions with lower energy and EPE [30]).In the end,we take ˆu ,ˆv as the final flow field estimate.The other parameters are λ=5,λ3=1.Alternatingly optimizing this new objective function (Classic-C-A )leads to similar results as the baseline Classic-C (Table 5).We also compare the energy of these solutions using the new objective and find the alternat-ing optimization produces the lowest energy solutions,as shown in Table 6.To do so,we set both the flow field u ,v and the auxiliary flow field ˆu ,ˆv to be the same in Eq.(2).In summary,we show that the heuristic median filter-ing step in Classic-C can now be viewed as energy min-imization of a new objective with a non-local term.The explicit formulation emphasizes the value of robustly inte-grating information over large neighborhoods and enables the improved model described below.6.Improved ModelBy formalizing the median filtering heuristic as an ex-plicit objective function,we can find ways to improve it.While median filtering in a large neighborhood has advan-tages as we have seen,it also has problems.A neighborhood centered on a corner or thin structure is dominated by the surround and computing the median results in oversmooth-ing as illustrated in Figure 3(a).Examining the non-local term suggests a solution.For a given pixel,if we know which other pixels in the area be-long to the same surface,we can weight them more highly.The modification to the objective function is achieved by introducing a weight into the non-local term [14,15]:∑i,j ∑(i ,j )∈N i,jw i,j,i ,j (|ˆu i,j −ˆu i ,j |+|ˆv i,j −ˆv i ,j |),(8)where w i,j,i ,j represents how likely pixel i ,j is to belongto the same surface as i,j .。
双三次插值(bicubic interpolation)原理及MATLAB源码实现
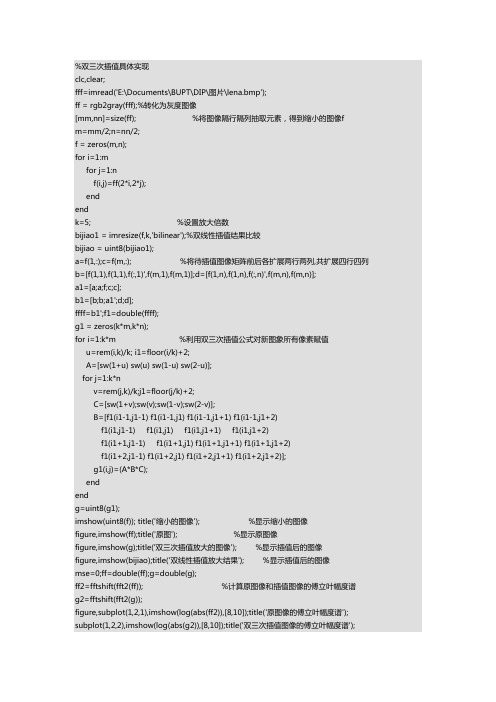
%双三次插值具体实现clc,clear;fff=imread('E:\Documents\BUPT\DIP\图片\lena.bmp');ff = rgb2gray(fff);%转化为灰度图像[mm,nn]=size(ff); %将图像隔行隔列抽取元素,得到缩小的图像fm=mm/2;n=nn/2;f = zeros(m,n);for i=1:mfor j=1:nf(i,j)=ff(2*i,2*j);endendk=5; %设置放大倍数bijiao1 = imresize(f,k,'bilinear');%双线性插值结果比较bijiao = uint8(bijiao1);a=f(1,:);c=f(m,:); %将待插值图像矩阵前后各扩展两行两列,共扩展四行四列b=[f(1,1),f(1,1),f(:,1)',f(m,1),f(m,1)];d=[f(1,n),f(1,n),f(:,n)',f(m,n),f(m,n)];a1=[a;a;f;c;c];b1=[b;b;a1';d;d];ffff=b1';f1=double(ffff);g1 = zeros(k*m,k*n);for i=1:k*m %利用双三次插值公式对新图象所有像素赋值u=rem(i,k)/k; i1=floor(i/k)+2;A=[sw(1+u) sw(u) sw(1-u) sw(2-u)];for j=1:k*nv=rem(j,k)/k;j1=floor(j/k)+2;C=[sw(1+v);sw(v);sw(1-v);sw(2-v)];B=[f1(i1-1,j1-1) f1(i1-1,j1) f1(i1-1,j1+1) f1(i1-1,j1+2)f1(i1,j1-1) f1(i1,j1) f1(i1,j1+1) f1(i1,j1+2)f1(i1+1,j1-1) f1(i1+1,j1) f1(i1+1,j1+1) f1(i1+1,j1+2)f1(i1+2,j1-1) f1(i1+2,j1) f1(i1+2,j1+1) f1(i1+2,j1+2)];g1(i,j)=(A*B*C);endendg=uint8(g1);imshow(uint8(f)); title('缩小的图像'); %显示缩小的图像figure,imshow(ff);title('原图'); %显示原图像figure,imshow(g);title('双三次插值放大的图像'); %显示插值后的图像figure,imshow(bijiao);title('双线性插值放大结果'); %显示插值后的图像mse=0;ff=double(ff);g=double(g);ff2=fftshift(fft2(ff)); %计算原图像和插值图像的傅立叶幅度谱g2=fftshift(fft2(g));figure,subplot(1,2,1),imshow(log(abs(ff2)),[8,10]);title('原图像的傅立叶幅度谱'); subplot(1,2,2),imshow(log(abs(g2)),[8,10]);title('双三次插值图像的傅立叶幅度谱');基函数代码:function A=sw(w1)w=abs(w1);if w<1&&w>=0A=1-2*w^2+w^3;elseif w>=1&&w<2A=4-8*w+5*w^2-w^3;elseA=0;end算法原理双三次插值又称立方卷积插值。
R语言 不规则数据的线性或三次样条插值

Package‘akima’September19,2011Version0.5-4Date2009-06-10Title Interpolation of irregularly spaced dataAuthor Fortran code by H.Akima R port by Albrecht Gebhardt<albrecht.gebhardt@uni-klu.ac.at>aspline function by ThomasPetzoldt<petzoldt@rcs.urz.tu-dresden.de>enhancements andcorrections by Martin Maechler<maechler@stat.math.ethz.ch>Maintainer Albrecht Gebhardt<albrecht.gebhardt@uni-klu.ac.at>Description Linear or cubic spline interpolation for irregular gridded dataLicensefile LICENSERepository CRANDate/Publication2009-11-0413:09:46R topics documented:akima (2)aspline (3)interp (5)interpp (8)Index1112akima akima Waveform Distortion Data for Bivariate InterpolationDescriptionakima is a list with components x,y and z which represents a smooth surface of z values at selected points irregularly distributed in the x-y plane.The data was taken from a study of waveform distortion in electronic circuits,described in:Hi-roshi Akima,"A Method of Bivariate Interpolation and Smooth Surface Fitting Based on Local Procedures",CACM,V ol.17,No.1,January1974,pp.18-20.ReferencesHiroshi Akima,"A Method of Bivariate Interpolation and Smooth Surface Fitting for Irregularly Distributed Data Points",ACM Transactions on Mathematical Software,V ol.4,No.2,June1978, pp.148-159.Copyright1978,Association for Computing Machinery,Inc.,reprinted by permission. Examples##Not run:library(rgl)data(akima)#datargl.spheres(akima$x,akima$z,akima$y, .5,color="red")rgl.bbox()#bivariate linear interpolation#interp:akima.li<-interp(akima$x,akima$y,akima$z,xo=seq(min(akima$x),max(akima$x),length=1 ),yo=seq(min(akima$y),max(akima$y),length=1 ))#interp surface:rgl.surface(akima.li$x,akima.li$y,akima.li$z,color="green",alpha=c( .5))#interpp:akima.p<-interpp(akima$x,akima$y,akima$z,runif(2 ,min(akima$x),max(akima$x)),runif(2 ,min(akima$y),max(akima$y)))#interpp points:rgl.points(akima.p$x,akima.p$z,akima.p$y,size=4,color="yellow")#bivariate spline interpolation#datargl.spheres(akima$x,akima$z,akima$y, .5,color="red")rgl.bbox()#bivariate cubic spline interpolation#interp:akima.si<-interp(akima$x,akima$y,akima$z,xo=seq(min(akima$x),max(akima$x),length=1 ),yo=seq(min(akima$y),max(akima$y),length=1 ),linear=FALSE,extrap=TRUE)#interp surface:rgl.surface(akima.si$x,akima.si$y,akima.si$z,color="green",alpha=c( .5))#interpp:akima.sp<-interpp(akima$x,akima$y,akima$z,runif(2 ,min(akima$x),max(akima$x)),runif(2 ,min(akima$y),max(akima$y)),linear=FALSE,extrap=TRUE)#interpp points:rgl.points(akima.sp$x,akima.sp$z,akima.sp$y,size=4,color="yellow")##End(Not run)aspline Univariate Akima interpolationDescriptionThe function returns a list of points which smoothly interpolate given data points,similar to a curve drawn by hand.Usageaspline(x,y=NULL,xout,n=5 ,ties=mean,method="original",degree=3)Argumentsx,y vectors giving the coordinates of the points to be interpolated.Alternatively a single plotting structure can be specified:see xy.coords.xout an optional set of values specifying where interpolation is to take place.n If xout is not specified,interpolation takes place at n equally spaced points spanning the interval[min(x),max(x)].ties Handling of tied x values.Either a function with a single vector argument re-turning a single number result or the string"ordered".method either"original"method after Akima(1970)or"improved"method after Akima(1991)degree if improved algorithm is selected:degree of the polynomials for the interpolat-ing functionDetailsThe original algorithm is based on a piecewise function composed of a set of polynomials,each of degree three,at most,and applicable to successive interval of the given points.In this method, the slope of the curve is determined at each given point locally,and each polynomial representinga portion of the curve between a pair of given points is determined by the coordinates of and theslopes at the points.ValueA list with components x and y,containing n coordinates which interpolate the given data points. ReferencesAkima,H.