Measuring and modelling the group membership in the Internet
质量管理与卓越绩效 (10)
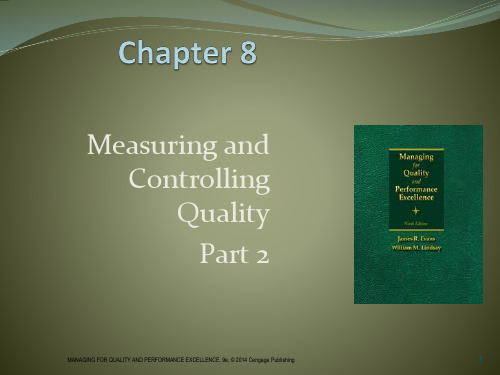
3. Determine initial control limits
Compute the upper and lower control limits Draw the center line (average) and control limits on the
chart
© 20114 Cengage Learning. All Rights Reserved. May not be scanned, copied or duplicated, or posted to a publicly accessible website, in whole or in part..
8
La Ventana Case Data
© 20114 Cengage Learning. All Rights Reserved. May not be scanned, copied or duplicated, or posted to a publicly accessible website, in whole or in part..
4
Constructing x-bar and R-Charts
Collect k = 25-30 samples, with sample sizes generally between n = 3 and 10
美赛 数学建模 埃博拉
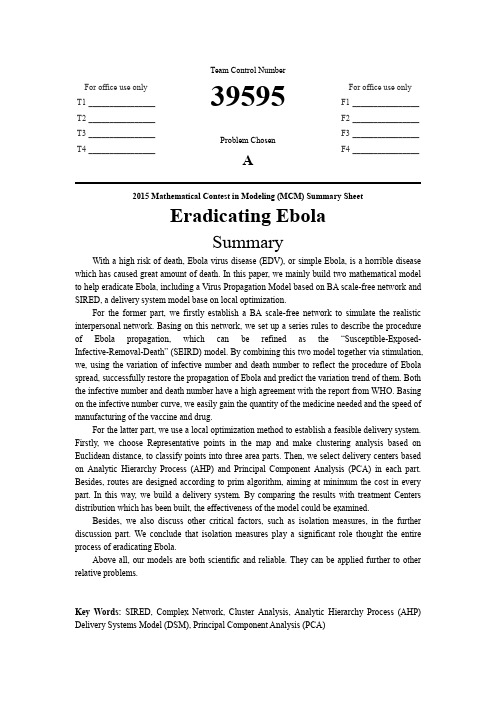
For office use only T1________________ T2________________ T3________________ T4________________Team Control Number39595Problem ChosenAFor office use onlyF1________________F2________________F3________________F4________________2015 Mathematical Contest in Modeling (MCM) Summary SheetEradicating EbolaSummaryWith a high risk of death, Ebola virus disease (EDV), or simple Ebola, is a horrible disease which has caused great amount of death. In this paper, we mainly build two mathematical model to help eradicate Ebola, including a Virus Propagation Model based on BA scale-free network and SIRED, a delivery system model base on local optimization.For the former part, we firstly establish a BA scale-free network to simulate the realistic interpersonal network. Basing on this network, we set up a series rules to describe the procedure of Ebola propagation, which can be refined as the “Susceptible-Exposed- Infective-Removal-Death” (SEIRD) model. By combining this two model toget her via stimulation, we, using the variation of infective number and death number to reflect the procedure of Ebola spread, successfully restore the propagation of Ebola and predict the variation trend of them. Both the infective number and death number have a high agreement with the report from WHO. Basing on the infective number curve, we easily gain the quantity of the medicine needed and the speed of manufacturing of the vaccine and drug.For the latter part, we use a local optimization method to establish a feasible delivery system. Firstly, we choose Representative points in the map and make clustering analysis based on Euclidean distance, to classify points into three area parts. Then, we select delivery centers based on Analytic Hierarchy Process (AHP) and Principal Component Analysis (PCA) in each part. Besides, routes are designed according to prim algorithm, aiming at minimum the cost in every part. In this way, we build a delivery system. By comparing the results with treatment Centers distribution which has been built, the effectiveness of the model could be examined.Besides, we also discuss other critical factors, such as isolation measures, in the further discussion part. We conclude that isolation measures play a significant role thought the entire process of eradicating Ebola.Above all, our models are both scientific and reliable. They can be applied further to other relative problems.Key Words:SIRED, Complex Network, Cluster Analysis, Analytic Hierarchy Process (AHP) Delivery Systems Model (DSM), Principal Component Analysis (PCA)Table of Content1.Introduction (1)1.1.Background (1)1.2.Restatement of the Problem (1)2.Assumptions and Notions (1)2.1.Assumptions and Justifications (1)2.2.Notions (2)3.The Virus Propagation Model Based on Complex Networks and SEIRD Model (3)3.1.Model Overview (3)plex Network Model (3)3.2.1.Small-World Network Model (3)3.2.2.BA Scale-Free Network Model (4)3.3.SIR-Based SEIRD Model (5)3.3.1.SIR Model (5)3.3.2.SEIRD Model (6)3.4.The Study of Infection Rate, Recovery Rate and Death Rate Based on the LeastSquare Method (6)3.4.1.The Relevant Calculation about Infection Rate (6)3.4.2.The Relevant Calculation about the Recovery Rate (7)3.4.3.The Relevant Calculation of Death Rate (7)3.5.The Simulation of the Transmission of Ebola Virus (8)3.5.1.The Simulation of Complex Network Model (9)3.5.2.The Simulation of Virus Transmission (10)3.6.Results and Result Analysis (11)3.6.1. A Complex Network Simulation Results Model (11)3.6.2.The Spread of the Virus the Simulation Results (12)4.Delivery Systems Model(DSM) Based on Local Optimization (13)4.1.Model Overview (13)4.2.Cluster Division Based on Cluster Analysis (14)4.3.Delivery Centers and Routes Planning Based on AHP and PCA (17)4.3.1The Three-hierarchy Structure (18)4.3.2Analytic Hierarchy Process and Principal Component Analysis for DSM .. 194.3.3Obtain the Centers (21)4.3.4Obtain the Routes (22)4.4.Results and Analysis (23)5.Other Critical Factors for Eradicating Ebola (24)5.1The Effect of the Time to Isolate Ebola on Fighting against Ebola (24)5.2The Effect of Timely Medical Treatment to Isolate Ebola on Fighting against Ebola (25)6.Results and results analysis (26)6.1.The virus propagation model based on complex networks (26)6.1.1.The contrast and analysis concerning the results of simulation and thereality (26)6.1.2.Forecast for the future (28)6.2.Delivery Systems Model Based on Local Optimization (28)7.Strengths and Weaknesses (29)7.1.Strengths (29)7.2.Weaknesses (29)8.Conclusion (30)9.Reference (30)10.Appendix (1)1.Introduction1.1.BackgroundWith a high risk of death, Ebola virus disease (EDV), or simple Ebola, is a disease of humans and other primates. Since its first outbreak in March 2014, over 8000 people have lost their lives. And till 3 February 2015, 22,495 suspected cases and 8,981 deaths had been reported. [1] However, this disease spreads only by direct contact with the bold or body fluids of a person who has developed symptoms of the disease. Following infection, patients will typically remain asymptomatic for a period of 2-21 days. During this time, tests for the virus will be negative, and patients are not infectious, posing no public health risk.[2] And recently, the world medicine association has announced that their new medication could stop Ebola and cure patients whose disease is not advanced. Thus, a feasible delivery system is in great demand and measures to eradicating Ebola should be taken immediately.1.2.Restatement of the ProblemWith the background mentioned above, we are required to build a model to help eradicate Ebola, which can be decomposed as:●Build a model, which can estimate the suspects number, exposed number,infect number, death number and recover numbers, to describe the spreadprocedure of the Ebola from its very beginning to the future.●Build an optimized model to help establish a possible and feasible deliverysystem including selecting delivery location and delivery system networkdesign.●Estimate of the quantity of the needed medicine and manufacturing speed ofvaccine or drug, based on the results of our models.●Discuss other critical factors which help eradicate Ebola.2.Assumptions and Notions2.1.Assumptions and JustificationsTo simplify the problem, we make the following basic assumptions, each of which is properly justified.●Assume that there is no people flow between countries after outbreak ofdisease in the country.After the outbreak, countries usually will ban thecontact between locals and foreigners to minimize the incoming of the virus.●Assuming that virus infection rate and fatality rate will not change bythe change of regions.Virus infection rate and fatality rate are largelydetermined by the nature of the virus itself. The different between differentregions just have a little effect and it will be ignored.