Combinatorial interpretations of the q-Faulhaber and q-Salié coefficients, preprint, arXiv
Neural Networks and Evolutionary Computation. Part II Hybrid Approaches in the Neuroscience
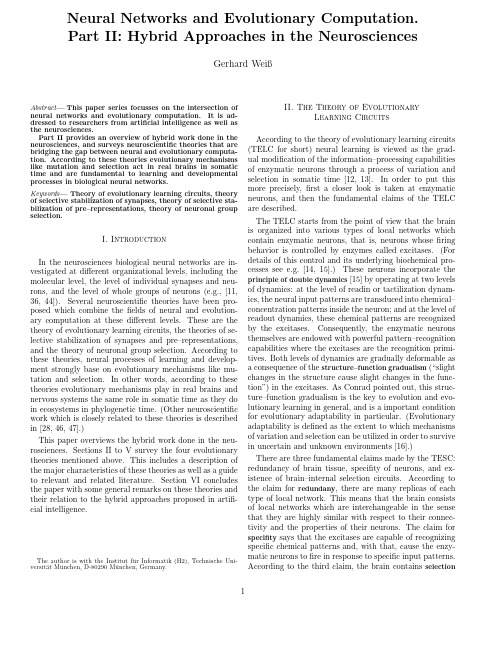
Neural Networks and Evolutionary Computation. Part II:Hybrid Approaches in the NeurosciencesGerhard WeißAbstract—This paper series focusses on the intersection of neural networks and evolutionary computation.It is ad-dressed to researchers from artificial intelligence as well as the neurosciences.Part II provides an overview of hybrid work done in the neurosciences,and surveys neuroscientific theories that are bridging the gap between neural and evolutionary computa-tion.According to these theories evolutionary mechanisms like mutation and selection act in real brains in somatic time and are fundamental to learning and developmental processes in biological neural networks.Keywords—Theory of evolutionary learning circuits,theory of selective stabilization of synapses,theory of selective sta-bilization of pre–representations,theory of neuronal group selection.I.IntroductionIn the neurosciences biological neural networks are in-vestigated at different organizational levels,including the molecular level,the level of individual synapses and neu-rons,and the level of whole groups of neurons(e.g.,[11, 36,44]).Several neuroscientific theories have been pro-posed which combine thefields of neural and evolution-ary computation at these different levels.These are the theory of evolutionary learning circuits,the theories of se-lective stabilization of synapses and pre–representations, and the theory of neuronal group selection.According to these theories,neural processes of learning and develop-ment strongly base on evolutionary mechanisms like mu-tation and selection.In other words,according to these theories evolutionary mechanisms play in real brains and nervous systems the same role in somatic time as they do in ecosystems in phylogenetic time.(Other neuroscientific work which is closely related to these theories is described in[28,46,47].)This paper overviews the hybrid work done in the neu-rosciences.Sections II to V survey the four evolutionary theories mentioned above.This includes a description of the major characteristics of these theories as well as a guide to relevant and related literature.Section VI concludes the paper with some general remarks on these theories and their relation to the hybrid approaches proposed in artifi-cial intelligence.The author is with the Institut f¨u r Informatik(H2),Technische Uni-versit¨a t M¨u nchen,D-80290M¨u nchen,Germany.II.The Theory of EvolutionaryLearning CircuitsAccording to the theory of evolutionary learning circuits(TELC for short)neural learning is viewed as the grad-ual modification of the information–processing capabilities of enzymatic neurons through a process of variation andselection in somatic time[12,13].In order to put this more precisely,first a closer look is taken at enzymatic neurons,and then the fundamental claims of the TELCare described.The TELC starts from the point of view that the brainis organized into various types of local networks which contain enzymatic neurons,that is,neurons whosefiringbehavior is controlled by enzymes called excitases.(For details of this control and its underlying biochemical pro-cesses see e.g.[14,15].)These neurons incorporate the principle of double dynamics[15]by operating at two levels of dynamics:at the level of readin or tactilization dynam-ics,the neural input patterns are transduced into chemical–concentration patterns inside the neuron;and at the level of readout dynamics,these chemical patterns are recognized by the excitases.Consequently,the enzymatic neurons themselves are endowed with powerful pattern–recognition capabilities where the excitases are the recognition primi-tives.Both levels of dynamics are gradually deformable as a consequence of the structure–function gradualism(“slight changes in the structure cause slight changes in the func-tion”)in the excitases.As Conrad pointed out,this struc-ture–function gradualism is the key to evolution and evo-lutionary learning in general,and is a important condition for evolutionary adaptability in particular.(Evolutionary adaptability is defined as the extent to which mechanisms of variation and selection can be utilized in order to survive in uncertain and unknown environments[16].)There are three fundamental claims made by the TESC: redundancy of brain tissue,specifity of neurons,and ex-istence of brain–internal selection circuits.According to the claim for redundany,there are many replicas of each type of local network.This means that the brain consists of local networks which are interchangeable in the sense that they are highly similar with respect to their connec-tivity and the properties of their neurons.The claim for specifity says that the excitases are capable of recognizing specific chemical patterns and,with that,cause the enzy-matic neurons tofire in response to specific input patterns. According to the third claim,the brain contains selectioncircuits which direct thefitness–oriented,gradual modifi-cation of the local networks’excitase configurations.These selection circuits include three systems:a testing system which allows to check the consequences(e.g.,pleasure or pain)of the outputs of one or several local networks for the organism;an evaluation system which assignsfitness values to the local networks on the basis of these consequences; and a growth–control system which stimulates or inhibits the production of the nucleic acids which code for the lo-cal networks’excitases on the basis of theirfitness values. The nucleic acids,whose variability is ensured by random somatic recombination and mutation processes,diffuse to neighbouring networks of the same type(where they per-form the same function because of the interchangeability property mentioned above).These claims imply that neu-ral learning proceeds by means of the gradual modification of the excitase configurations in the brain’s local networks through the repeated execution of the following evolution-ary learning cycle:1.Test and evaluation of the enzymatic neuron–basedlocal networks.As a result,afitness value is assigned to each network.2.Selection of local networks.This involves thefitness–oriented regulation of the production of the excitase–coding nucleic acids,as well as their spreading to ad-jacent interchangeable networks.3.Application of somatic recombination and selection tothese nucleic acids.This maintains the range of the excitase configurations.The execution stops when a local network is found which has a sufficiently highfitness.Conrad emphasized that this evolutionary learning cycle is much more efficient than nat-ural evolution because the selection circuits enable an in-tensive selection even if there is hardly a difference between thefitness values of the interchangeable networks. Finally,some references to related work.The TESC is part of extensive work focussing on the differences be-tween the information processing capabilities of biological (molecular)systems and conventional computers;see e.g. [15,16,17].A computational specification of the ESCM which concentrates on the pattern–processing capabilities of the enzymatic neurons,together with its sucessful appli-cation to a robot–control task,is contained in[29,30,31]. Another computational specification which concentrates on the intraneuronal dynamics of enzymatic neurons is de-scribed in[32].A combination of these two specifications is described in[33].Further related work being of particu-lar interest from a computational point of view is presented in[1,18].III.The Theory of Selective Stabilizationof SynapsesThe theory of selective stabilization of synapses(TSSS for short)is presented in[7,8].This theory accounts for neural processes of learning and development by postulat-ing that a somatic,evolutionary selection mechanism acts at the level of synapses and contributes to the wiring pat-tern in the adult brain.Subsequently the neurobiological basis and the major claims of the TSSS are depicted. The neurobiological basis of the TSSS comprises aspects of both neurogenesis and neurogenetics.In vertebrates one can distinguish several processes of brain development. These are the cellular processes of cell division,movement, adhesion,differentiation,and death,and the synaptic pro-cesses of connection formation and elimination.(For de-tails see e.g.[19,20,38].)The TSSS focusses on the“synap-tic aspect”of neurogenesis;it deals with the outgrowth and the stabilization of synapses,and takes the developmental stage where maximal synaptic wiring exists as its initial state.The neurogenetic attidue of the TSSS constitutes a compromise between the preformist(“specified–by–genes”) and the empirist(“specified–by–activity”)view of brain development.It is assumed that the genes involved in brain development,the so–called genetic envelope,only specify the invariant characters of the brain.This includes,in particular,the connections between the main categories of neurons(i.e.,between groups of neurons which are of the same morphological and biochemical type)and the rules of synaptic growth and stabilization.These rules allow for an activity–dependent,epigenetic synapse formation within the neuronal categories.