(1970)A new method of interpolation and smooth curvefitting based on local proce-dures,J.ACM17(4),589-602Akima,H.(1991)A Method of Univariate Interpolation that Has the Accuracy of a Third-degree Polynomial.ACM Transactions on Mathematical Software,17(3),341-366.See Alsoapprox,splineExamples##regular spaced datax<-1:1y<-c(rnorm(5),c(1,1,1,1,3))xnew<-seq(-1,11, .1)plot(x,y,ylim=c(-3,3),xlim=range(xnew))lines(spline(x,y,xmin=min(xnew),xmax=max(xnew),n=2 ),col="blue")lines(aspline(x,y,xnew),col="red")lines(aspline(x,y,xnew,method="improved"),col="black",lty="dotted")lines(aspline(x,y,xnew,method="improved",degree=1 ),col="green",lty="dashed") ##irregular spaced datax<-sort(runif(1 ,max=1 ))y<-c(rnorm(5),c(1,1,1,1,3))xnew<-seq(-1,11, .1)plot(x,y,ylim=c(-3,3),xlim=range(xnew))lines(spline(x,y,xmin=min(xnew),xmax=max(xnew),n=2 ),col="blue")lines(aspline(x,y,xnew),col="red")lines(aspline(x,y,xnew,method="improved"),col="black",lty="dotted")lines(aspline(x,y,xnew,method="improved",degree=1 ),col="green",lty="dashed") ##an example of Akima,1991x<-c(-3,-2,-1, ,1,2,2.5,3)y<-c( , , , ,-1,-1, ,2)plot(x,y,ylim=c(-3,3))lines(spline(x,y,n=2 ),col="blue")lines(aspline(x,y,n=2 ),col="red")lines(aspline(x,y,n=2 ,method="improved"),col="black",lty="dotted")lines(aspline(x,y,n=2 ,method="improved",degree=1 ),col="green",lty="dashed")interp Gridded Bivariate Interpolation for Irregular DataDescriptionThese functions implement bivariate interpolation onto a grid for irregularly spaced input data.Bilinear or bicubic spline interpolation is applied using different versions of algorithms from Akima.Usageinterp(x,y,z,xo=seq(min(x),max(x),length=4 ),yo=seq(min(y),max(y),length=4 ),linear=TRUE,extrap=FALSE,duplicate="error",dupfun=NULL,ncp=NULL) interp.old(x,y,z,xo=seq(min(x),max(x),length=4 ),yo=seq(min(y),max(y),length=4 ),ncp= ,extrap=FALSE,duplicate="error",dupfun=NULL)interp.new(x,y,z,xo=seq(min(x),max(x),length=4 ),yo=seq(min(y),max(y),length=4 ),linear=FALSE,ncp=NULL,extrap=FALSE,duplicate="error",dupfun=NULL)Argumentsx vector of x-coordinates of data points.Missing values are not accepted.y vector of y-coordinates of data points.Missing values are not accepted.z vector of z-coordinates of data points.Missing values are not accepted.x,y,and z must be the same length and may contain no fewer than four points.The points of x and y cannot be collinear,i.e,they cannot fall on the same line(two vectors x and y such that y=ax+b for some a,b will not be accepted).interp is meant for cases in which you have x,y values scattered over a planeand a z value for each.If,instead,you are trying to evaluate a mathematicalfunction,or get a graphical interpretation of relationships that can be describedby a polynomial,try outer().xo vector of x-coordinates of output grid.The default is40points evenly spaced over the range of x.If extrapolation is not being used(extrap=FALSE,the de-fault),xo should have a range that is close to or inside of the range of x for theresults to be meaningful.yo vector of y-coordinates of output grid;analogous to xo,see above.linear logical–indicating wether linear or spline interpolation should be used.super-sedes old ncp parameterncp deprecated,use parameter linear.Now only used by interp.old().meaning was:number of additional points to be used in computing partialderivatives at each data point.ncp must be either (partial derivatives are notused),or at least2but smaller than the number of data points(and smaller than25).extrap logicalflag:should extrapolation be used outside of the convex hull determined by the data points?duplicate character string indicating how to handle duplicate data points.Possible values are"error"produces an error message,"strip"remove duplicate z values,"mean","median","user"calculate mean,median or user defined function(dupfun)of duplicate z values.dupfun a function,applied to duplicate points if duplicate="user".DetailsIf linear is TRUE(default),linear interpolation is used in the triangles bounded by data points.Cubic interpolation is done if linear is set to FALSE.If extrap is FALSE,z-values for points outside the convex hull are returned as NA.No extrapolation can be performed for the linear case.The interp function handles duplicate(x,y)points in different ways.As default it will stop with an error message.But it can give duplicate points an unique z value according to the parameter duplicate(mean,median or any other user defined function).The triangulation scheme used by interp works well if x and y have similar scales but will appear stretched if they have very different scales.The spreads of x and y must be within four orders of magnitude of each other for interp to work.Valuelist with3components:x,y vectors of x-and y-coordinates of output grid,the same as the input argument xo,or yo,if present.Otherwise,their default,a vector40points evenly spacedover the range of the input x.z matrix offitted z-values.The value z[i,j]is computed at the x,y point xo[i], yo[j].z has dimensions length(xo)times length(yo).Noteinterp is a wrapper for the two versions interp.old(it uses(almost)the same Fortran code from Akima1978as the S-Plus version)and interp.new(it is based on new Fortran code from Akima 1996).For linear interpolation the old version is choosen,but spline interpolation is done by the new version.Earlier versions(pre0.5-1)of interp used the parameter ncp to choose between linear and cubic interpolation,this is now done by setting the logical parameter e of ncp is still possible, but is deprecated.The resulting structure is suitable for input to the functions contour and image.Check the require-ments of these functions when choosing values for xo and yo.ReferencesAkima,H.(1978).A Method of Bivariate Interpolation and Smooth Surface Fitting for Irregularly Distributed Data Points.ACM Transactions on Mathematical Software4,148-164.Akima,H.(1996).Algorithm761:scattered-data surfacefitting that has the accuracy of a cubic polynomial.ACM Transactions on Mathematical Software22,362–371.See Alsocontour,image,approx,spline,aspline,outer,expand.grid.Examplesdata(akima)plot(y~x,data=akima,main="akima example data")with(akima,text(x,y,formatC(z,dig=2),adj=- .1))##linear interpolationakima.li<-interp(akima$x,akima$y,akima$z)image(akima.li,add=TRUE)contour(akima.li,add=TRUE)points(akima,pch=3)##increase smoothness(using finer grid):akima.smooth<-with(akima,interp(x,y,z,xo=seq( ,25,length=1 ),yo=seq( ,2 ,length=1 )))image(akima.smooth,main="interp(<akima data>,*)on finer grid")contour(akima.smooth,add=TRUE,col="thistle")points(akima,pch=3,cex=2,col="blue")#use triangulation package to show underlying triangulation:if(library(tripack,logical.return=TRUE))plot(tri.mesh(akima),add=TRUE,lty="dashed")#use only15points(interpolation only within convex hull!)akima.part<-with(akima,interp(x[1:15],y[1:15],z[1:15]))image(akima.part)title("interp()on subset of only15points")contour(akima.part,add=TRUE)points(akima$x[1:15],akima$y[1:15],col="blue")##spline