●Assume that there are only rail, road and aircraft for transportation. Inthe West Africa, waterage is rare. Rail, and road are for nearby transportationwhile aircraft is for faraway.2.2. NotionsAll the variables used in this paper are listed in Table 2.1 and Table 2.2.Table 2.1 Symbols for Virus Propagation Model(VPM)SymbolDefinition Units βInfection Rate for the Susceptible in SIR Model or SEIRD Model unitless γRecovery Rate for the Infective in SIR Model unitless rRateRecovery Rate for the Infective in SEIRD Model unitless dRateDeath Rate for the Infective in the SIR Model or SEIRD Model unitless ∆N iNew Patients on a Daily Basis person N i−1The Total Number of Patients in the Previous Day person nThe Average Degree of Each Node in the Network unitless tTime S ∆D iThe New Death Toll on a Daily Basis person D i−1The Total Number of Patients in the Previous Day person moThe Initial Number of Nodes in the BA Scale-Free Network node mThe Number of Added Sides from One New Node in the BA Scale-Free Network side ∏iThe Probability for the Connection between New Nodes and the Existing Node I in the BA Scale-Free Network unitless NThe Total Number of Nodes in the BA Scale-Free Network node k iThe Degree of Node I in the BA Scale-Free Network unitless eThe Number of Sides in the BA Scale-Free Network side n SThe Number of the Susceptible in the SEIRD Model person n EThe Number of the Exposed in the SEIRD Model person n IThe Number of the Infective in the SEIRD Model person n RThe Number of the Removal in the SEIRD Model person n DThe Number of the Dead in the SEIRD Model person RThe Probability of Virus Propagation from the Recovered unitless RandomE The Number of the Exposed Who Have Reached the Exposed Time Limit Ranging from 2 to 21 Days at the Current Momentperson n E(一天)t The Number of the Exposed Who Have Reached the Final Day of the ExposedTime Limit yet not quarantine personTable 2.2 Symbols for Delivery Systems Model(DSM)SymbolDefinition Units Athe judging matrix unitless a ijThe element of judging matrix unitless λmaxthe greatest eigenvalue of matrix A unitless CI the indicator of consistency check unitlessCR the consistency ratio unitlessRI the random consistency index unitlessCW the weight vector for criteria level unitlessAW the weight vector for alternatives level unitlessY the evaluation grade unitlessV A set of points unitlessV i,V j the point of V unitlessE A set of edges unitlessDis ij The real distance of i and j unitless Arrive ij Judging for whether there is an side between i and j unitless3.The Virus Propagation Model Based on Complex Networks and SEIRD Model3.1.Model OverviewWe aim to build a Susceptible-Exposed-Infective-Removal-Death (SEIRD) virus propagation model which is based on Susceptible-Infective-Removal (SIR) model. The aimed model is featured by complex networks, which exhibit two statistical characteristics, including the Small-World Effect and the Scale-Free Effect. These characteristics could produce relatively real person-to-person and region-to-region networks. Through the statistics of the existing patients and deaths, we will try to find the relationship among the infection rate, the recovery rate and the death rate with the change of time. Then, with the help of SEIRD model, these statistics would be used to simulate the current situation concerning the number of the susceptible, the exposed, the infective, the recovered and the dead and conduct the prediction of the future.plex Network ModelResearches have shown that the person-to-person networks in real life exhibit the Small-World Effect and Scale-Free Effect. Here we will introduce the Small-World Network Model by Watts and Strogatz, and the Scale-Free Network Model by Barabdsi and Albert [3].3.2.1.Small-World Network ModelSince random network and regular network could neither properly present some important characteristics of real network, Watts and Strogatz proposed a new network model between the random network and regular network in 1998, namely WS Small-World Network Model, the construction algorithm of which is as follows.Start from a regular network: consider a regular network which contains N nodes, and these nodes form a ring. Each node is linked with its adjacent nodes, the number of which is K/Z on both left side and right side. Also, K is an even number.Randomized re-connection: the probability P will witness a random re connection with each side in the network. In other words, an endpoint of a certain side will remain unchanged and the other endpoint would be the node in the random selection.There are two rules. The first is two different nodes will at most have one side. The second is every node cannot have a side which is connected with this node [4].Randomized re-connection in construction algorithm of the WS Small-World Model may damage connectivity of network, so Newman and Watts improved this model in 1999. The new one is called NW Small-World Model, the construction algorithm of which is as follows.Start from a regular network: consider a regular network which contains N nodes, and these nodes form a ring. Each node is linked with its adjacent nodes, the number of which is K/Z on both left side and right side. Also, K is an even number.Randomized addition of sides: the probability P will witness the random selection of two nodes and the subsequent addition of a side between these two nodes. There are two rules. The first is two different nodes will at most have one side. The second is every node cannot have a side which is connected with this node[4].The network constructed by the two models are shown in Fig 3.1.Fig 3. 1 WS Small-World network and NW Small-World network3.2.2.BA Scale-Free Network ModelIn October, 1999, Barabdsi and Albert published article in Science called "Emergence of Scaling in Random Networks" [5], which proposed an important discovery that the distribution function of connectivity for many complex networks exhibit a form of power laws. Since no obvious length characteristics of connectivity could be seen among nodes in these networks, so they are called scale-free networks.As for the cause of power laws distribution, Barabasi and Albert believe that many previous network models did not take into account two important characteristics of actual networks: the consistent expanding of network and the nature of new nodes’ prior connection in the network. These characteristics will not only make node degrees which are relatively larger increase much faster, but also produce more new nodes, thus node degrees will become even larger. Then we could see the Matthew Effect. [4]Based on the Scale-Free Network, Barabasi and Albert proposed a scale-free network model, called BA Model, the construction algorithm of which is as followsi.The expanding of network: start from a network which has Mo nodes, thenintroduce a new node after each time interval and connect this node with mnodes. The prerequisite is m≤m0.ii.Prior connection: the probability between a new node and an existing node iis ∏i, the node degree of i is k i, and the node degree of j is k j. These threefactors should satisfy the following equation.∏i=k i/∑k jj(3-1) After t steps,this algorithm could lead to a network featured by m0+t nodes and m×t sides.The network of BA Model is shown in Fig 3.2.Fig 3.2 BA Scale-Free Network3.3.SIR-Based SEIRD Model3.3.1.SIR ModelSIR is the most classic model in the epidemic models, in which S represents susceptible, I represents infective and R represents removal. Specifically, the susceptible are those who are not infected, yet vulnerable to be infected after contact with the confirmed patients. The infective are those who have got the disease and could pass it to the susceptible. As for the removal, it refers to those who are quarantined or immune to a certain disease after they have recovered.In the disease propagation, SIR Model is built with the infection rate as β, the recovery rate as γ, which is shown in Fig 3.3:Fig 3.3 SIR propagation modelThis model is suitable epidemics which have the following features: no latency, only propagated by the patients, difficult to cause death, patients are immune to this disease after recovery once and for all. As for the Ebola virus, this model is insufficient to present the propagation process. Therefore, we propose the SEIRD model based on the SIR model and overcome the defects of the SIR model, thus making the SEIRD model more suitable for the research of Ebola virus.3.3.2.SEIRD ModelThe characteristics of Ebola virus are shown in Table 3.1:Table 3.1 The characteristics of Ebola virus characteristicsDetailsLatency Exposed period ranging from 2 to 21days with no infectivity during this stage[2]Retention After the recovery, there is still a certain chance of propagation[6]Immunity Recovery is accompanied with lifelong immunityTherefore, it is needed to add E (the exposed) and D (the dead) in the SIR model.E represents those who have been infected, have no symptoms, and not contagious. But within 2-21 days, the exposed will become contagious. D represents those who are dead and not contagious.From Table 3.1, we know that Ebola virus has the feature of retention, because even when they are in the state