(As Changeux formulated:“The genetic envelope offers a hazily outlined network,the ac-tivity defines its angles.”[3,p.193])The TSSS makes three major claims.First,at the crit-ical stage of maximal connectivity there is a significant but limited redundany within the neuronal categories as regards the specifity of the synapses.Second,at this time of so–called“structural redundany”any synapse may exist under(at least)three states of plasticity:labile,stable,and degenerate.Only the labile and stable synapses transmit nerve impulses,and the acceptable state transitions are those from labile to either stable or degenerate and from stable to labile.Especially,the state transition of a synapse is epigenetically regulated by all signals received by the postsynaptic soma during a given time interval.(The max-imal synaptic connectivity,the mechanisms of its develop-ment,and the regulative and integrative properties of the soma are determinate expressions of the genetic envelope.) Third,the total activity of the developing network leads to the selective stabilization of some synapses,and to the re-gression of their functional equivalents.As a consequence, structural redundancy decreases and neuronal singularity (i.e.,individual connectivity)increases.This provides a plausible explanation of the naturally occuring connection elimination occuring during neural development.For further readings in the TSSS see e.g.[4,5,6,10].IV.The Theory of Selective Stabilizationof Pre–RepresentationsThe theory of selective stabilization of pre–representa-tions(TSSP for short)can be viewed as an extension of the TSSS.This theory provides a selectionist view of neurallearning and development in the adult brain by postulating that somatic selection takes place at the level of neural networks[5,10,27].Similar to the theory of neuronal group selection(section V),the TSSP may be viewed as an attempt to show how neurobiology and psychology are related to each other.There are two major claims made by the TSSP.Thefirst claim is that there exist mental objects or“neural repre-sentations”in the brain.A mental object is defined as a physical state achieved by the correlated and transitory (both electrical and chemical)activity of a cell assembly consisting of a large number of neurons having different singularities.According to the TSSP,three classes of men-tal objects are distinguished.First,primary percepts;these are labile mental objects whose activation depends on the direct interaction with the outside world and is caused by sensory stimulations.Second,stored representations;these are memory objects whose evocation does not demand en-vironmental interaction and whose all–or–none activity be-havior results from a stable,cooperative coupling between the neurons.And third,pre–representations;these are mental objects which are generated before and concomitant with any environmental interaction.Pre–representations are labile and of great variety and variability;they result from the spontaneous but correlatedfiring of neurons or groups of neurons.The second claim made by the TSSP is that learning in the adult brain corresponds to the se-lective stabilization of pre–representations,that means,the transition from selected pre–representations to stored rep-resentations.This requires,in the simplest case,the inter-action with the environment,the criterion of selection is the resonance(i.e.,spatial overlapping orfiring in phase) between a primary percept and a pre–representation.Further literature on the TSSP.In[9]the two selective–stabilization theories,TSSS and TSSP,are embedded in more general considerations on the neural basis of cogni-tion.A formal model of neural learning and development on the basis of the TSSP is described in[22,43].V.The Theory of Neuronal Group SelectionThe theory of neuronal group selection(TNGS for short) or“neural Darwinism”[23,25]is the most rigorous and elaborate hybrid approach in the neurosciences.This the-ory,which has attracted much attention especially in the last few years,bridges the gap between biology and psy-chology by postulating that somatic selection is the key mechanism which establishes the connection between the structure and the function of the brain.As done in the preceding sections,below the major ideas of the TNGS are described.There are three basic claims.First,during prenatal and early postnatal development,primary repertoires of degen-erate neuronal groups were formed epigenetically by selec-tion.According to the TNGS a neuronal group is consid-ered as a local anatomical entity which consists of hundreds to thousands of strongly connected neurons,and degener-ate neuronal groups are groups that have different struc-tures but carry out the same function more or less well (they are nonisomorphic but isofunctional).The concept of degeneracy is fundamental to the TNGS;it implies both structural diversity and functional redundancy and,hence, ensures both a wide range of recognition and the reliabil-ity against the loss of neural tissue.Degeneracy naturally origins from the processes of brain development which are assumed to occur in an epigenetic manner and to elabo-rate several selective events at the cellular level.According to the regulator hypothesis,these complex developmental processes,as well as the selective events accompaning these processes,are guided by a relatively small number of cell adhesion molecules.Second,in the(postnatal)phase of be-havioral experience,a secondary repertoire of functioning neuronal groups is formed by selection among the preexist-ing groups of each primary repertoire.This group selection is accomplished by epigenetic modifications of the synap-tic strenghts without change of the connectivity pattern. According to the dual rules model,these modifications are realized by two synaptic rules that operate upon popula-tions of synapses in a parallel and independent fashion: a presynaptic rule which applies to long–term changes in the whole target neuron and which affects a large num-ber of synapses;and a postsynaptic rule which applies to short–term changes at individual synapses.The function-ing groups are more likely to respond to identical or simi-lar stimuli than the non–selected groups and,hence,con-tribute to the future behavior of the organism.A funda-mental operation of the functional groups is to compete for neurons that belong to other groups;this competition affects the groups’functional properties and is assumed to play a central role in the formation and organization of cerebral cortical maps.Third,reentry–phasic sig-naling over re–entrant(reciprocal and cyclic)connections between different repertoires,in particular between topo-graphic maps–allows for the spatiotemporal correlation of the responses of the repertoires at all levels in the brain. This kind of phasic signaling is viewed as an important mechanism supporting group selection and as being essen-tial both to categorization and the development of con-sciousness.Reentry implies two fundamental neural struc-tures:first,classification couples,that is,re–entrant reper-toires that can perform classifications more complex than a single involved repertoire could do;and second,global map-pings,that is,re-entrant repertoires that correlate sensory input and motor activity.Some brief notes on how the TNGS accounts for psy-chological functions.Following Edelman’s argumentation, categories do not exist apriori in the world(the world is “unlabeled”),and categorization is the fundamental prob-lem facing the nervous system.This problem is solved by means of group selection and reentry.Consequently,cat-egorization largely depends on the organism’s interaction with its environment and turns out to be the central neural operation required for all other operations.Based on this view of categorization,Edelman suggests that memory is “the enhanced ability to categorize or generalize associa-tively,not the storage of features or attributes of objects as a list”[25,p.241]and that learning,in the minimal case,is the“categorization of complexes of adaptive value under conditions of expectancy”[25,p.293].There is a large body of literature on the TNGS.The most detailed depiction of the theory is provided in Edel-man’s book[25].In order to be able to test the TNGS, several computer models have been constructed which em-body the theory’s major ideas.These models are Darwin I[24],Darwin II[26,39,25],and Darwin III[40,41].Re-views of the TNGS can be found in e.g.