interpolation,two variants(AMS526"Old",AMS761"New")##-----------------------------------------------------------------##"Old":use5points to calculate derivatives->many NAsakima.sO<-interp.old(akima$x,akima$y,akima$z,xo=seq( ,25,length=1 ),yo=seq( ,2 ,length=1 ),ncp=5) table(is.na(akima.sO$z))##399 NA’s;=4 %akima.sO<-with(akima,interp.old(x,y,z,xo=seq( ,25,length=1 ),yo=seq( ,2 ,len=1 ),ncp=4)) sum(is.na(akima.sO$z))##still3429image(akima.sO,main="interp.old(*,ncp=4)[almost useless]")contour(akima.sO,add=TRUE)##"New:"akima.spl<-with(akima,interp.new(x,y,z,xo=seq( ,25,length=1 ),yo=seq( ,2 ,length=1 ))) ##equivalent call via setting linear=FALSE in interp():akima.spl<-with(akima,interp(x,y,z,xo=seq( ,25,length=1 ),yo=seq( ,2 ,length=1 ),linear=FALSE))contour(akima.spl,main="smooth interp(*,linear=FALSE)")points(akima)full.pal<-function(n)hcl(h=seq(34 ,2 ,length=n))cool.pal<-function(n)hcl(h=seq(12 , ,length=n)+15 )warm.pal<-function(n)hcl(h=seq(12 , ,length=n)-3 )filled.contour(akima.spl,color.palette=full.pal,plot.axes={axis(1);axis(2);title("smooth interp(*,linear=FALSE)");points(akima,pch=3,col=hcl(c=1 ,l=2 ))}) #no extrapolation!##example with duplicate points:data(airquality)air<-subset(airquality,!is.na(Temp)&!is.na(Ozone)&!is.na(Solar.R))#gives an error{duplicate..}:try(air.ip<-interp(air$Temp,air$Solar.R,air$Ozone,linear=FALSE))#use mean of duplicate points:air.ip<-with(air,interp(Temp,Solar.R,log(Ozone),duplicate="mean",linear=FALSE))image(air.ip,main="Airquality:Ozone vs.Temp and Solar.R")with(air,points(Temp,Solar.R))interpp Pointwise Bivariate Interpolation for Irregular DataDescriptionIf ncp is zero,linear interpolation is used in the triangles bounded by data points.Cubic interpo-lation is done if partial derivatives are used.If extrap is FALSE,z-values for points outside the convex hull are returned as NA.No extrapolation can be performed if ncp is zero.The interpp function handles duplicate(x,y)points in different ways.As default it will stop with an error message.But it can give duplicate points an unique z value according to the parameter duplicate(mean,median or any other user defined function).The triangulation scheme used by interp works well if x and y have similar scales but will appear stretched if they have very different scales.The spreads of x and y must be within four orders of magnitude of each other for interpp to work.Usageinterpp(x,y,z,xo,yo,linear=TRUE,extrap=FALSE,duplicate="error",dupfun=NULL,ncp)Argumentsx vector of x-coordinates of data points.Missing values are not accepted.y vector of y-coordinates of data points.Missing values are not accepted.z vector of z-coordinates of data points.Missing values are not accepted.x,y,and z must be the same length and may contain no fewer than four points.The points of x and y cannot be collinear,i.e,they cannot fall on the same line(two vectors x and y such that y=ax+b for some a,b will not be accepted).xo vector of x-coordinates of points at which to evaluate the interpolating function.yo vector of y-coordinates of points at which to evaluate the interpolating function.linear logical–indicating wether linear or spline interpolation should be used.super-sedes old ncp parameterncp deprecated,use parameter linear.Now only used by interpp.old().meaning was:number of additional points to be used in computing partialderivatives at each data point.ncp must be either (partial derivatives are notused,=linear interpolation),or at least2but smaller than the number of datapoints(and smaller than25).extrap logicalflag:should extrapolation be used outside of the convex hull determinedby the data points?duplicate indicates how to handle duplicate data points.Possible values are"error"-pro-duces an error message,"strip"-remove duplicate z values,"mean","median","user"-calculate mean,median or user defined function of duplicate z values.dupfun this function is applied to duplicate points if duplicate="user"Valuelist with3components:x vector of x-coordinates of output points,the same as the input argument xo.y vector of y-coordinates of output points,the same as the input argument yo.zfitted z-values.The value z[i]is computed at the x,y point x[i],y[i].NOTEUse interp if interpolation on a regular grid is wanted.The two versions interpp.old and interpp.new refer to Akimas Fortran code from1978and1996resp.The call wrapper interpp chooses interpp.old for linear and interpp.new for cubicspline interpolation.Earlier versions(pre0.5-1)of interpp used the parameter ncp to choose between linear and cubicinterpolation,this is now done by setting the logical parameter e of ncp is still possible,but is deprecated.ReferencesAkima,H.(1978).A Method of Bivariate Interpolation and Smooth Surface Fitting for Irregularly Distributed Data Points.ACM Transactions on Mathematical Software,4,148-164.Akima,H.(1996).Algorithm761:scattered-data surfacefitting that has the accuracy of a cubic polynomial.ACM Transactions on Mathematical Software,22,362-371.See Alsocontour,image,approxfun,splinefun,outer,expand.grid,interp,aspline.Examplesdata(akima)#linear interpolation at points(1,2),(5,6)and(1 ,12)akima.lip<-interpp(akima$x,akima$y,akima$z,c(1,5,1 ),c(2,6,12))akima.lip$z#spline interpolationakima.sip<-interpp(akima$x,akima$y,akima$z,c(1,5,1 ),c(2,6,12),linear=FALSE)akima.sip$zIndex∗Topic arithaspline,3∗Topic datasetsakima,2∗Topic dplotaspline,3interp,5interpp,8akima,2approx,4,7approxfun,10aspline,3,7,10contour,6,7,10expand.grid,7,10image,6,7,10interp,5,10interpp,8outer,7,10spline,4,7splinefun,10xy.coords,311。
Image Super-Resolution via Sparse Representation

1Image Super-Resolution via Sparse Representation Jianchao Yang,Student Member,IEEE,John Wright,Student Member,IEEE Thomas Huang,Life Fellow,IEEEand Yi Ma,Senior Member,IEEEAbstract—This paper presents a new approach to single-image superresolution,based on sparse signal representation.Research on image statistics suggests that image patches can be well-represented as a sparse linear combination of elements from an appropriately chosen over-complete dictionary.Inspired by this observation,we seek a sparse representation for each patch of the low-resolution input,and then use the coefficients of this representation to generate the high-resolution output.Theoretical results from compressed sensing suggest that under mild condi-tions,the sparse representation can be correctly recovered from the downsampled signals.By jointly training two dictionaries for the low-and high-resolution image patches,we can enforce the similarity of sparse representations between the low resolution and high resolution image patch pair with respect to their own dictionaries.Therefore,the sparse representation of a low resolution image patch can be applied with the high resolution image patch dictionary to generate a high resolution image patch. The learned dictionary pair is a more compact representation of the patch pairs,compared to previous approaches,which simply sample a large amount of image patch pairs[1],reducing the computational cost substantially.The