of removal, it is still possible these recovered will be infectious.In the process of disease propagation, SEIRD has witnessed the infection rate as β and the recovery rate as rRate and dRate,which is shown as follows.Fig 3.4SEIRD propagation model3.4.The Study of Infection Rate, Recovery Rate and Death RateBased on the Least Square MethodAs for the calculation of infection rate, recovery rate and death rate, we could make use of the least square method to match the daily confirmed patients and the dead toll, thus getting the function about the relationship with the passage of time.And we choose the relevant data from Guinea since it is in severely hit by the Ebola outbreak in West Africa. The variance regarding the total number of patients and the total death toll could be seen in Appendix 11.1.3.4.1.The Relevant Calculation about Infection RateThe infection rate refers to the probability that the susceptible are in contact (here it refers to the contact with body fluids) with un-isolated patients and infected with the virus. For each infective patient, the number of side is the node degree, namely the number whom he or she could infect. Therefore, the infection rate could be calculatedin this way: the number of new patients each day divides the possible number of whom each confirmed patient could infect. The number of new patients is ∆N i. The total number of patients in the previous day is N i−1. The average node degree in the network is n. The equation is as follows.β=∆N i/(N i−1×n)(3-2) The β could be calculated based on the total number o f patients in Guinea (see Appendix 11.1). Then with time data and the method of least square method, we could do data fitting and calculate the time-dependent equation. The fitting image is listed in Fig 3.5.Fig 3.5 The fitting result image of β with the passage of timeThe result of fitting curve is:β=0.0367×t−0.3189/n(3-3) And n is the average degree of person-to-person network in the process of simulation.3.4.2.The Relevant Calculation about the Recovery RateThe recovery rate refers to the probability of recovery for those who have been infected. Since at present, few instances of recovery from Ebola disease could be witness in the world (probability is almost close to zero), so the rRate here is set to be 0.001.3.4.3.The Relevant Calculation of Death RateThe death rate refers to the probability that patients become dead in process of treatment. And the death rate is calculated in the following way: the total number of new deaths every day divides the total number of patients in the previous day. The total number of new deaths in a new day is ∆D i. The total number of patients in the previous day is D i−1. The equation isdRate=∆D i/D i−1(3-4) The dRate could be calculated based on the total number of dead patients and the total number of patients in Guinea (see Appendix 11.1). Then with time data and the method of least square method, we could do data fitting and calculate the time-dependent equation. The fitting image is listed in Fig 3.6.Fig 3.6 The fitting result image of dRate with the passage of time The result of fitting curve is:dRate=(−6.119e−07)×t2 −0.0001562×t + 0.01558(3-5) 3.5.The Simulation of the Transmission of Ebola VirusThe simulation for Ebola will mainly be divided into two aspects, namely the simulation of complex network model and that of virus spread. The related flow chart will be shown in Fig 3.7.Fig 3.7 Flow chat of Stimulation of Ebola Virus Transmission3.5.1.The Simulation of Complex Network ModelFrom the previous introduction about complex network model, BA Scale-Free Network Model has displayed the Matthew Effect, which means the stronger would be much stronger and the weaker would be much weaker. In social networks, this effect is also widely seen. Take one person who just joins in a group for an example, he would normally contact with those who have the largest circle of friends. Therefore, those who get the least friends can hardly know more new friends. This finally leadsto a phenomenon that the person who is most acquainted will have more and more friends and vice versa.Based on this, the BA Scale-Free Network Model is apparently superior to that of Small-World Model. As a result of that,we would use the former to simulate the interpersonal network.According to the rules of BA network model, we should start from a network which has Mo nodes, then introduce a new node after each time interval and connect this node with m nodes. The prerequisite is m≤m0.During the connection process, the probability between a new node and an existing node i is ∏i, the node degree of i k i, and the node degree of j is k j. These three factors should satisfy the following equation.∏i=k i/∑k jj(3-6) Specifically, when the existing nodes have larger node degrees, it would be much more easier for the new ones to connect with the existing ones.After t steps, there would be a BA Scale-Free Network Model. The number of its nodes is expressed as N and the number of its sides is expressed as e:N=m0+t(3-7)e=m×t(3-8) The population of Sierra Leone now is 6.1 million and we would use this datum to produce its interpersonal network. For more details, please refer to Appendix 11.2.3.5.2.The Simulation of Virus TransmissionIn the transmission process, we assume the infection rate is β, the recovery rate is rRate, and the death rate is dRate. Based on the fitting results we previously get, we can simulate the virus transmission situation as time goes.Here comes the details.In the first place, there would be one patient who initiates the epidemic. Every single day, the virus would transmit to others among the main network and the probability of one-time propagation is β. Also, the patients would have rRate of recovery and dRate of death. Meanwhile, if the patient has been infected for 30 days, he or she would die anyway. The exposed would be in a latent period, during which they are not infectious and asymptomatic. In 2 to 21 days, these exposed ones would become infectious.n S、n E、n I、n R、n D represent the 5 different numbers of people in the SEIRD Model. t means time step (or a day),R represents the probability that those who have recovered patients would infect others. RandomE denotes the number of exposed patients who have reached the period of 2 to 21 days at the current moment.Here is the formula showing the changes in the numbers of those five types of people.n S t+1=n S t−n I t×n×β−n R t×n×β×R(3-9) n E t+1=n E t+n I t×n×β+n R t×n×β×R−RandomE(3-10) n I t+1=n I t+RandomE−n I t×rRate−n I t×dRate(3-11)n R t+1=n R t+n I t×rRate(3-12)n D t+1=n D t+n I t×dRate(3-13) After that, when the transmission has reached a certain scale (20 days after the transmission), the international organizations would adopt the measure of quarantine towards infective patients to avoid further contagion. As for the exposed patients, since they could not be quarantined immediately, so they have one day to infect others and in the next day, they would be quarantined at once.Finally, for those who have recovered, there is still a certain chance that they will propagate the disease within their networks.n Ed t on behalf of the moments lurk in reaching the last day with infectious but has not yet been isolated number. The process of five types of personnel number change:It represents the number of the exposed who have reached their last day of latency, begin to be contagious and have not yet been quarantined at the current moment. During this process, the formula exhibiting changes for these five categories of people could be listed as follows.n S t+1=n S t−n Ed t×n×β−n R t×n×β×R(3-14) n E t+1=n E t+n Ed t×n×β+n R t×n×β×R−RandomE(3-15)n I t+1=n I t+RandomE−n I t×rRate−n I t×dRate(3-16)n R t+1=n R t+n I t×rRate(3-17)n D t+1=n D t+n I t×dRate(3-18)3.6.Results and Result Analysis3.6.1. A Complex Network Simulation Results ModelPersonnel network is illustrated as Fig 3.8. Because of the population is too large so it is difficult to figure out. We use a red point to represent 10000 persons.Fig 3.8 Personnel relation network diagramThe probability distribution of nodes in a network of degrees is illustrated as Fig 3.9.The same node degrees set in 2-4, in it with degree of 4most.On behalf of each person every day in the network average fluid contact with 2-4 people.Fig 3.9 The probability of the node degree distribution map network3.6.2.The