[21,34,35,42,37].VI.Concluding RemarksThis paper overviewed neuroscientific theories which view real brains as evolutionary systems or“Darwin machines”[2].This point of view is radically opposed to traditional in-structive theories which postulate that brain development is directed epigenetically during an organism’s interaction with its environment by rules for a more or less precise brain wiring.Nowadays most researchers agree that the instructive theories are very likely to be wrong und unre-alistic,and that the evolutionary theories offer interesting and plausible alternatives.In particular,there is an in-creasing number of neurobiological facts and observations described in the literature which indicate that evolutionary mechanisms(and in particular the mechanism of selection) as postulated by the evolutionary theories are indeed fun-damental to the neural processes in our brains.Somefinal notes on the relation between the hybrid work done in the neurosciences and the hybrid work done in artificial intelligence(see part I of this paper series[45]). Whereas the neuroscientific approaches aim at a better un-derstanding of the developmental and learning processes in real brains,the artificial intelligence approaches typically aim at the design of artificial neural networks that are ap-propriate for solving specific real-world tasks.Despite this fundamental difference and its implications,however,there are several aspects and questions which are elementary and significant to both the neuroscientific and the artificial in-telligence approaches:•Symbolic–subsymbolic intersection(e.g.,“What are the neural foundations of high-level,cognitive abili-ties like concept formation?”and“How are symbolic entities encoded in the neural tissue?”),•Brain wiring(e.g.,“What are the principles of neural development?”and“How are the structure and the function of neural networks related to each other?”),•Genetic encoding(e.g.,“How and to what extend are neural networks genetically encoded?”),and •Evolutionary modification(e.g.,“At what network level and at what time scale do evolutionary mechanisms operate?”and“In how far do the evolutionary mech-anisms influence the network structure?”).Because of this correspondence of interests and research topics it would be useful and stimulating for the neurosci-entific and the artificial intelligence community to be aware of each others hybrid work.This requires an increased in-terdisciplinary transparency.To offer such a transparency is a major intention of this paper series.References[1]Akingbehin,K.&Conrad,M.(1989).A hybrid architecture forprogrammable computing and evolutionary learning.Parallel Distributed Computing,6,245–263.[2]Calvin,W.H.(1987).The brain as a Darwin machine.Nature,330,pp.33–43.[3]Changeux,J.–P.(1980).Genetic determinism and epigenesisof the neuronal network:Is there a biological compromise be-tween Chomsky and Piaget?In M.Piatelli–Palmarini(Ed.), Language and learning–The debate between Jean Piaget and Noam Chomsky(pp.184–202).Routledge&Kegan Paul. [4]Changeux,J.–P.(1983).Concluding remarks:On the“singu-larity”of nerve cells and its ontogenesis.In J.–P.Changeux, J.Glowinski,M.Imbert,&F.E.Bloom(Eds.),Molecular and cellular interactions underlying higher brain function(pp.465–478).Elsevier Science Publ.[5]Changeux,J.–P.(1983).L’Homme neuronal.Fayard.[6]Changeux,J.–P.(1985).Remarks on the complexity of thenervous system and its ontogenesis.In J.Mehler&R.Fox (Eds.),Neonate cognition.Beyond the blooming buzzing con-fusion(pp.263–284).Lawrence Erlbaum.[7]Changeux,J.–P.,Courrege,P.,&Danchin,A.(1973).A theoryof the epigenesis of neuronal networks by selective stabilization of synapses.In Proceedings of the National Academy of Sciences USA,70(10),2974–2978.[8]Changeux,J.–P.,&Danchin,A.(1976).Selective stabilizationof developing synapses as a mechanism for the specification of neuronal networks.Nature,264,705–712.[9]Changeux,J.–P.,&Dehaene,S.(1989).Neuronal models ofcognitive functions.Cognition,33,63–109.[10]Changeux,J.–P.,Heidmann,T.,&Patte,P.(1984).Learningby selection.In P.Marler&H.S.Terrace(Eds.),The biology of learning(pp.115–133).Springer.[11]Changeux,J.–P.,&Konoshi,M.(Eds.).(1986).The neural andmolecular basis of learning.Springer.[12]Conrad,M.(1974).Evolutionary learning cicuits.Journal ofTheoretical Biology,46,167–188.[13]Conrad M.(1976).Complementary models of learning andmemory.BioSystems,8,119–138.[14]Conrad,M.(1984).Microscopic–macroscopic interface in bio-logical information processing.BioSystems,16,345–363. [15]Conrad,M.(1985).On design principles for a molecular com-munications of the ACM,28(5),464–480.[16]Conrad,M.(1988).The price of programmability.In R.Herken(Ed.),The universal Turing machine–A half–century survey (pp.285–307).Kammerer&Unverzagt.[17]Conrad,M.(1989).The brain–machine disanalogy.BioSystems,22,197–213.[18]Conrad,M.,Kampfner,R.R.,Kirby,K.G.,Rizki, E.N.,Schleis,G.,Smalz,R.,&Trenary,R.(1989).Towards an ar-tificial brain.BioSystems,23,175–218.[19]Cowan,W.M.(1978).Aspects of neural development.Interna-tional Reviews of Physiology,17,150–91.[20]Cowan,W.M.,Fawcett,J.W.,O’Leary,D.D.M.,&Stanfield,B.B.(1984).Regressive events in neurogenesis.Science,225,1258–1265.[21]Crick,F.(1989).Neural Edelmanism.Trends in Neurosciences,12,240–248.(Reply from G.N.Reeke,R.Michod and F.Crick: Trends in Neurosciences,13,11–14.)[22]Dehaene,S.,Changeux,J.–P.,&Nadal,J.–P.(1987).Neuralnetworks that learn temporal sequences by selection.In Proceed-ings of the National Academy of Sciences USA,84,pp.2727–2731.[23]Edelman,G.M.(1978).Group selection and phasic reentrantsignaling:A theory of higher brain function.In G.M.Edelman &V.B.Mountcastle(Eds.),The mindful brain.Cortical organi-zation and the group–selective theory of higher brain functions (pp.51–100).The MIT Press.[24]Edelman,G.M.(1981).Group selection as the basis for higherbrain function.In F.O.Schmitt,F.G.Worden,G.Adelman&S.G.Dennis(Eds.),The organization of the cerebral cortex (pp.535–563).The MIT Press.[25]Edelman,G.M.(1987).Neural Darwinism.The theory of neu-ronal group selection.Basic Books.[26]Edelman,G.M.,&Reeke,G.N.(1982).Selective networkscapable of representative transformations,limited generaliza-tions,and associative memory.In Proceedings of the National Academy of Sciences USA,79,2091–2095.[27]Heidmann, A.,Heidmann,T.M.,&Changeux,J.–P.(1984).Stabilisation selective de representations neuronales par reso-nance entre“presepresentations”spontanes du reseau cerebral et“percepts”.In C.R.Acad.Sci.Ser.III,299,839–844. [28]Jerne,N.K.(1967).Antibodies and learning:selection vs.in-struction.In G.C.Quarton,T.Melnechuk&F.O.Schmitt (Eds.),The neurosciences:a study program(pp.200–205).Rockefeller University Press.[29]Kampfner,R.R.(1988).Generalization in evolutionary learn-ing with enzymatic neuron–based systems.In M.Kochen&H.M.Hastings(Eds.),Advances in cognitive science.Steps to-ward convergence(pp.190–209).Westview Press,Inc.[30]Kampfner,R.R.,&Conrad,M.(1983).Computational model-ing of evolutionary learning processes in the brain.Bulletin of Mathematical Biology,45(6),931–968.[31]Kampfner,R.R.,&Conrad,M.(1983).Sequential behaviorand stability properties of enzymatic neuron networks.Bulletin of Mathematical Biology,45(6),969–980.[32]Kirby,K.G.,&Conrad,M.(1984).The enzymatic neuron asa reaction–diffusion network of cyclic nucleotides.Bulletin ofMathematical Biology,46,765–782.[33]Kirby,K.G.,&Conrad,M.(1986).Intraneuronal dynamics asa substrate for evolutionary learning.In Physica22D,205–215.[34]Michod,R.E.(1989).Darwinian selection in the brain.Evolu-tion,(3),694–696.[35]Nelson,R.J.(1989).Philosophical issues in Edelman’s neuralDarwinism.Journal of Experimental and Theoretical Artificial Intelligence,1,195–208.[36]Neuroscience Research(1986).Special issue3:Synaptic plastic-ity,memory and learning.[37]Patton,P.,&Parisi,T.(1989).Brains,computation,and selec-tion:An essay review of Gerald Edelman’s Neural Darwinism.Psychobiology,17(3),326–333.[38]Purves,D.,&Lichtman,J.W.(1985).Principles of neural de-velopment.Sinauer Associates Inc.[39]Reeke,G.N.jr.,&Edelman,G.M.(1984).Selective networksand recognition automata.In Annals of the New York Academy of Sciences,426(Special issue on computer culture),181–201.[40]Reeke,G.N.jr.,&Edelman,G.M.(1988).Real brains andartificial intelligence.Deadalus,117(1),143–173.[41]Reeke,G.N.jr.,Sporns,O.,&Edelman,G.M.(1988).Syn-thetic neural modeling:a Darwinian approach to brain theory.In R.Pfeifer,Z.Schreter,F.Fogelman–Soulie&L.Steels(Eds.), Connectionism in perspective.Elsevier.[42]Smoliar,S.(1989).Book review of[25].Artificial Intelligence,39,121–139.[43]Toulouse,G.,Dehaene,S.,&Changeux,J.–P.(1986).Spin glassmodels of learning by selection.In Proceedings of the National Academy of Sciences USA,83,1695–1698.[44]Trends in Neurosciences(1988).Vol.11(4),Special issue:Learn-ing,memory.[45]Weiß,G.(1993,submitted to IEEE World Congress on Compu-tational Intelligence).Neural networks and evolutionary com-putation.Part I:Hybrid approaches in artificial intelligence. [46]Young,J.Z.(1973).Memory as a selective process.In Aus-tralian Academy of Science Report:Symposium on Biological Memory;Australian Academy of Science(pp.25–45).[47]Young,J.Z.(1975).Sources of discovery in neuroscience.InF.G.Worden,J.P.Swazey&G.Edelman(Eds.),The neu-rosciences:Paths of discovery(pp.15–46).Oxford University Press.。
The
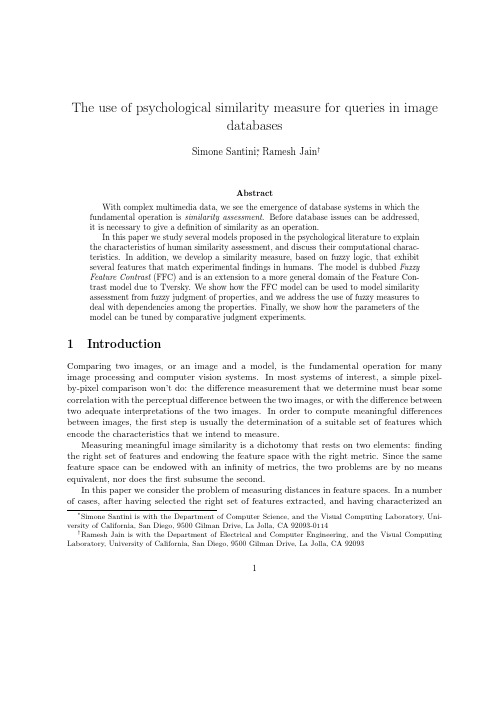
1paring two images, or an image and a model, is the fundamental operation for many image processing and computer vision systems. In most systems of interest, a simple pixelby-pixel comparison won’t do: the difference measurement that we determine must bear some correlation with the perceptual difference between the two images, or with the difference between two adequate interpretations of the two images. In order to compute meaningful differences between images, the first step is usually the determination of a suitable set of features which encode the characteristics that we intend to measure. Measuring meaningful image similarity is a dichotomy that rests on two elements: finding the right set of features and endowing the feature space with the right metric. Since the same feature space can be endowed with an infinity of metrics, the two problems are by no means equivalent, nor does the first subsume the second. In this paper we consider the problem of measuring distances in feature spaces. In a number of cases, after having selected the right set of features extracted, and having characterized an