effectiveness of such a sparsity prior is demonstrated for both general image super-resolution and the special case of face hallucination.In both cases,our algorithm generates high-resolution images that are competitive or even superior in quality to images produced by other similar SR methods.In addition,the local sparse modeling of our approach is naturally robust to noise,and therefore the proposed algorithm can handle super-resolution with noisy inputs in a more unified framework.I.I NTRODUCTIONSuper-resolution(SR)image reconstruction is currently a very active area of research,as it offers the promise of overcoming some of the inherent resolution limitations of low-cost imaging sensors(e.g.cell phone or surveillance cameras)allowing better utilization of the growing capability of high-resolution displays(e.g.high-definition LCDs).Such resolution-enhancing technology may also prove to be essen-tial in medical imaging and satellite imaging where diagnosis or analysis from low-quality images can be extremely difficult. Conventional approaches to generating a super-resolution im-age normally require as input multiple low-resolution images of the same scene,which are aligned with sub-pixel accuracy. The SR task is cast as the inverse problem of recovering the original high-resolution image by fusing the low-resolution images,based on reasonable assumptions or prior knowledge about the observation model that maps the high-resolution im-age to the low-resolution ones.The fundamental reconstruction Jianchao Yang and Thomas Huang are with Beckman Institute,Uni-versity of Illinois Urbana-Champaign,Urbana,IL61801USA(email: jyang29@;huang@).John Wright and Yi Ma are with CSL,University of Illinois Urbana-Champaign,Urbana,IL61801USA(email:jnwright@; yima@).constraint for SR is that the recovered image,after applying the same generation model,should reproduce the observed low-resolution images.However,SR image reconstruction is gen-erally a severely ill-posed problem because of the insufficient number of low resolution images,ill-conditioned registration and unknown blurring operators,and the solution from the reconstruction constraint is not unique.Various regularization methods have been proposed to further stabilize the inversion of this ill-posed problem,such as[2],[3],[4].However,the performance of these reconstruction-based super-resolution algorithms degrades rapidly when the desired magnification factor is large or the number of available input images is small.In these cases,the result may be overly smooth,lacking important high-frequency details[5].Another class of SR approach is based on interpolation[6],[7], [8].While simple interpolation methods such as Bilinear or Bicubic interpolation tend to generate overly smooth images with ringing and jagged artifacts,interpolation by exploiting the natural image priors will generally produce more favorable results.Dai et al.[7]represented the local image patches using the background/foreground descriptors and reconstructed the sharp discontinuity between the two.Sun et.al.[8]explored the gradient profile prior for local image structures and ap-plied it to super-resolution.Such approaches are effective in preserving the edges in the zoomed image.However,they are limited in modeling the visual complexity of the real images. For natural images withfine textures or smooth shading,these approaches tend to produce watercolor-like artifacts.A third category of SR approach is based on ma-chine learning techniques,which attempt to capture the co-occurrence prior between low-resolution and high-resolution image patches.[9]proposed an example-based learning strat-egy that applies to generic images where the low-resolution to high-resolution prediction is learned via a Markov Random Field(MRF)solved by belief propagation.[10]extends this approach by using the Primal Sketch priors to enhance blurred edges,ridges and corners.Nevertheless,the above methods typically require enormous databases of millions of high-resolution and low-resolution patch pairs,and are therefore computationally intensive.[11]adopts the philosophy of Lo-cally Linear Embedding(LLE)[12]from manifold learning, assuming similarity between the two manifolds in the high-resolution and the low-resolution patch spaces.Their algorithm maps the local geometry of the low-resolution patch space to the high-resolution one,generating high-resolution patch as a linear combination of ing this strategy,more patch patterns can be represented using a smaller training database.However,using afixed number K neighbors for reconstruction often results in blurring effects,due to over-or under-fitting.In our previous work[1],we proposed a method2for adaptively choosing the most relevant reconstruction neigh-bors based on sparse coding,avoiding over-or under-fitting of [11]and producing superior results.However,sparse codingover a large sampled image patch database directly is too time-consuming.While the mentioned approaches above were proposed forgeneric image super-resolution,specific image priors can be incorporated when tailored to SR applications for specificdomains such as human faces.This face hallucination prob-lem was addressed in the pioneering work of Baker and Kanade[13].However,the gradient pyramid-based predictionintroduced in[13]does not directly model the face prior,and the pixels are predicted individually,causing discontinuities and artifacts.Liu et al.[14]proposed a two-step statisticalapproach integrating the global PCA model and a local patch model.Although the algorithm yields good results,the holistic PCA model tends to yield results like the mean face and theprobabilistic local patch model is complicated and compu-tationally demanding.Wei Liu et al.[15]proposed a newapproach based on TensorPatches and residue compensation. While this algorithm adds more details to the face,it also introduces more artifacts.This paper focuses on the problem of recovering the super-resolution version of a given low-resolution image.Similar to the aforementioned learning-based methods,we will relyon patches from the input image.However,instead of work-ing directly with the image patch pairs sampled from high-and low-resolution images[1],we learn a compact repre-sentation for these patch pairs to capture the co-occurrence prior,significantly improving the speed of the algorithm.Our approach is motivated by recent results in sparse signal representation,which suggest that the linear relationships among high-resolution signals can be accurately recoveredfrom their low-dimensional projections[16],[17].Although the super-resolution problem is very ill-posed,making preciserecovery impossible,the image patch sparse representation demonstrates both effectiveness and robustness in regularizing the inverse problem.a)Basic Ideas:To be more precise,let D∈R n×K be an overcomplete dictionary of K atoms(K>n),and suppose a signal x∈R n can be represented as a sparse linearcombination with respect to D.That is,the signal x can be written as x=Dα0where whereα0∈R K is a vector with very few( n)nonzero entries.In practice,we might onlyobserve a small set of measurements y of x:y.