Spread of the Virus the Simulation ResultsThe number of every kind of the curveof the change over time in SEIRD model is illustrated in Fig 3.10. Due to the large population, the graph is local amplification. It is unable to find the number of susceptible people in the picture. For the rest of the curve, black represents the exposed, red represents the sufferer and pink for the removed.Fig 3.10 The number of SEIR model with the change of time4.Delivery Systems Model(DSM) Based on Local Optimization4.1.Model OverviewOptimized distributing is the most significant problem while building Delivery Systems, and it is a NP (nondeterministic polynomial) problem. In order to studied the problem, Li Zong-yong, Li Yue and Wang Zhi-xue organized an optimized distributing algorithm based on genetic algorithm in 2006[7]. In addition, Liu Hai-yan, Li Zong-ping, Ye Huai-zhen[8]discussed logistics distribution center allocation problem based on optimization method. With the help of current literature, we build a Delivery Systems Model (DSM) for drug and vaccine delivery, based on Local Optimization.In this topic, in order to establish the feasible delivery systems for WesternAfrica, we take Sierra Leone as an example. There is 14 Districts in Sierra Leone.In this model, we choose points based on Sierra Leone politics. Representative point in every District is selected. The points are located by longitude and latitude. We use Euclidean distance-based clustering analysis to process the data, so that the point set will be classified into three sub-set. Every sub-set is a part. Then, one point in every sub-set will be selected as a delivery center based on Analytic Hierarchy Process (AHP) and Principal Component Analysis (PCA). Besides, we will design the routes based on Prim algorithm, aiming at minimum the cost in every sub-set. In this way, we will build a delivery system.In addition, we will compare the results with Treatment Centers distribution which has been built, to analyze the model.4.2. Cluster Division Based on Cluster AnalysisThere are 14 districts in Sierra Leone. In every district, we choose the center position as a point. In this model, the first step is to cluster. Cluster Analysis is based on similarity. In this model, the similarity could be measured by geography distance. There is different method for cluster.Suppose there are n variable, and the objects are x and y1212(,,,),(,,,),n n x x x x y y y y ==By Euclidean distance, the distance can be calculated by (4-1)(,)d x y =(4-1)By Cosine Similarity distance, the distance can be calculated by (4-2)2(,)ni ix yd x y =∑(4-2)In this model, we use Euclidean distance. We cluster 14 districts into three Parts. First of all, we need to know about the distances between districts. The 14 Districts of Sierra Leone are located by longitude and latitude. Establish a right angle coordinate system. Set the longitude as the abscissa and latitude as ordinate. Set the Greenwich meridian and the Equator as 0 degree. The West and the South are regarded as negative while the East and the North are regarded as positive. In addition, the data related to the latitude and longitude would be converted to standard decimal form. For example, point (1330',830'W N ︒︒) is located as (-13.5, 8.5).According to World Health Organization (WHO )[9]statistics data, the basic data of Sierra Leone’ Districts are obtained, which is shown in Table 4.1 .。
RBM_guide_en
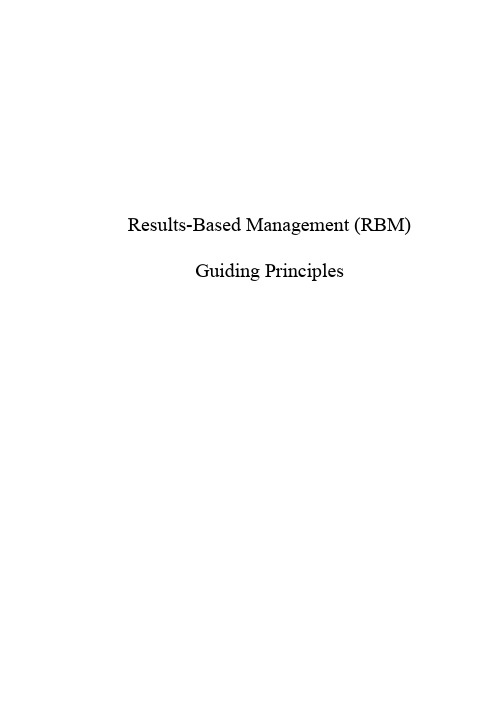
Results-Based Management (RBM) Guiding PrinciplesThis document is based on the UNESCO Results-Based Programming, Management and Monitoring (RBM) Guiding Principles, UNESCO Paris, Bureau of Strategic Planning, January 2008, and translated into Russian by the 3INDEX1. PREFACE (4)2. BRIEF HISTORICAL BACKGROUND (5)3. WHAT IS RBM? (6)4. WHAT IS A RESULT? (8)5. HOW TO FORMULATE AN EXPECTED RESULT? (8)6. WHAT IS THE RELATIONSHIP BETWEEN INTERVENTIONS,OUTPUTS AND RESULTS? (12)7. MONITORING OF IMPLEMENTATION (15)8. ANNEXES (23)This document is based on the UNESCO Results-Based Programming, Management and Monitoring (RBM) Guiding Principles, UNESCO Paris, Bureau of Strategic Planning, January 2008, and translated into Russian by the 41. PREFACEIt is said that if you do not know where you are going, any road will take you there. This lack of direction is what results-based management (RBM) is supposed to avoid. It is about choosing a direction and destination first, deciding on the route and intermediary stops required to get there, checking progress against a map and making course adjustments as required in order to realise the desired objectives.For many years, the international organizations community has been working to deliver services and activities and to achieve results in the most effective way. Traditionally, the emphasis was on managing inputs and activities and it has not always been possible to demonstrate these results in a credible way and to the full satisfaction of taxpayers, donors and other stakeholders. Their concerns are straightforward and legitimate: they want to know what use their resources are being put to and what difference these resources are making to the lives of people. In this line, RBM was especially highlighted in the “2005 Paris Declaration on Aid Effectiveness” as part of the efforts to work together in a participatory approach to strengthen country capacities and to promote accountability of all major stakeholders in the pursuit of results.It is usually argued that complex processes such as development are about social transformation, processes which are inherently uncertain, difficult, not totally controllable and - therefore - which one cannot be held responsible for. Nonetheless, these difficult questions require appropriate responses from the professional community and, in particular, from multilateral organizations to be able to report properly to stakeholders, and to learn from experience, identify good practices and understand what the areas for improvements are.The RBM system aims at responding to these concerns by setting out clear expected results expected for programme activities, by establishing performance indicators to monitor and assess progress towards achieving the expected results and by enhancing accountability of the organization as a whole and of persons in charge. It helps to answer the “so what” question, recognizing that we cannot assume that successful implementation of programmes is necessarily equivalent to actual improvements in the development situation.This paper is intended to assist in understanding and using the basic concepts and principles of results-based management.General information on RBM concept in this document is based on materials of some UN agencies. Bearing in mind that different RBM terminology is used by different actors due to each and everyone's specific context, it is important to ensure that the definitions are clear to all involved in the result based management within an organization. Inspite of different terminology used by different actors, the chain itself proceeds from activities to results/impacts.This document is based on the UNESCO Results-Based Programming, Management and Monitoring (RBM) Guiding Principles, UNESCO Paris, Bureau of Strategic Planning, January 2008, and translated into Russian by the 52. BRIEF HISTORICAL BACKGROUNDAs such, the concept of RBM is not really new. Its origins date back to the 1950’s. In his book “The practice of Management”, Peter Drucker introduced for the first time the concept of “Management by Objectives” (MBO) and its principles:-Cascading of organizational goals and objectives,-Specific objectives for each member of the Organization-Participative decision-making-Explicit time period-Performance evaluation and feedbackAs we will see further on, these principles are very much in line with the RBM approach.MBO was first adopted by the private sector and then evolved into the Logical Framework (Logframe) for the public sector. Originally developed by the United States Department of Defense, and adopted by the United States Agency for International Development (USAID) in the late 1960s, the logframe is an analytical tool used to plan, monitor, and evaluate projects. It derives its name from the logical linkages set out by the planners to connect a project’s means with its ends.During the 1990s, the public sector was undergoing