Binomial coefficient
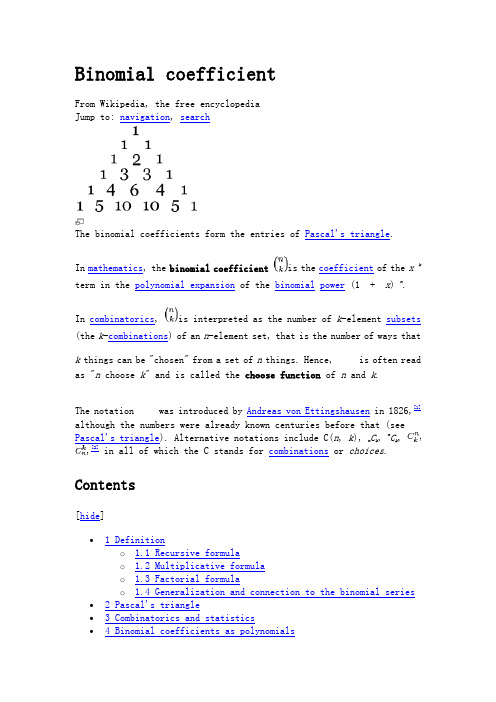
Binomial coefficientFrom Wikipedia, the free encyclopediaJump to: navigation, searchThe binomial coefficients form the entries of Pascal's triangle.In mathematics, the binomial coefficient is the coefficient of the x k term in the polynomial expansion of the binomial power (1 + x)n.In combinatorics, is interpreted as the number of k-element subsets (the k-combinations) of an n-element set, that is the number of ways thatk things can be "chosen" from a set of n things. Hence, is often read as "n choose k" and is called the choose function of n and k.The notation was introduced by Andreas von Ettingshausen in 1826,[1] although the numbers were already known centuries before that (seePascal's triangle). Alternative notations include C(n, k), n C k, n C k,,[2] in all of which the C stands for combinations or choices.Contents[hide]∙ 1 Definitiono 1.1 Recursive formulao 1.2 Multiplicative formulao 1.3 Factorial formulao 1.4 Generalization and connection to the binomial series ∙ 2 Pascal's triangle∙ 3 Combinatorics and statistics∙ 4 Binomial coefficients as polynomialso 4.1 Binomial coefficients as a basis for the space of polynomialso 4.2 Integer-valued polynomialso 4.3 Example∙ 5 Identities involving binomial coefficientso 5.1 Powers of -1o 5.2 Series involving binomial coefficientso 5.3 Identities with combinatorial proofso 5.4 Continuous identities∙ 6 Generating functionso 6.1 Ordinary generating functions∙7 Divisibility properties∙8 Bounds and asymptotic formulas∙9 Generalizationso9.1 Generalization to multinomialso9.2 Generalization to negative integerso9.3 Taylor serieso9.4 Binomial coefficient with n=1/2o9.5 Identity for the product of binomial coefficientso9.6 Partial Fraction Decompositiono9.7 Newton's binomial serieso9.8 Two real or complex valued argumentso9.9 Generalization to q-serieso9.10 Generalization to infinite cardinals∙10 Binomial coefficient in programming languages∙11 See also∙12 Notes∙13 References[edit] DefinitionFor natural numbers(taken to include 0) n and k, the binomial coefficientcan be defined as the coefficient of the monomial X k in the expansion of (1 + X)n. The same coefficient also occurs (if k≤ n) in the binomial formula(valid for any elements x,y of a commutative ring), which explains the name "binomial coefficient".Another occurrence of this number is in combinatorics, where it gives the number of ways, disregarding order, that a k objects can be chosen from among n objects; more formally, the number of k-element subsets (ork-combinations) of an n-element set. This number can be seen to be equal to the one of the first definition, independently of any of the formulas below to compute it: if in each of the n factors of the power (1 + X)n one temporarily labels the term X with an index i(running from 1 to n), then each subset of k indices gives after expansion a contribution X k, and the coefficient of that monomial in the result will be the number ofsuch subsets. This shows in particular that is a natural number for any natural numbers n and k. There are many other combinatorial interpretations of binomial coefficients (counting problems for which the answer is given by a binomial coefficient expression), for instance the number of words formed of n bits(digits 0 or 1) whose sum is k, but most of these are easily seen to be equivalent to counting k-combinations.Several methods exist to compute the value of without actually expanding a binomial power or counting k-combinations.[edit] Recursive formulaOne has a recursive formula for binomial coefficientswith as initial valuesThe formula follows either from tracing the contributions to X k in (1 + X)n−1(1 + X), or by counting k-combinations of {1, 2, ..., n} that containn and that do not contain n separately. It follows easily thatwhen k > n, and for all n, so the recursion can stop when reaching such cases. This recursive formula then allows the construction of Pascal's triangle.[edit] Multiplicative formulaA more efficient method to compute individual binomial coefficients is given by the formulaThis formula is easiest to understand for the combinatorial interpretation of binomial coefficients. The numerator gives the number of ways to select a sequence of k distinct objects, retaining the order of selection, from a set of n objects. The denominator counts the number of distinct sequences that define the same k-combination when order is disregarded.[edit] Factorial formulaFinally there is a formula using factorials that is easy to remember:where n! denotes the factorial of n. This formula follows from the multiplicative formula above by multiplying numerator and denominator by (n−k)!; as a consequence it involves many factors common to numerator and denominator. It is less practical for explicit computation unless common factors are first canceled (in particular since factorial values grow very rapidly). The formula does exhibit a symmetry that is less evident from the multiplicative formula (though it is from the definitions)[edit] Generalization and connection to the binomial seriesThe multiplicative formula allows the definition of binomial coefficients to be extended[note 1] by replacing n by an arbitrary number α (negative, real, complex) or even an element of any commutative ring in which all positive integers are invertible:With this definition one has a generalization of the binomial formula (with one of the variables set to 1), which justifies still calling thebinomial coefficients:This formula is valid for all complex numbers α and X with |X| < 1. It can also be interpreted as an identity of formal power series in X, where it actually can serve as definition of arbitrary powers of series with constant coefficient equal to 1; the point is that with this definition all identities hold that one expects for exponentiation, notablyIf αis a nonnegative integer n, then all terms with k > n are zero, and the infinite series becomes a finite sum, thereby recovering the binomial formula. However for other values of α, including negative integers and rational numbers, the series is really infinite.[edit] Pascal's triangleMain article: Pascal's ruleMain article: Pascal's trianglePascal's rule is the important recurrence relationwhich can be used to prove by mathematical induction that is a natural number for all n and k, (equivalent to the statement that k! divides the product of k consecutive integers), a fact that is not immediately obvious from formula (1).Pascal's rule also gives rise to Pascal's triangle:0: 11: 1 12: 1 2 13: 1 3 3 14: 1 4 6 4 15: 1 5 10 10 5 16: 1 6 15 20 15 6 17: 1 7 21 35 35 21 7 18: 1 8 28 56 70 56 28 8 1Row number n contains the numbers for k= 0,…,n. It is constructed by starting with ones at the outside and then always adding two adjacent numbers and writing the sum directly underneath. This method allows the quick calculation of binomial coefficients without the need for fractions or multiplications. For instance, by looking at row number 5 of the triangle, one can quickly read off that(x + y)5 = 1x5 + 5x4y + 10x3y2 + 10x2y3 + 5x y4 + 1y5.The differences between elements on other diagonals are the elements in the previous diagonal, as a consequence of the recurrence relation (3) above.[edit] Combinatorics and statisticsBinomial coefficients are of importance in combinatorics, because they provide ready formulas for certain frequent counting problems:There are ways to choose k elements from a set of n elements.See Combination.∙There are ways to choose k elements from a set of n if repetitions are allowed. See Multiset.∙There are strings containing k ones and n zeros.∙There are strings consisting of k ones and n zeros such that no two ones are adjacent.∙The Catalan numbers are∙The binomial distribution in statistics is∙The formula for a Bézier curve.[edit] Binomial coefficients as polynomialsFor any nonnegative integer k, the expression can be simplified and defined as a polynomial divided by k!:This presents a polynomial in t with rational coefficients.As such, it can be evaluated at any real or complex number t to define binomial coefficients with such first arguments. These "generalized binomial coefficients" appear in Newton's generalized binomial theorem.For each k, the polynomial can be characterized as the unique degree k polynomial p(t) satisfying p(0) = p(1) = ... = p(k− 1) = 0 and p(k) = 1.Its coefficients are expressible in terms of Stirling numbers of the first kind, by definition of the latter:The derivative of can be calculated by logarithmic differentiation:[edit] Binomial coefficients as a basis for the space of polynomialsOver any field containing Q, each polynomial p(t) of degree at most d isuniquely expressible as a linear combination . The coefficient a k is the k th difference of the sequence p(0), p(1), …, p(k). Explicitly,[note 2][edit] Integer-valued polynomialsEach polynomial is integer-valued: it takes integer values at integer inputs. (One way to prove this is by induction on k, using Pascal's identity.) Therefore any integer linear combination of binomial coefficient polynomials is integer-valued too. Conversely, (3.5) shows that any integer-valued polynomial is an integer linear combination of these binomial coefficient polynomials. More generally, for any subring R of a characteristic 0 field K, a polynomial in K[t] takes values in R at all integers if and only if it is an R-linear combination of binomial coefficient polynomials.