=L x=L Dα0,(1) where L∈R k×n with k<n is a projection matrix.In our super-resolution context,x is a high-resolution image(patch), while y is its low-resolution counter part(or features extracted from it).If the dictionary D is overcomplete,the equation x=Dαis underdetermined for the unknown coefficientsα. The equation y=L Dαis even more dramatically under-determined.Nevertheless,under mild conditions,the sparsest solutionα0to this equation will be unique.Furthermore,if D satisfies an appropriate near-isometry condition,then for a wide variety of matrices L,any sufficiently sparse linear representation of a high-resolution image patch x interms Fig.1.Reconstruction of a raccoon face with magnification factor2.Left: result by our method.Right:the original image.There is little noticeable difference visually even for such a complicated texture.The RMSE for the reconstructed image is5.92(only the local patch model is employed).of the D can be recovered(almost)perfectly from the low-resolution image patch[17],[18].1Fig.1shows an example that demonstrates the capabilities of our method derived fromthis principle.The image of the raccoon face is blurred anddownsampled to half of its original size in both dimensions. Then we zoom the low-resolution image to the original sizeusing the proposed method.Even for such a complicated texture,sparse representation recovers a visually appealingreconstruction of the original signal.Recently sparse representation has been successfully appliedto many other related inverse problems in image processing, such as denoising[19]and restoration[20],often improving onthe state-of-the-art.For example in[19],the authors use theK-SVD algorithm[21]to learn an overcomplete dictionary from natural image patches and successfully apply it to theimage denoising problem.In our setting,we do not directlycompute the sparse representation of the high-resolution patch. Instead,we will work with two coupled dictionaries,D h forhigh-resolution patches,and D l for low-resolution ones.The sparse representation of a low-resolution patch in terms of D l will be directly used to recover the corresponding high-resolution patch from D h.We obtain a locally consistent solution by allowing patches to overlap and demanding that thereconstructed high-resolution patches agree on the overlappedareas.In this paper,we try to learn the two overcomplete dictionaries in a probabilistic model similar to[22].To enforce that the image patch pairs have the same sparse representations with respect to D h and D l,we learn the two dictionaries simultaneously by concatenating them with proper normal-ization.The learned compact dictionaries will be applied to both generic image super-resolution and face hallucination to demonstrate their effectiveness.Compared with the aforementioned learning-based methods,our algorithm requires only two compact learned dictionaries, instead of a large training patch database.The computation, mainly based on linear programming or convex optimization, is much more efficient and scalable,compared with[9],[10], [11].The online recovery of the sparse representation uses the low-resolution dictionary only–the high-resolution dictionary 1Even though the structured projection matrix defined by blurring and downsampling in our SR context does not guarantee exact recovery ofα0, empirical experiments indeed demonstrate the effectiveness of such a sparse prior for our SR tasks.3is used to calculate thefinal high-resolution image.The computed sparse representation adaptively selects the most relevant patch bases in the dictionary to best represent each patch of the given low-resolution image.This leads to superior performance,both qualitatively and quantitatively,compared to the method described in[11],which uses afixed number of nearest neighbors,generating sharper edges and clearer textures.In addition,the sparse representation is robust to noise as suggested in[19],and thus our algorithm is more robust to noise in the test image,while most other methods cannot perform denoising and super-resolution simultaneously.b)Organization of the Paper:The remainder of this paper is organized as follows.Section II details our formula-tion and solution to the image super-resolution problem based on sparse representation.Specifically,we study how to apply sparse representation for both generic image super-resolution and face hallucination.In Section III,we discuss how to learn the two dictionaries for the high-and low-resolution image patches respectively.Various experimental results in Section IV demonstrate the efficacy of sparsity as a prior for regularizing image super-resolution.c)Notations:X and Y denote the high-and low-resolution images respectively,and x and y denote the high-and low-resolution image patches respectively.We use bold uppercase D to denote the dictionary for sparse coding, specifically we use D h and D l to denote the dictionaries for high-and low-resolution image patches respectively.Bold lowercase letters denote vectors.Plain uppercase letters denote regular matrices,i.e.,S is used as a downsampling operation in matrix form.Plain lowercase letters are used as scalars.II.I MAGE S UPER-R ESOLUTION FROM S PARSITYThe single-image super-resolution problem asks:given a low-resolution image Y,recover a higher-resolution image X of the same scene.Two constraints are modeled in this work to solve this ill-posed problem:1)reconstruction constraint, which requires that the recovered X should be consistent with the input Y with respect to the image observation model; and2)sparsity prior,which assumes that the high resolution patches can be sparsely represented in an appropriately chosen overcomplete dictionary,and that their sparse representations can be recovered from the low resolution observation.1)Reconstruction constraint:The observed low-resolution image Y is a blurred and downsampled version of the high resolution image X:Y=SH X(2) Here,H represents a blurringfilter,and S the downsampling operator.Super-resolution remains extremely ill-posed,since for a given low-resolution input Y,infinitely many high-resolution images X satisfy the above reconstruction constraint.We further regularize the problem via the following prior on small patches x of X:2)Sparsity prior:The patches x of the high-resolution image X can be represented as a sparse linear combination in a dictionary D h trained from high-resolution patches sampled from training images:x≈D hαfor someα∈R K with α 0 K.