extensive reforms in response to economic, social and political pressures. Public deficits, structural problems, growing competitiveness and globalization, a shrinking public confidence in government and growing demands for better and more responsive services as well as for more accountability were all contributing factors. In the process, the logical framework approach was gradually introduced in the public sector in many countries (mainly member States of the Organization for Economic Co-operation and Development (OECD). This morphed during the same decade in RBM as an aspect of the New Public Management, a label used to describe a management culture that emphasizes the centrality of the citizen or customer as well as the need for accountability for results.This was followed by the establishment of RBM in international organizations. Most of the United Nations system organizations were facing similar challenges and pressures from Member States to reform their management systems and to become more effective, transparent, accountable and results-oriented. A changeover to a results-based culture is however a lengthy and difficult process that calls for the introduction of new attitudes and practices as well as for sustainable capacity-building of staff.This document is based on the UNESCO Results-Based Programming, Management and Monitoring (RBM) Guiding Principles, UNESCO Paris, Bureau of Strategic Planning, January 2008, and translated into Russian by the 63. WHAT IS RBM?Results -based management (RBM) can mean different things to different people/organizations. A simple explanation is that RBM is a broad management strategy aimed at changing the way institutions operate, by improving performance, programmatic focus and delivery. It reflects the way an organization applies processes and resources to achieve interventions targeted at commonly agreed results.Results-based management is a participatory and team-based approach to programme planning and focuses on achieving defined and measurable results and impact. It is designed to improve programme delivery and strengthen management effectiveness, efficiency and accountability.RBM helps moving the focus of programming, managing and decision-making from inputs and processes to the objectives to be met. At the planning stage it ensures that there is a necessary and sufficient sum of the interventions to achieve an expected result.During the implementation stage RBM helps to ensure and monitor that all available financial and human resources continue to support the intended results.To maximize relevance, the RBM approach must be applied, without exceptions, to all organizational units and programmes. Each is expected to define anticipated results for its own work, which in an aggregative manner contributes to the achievement of the overall or high-level expected outcomes for the organization as a whole, irrespective of the scale, volume or complexity involved.RBM seeks to overcome what is commonly called the “activity trap”, i.e. getting so involved in the nitty-gritty of day-to-day activities that the ultimate purpose or objectives are being forgotten. This problem is pervasive in many organizations: project/programme managers frequently describe the expected results of their project/programme as “We provide policy advice to partners”, “We train journalists for the promotion of freedom of expression”, “We do research in the field of fresh water management”, etc., focusing more on the type of activities undertaken rather than on the ultimate changes that these activities are supposed to induce, e.g. in relation to a certain group of beneficiaries.An emphasis on results requires more than the adoption of new administrative and operational systems, it needs above all a performance-oriented management culture that supports and encourages the use of new management approaches. While from an institutional point of view, the primordial purpose of the RBM approach is to generate and use performance information for accountability reporting to external stakeholders and for decision-making, the first beneficiaries are the managers themselves. They will have much more control over the activities they are responsible for, be in a better position to take well-informed decisions, be able to learn from their successes or failures and to share this experience with their colleagues and all other stakeholders. The processes or phases of RBMThe formulation of expected results is part of an iterative process along with the definition of a strategy for a particular challenge or task. The two concepts – strategy and expected results - areThis document is based on the UNESCO Results-Based Programming, Management and Monitoring (RBM) Guiding Principles, UNESCO Paris, Bureau of Strategic Planning, January 2008, and translated into Russian by the 7closely linked, and both have to be adjusted throughout a programming process so as to obtain the best possible solution.In general, organizational RBM practices can be cast in twelve processes or phases, of which the first seven relate to results-oriented planning.1)Analyzing the problems to be addressed and determining their causes and effects;2)Identifying key stakeholders and beneficiaries, involving them in identifying objectivesand in designing interventions that meet their needs;3)Formulating expected results, in clear, measurable terms;4)Identifying performance indicators for each expected result, specifying exactly what is tobe measured along a scale or dimension;5)Setting targets and benchmarks for each indicator, specifying the expected or plannedlevels of result to be achieved by specific dates;6)Developing a strategy by providing the conceptual framework for how expected resultsshall be realized, identifying main modalities of action reflective of constraints andopportunities and related implementation schedule;7)Balancing expected results and the strategy foreseen with the resources available;8) Managing and monitoring progress towards results with appropriate performancemonitoring systems drawing on data of actual results achieved;9)Reporting and self-evaluating, comparing actual results vis-à-vis the targets and reportingon results achieved, the resources involved and eventual discrepancies between the“expected” and the “achieved” results;10)Integrating lessons learned and findings of self-evaluations, interpreting the informationcoming from the monitoring systems and finding possible explanations to eventualdiscrepancies between the “expected” and the “achieved”.11) Disseminating and discussing results and lessons learned in a transparent and iterativeway.12) Using performance information coming from performance monitoring and evaluationsources for internal management learning and decision-making as well as for externalreporting to stakeholders on results achieved.This document is based on the UNESCO Results-Based Programming, Management and Monitoring (RBM) Guiding Principles, UNESCO Paris, Bureau of Strategic Planning, January 2008, and translated into Russian by the 84. WHAT IS A RESULT?A result is the “raison d’être” of an intervention. A result can be defined as a describable and measurable change in state due to a cause and effect relationship induced by that intervention. Expected results are answers to problems identified and focus on changes that an intervention is expected to bring about. A result is achieved when the outputs produced further the purpose of the intervention.It often relates to the use of outputs by intended beneficiaries and is therefore usually not under full control of an implementation team.5. HOW TO FORMULATE AN EXPECTED RESULT?Formulate the expected results from the beneficiaries’ perspectiveFormulating expected results from the beneficiaries’ perspective will facilitate focusing on the changes expected rather than on what is planned to be done or the outputs to be produced. This is particularly important at the country level, where UNESCO seeks to respond to the national development priorities of a country. Participation is key for improving the quality, effectiveness and sustainability of interventions. When defining an intervention and related expected results one should therefore ask:Who participated in the definition of the expected results?Were key project stakeholders and beneficiaries involved in defining the scope of the project and key intervention strategies?Is there ownership and commitment from project stakeholders to work together to achieve identified expected results?This document is based on the UNESCO Results-Based Programming, Management and Monitoring (RBM)Guiding Principles, UNESCO Paris, Bureau of Strategic Planning, January 2008, and translated into Russian by the 9Use “change” language instead of “action” languageThe expected result statement should express a concrete, visible, measurable change in state or a situation. It should focus on what is to be different rather than what is to be done and should express it as concretely as possible. Completed activities are not results, results are the actual benefits or effects of completed activities.Action language Change language… expresses results from the provider’s perspective:• To promote literacy by providing schools and teaching material. … can often be interpreted in many ways: • To promote the use of computers. … focuses on completion of activities:• To train teachers in participatory teaching.