[edit] ExampleThe integer-valued polynomial 3t(3t + 1)/2 can be rewritten as[edit] Identities involving binomial coefficientsFor any nonnegative integers n and k,This follows from (2) by using (1 + x)n = x n·(1 + x−1)n. It is reflected in the symmetry of Pascal's triangle. A combinatorial interpretation of this formula is as follows: when forming a subset of k elements (from a set of size n), it is equivalent to consider the number of ways you can pick k elements and the number of ways you can exclude n−k elements. The factorial definition lets one relate nearby binomial coefficients. For instance, if k is a positive integer and n is arbitrary, thenand, with a little more work,[edit] Powers of -1A special binomial coefficient is ; it equals powers of -1:[edit] Series involving binomial coefficientsThe formulais obtained from (2) using x = 1. This is equivalent to saying that the elements in one row of Pascal's triangle always add up to two raised to an integer power. A combinatorial interpretation of this fact involving double counting is given by counting subsets of size 0, size 1, size 2, and so on up to size n of a set S of n elements. Since we count the number of subsets of size i for 0 ≤ i≤ n, this sum must be equal to the number of subsets of S, which is known to be 2n. That is, Equation 5 is a statement that the power set for a finite set with n elements has size 2n.The formulasandfollow from (2), after differentiating with respect to x (twice in the latter) and then substituting x = 1.Vandermonde's identityis found by expanding (1 + x)m (1 + x)n−m = (1 + x)n with (2). As is zero if k > n, the sum is finite for integer n and m. Equation (7a) generalizes equation (3). It holds for arbitrary, complex-valued m and n, the Chu-Vandermonde identity.A related formula isWhile equation (7a) is true for all values of m, equation (7b) is true for all values of j between 0 and k inclusive.From expansion (7a) using n=2m, k = m, and (4), one findsLet F(n) denote the n th Fibonacci number. We obtain a formula about the diagonals of Pascal's triangleThis can be proved by induction using (3) or by Zeckendorf's representation (Just note that the lhs gives the number of subsets of {F(2),...,F(n)} without consecutive members, which also form all the numbers below F(n+1)).Also using (3) and induction, one can show thatAgain by (3) and induction, one can show that for k = 0, ... , n−1as well aswhich is itself a special case of the result from the theory of finite differences that for any polynomial P(x) of degree less than n,[citation needed]Differentiating (2) k times and setting x = −1 yields this for, when 0 ≤ k< n, and the general case follows by taking linear combinations of these.When P(x) is of degree less than or equal to n,where a n is the coefficient of degree n in P(x).More generally for 13b,where m and d are complex numbers. This follows immediately applying (13b) to the polynomial Q(x):=P(m + dx)instead of P(x), and observing that Q(x) has still degree less than or equal to n, and that its coefficient of degree n is d n a n.The infinite seriesis convergent for k≥ 2. This formula is used in the analysis of the German tank problem. It is equivalent to the formula for the finite sumwhich is proved for M>m by induction on M.Using (8) one can deriveand.[edit] Identities with combinatorial proofsMany identities involving binomial coefficients can be proved by combinatorial means. For example, the following identity for nonnegativeintegers (which reduces to (6) when q = 1):can be given a double counting proof as follows. The left side counts the number of ways of selecting a subset of [n] of at least q elements, and marking q elements among those selected. The right side counts the same parameter, because there are ways of choosing a set of q marks and they occur in all subsets that additionally contain some subset of the remaining elements, of which there are 2n−q.The recursion formulawhere both sides count the number of k-element subsets of {1, 2, . . . , n} with the right hand side first grouping them into those which contain element n and those which don’t.The identity (8) also has a combinatorial proof. The identity readsSuppose you have 2n empty squares arranged in a row and you want to mark(select) n of them. There are ways to do this. On the other hand, you may select your n squares by selecting k squares from among the first n and n−k squares from the remaining n squares. This givesNow apply (4) to get the result.[edit] Continuous identitiesCertain trigonometric integrals have values expressible in terms of binomial coefficients:For andThese can be proved by using Euler's formula to convert trigonometric functions to complex exponentials, expanding using the binomial theorem, and integrating term by term.[edit] Generating functions[edit] Ordinary generating functionsFor a fixed n, the ordinary generating function of the sequenceis:For a fixed k, the ordinary generating function of the sequenceis:The bivariate generating function of the binomial coefficients is:[edit] Divisibility propertiesIn 1852, Kummer proved that if m and n are nonnegative integers and p isa prime number, then the largest power of p dividing equals p c, where c is the number of carries when m and n are added in base p. Equivalently,the exponent of a prime p in equals the number of positive integers j such that the fractional part of k/p j is greater than the fractionalpart of n/p j. It can be deduced from this that is divisible byn/gcd(n,k).A somewhat surprising result by David Singmaster (1974) is that any integer divides almost all binomial coefficients. More precisely, fix aninteger d and let f(N) denote the number of binomial coefficientswith n < N such that d divides . ThenSince the number of binomial coefficients with n < N is N(N+1) / 2, this implies that the density of binomial coefficients divisible by d goes to 1.Another fact: An integer n≥ 2 is prime if and only if all the intermediate binomial coefficientsare divisible by n.Proof: When p is prime, p dividesfor all 0 < k < pbecause it is a natural number and the numerator has a prime factor p but the denominator does not have a prime factor p.When n is composite, let p be the smallest prime factor of n and let k = n/p. Then 0 < p < n andotherwise the numerator k(n−1)(n−2)×...×(n−p+1) has to be divisible by n = k×p, this can only be the case when (n−1)(n−2)×...×(n−p+1) is divisible by p. But n is divisible by p, so p does not divide n−1, n−2, ..., n−p+1 and because p is prime, we know that p does not divide(n−1)(n−2)×...×(n−p+1) and so the numerator cannot be divisible by n. [edit] Bounds and asymptotic formulasThe following bounds for hold:Stirling's approximation yields the bounds:and, in general,for m≥ 2 and n≥ 1,and the approximationasThe infinite product formula (cf. Gamma function, alternative definition)yields the asymptotic formulasas .This asymptotic behaviour is contained in the approximationas well. (Here H k is the k th harmonic number and γis the Euler–Mascheroni constant).The sum of binomial coefficients can be bounded by a term exponential in n and the binary entropy of the largest n/ k that occurs. More precisely,for and , it holdswhere is the binary entropy of ε.[3]A simple and rough upper bound for the sum of binomial coefficients is given by the formula below (not difficult to prove)[edit] Generalizations[edit] Generalization to multinomialsBinomial coefficients can be generalized to multinomial coefficients. They are defined to be the number:whereWhile the binomial coefficients represent the coefficients of (x+y)n, the multinomial coefficients represent the coefficients of the polynomialSee multinomial theorem. The case r = 2 gives binomial coefficients:The combinatorial interpretation of multinomial coefficients is distribution of n distinguishable elements over r (distinguishable) containers, each containing exactly k i elements, where i is the index of the container.Multinomial coefficients have many properties similar to these of binomial coefficients, for example the recurrence relation:and symmetry:where (σi) is a permutation of (1,2,...,r).[edit] Generalization to negative integersIf , thenextends to all n.