(3) The sparse representationαwill be recovered by representing patches y of the input image Y,with respect to a low resolution dictionary D l co-trained with D h.The dictionary training process will be discussed in Section III.We apply our approach to both generic images and face images.For generic image super-resolution,we divide the problem into two steps.First,as suggested by the sparsity prior(3),wefind the sparse representation for each local patch,respecting spatial compatibility between neighbors. Next,using the result from this local sparse representation, we further regularize and refine the entire image using the reconstruction constraint(2).In this strategy,a local model from the sparsity prior is used to recover lost high-frequency for local details.The global model from the reconstruction constraint is then applied to remove possible artifacts from thefirst step and make the image more consistent and natural. The face images differ from the generic images in that the face images have more regular structure and thus reconstruction constraints in the face subspace can be more effective.For face image super-resolution,we reverse the above two steps to make better use of the global face structure as a regularizer. Wefirstfind a suitable subspace for human faces,and apply the reconstruction constraints to recover a medium resolution image.We then recover the local details using the sparsity prior for image patches.The remainder of this section is organized as follows:in Section II-A,we discuss super-resolution for generic images. We will introduce the local model based on sparse represen-tation and global model based on reconstruction constraints. In Section II-B we discuss how to introduce the global face structure into this framework to achieve more accurate and visually appealing super-resolution for face images.A.Generic Image Super-Resolution from Sparsity1)Local model from sparse representation:Similar to the patch-based methods mentioned previously,our algorithm tries to infer the high-resolution image patch for each low-resolution image patch from the input.For this local model, we have two dictionaries D h and D l,which are trained to have the same sparse representations for each high-resolution and low-resolution image patch pair.We subtract the mean pixel value for each patch,so that the dictionary represents image textures rather than absolute intensities.In the recovery process,the mean value for each high-resolution image patch is then predicted by its low-resolution version.For each input low-resolution patch y,wefind a sparse representation with respect to D l.The corresponding high-resolution patch bases D h will be combined according to these coefficients to generate the output high-resolution patch x. The problem offinding the sparsest representation of y can be formulated as:min α 0s.t. F D lα−F y 22≤ ,(4)4 where F is a(linear)feature extraction operator.The main roleof F in(4)is to provide a perceptually meaningful constraint2on how closely the coefficientsαmust approximate y.We willdiscuss the choice of F in Section III.Although the optimization problem(4)is NP-hard in gen-eral,recent results[23],[24]suggest that as long as the desiredcoefficientsαare sufficiently sparse,they can be efficientlyrecovered by instead minimizing the 1-norm3,as follows:min α 1s.t. F D lα−F y 22≤ .(5)Lagrange multipliers offer an equivalent formulationminαF D lα−F y 22+λ α 1,(6)where the parameterλbalances sparsity of the solutionandfidelity of the approximation to y.Notice that this isessentially a linear regression regularized with 1-norm on thecoefficients,known in statistical literature as the Lasso[27].Solving(6)individually for each local patch does notguarantee the compatibility between adjacent patches.Weenforce compatibility between adjacent patches using a one-pass algorithm similar to that of[28].4The patches areprocessed in raster-scan order in the image,from left to rightand top to bottom.We modify(5)so that the super-resolutionreconstruction D hαof patch y is constrained to closelyagree with the previously computed adjacent high-resolutionpatches.The resulting optimization problem ismin α 1s.t. F D lα−F y 22≤ 1,P D hα−w 22≤ 2,(7)where the matrix P extracts the region of overlap betweenthe current target patch and previously reconstructed high-resolution image,and w contains the values of the previouslyreconstructed high-resolution image on the overlap.The con-strained optimization(7)can be similarly reformulated as:minα˜Dα−˜y 22+λ α 1,(8)where˜D=F D lβP D hand˜y=F yβw.The parameterβcontrols the tradeoff between matching the low-resolution input andfinding a high-resolution patch that is compatible with its neighbors.In all our experiments,we simply set β=1.Given the optimal solutionα∗to(8),the high-resolution patch can be reconstructed as x=D hα∗.2Traditionally,one would seek the sparsestαs.t. D lα−y 2≤ .For super-resolution,it is more appropriate to replace this2-norm with a quadratic norm · F T F that penalizes visually salient high-frequency errors.3There are also some recent works showing certain non-convex optimization problems can produce superior sparse solutions to the 1convex problem,e.g., [25]and[26].4There are different ways to enforce compatibility.In[11],the values in the overlapped regions are simply averaged,which will result in blurring effects. The greedy one-pass algorithm[28]is shown to work almost as well as the use of a full MRF model[9].Our algorithm,not based on the MRF model, is essentially the same by trusting partially the previously recovered high resolution image patches in the overlapped regions.Algorithm1(Super-Resolution via Sparse Representation). 1:Input:training dictionaries D h and D l,a low-resolutionimage Y.2:For each3×3patch y of Y,taken starting from the upper-left corner with1pixel overlap in each direction,•Compute the mean pixel value m of patch y.•Solve the optimization problem with˜D and˜y definedin(8):minα ˜Dα−˜y 22+λ α 1.•Generate the high-resolution patch x=D hα∗.Putthe patch x+m into a high-resolution image X0. 3:End4:Using gradient descent,find the closest image to X0 which satisfies the reconstruction constraint:X∗=arg minXSH X−Y 22+c X−X0 22.5:Output:super-resolution image X∗.2)Enforcing global reconstruction constraint:Notice that (5)and(7)do not demand exact equality between the low-resolution patch y and its reconstruction D lα.Because of this,and also because of noise,the high-resolution image X0produced by the sparse representation approach of the previous section may not satisfy the reconstruction constraint (2)exactly.We eliminate this discrepancy by projecting X0 onto the solution space of SH X=Y,computingX∗=arg minXSH X−Y 22+c X−X0 22.