…describes changes in the conditions of beneficiaries:• Young children have access to schoolfacilities and learn to read and write.... sets precise criteria for success:• People in undersupplied areas haveincreased knowledge of how to benefit from the use of a computer and haveaccess to a computer.... focuses on results, leaving options on how to achieve them (how this will be achievedwill be clarified in the activity description): • Teachers know how to teach in a participatory way and use thesetechniques in their daily workMake sure your expected results are SMARTAlthough the nature, scope and form of expected results differ considerably, an expected result should meet the following criteria (be “SMART”):Specific: It has to be exact, distinct and clearly stated. Vague language or generalities are notresults. It should identify the nature of expected changes, the target, the region, etc. It should be as detailed as possible without being wordy.Measurable: It has to be measurable in some way, involving qualitative and/or quantitativecharacteristics.Achievable: It has to be achievable with the human and financial resources available(‘realistic’).Relevant: It has to respond to specific and recognized needs or challenges and to be withinmandate.Time-bound: It has to be achieved in a stated time-frame or planning period.This document is based on the UNESCO Results-Based Programming, Management and Monitoring (RBM) Guiding Principles, UNESCO Paris, Bureau of Strategic Planning, January 2008, and translated into Russian by the 10Once a draft expected results statement has been formulated, it is useful to test its formulation going through the SMART criteria. This process enhances the understanding of what is pursued, and is of help in refining an expected result in terms of their achievability and meaningfulness.Example: if we consider a work plan to be undertaken in a specific country that includes the expected results statement “Quality of primary education improved”, the application of the SMART questioning could be as follows:1. Is it “Specific”?What does “quality” actually mean in this context? What does an “improvement” of quality in primary education amount to concretely? Who are the relevant stakeholders involved? Are we working on a global level, or are we focusing on a particular region or country?In responding to the need of being specific, a possible expected result formulation could finally be:“Competent authorities in Country X adopted the new education plan reviewed on the basis of international best practices and teachers and school personnel implement it.”2.Is it “Measurable”?Can I find manageable performance indicators that can tell about the level of achievement?Possible Performance Indicators could be:- % of teachers following the curriculum developed on the basis of the new education plan (baseline 0%, benchmark 60%)- % of schools using quality teaching material (baseline 10%, benchmark 90%)3.Is it “Achievable”?Do I have enough resources available to attain the expected result? I need to consider both financial and human resources. If the answer is negative, I have to either reconsider and adjust the scope of the project or mobilize additional resources.4.Is it “Relevant”?Is the expected result coherent with the upstream programming element based on. of its domains?If the answer is negative I should drop the activity.5.Is it “Time-bound”?The expected result should be achievable within the given timeframe for programming processes this period.This document is based on the UNESCO Results-Based Programming, Management and Monitoring (RBM) Guiding Principles, UNESCO Paris, Bureau of Strategic Planning, January 2008, and translated into Russian by the 11Improving the results formulation: the SMART processFind a proper balance among the three RsOnce an intervention is formulated, it can be useful to check and improve its design against yet another concept – namely, establishing a balance between three variables Results (describable and measurable change in state that is derived from a cause and effect relationship), Reach (the breadth and depth of influence over which the intervention aims at spreading its resources) and Resources (human, organisational, intellectual and physical/material inputs that are directly or indirectly invested in the intervention).Unrealistic project plans often suffer from a mismatch among these three key variables. It is generally useful to check the design of a project by verifying the three Rs by moving back and forth along the project structure and by ensuring that the logical links between the resources, results and the reach are respected.It is rather difficult to construct a results-based design in one sitting. Designs usually come together progressively and assumptions and risks have to be checked carefully and constantly along the way.This document is based on the UNESCO Results-Based Programming, Management and Monitoring (RBM) Guiding Principles, UNESCO Paris, Bureau of Strategic Planning, January 2008, and translated into Russian by the 126. WHAT IS THE RELATIONSHIP BETWEEN INTERVENTIONS,OUTPUTS AND RESULTS?Interventions, outputs and results are often confused. Interventions describe what we do in order to produce the changes expected. The completion of interventions leads to the production of outputs. Results are finally the effects of outputs on a group of beneficiaries. For example, the implementation of training workshops (activity) will lead to trainees with new skills or abilities (outputs). The expected result identifies the behavioral change among the people that were trained leading to an improvement in the performance of, say, an institution the trainees are working in, which is the ultimate purpose of the activity.If we move our focus from what we do to what we want the beneficiaries to do after they have been reached by our intervention, we may realize that additional types of activities could be necessary to make sure we will be able to achieve the expected results.It is important that a project is driven by results and not activities.Defining expected results:is not an exact science;includes a solid understanding of the socio-economic, political and cultural context;is influenced by available resources, the degree of beneficiary reached and potential risk factors;requires participation of key stakeholders.The following examples may help to understand the relationship between interventions, outputs and results, but should not be seen as a generally applicable master copy as every intervention is different from another.TITLE OF THEACTIVITYINTERVENTIONS OUTPUTS RESULTSCapacity building for sustainable development in the field of watermanagement • Compilation of bestpractices in the field ofwater informationmanagement.• Develop Internet-based informationsystems/web pages anddatabases and othertools (software,guidelines, databases)to transfer and sharewater information.• Organization ofWater InformationSummits.• Best practices formanagement of waterinformation collectedand disseminated.• Software, guidelines,database, etc. availableto the institutionsconcerned.• Water InformationSummits attended bypolicy makers andrepresentatives ofinstitutions concerned.