[edit] Taylor seriesUsing Stirling numbers of the first kind the series expansion around any arbitrarily chosen point z0 is[edit] Binomial coefficient with n=1/2The definition of the binomial coefficients can be extended to the case where n is real and k is integer.In particular, the following identity holds for any non-negative integer k :This shows up when expanding into a power series using the Newtonbinomial series :[edit] Identity for the product of binomial coefficientsOne can express the product of binomial coefficients as a linear combination of binomial coefficients:where the connection coefficients are multinomial coefficients. In terms of labelled combinatorial objects, the connection coefficients represent the number of ways to assign m+n-k labels to a pair of labelled combinatorial objects of weight m and n respectively, that have had their first k labels identified, or glued together, in order to get a new labelled combinatorial object of weight m+n-k. (That is, to separate the labels into 3 portions to be applied to the glued part, the unglued part of the first object, and the unglued part of the second object.) In this regard, binomial coefficients are to exponential generating series what falling factorials are to ordinary generating series.[edit] Partial Fraction DecompositionThe partial fraction decomposition of the inverse is given byand[edit] Newton's binomial seriesNewton's binomial series, named after Sir Isaac Newton, is one of the simplest Newton series:The identity can be obtained by showing that both sides satisfy the differential equation (1+z) f'(z) = αf(z).The radius of convergence of this series is 1. An alternative expression iswhere the identityis applied.[edit] Two real or complex valued argumentsThe binomial coefficient is generalized to two real or complex valued arguments using the gamma function or beta function viaThis definition inherits these following additional properties from Γ:moreover,The resulting function has been little-studied, apparently first being graphed in (Fowler 1996). Notably, many binomial identities fail:but for n positive (so −n negative). The behavior is quite complex, and markedly different in various octants (that is, with respect to the x and y axes and the line y= x), with the behavior for negative x having singularities at negative integer values and a checkerboard of positive and negative regions:∙in the octant it is a smoothly interpolated form of the usual binomial, with a ridge ("Pascal's ridge").∙in the octant and in the quadrant the function is close to zero.∙in the quadrant the function is alternatingly very large positive and negative on the parallelograms with vertices ( −n,m + 1),( −n,m),( −n− 1,m− 1),( −n− 1,m) ∙in the octant 0 > x > y the behavior is again alternatingly very large positive and negative, but on a square grid.∙in the octant − 1 > y > x + 1 it is close to zero, except for near the singularities.[edit] Generalization to q-seriesThe binomial coefficient has a q-analog generalization known as the Gaussian binomial coefficient.[edit] Generalization to infinite cardinalsThe definition of the binomial coefficient can be generalized to infinite cardinals by defining:where A is some set with cardinalityα. One can show that the generalized binomial coefficient is well-defined, in the sense that no matter whatset we choose to represent the cardinal number α, will remain the same. For finite cardinals, this definition coincides with the standard definition of the binomial coefficient.Assuming the Axiom of Choice, one can show that for any infinite cardinal α.[edit] Binomial coefficient in programming languagesThe notation is convenient in handwriting but inconvenient for typewriters and computer terminals. Many programming languages do not offer a standard subroutine for computing the binomial coefficient, but for example the J programming language uses the exclamation mark: k ! n .Naive implementations of the factorial formula, such as the following snippet in C:int choose(int n, int k) {return factorial(n) / (factorial(k) * factorial(n - k));}are prone to overflow errors, severely restricting the range of input values. A direct implementation of the multiplicative formula works well:unsigned long long choose(unsigned n, unsigned k) {if (k > n)return 0;if (k > n/2)k = n-k; // Take advantage of symmetrylong double accum = 1;unsigned i;for (i = 1; i <= k; i++)accum = accum * (n-k+i) / i;return accum + 0.5; // avoid rounding error}Another way to compute the binomial coefficient when using large numbers is to recognize thatlnΓ(n) is a special function that is easily computed and is standard in some programming languages such as using log_gamma in Maxima, LogGamma in Mathematica, or gammaln in MATLAB. Roundoff error may cause the returned value not to be an integer.[edit] See also∙Central binomial coefficient∙Binomial transform∙Star of David theorem∙Table of Newtonian series∙List of factorial and binomial topics∙Multiplicities of entries in Pascal's triangle∙Sun's curious identity[edit] Notes1.^See (Graham, Knuth & Patashnik 1994), which also defines fork< 0. Alternative generalizations, such as to two real or complex valued arguments using the Gamma function assign nonzero values tofor k< 0, but this causes most binomial coefficient identities to fail, and thus is not widely used majority of definitions. Onesuch choice of nonzero values leads to the aesthetically pleasing "Pascal windmill" in Hilton, Holton and Pedersen, Mathematicalreflections: in a room with many mirrors, Springer, 1997, but causes even Pascal's identity to fail (at the origin).2.^This can be seen as a discrete analog of Taylor's theorem. It isclosely related to Newton's polynomial. Alternating sums of this form may be expressed as the Nörlund–Rice integral.[edit] References1.^Nicholas J. Higham. Handbook of writing for the mathematicalsciences. SIAM. p. 25. ISBN0898714206.2.^ G. E. Shilov (1977). Linear algebra. Dover Publications.ISBN9780486635187.3.^ see e.g. Flum & Grohe (2006, p. 427)∙Fowler, David(January 1996). "The Binomial Coefficient Function".The American Mathematical Monthly(Mathematical Association ofAmerica) 103 (1): 1–17. doi:10.2307/2975209./stable/2975209∙Knuth, Donald E.(1997). The Art of Computer Programming, Volume 1: Fundamental Algorithms (Third ed.). Addison-Wesley.pp. 52–74. ISBN0-201-89683-4.∙Graham, Ronald L.; Knuth, Donald E.; Patashnik, Oren (1994).Concrete Mathematics (Second ed.). Addison-Wesley.pp. 153–256. ISBN0-201-55802-5.∙Singmaster, David (1974). "Notes on binomial coefficients. III.Any integer divides almost all binomial coefficients". J. LondonMath. Soc. (2)8: 555–560. doi:10.1112/jlms/s2-8.3.555.∙Bryant, Victor (1993). Aspects of combinatorics. Cambridge University Press.∙Arthur T. Benjamin; Jennifer Quinn, Proofs that Really Count: The Art of Combinatorial Proof, Mathematical Association of America,2003.∙Flum, Jörg; Grohe, Martin (2006). Parameterized Complexity Theory.Springer. ISBN978-3-540-29952-3./east/home/generic/search/results?SGWID=5-40109-22-141358322-0.This article incorporates material from the following PlanetMath articles, which are licensed under the Creative Commons Attribution/Share-Alike License: Binomial Coefficient, Bounds for binomial coefficients, Proof that C(n,k) is an integer, Generalized binomial coefficients.。
the greeks assumed that the structure of language
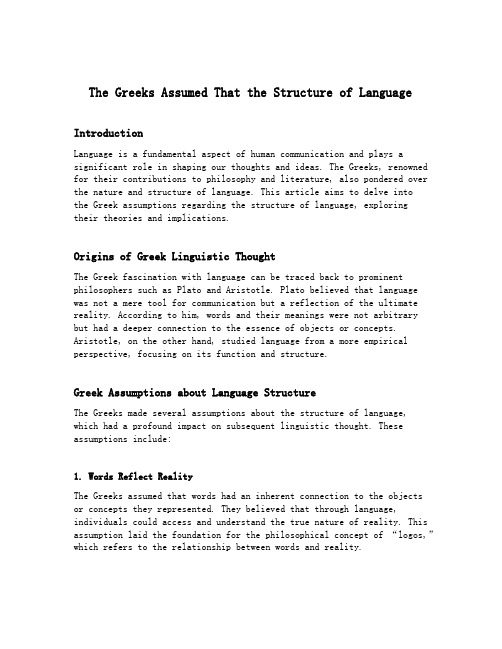
The Greeks Assumed That the Structure of LanguageIntroductionLanguage is a fundamental aspect of human communication and plays a significant role in shaping our thoughts and ideas. The Greeks, renowned for their contributions to philosophy and literature, also pondered over the nature and structure of language. This article aims to delve intothe Greek assumptions regarding the structure of language, exploringtheir theories and implications.Origins of Greek Linguistic ThoughtThe Greek fascination with language can be traced back to prominent philosophers such as Plato and Aristotle. Plato believed that language was not a mere tool for communication but a reflection of the ultimate reality. According to him, words and their meanings were not arbitrarybut had a deeper connection to the essence of objects or concepts. Aristotle, on the other hand, studied language from a more empirical perspective, focusing on its function and structure.Greek Assumptions about Language StructureThe Greeks made several assumptions about the structure of language,which had a profound impact on subsequent linguistic thought. These assumptions include:1. Words Reflect RealityThe Greeks assumed that words had an inherent connection to the objectsor concepts they represented. They believed that through language, individuals could access and understand the true nature of reality. This assumption laid the foundation for the philosophical concept of “logos,” which refers to the relationship between words and reality.2. Language Is Composed of Basic ElementsThe Greeks recognized that language could be broken down into smaller units with distinctive meanings. They postulated that these basic elements, known as morphemes, combined to form words. This assumption paved the way for the development of morphological analysis in linguistics, which studies the internal structure of words.3. Syntax and Grammar Govern LanguageAncient Greek philosophers acknowledged the importance of syntax and grammar in organizing and conveying meaning. They recognized that language followed specific rules and structures that determined the relationships between words in a sentence. This assumption laid the groundwork for syntactical analysis, which explores the arrangement of words and phrases in a