(9) The solution to this optimization problem can be efficiently computed using gradient descent.The update equation for this iterative method isX t+1=X t+ν[H T S T(Y−SH X t)+c(X−X0)],(10) where X t is the estimate of the high-resolution image after the t-th iteration,νis the step size of the gradient descent. We take result X∗from the above optimization as our final estimate of the high-resolution image.This image is as close as possible to the initial super-resolution X0given by sparsity,while respecting the reconstruction constraint.The entire super-resolution process is summarized as Algorithm1.3)Global optimization interpretation:The simple SR algo-rithm outlined in the previous two subsections can be viewed as a special case of a more general sparse representation framework for inverse problems in image processing.Related ideas have been profitably applied in image compression, denoising[19],and restoration[20].In addition to placing our work in a larger context,these connections suggest means of further improving the performance,at the cost of increased computational complexity.Given sufficient computational resources,one could in prin-ciple solve for the coefficients associated with all patches simultaneously.Moreover,the entire high-resolution image X itself can be treated as a variable.Rather than demanding that X be perfectly reproduced by the sparse coefficientsα,we can penalize the difference between X and the high-resolution image given by these coefficients,allowing solutions that5are not perfectly sparse,but better satisfy the reconstruction constraints.This leads to a large optimization problem:X ∗=arg min X ,{αij }SH X −Y 22+λi,jαij 0+γi,jD h αij −P ij X 22+τρ(X ) .(11)Here,αij denotes the representation coefficients for the (i,j )th patch of X ,and P ij is a projection matrix that selects the (i,j )th patch from X .ρ(X )is a penalty function that encodes additional prior knowledge about the high-resolution image.This function may depend on the image category,or may take the form of a generic regularization term (e.g.,Huber MRF,Total Variation,Bilateral Total Variation).Algorithm 1can be interpreted as a computationally efficient approximation to (11).The sparse representation step recovers the coefficients αby approximately minimizing the sum of the second and third terms of (11).The sparsity term αij 0is relaxed to αij 1,while the high-resolution fidelity term D h αij −P ij X 2is approximated by its low-resolution version F D l αij −F y ij 2.Notice,that if the sparse coefficients αare fixed,the third term of (11)essentially penalizes the difference between the super-resolution image X and the reconstruction given by the coefficients: i,j D h αij −P ij X 22≈ X 0−X 22.Hence,for small γ,the back-projection step of Algorithm 1approximately minimizes the sum of the first and third terms of (11).Algorithm 1does not,however,incorporate any prior be-sides sparsity of the representation coefficients –the term ρ(X )is absent in our approximation.In Section IV we will see that sparsity in a relevant dictionary is a strong enough prior that we can already achieve good super-resolution per-formance.Nevertheless,in settings where further assumptions on the high-resolution signal are available,these priors can be incorperated into the global reconstruction step of our algorithm.B.Face super-resolution from SparsityFace image resolution enhancement is usually desirable in many surveillance scenarios,where there is always a large distance between the camera and the objects (people)of interest.Unlike the generic image super-resolution discussed earlier,face images are more regular in structure and thus should be easier to handle.Indeed,for face super-resolution,we can deal with lower resolution input images.The basic idea is first to use the face prior to zoom the input to a reasonable medium resolution,and then to employ the local sparsity prior model to recover details.To be precise,the solution is also approached in two steps:1)global model:use reconstruction constraint to recover a medium high-resolution face image,but the solution is searched only in the face subspace;and 2)local model:use the local sparse model to recover the image details.a)Non-negative matrix factorization:In face super-resolution,the most frequently used subspace method for mod-eling the human face is Principal Component Analysis (PCA),which chooses a low-dimensional subspace that captures as much of the variance as possible.However,the PCA bases are holistic,and tend to generate smooth faces similar to the mean.Moreover,because principal component representations allow negative coefficients,the PCA reconstruction is often hard to interpret.Even though faces are objects with lots of variance,they are made up of several relatively independent parts such as eyes,eyebrows,noses,mouths,checks and chins.Nonnegative Matrix Factorization (NMF)[29]seeks a representation of the given signals as an additive combination of local features.To find such a part-based subspace,NMF is formulated as the following optimization problem:arg min U,VX −UV 22s.t.U ≥0,V ≥0,(12)where X ∈R n ×m is the data matrix,U ∈R n ×r is the basismatrix and V ∈R r ×m is the coefficient matrix.In our context here,X simply consists of a set of pre-aligned high-resolution training face images as its column vectors.The number of the bases r can be chosen as n ∗m/(n +m )which is smaller than n and m ,meaning a more compact representation.It can be shown that a locally optimum of (12)can be obtained via the following update rules:V ij ←−V ij (U T X )ij(U TUV )ij U ki ←−U ki (XV T )ki(UV V T )ki,(13)where 1≤i ≤r ,1≤j ≤m and 1≤k ≤n .The obtained basis matrix U is often sparse and localized.b)Two step face super-resolution:Let X and Y denote the high resolution and low resolution faces respectively.Y is obtained from X by smoothing and downsampling as in Eq.2.We want to recover X from the observation Y .In this paper,we assume Y has been pre-aligned to the training database by either manually labeling the feature points or with some automatic face alignment algorithm such as the method used in [14].We can achieve the optimal solution for X based on the Maximum a Posteriori (MAP)criteria,X ∗=arg max Xp (Y |X )p (X ).(14)p (Y |X )models the image observation process,usually with Gaussian noise assumption on the observation Y ,p (Y |X )=1/Z exp(− SHU c −Y 22/(2∗σ2))with Z being a nor-malization factor.p (X )is a prior on the underlying high resolution image X ,typically in the exponential form p (X )=exp(−cρ(X )).Using the rules in (13),we can obtain the basis matrix U ,which is composed of sparse bases.Let Ωdenote the face subspace spanned by U .Then in the subspace Ω,the super-resolution problem in (14)can be formulated using the reconstruction constraints as:c ∗=arg min cSHU c −Y 22+ηρ(U c )s.t.c ≥0,(15)where ρ(U c )is a prior term regularizing the high resolution solution,c ∈R r ×1is the coefficient vector in the subspace Ω。
- 1、下载文档前请自行甄别文档内容的完整性,平台不提供额外的编辑、内容补充、找答案等附加服务。
- 2、"仅部分预览"的文档,不可在线预览部分如存在完整性等问题,可反馈申请退款(可完整预览的文档不适用该条件!)。
- 3、如文档侵犯您的权益,请联系客服反馈,我们会尽快为您处理(人工客服工作时间:9:00-18:30)。
Circulant Matrices
• Property: Let f(x)=a0+a1x +…+ an-1xn-1, ε = e 2π i / n then eigenvalues, eigenvectors and determinant of M are
Eigenvalues: Eigenvectors: Determinant:
i ' i i −1
Vertex Consistency Problem
• Matrix form
0 ⋯ 0 µ1 (0) ∂ 2 S 1 r1 v1 (0) µ (0) v (0) ⋯ 0 0 ∂u1∂u2 r2 2 2 ⋮ ⋮ ⋱ ⋮ ⋮ ⋮ = ⋮ 2 1 ⋯ µn −1 (0) vn −1 (0) 0 ∂ S rn −1 0 0 µn (0) vn (0) ∂un ∂u1 rn ⋯ 0
• Research
CAGD Geometry Processing Scientific Visualization
Authors
Georges-Pierre Bonneau • Main Posts
Professor at Université Joseph Fourier Researcher at LJK
∂S i ∂2 S i ∂S i ∂S i −1 ' ' ri = Φ (0) (0, 0) + Φ i (0) (0, 0) − vi (0) (0, 0) − µi (0) (0, 0) ∂ui ∂ui ∂ui ∂ui +1 ∂ui −1
' i
It is generally unsolvable when n is even
λk = f (ε k ),
n −1
k = 0,..., n − 1.