• Relevant institutionsassisted for the• Relevant institutionshave adapted andimplemented bestpractices for waterinformationmanagement.This document is based on the UNESCO Results-Based Programming, Management and Monitoring (RBM) Guiding Principles, UNESCO Paris, Bureau of Strategic Planning, January 2008, and translated into Russian by the 13• Provision of technical assistance. implantation of best practices.Contribution to a reconstruction programme for the education system in country X • Assessing thesituation with regard tothe national educationsystem• Organization ofworkshops fordecision-makers andexperts to discuss andselect a new educationplan.• Definition of aneducation planensuring an adequatelearning environmentin country X.• Provision oftechnical assistance• Organization oftraining workshops forteachers and schoolpersonnel• Development ofteaching and learningmaterial.• Situation analysisreportcompleted.• Workshops attendedby relevant decision-makers and experts.• Education plandeveloped andavailable to localcounterparts.• Teachers and schoolpersonnel trained.• Implementationcapacities of localcounterparts improved.• Teaching andlearning materialsdelivered.• Relevant authoritieshave adopted the neweducation plan andteachers and schoolpersonnel implement it.UNESCO Prize for Tolerance • Selection andinformation of jury.• Preparation ofbrochures, informationmaterial.• Development andorganization of anInformation campaign.• Advertising the prize.• Development ofpartnerships for theidentification of a listof candidates.• Organization of theaward ceremony.• Organization of pressconferences.• Follow-up andassessment of mediacoverage.• Jury nominated andagreeable to mainstakeholders.• Brochures, leaflets,videos produced anddisseminated.• Informationcampaignimplemented• List of candidatescompleted andagreeable to mainstakeholders.• Prize-winnernominated.• Press Conferenceorganized and attendedby identifiedjournalists.• Media coverage of• Concept of tolerancespread among thegeneral public in acountry/region/globally.This document is based on the UNESCO Results-Based Programming, Management and Monitoring (RBM) Guiding Principles, UNESCO Paris, Bureau of Strategic Planning, January 2008, and translated into Russian by the 14theevent.Promotion of the UNESCO Universal Declaration of Cultural Diversity • Develop guidelineson the application ofthe Declaration indifferent fields.• Inform about theDeclaration.• Organization ofworkshops on theDeclaration for policy-makers and keydecision-makers.• Guidelines on theapplication of theDeclaration indifferent fieldsproduced.• Workshops on theDeclaration organizedand guidelines on theDeclaration distributedto persons attendingthe workshops.• Decision-makers putto practice theguidelines on theapplication of theDeclaration in differentfields.Increasing access toquality basic education for children through community learning centres • Discussions withlocal authorities.• Assessing thefeasibility ofcommunity-basedlearning centre incommunity X.• Preliminarydiscussions with localstakeholders.• Sensitizationseminars for localleaders and communitymembers.• Curriculumdevelopment ofcommunity-basedlearning centres.• Selection of trainingpersonnel among thelocal community.• Adapting trainingmaterial• Training ofpersonnel.• Production ofinformation materialfor local authorities.• Meetings with localauthorities for thereplication of suchcentres.• Provision of• Principle agreementby local authorities.• Feasibility studycompleted and genderanalysis produced anddisseminated.• Principle agreementby community leaders.• Community Centreproposal completedand submitted to localauthorities andcommunity leaders.• Personnel selected.• Curriculum andtraining material forcommunity-basedlearning centresdeveloped.• Managers andteachers have thenecessary skills toimplement theirfunctions.• Brochures & videosdeveloped anddisseminated.• Local leaders andcommunity membersinformed, sensitizedand convinced.• The centre isoperational and is anintegral part of thecommunity life.• Steps are taken bylocal authorities forreplicating thisinitiative in othercommunities.This document is based on the UNESCO Results-Based Programming, Management and Monitoring (RBM) Guiding Principles, UNESCO Paris, Bureau of Strategic Planning, January 2008, and translated into Russian by the 15technical assistance tolocal authorities forreplicating suchcentres in othercommunities.We can anticipate a few challenges in this process:-The nature of expected results: it is obvious that the nature, magnitude, meaning of “expected results” cannot be the same among the different levels. Nevertheless, it iscrucial that all these results build a chain of meaningful achievements, bridging thegap between the mandate and the strategic objectives of the organisation actuallyachieves in its daily operations.-Reconciling global and local dimensions: RBM stresses results and greater focus; this should be done without sacrificing the organisation’s global mandate and itscommitment to decentralisation and responsiveness to country needs and priorities: agood balance has to be found between global and field-oriented approaches.7. MONITORING OF IMPLEMENTATIONMonitoring can be described as “a continuing function that uses systematic collection of data on specified indicators to provide management and the main stakeholders of an ongoing (…) intervention with indications of the extent of progress and achievement of objectives and progress in the use of allocated funds” (Source: OECD RBM Glossary).The function of a monitoring system is to compare “the planned” with “the actual”. A complete monitoring system needs to provide information about the use of resources, the activities implemented, the outputs produced and the results achieved. What we are focusing on here is a results-based monitoring system: at the planning stage, through its monitoring system, the officer in charge has to translate the objectives of the intervention in expected results and related performance indicators and to set the baseline and targets for each of them. During implementation, he needs to routinely collect data on these indicators, to compare actual levels of performance indicators with targets, to report progress and take corrective measures whenever required.As a general rule, no extra resources (neither human, nor financial) will be allowed for monitoring tasks, hence the responsible person has to ensure that these tasks can be undertaken with the budget foreseen (as a general rule of thumb, about 5% of the resources should be set aside for this purpose). Selection and formulation of performance indicatorsMonitoring is done through the use of appropriate performance indicators. When conceiving an intervention, the responsible officer is also required to determine appropriate performance indicators。
插电式混合动力汽车控制策略与建模

106机械设计与制造Machinery Design & Manufacture第3期2021年3月插电式混合动力汽车控制策略与建模宫唤春(燕京理工学院,北京065201)摘要:为了深入分析插电式混合动力汽车能量管理控制策略就需要建立准确的插电式混合动力汽车仿真测试模型,分析影响能量管理系统的因素。
利用M A T L A B/S I M U L I N K软件基于实验数据和理论模型相结合的方法对插电式混合动力汽车建模,根据插电式混合动力汽车传动系部件的工作特征对应建立各部件的数学模型,并建立了基于规则的能量管理控制策略对整车的动力性与经济性进行计算仿真验证,计算结果表明建立的插电式混合动力汽车仿真糢型和能量管理控制策略能够有效确保发动机处于高效区域运行并改善整车燃油经济性,控制策略可靠有效。
关键词:插电式混合动力汽车;建模;能量管理;控制策略中图分类号:T H16文献标识码:A文章编号:1001-3997(2021)03-0106-04Control Strategy and Modeling of Plug-in Hybrid Electric VehiclesGONG Huan-chun(Yanching Institute of Technology, Beijing 065201, China)Abstract :/n order to deeply analyze the energy management control strategy o f plug-in hybrid vehicles, it is necessary to establish an accurate plug-in hybrid vehicle simulation test model and analyze the factors affecting the energy management systerruThe M A T L A B/S I M U L I N K software is used to model the p lu g—in hybrid vehicle based on the combination of experimented data and theoretical model. The mathematical model o f each component is established according to the working characteristics o f the powertrain o f the p lu g-in hybrid vehicle y and the basis is established. The energy management and control strategy o f the rule calculates and verifies the power and economy o f the vehicle. The calculation results show that the plug—in hybrid vehicle simulation model and energy management control strategy established in this paper can effectively ensure that the engine is running in an efficient area and improve the whole. Vehicle fu el economy, control strategy is reliableand effective.Key Words:Plug-in Hybrid Vehicle; Modeling; Energy Management; Control Strategyl引言插电式混合动力汽车(Plug-in Hybrid Electric Vehicle, P H E V)是基于传统混合动力汽车衍生出的一种车辆,该类型汽车可以直 接接人电网进行充电,纯电动模式下续驶里程更远,同时统发动 机更省油等优点,已经成为电动汽车领域重点研发的产品之一插电式混合动力汽车对动力传动系统的设计及能量管理系统控制等要求较高从而使得其工作模式与传动混合动力汽车相比更为复杂。