sentence.4. Language Is InnateThe Greeks assumed that the ability to acquire and understand language was innate to humans. They believed that language proficiency stemmed from natural predispositions rather than external influences. This assumption aligns with modern theories of language acquisition, such as Noam Chomsk y’s concept of a Universal Grammar.Implications of Greek Linguistic ThoughtThe Greek assumptions about language structure had far-reaching implications for various disciplines, including linguistics, philosophy, and literature. Some of these implications are:1. Language as a Mirror of RealityThe concept of language reflecting reality influenced subsequent philosophical and metaphysical thought. It prompted thinkers to explore the relationship between language, perception, and knowledge. This exploration ultimately shaped diverse philosophical schools, such as phenomenology and hermeneutics.2. Development of Linguistic AnalysisThe Greek assumptions regarding the composition of language elements and the importance of syntax and grammar laid the groundwork for linguistic analysis. These assumptions influenced the development of structural linguistics, generative grammar, and other linguistic theories that investigate the form and function of language.3. Influence on Literary StylesGreek linguistic thought permeated literary works, influencing writing styles and literary devices. Writers began incorporating rhetorical techniques, such as metaphors and analogies, to convey deeper meanings and evoke emotional responses. These techniques shaped the foundations of poetry, prose, and dramatic literature.4. Evolution of Language EducationThe Greek assumptions about language being innate and governed by rules contributed to the development of language education methodologies. They inspired instructional approaches that emphasize the systematic teaching of grammar, syntax, and vocabulary. These approaches continue to influence language teaching methodologies worldwide.ConclusionThe Greeks’ assumptions about the structure of language have left an indelible mark on human understanding and exploration of linguistic phenomena. Their belief that language reflects reality, the recognition of basic language elements, the importance of syntax and grammar, and the innate nature of language have shaped various disciplines. From philosophy to linguistics, and literature to education, the Greek assumptions continue to shape our understanding and appreciation of language.。
Definition and Interpretation(定义及解释规则翻译)

Definition and Interpretation(定义及解释规则翻译)翻译实践1. "Affiliate" means any person or company that directly or indirectly controls a Party or is directly or indirectly controlled by a Party, including a Party's parent or subsidiary, or is under direct or indirect common control with such Party. For the purpose of this Agreement, "control" shall mean either the ownership of fifty per cent (50%) or more of the ordinary share capital of the company carrying the right to vote at general meetings or the power to nominate a majority of the board of directors of the Company.2. "Proprietary Know-how" shall mean processes, methods and manufacturing techniques, experience and other information and materials including but not limited to the Technical Information and Technical Assistance supplied or rendered by the Licensor to the Licensee hereunder which have been developed by and are known to the Licensor on the date hereof and/or which may be further developed by the Licensor or become known to it during the continuance of this Agreement excepting, however, any secret know-how acquired by the Licensor from third parties which the Licensor is precluded from disclosing to the Licensee.3. "Proprietary Information" means the information, whether patentable or not, disclosed to the CJV by either Party or its Affiliates or disclosed by the CJV to either Party or its Affiliates during the term of this Contract, including technology, inventions, creations, know-how, formulations, recipes, specifications, designs, methods, processes, techniques, data, rights, devices, drawings, instructions, expertise, trade practices, trade secrets and such commercial, economic, financial or other information as is generally treated as confidential by the disclosing Party, its Affiliates, or the CJV, as the case may be; provided that when such information is in unwritten or intangible form, the disclosing Party, its Affiliates or the CJV shall, within one month of making the disclosure, provide the other Partyand/or the CJV with a written confirmation that such information constitutes its Proprietary Information.4. "Encumbrances" include any option, right to acquire, right of preemption, mortgage, charge, pledge, lien, hypothecation, title creation, right of set-off, counterclaim, trust arrangement or other security or any equity or restriction (including any relevant restriction imposed under the relevant law).5. In this Agreement, unless the context otherwise requires:a) headings are for convenience only and shall not affect the interpretation - 27 -of this Agreement;b) words importing the singular include the plural and vice versa; c) words importing a gender include any gender;d) an expression importing a natural person includes any company, partnership, joint venture, association, corporation or other body corporate and any governmental agency;e) a reference to any law, regulation or rule includes all laws, regulations, or rules amending, consolidating or replacing them, and a reference to a law includes all regulations and rules under that law;f) a reference to a document includes an amendment or supplement to, or replacement or novation of, that document;g) a reference to a party to any document includes that party's successors and permitted assigns;h) a reference to an agreement includes an undertaking, agreement or legally enforceable arrangement or understanding whether or not in writing;i) a warranty, representation, undertaking, indemnity, covenant or agreement on the part of two or more persons binds them jointly and severally; andj) the schedules, annexures and appendices to this Agreement shall form an integral part of this Agreement.参考译文:定义、解释1. “关联公司”指直接或间接控制一方(包括其母公司或子公司)或受一方直接或间接控制,或与该方共同受直接或间接控制的任何人或公司。
隐含作者、第二自我与自我的多重性--小议叙事理论中“隐含作者”概念的争议与潜力
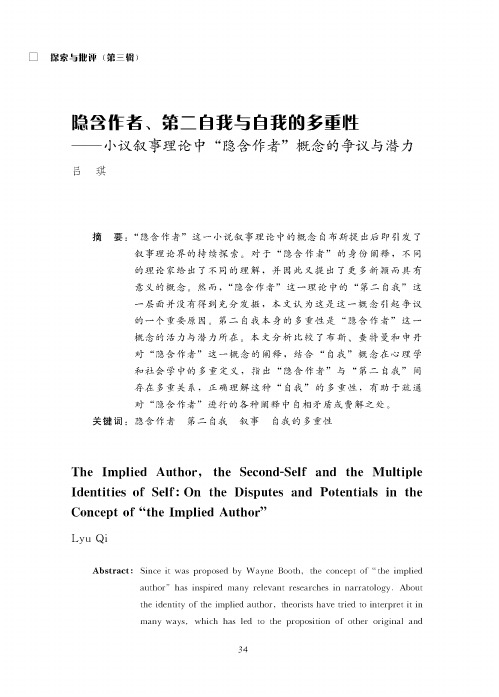
隐含作者、第二自我与自我的多重性----小议叙事理论中“隐含作者”概念的争议与潜力吕琪摘要:“隐含作者”这一小说叙事理论中的概念自布斯提出后即引发了叙事理论界的持续探索”对于“隐含作者”的身份阐释,不同的理论家给出了不同的理解,并因此又提出了更多新颖而具有意义的概念。
然而,“隐含作者”这一理论中的“第二自我”这一层面并没有得到充分发掘,本文认为这是这一概念引起争议的一个重要原因”第二自我本身的多重性是“隐含作者”这一概念的活力与潜力所在”本文分析比较了布斯、查特曼和申丹对“隐含作者”这一概念的阐释,结合“自我”概念在心理学和社会学中的多重定义,指出“隐含作者”与“第二自我”间存在多重关系,正确理解这种“自我”的多重性,有助于疏通对“隐含作者”进行的各种阐释中自相矛盾或费解之处”关键词:隐含作者第二自我叙事自我的多重性The Implied Author,the Second-Self and the Multiple Identities of Self:On the Disputes and Potentials in the Concept of“the Implied Author"Lyu QiAbstract:Since it was proposed by Wayne Booth,the concept of"the implied author”has inspired many relevant researches in narratology.Aboutthe identity of the implied author ,theorists have tried to interpret t inmany ways ,which has led to the proposition of other original andmeaningful concepts.However,the idea of the second-self in theconcept of the implied author has not been thoroughly discussed yet,whichthise s aytends oconsiderasoneof hemosimporan reasonsforthedispuesaroundthisconcep.In some way,he dynamics andpoenials ofthe conceptofthe implied auhorlieinthe mulipleidentities embedded in the second-self.This essay compares theinterpretations of three theorists,Booth,Chatman and Shen,andpointsoutthatthecomplicatedrelationshipbetweentheimpliedauthorandthesecond-selfhasbeencommonlyneglectedandthushasarousedcontradictionsorconfusionintheirinterpretationsKeywords:the Implied Author;the Second-self;Narratology;Multiple identities ofself“隐含作者”这一叙事理论概念自韦恩•布斯(WayneBooth)在《小说修辞学》(1961)中提出后即引起了批评家和理论家极大的关注。
第九讲 interpretation Guiding Lesson
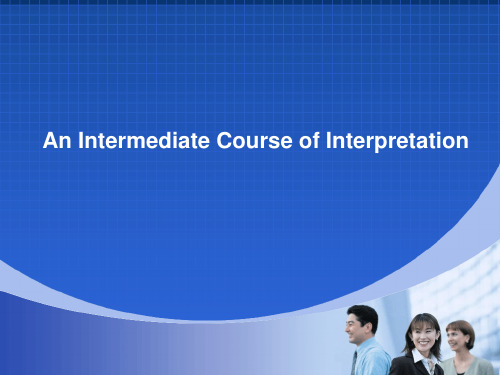
III Interpretation and the Interpreter
Professional classification: Consecutive interpretation Simultaneous interpretation Sight interpretation (Sight simultaneous interpretation) Court interpretation (庭审)
III Interpretation and the Interpreter
1)
According to interpreting modes Alternating interpretation Consecutive interpretation Simultaneous interpretation Whispering interpretation Sight interpretation Sign language interpretation
III Interpretation and the Interpreter
The definition of Interpretation
1) Interpretation is a vocal translation to the information delivered by vocal utterance and text. (Shuttleworth & Cowie, 1997:82) 2) In its purest form, consecutive interpretation is a mode in which the interpreter begins their interpretation of a complete message after the speaker has stopped producing the source utterance. At the time that the interpretation is rendered the interpreter is the only person in the communication environment who is producing a message. By Roberto Santiago
Enumerations of Permutations by Circular Descent Sets
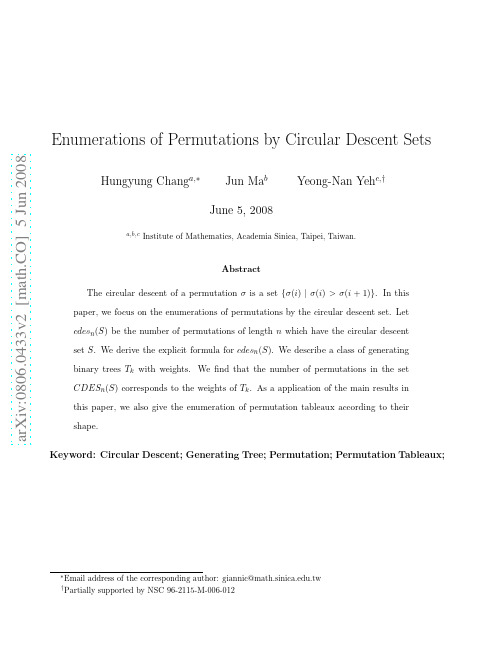
Keyword: Circular Descent; Generating Tree; Permutation; Permutation Tableaux;
∗ †
Email address of the corresponding author: giannic@.tw Partially supported by NSC 96-2115-M-006-012
Institute of Mathematics, Academia Sinica, Taipei, Taiwan.