vk = (1, ε k , ε 2 k ,..., ε ( n −1) k )T
det( M ) = ∏ f (ε k )
k =0
Examples of Circulant Matrices
• Determine the singularity of
Φ i (1)
2π ∂S i 1 ∂S i 1 ∂S i−1 − cos( ) (1,0) = (0,0) + (0,0), ɶ ɶ ni ∂ui 2 ∂ui+1 2 ∂ui−1 2π 1 2π cos n (1 − 2ui ) ui ∈[0, 2 ] ⇒ Φ i (1) = − cos( ) choose Φ (u ) = i i ni − cos 2π (2ui −1) ui ∈[ 1 ,1] ni 2 n is the order of v
• Non-trivial solution require
det(•) = ∏ (cos(2kπ / n) − Φ0 ) = 0, choose Φ0 = cos(2π / n)
k =0
Choice of Φ i ( u i )
• Determine Φi (1)
2π ∂S i 1 ∂S i 1 ∂S i−1 cos( ) (0,0) = (0,0) + (0,0), ɶ ɶ ɶ ni ∂ui 2 ∂ui+1 2 ∂ui−1 ɶ ɶ ɶ ui = 1− ui , ui−1 = ui−1, ui+1 = ui+1
• Matrix form
Tt = Φ d1 + Φ d 2
a0 a n −1 M= ⋮ a1 a1 a0 ⋮ a2 ⋯ an − 2 a1 ⋯ an −3 ⋮ ⋱ ⋮ a3 ⋯ an −1 an −1 an − 2 ⋮ a0 n×n
a2
• Remark: Circulant matrix is a special case of Toeplitz matrix
∏
• Compute the rank of
1 cos(2π / n) cos(4π / n) cos(2π / n) 1 cos(2π / n) A= ⋮ ⋮ ⋮ cos(2(n−1)π / n) cos(2(n−2)π / n) cos(2(n−3)π / n) ⋯ cos(2(n−1)π / n) ⋯ cos(2(n−2)π / n) ⋱ ⋮ ⋯ 1 n×n
Authors
Stefanie Hahmann • Main Posts
Professor at Institut National Polytechnique de Grenoble (INPG), France Researcher at Laboratorie Jean Kuntzmann (LJK)
Geometric Modeling and Processing 2008
Bicubic interpolation of arbitrary quad meshes using a 4-split
S. Hahmann G.P. Bonneau B. Caram. 20, 2008
Let vi (ui ) ≡ µi (ui ) ≡ 1/ 2, ∀ui ∈ [0,1], then
∂S i 1 ∂S i 1 ∂S i −1 Φ i (ui ) (ui , 0) = (ui , 0) + (0, ui ), ∂ui 2 ∂ui +1 2 ∂ui −1 ui ∈ [0,1], i = 1,..., n.
A peep of HD 3D Animation
From Appleseed EX Machina (2007)
Subdivision Surface
Doo-Sabin 细分方法
Catmull-Clark 细分方法
Loop 细分方法
Butterfly 细分方法
From PhD thesis of Zhang Jinqiao
rank ( A) = 2
Vertex Consistency Problem
• For C2 surface assembling
∂S i ∂S i ∂ S i −1 (u i , 0) = vi (u i ) (u i , 0) + µ i (u i ) (0, u i ), Φ i (u i ) ∂ui ∂ u i +1 ∂ u i −1 u i ∈ [0,1], i = 1,..., n.
• Circulant Matrices • Vertex Consistency Problem • Surface Construction by Steps
Applications of Surface Modeling
• • • • Medical imaging Geological modeling Scientific visualization 3D computer graphic animation
• Simplification of G1 continuity condition
∂S i ∂S i ∂S i −1 Φ i (ui ) (ui , 0) = vi (ui ) (ui , 0) + µi (ui ) (0, ui ), ∂ui ∂ui +1 ∂ui −1 ui ∈ [0,1], i = 1,..., n.
∂ S ∂ S (0, 0) = (0, 0) ∂ u i ∂ u i +1 ∂ u i +1 ∂ u i
2 i 2 i
then
∂2 S i ∂ 2 S i −1 vi (0) (0, 0) + µ i (0) (0, 0) ∂ui ∂ui +1 ∂ui −1∂ui ∂S i ∂2 S i = Φ i' (0) (0, 0) + Φ i (0) (0, 0) ∂ui ∂ui ∂ui ∂S ∂S ' − v (0) (0, 0) − µ i (0) (0, 0) ∂ui +1 ∂ui −1
i i
Step 1:Determine Boundary Curve
• Differentiate G1 continuity equation and specialize at ui=0, then
1 ∂2 S i 1 ∂ 2 S i −1 ∂S i ∂2 S i (0,0) + (0,0) = Φ1 (0) (0,0) + Φ0 2 (0,0) 2 ∂ui ∂ui +1 2 ∂ui −1∂ui ∂ui ∂ui where Φ1 = Φi' (0), Φ0 = Φi (0) = cos(2π / n)
0.5 0 ⋯ 0 0.5 0.5 0.5 ⋯ 0 0 T = ⋮ ⋮ ⋱ ⋮ ⋮ 0 0 ⋯ 0.5 0 0 0 ⋯ 0.5 0.5 n×n
Solution:
f(x)=0.5+0.5xn-1,
ε =e
2π i / n
det(T ) = 0 ⇔ λk = f (ε k ) = 0.5(1 + e2k ( n−1)π i / n ) = 0, k = 0,..., n − 1 ⇔ 2k (n − 1) / n = 2m + 1, m ∈ ℤ ⇔ 2| n
• Research
CAGD Visualization
Outline
• Applications of surface modeling • Background
Subdivision surface Global tensor product surface Locally constructed surface
Sketch of the Algorithm