Abstract The circular descent of a permutation σ is a set {σ (i) | σ (i) > σ (i + 1)}. In this paper, we focus on the enumerations of permutations by the circular descent set. Let cdesn (S ) be the number of permutations of length n which have the circular descent set S . We derive the explicit formula for cdesn (S ). We describe a class of generating binary trees Tk with weights. We find that the number of permutations in the set CDESn (S ) corresponds to the weights of Tk . As a application of the main results in this paper, we also give the enumeration of permutation tableaux according to their shape.
- 1、下载文档前请自行甄别文档内容的完整性,平台不提供额外的编辑、内容补充、找答案等附加服务。
- 2、"仅部分预览"的文档,不可在线预览部分如存在完整性等问题,可反馈申请退款(可完整预览的文档不适用该条件!)。
- 3、如文档侵犯您的权益,请联系客服反馈,我们会尽快为您处理(人工客服工作时间:9:00-18:30)。
(5) (6) (7)
m
S2m,n (q ) = (1 − q
m
)
k =0
− q 2 )m−k Qm,m−k (q 2 ) ([n43; 2 )
i=0
1
1
T2m,n (q ) =
k =1
(−q n )m−k
Gm,m−k (q ) ([n][n m−k m−i ) (1 + q i=0
+ 1])k ,
and T2m−1,n (q ) = (−1) +
m+n
Hm,m−1 (q )
1
1 2
q (m− 2 )n (1 + q 2 )m
1 1
1
m−1 i=0 (1
1
+ q m−i− 2 )
m−k i=0 (1
1
1 − q n+ 2 1 − q2
1
m k =1
(−q n )m−k
Hm,m−k (q 2 )([n][n + 1])k−1 (1 + q 2 )m−k+1 + q m−i− 2 )
Table 2. Values of Qm,k (q ) for 0 ≤ k < m ≤ 4.
k\m 0 1 2 3 1 1 2 1 1 3 1 2q 2 + q + 2 2q 2 + q + 2 4 1 3q 4 + 2q 3 + 4q 2 + 2q + 3 (q 2 + q + 1)(5q 4 + q 3 + 9q 2 + q + 5) (q 2 + q + 1)(5q 4 + q 3 + 9q 2 + q + 5)
Proof. Using the definition (9) of the complete homogeneous functions we have
k\m 0 1 2 3 4 0 1 1 1 2 1 1 3 1 2(q + 1) 2(q + 1) 4 1 3q 2 + 4q + 3 (q + 1)(5q 2 + 8q + 5) (q + 1)(5q 2 + 8q + 5) 5 1 2(q + 1)(2q 2 + q + 2) (q + 1)(9q 4 + 19q 3 + 29q 2 + 19q + 9) 2(q + 1)2 (q 2 + q + 1)(7q 2 + 11q + 7) 2(q + 1)2 (q 2 + q + 1)(7q 2 + 11q + 7)
n≥0
1 . (1 − x1 t)(1 − x2 t) . . . (1 − xr t)
For r, s ≥ 0, let hn ({1}r , {q }s ) denote the n-th complete homogeneous functions in r + s variables, of which r are specialized to 1 and the others to q , i.e., hn ({1}r , {q }s )z n = 1 (1 − z )r (1 − qz )s . (9)
1. Introduction In the early seventeenth century, Johann Faulhaber [1] (see also [5]) considered the sums of m and provided formulas for the coefficients f powers Sm,n = n m,k (0 ≤ m ≤ 8) in k =1 k S2m+1,n = 1 2
n≥0
By convention, hn ({1}r , {q }s ) = 0 if r < 0 or s < 0. For convenience, we also write hn ({1, q }r ) instead of hn ({1}r , {q }r ). We first prove the following result. Lemma 2.1. Let a and b be non-negative integers, then [l+1] [l]−[l+1]z − k l 2 q [l] 1 zm = hm−2k ({1}k+a , {q }k+b ) [l]−[l+1]z + 2 [l] [2l] m≥0 k ≥0 ql [l]−[l+1]z +
arXiv:math/0506274v1 [math.CO] 14 Jun 2005
COMBINATORIAL INTERPRETATIONS OF THE q -FAULHABER AND ´ COEFFICIENTS q -SALIE
VICTOR J. W. GUO, MARTIN RUBEY, AND JIANG ZENG Dedicated to Xavier Viennot on the occasion of his sixtieth birthday Abstract. Recently, Guo and Zeng discovered two families of polynomials featuring in a q analogue of Faulhaber’s formula for the sums of powers and a q -analogue of Gessel-Viennot’s formula involving Sali´ e’s coefficients for the alternating sums of powers. In this paper, we show that these are polynomials with symmetric, nonnegative integral coefficients by refining Gessel-Viennot’s combinatorial interpretations.
´ COEFFICIENTS q -FAULHABER AND q -SALIE
3
Table 3. Values of Gm,k (q ) for 0 ≤ k < m ≤ 5.
k\m 0 1 2 3 4 1 1 2 1 2 3 1 3(q + 1) 6(q + 1) 4 1 4(q 2 + q + 1) 2(q + 1)(5q 2 + 7q + 5) 4(q + 1)(5q 2 + 7q + 5) 5 1 5(q + 1)(q 2 + 1) 5(q + 1)(3q 4 + 4q 3 + 8q 2 + 4q + 3) 5(q + 1)2 (7q 4 + 14q 3 + 20q 2 + 14q + 7) 10(q + 1)2 (7q 4 + 14q 3 + 20q 2 + 14q + 7)
Recall that a polynomial f (x) = a0 + a1 x + · · · + an xn of degree n has symmetric coefficients if ai = an−i for 0 ≤ i ≤ n. The tables above suggest that the coefficients of the polynomials Pm,k , Qm,k , Gm,k and Hm,k are nonnegative and symmetric. The aim of this paper is to prove this fact by showing that the coefficients count certain families of non-intersecting lattice paths. 2. Inverses of matrices Recall that the n-th complete homogeneous functions in r variables x1 , x2 , . . . , xr has the following generating function: hn (x1 , . . . , xr )tn =
1
.
(8)
Comparing with (3) and (4), we have fm,k = (−1)m−k and sm,k = (−1)m−k 2k−m Gm,m−k (1), k! Pm,m−k (1) (m + 1)!
but the numbers corresponding to Qm,k (1) and Hm,k (1) do not seem to be studied in the literature. The first values of Pm,k , Qm,k , Gm,k and Hm,k are given in Tables 1–4, respectively. Table 1. Values of Pm,k (q ) for 0 ≤ k < m ≤ 5.
m
sm,k (n(n + 1))k .
k =1
(2)
In particular, they proved that the Faulhaber coefficients fm,k and the Sali´ e coefficients sm,k count certain families of non-intersecting lattice paths. Recently, two of the authors [4], continuing work of Michael Schlosser [7], Sven Ole Warnaar [8] and Kristina Garrett and Kristen Hummel [2], have found q -analogues of (1) and (2). More k −q k precisely, setting [k] = 11 i=1 [k ], and −q , [k ]! =