Thermal Science and Engineering
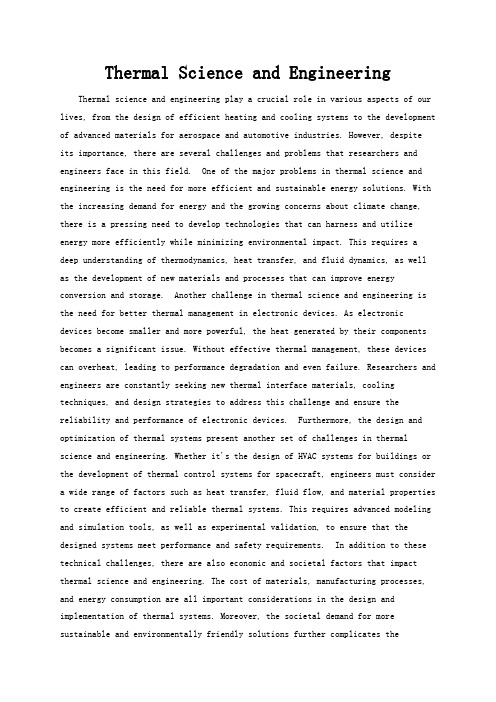
Thermal Science and Engineering Thermal science and engineering play a crucial role in various aspects of our lives, from the design of efficient heating and cooling systems to the development of advanced materials for aerospace and automotive industries. However, despiteits importance, there are several challenges and problems that researchers and engineers face in this field. One of the major problems in thermal science and engineering is the need for more efficient and sustainable energy solutions. With the increasing demand for energy and the growing concerns about climate change, there is a pressing need to develop technologies that can harness and utilize energy more efficiently while minimizing environmental impact. This requires a deep understanding of thermodynamics, heat transfer, and fluid dynamics, as well as the development of new materials and processes that can improve energy conversion and storage. Another challenge in thermal science and engineering is the need for better thermal management in electronic devices. As electronic devices become smaller and more powerful, the heat generated by their components becomes a significant issue. Without effective thermal management, these devices can overheat, leading to performance degradation and even failure. Researchers and engineers are constantly seeking new thermal interface materials, cooling techniques, and design strategies to address this challenge and ensure the reliability and performance of electronic devices. Furthermore, the design and optimization of thermal systems present another set of challenges in thermal science and engineering. Whether it's the design of HVAC systems for buildings or the development of thermal control systems for spacecraft, engineers must consider a wide range of factors such as heat transfer, fluid flow, and material properties to create efficient and reliable thermal systems. This requires advanced modeling and simulation tools, as well as experimental validation, to ensure that the designed systems meet performance and safety requirements. In addition to these technical challenges, there are also economic and societal factors that impact thermal science and engineering. The cost of materials, manufacturing processes, and energy consumption are all important considerations in the design and implementation of thermal systems. Moreover, the societal demand for more sustainable and environmentally friendly solutions further complicates thedevelopment of thermal technologies, as engineers must balance performance, cost, and environmental impact. Despite these challenges, there are also many opportunities and exciting developments in thermal science and engineering. The emergence of additive manufacturing and advanced materials, for example, has the potential to revolutionize the design and performance of thermal systems. Similarly, the integration of renewable energy sources and the development of smart grid technologies offer new possibilities for more efficient and sustainable energy utilization. In conclusion, thermal science and engineering present a wide range of challenges, from the need for more efficient energy solutions to the design and optimization of thermal systems. However, with continued research and innovation, there are also many opportunities to address these challenges and develop new technologies that can improve our lives and our environment. By addressing these challenges, engineers and researchers can contribute to a more sustainable and energy-efficient future.。
System Modeling and Simulation
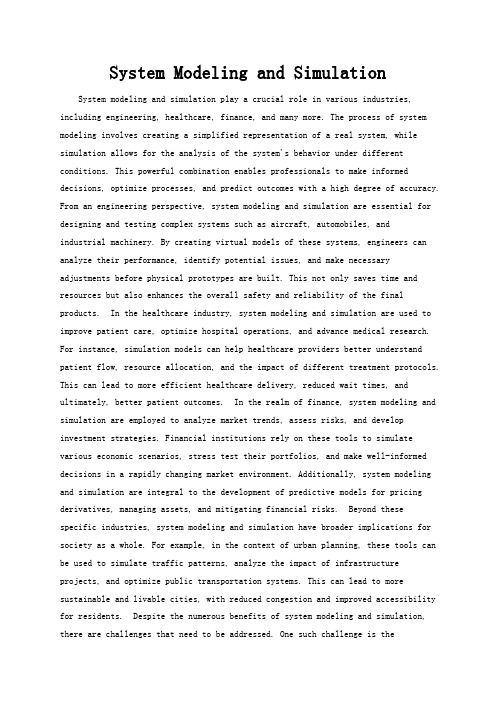
System Modeling and Simulation System modeling and simulation play a crucial role in various industries, including engineering, healthcare, finance, and many more. The process of system modeling involves creating a simplified representation of a real system, while simulation allows for the analysis of the system's behavior under different conditions. This powerful combination enables professionals to make informed decisions, optimize processes, and predict outcomes with a high degree of accuracy. From an engineering perspective, system modeling and simulation are essential for designing and testing complex systems such as aircraft, automobiles, andindustrial machinery. By creating virtual models of these systems, engineers can analyze their performance, identify potential issues, and make necessary adjustments before physical prototypes are built. This not only saves time and resources but also enhances the overall safety and reliability of the final products. In the healthcare industry, system modeling and simulation are used to improve patient care, optimize hospital operations, and advance medical research. For instance, simulation models can help healthcare providers better understand patient flow, resource allocation, and the impact of different treatment protocols. This can lead to more efficient healthcare delivery, reduced wait times, and ultimately, better patient outcomes. In the realm of finance, system modeling and simulation are employed to analyze market trends, assess risks, and develop investment strategies. Financial institutions rely on these tools to simulate various economic scenarios, stress test their portfolios, and make well-informed decisions in a rapidly changing market environment. Additionally, system modeling and simulation are integral to the development of predictive models for pricing derivatives, managing assets, and mitigating financial risks. Beyond thesespecific industries, system modeling and simulation have broader implications for society as a whole. For example, in the context of urban planning, these tools can be used to simulate traffic patterns, analyze the impact of infrastructureprojects, and optimize public transportation systems. This can lead to more sustainable and livable cities, with reduced congestion and improved accessibility for residents. Despite the numerous benefits of system modeling and simulation, there are challenges that need to be addressed. One such challenge is thecomplexity of creating accurate models that capture all relevant aspects of a system. This requires a deep understanding of the system's behavior, as well as the availability of reliable data for validation and calibration. Additionally, the computational resources required for running simulations of large-scale systems can be substantial, necessitating efficient algorithms and high-performance computing infrastructure. Furthermore, the interpretation of simulation results and the translation of findings into actionable insights can be a daunting task. It requires interdisciplinary collaboration between domain experts, data scientists, and simulation specialists to ensure that the outcomes are meaningful and applicable in real-world scenarios. Moreover, there is a need for continuous refinement and validation of simulation models to keep them relevant and accurate in dynamic environments. From a human perspective, the use of system modeling and simulation can evoke a sense of empowerment and confidence in decision-making. Professionals who leverage these tools are better equipped to anticipate challenges, explore innovative solutions, and make evidence-based choices. This can lead to a greater sense of control over complex systems and a reduced fear of the unknown, ultimately fostering a culture of continuous improvement and resilience. In conclusion, system modeling and simulation are indispensable tools that have far-reaching implications across various industries and societal domains. While they offer tremendous potential for innovation and progress, it is essential to acknowledge the challenges associated with their application and to work towards overcoming them through collaboration, innovation, and a commitment to excellence. As technology continues to advance, the future of system modeling and simulation holds great promise for shaping a more efficient, sustainable, and prosperous world.。
MODELLING IN THE PRODUCT/PROCESS LIFECYCLE
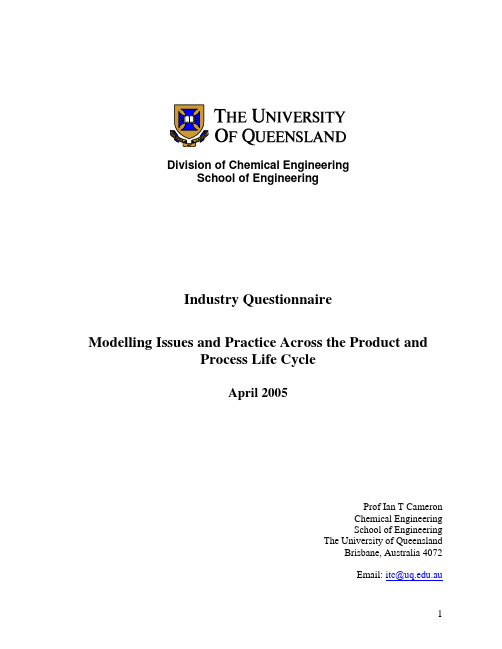
Division of Chemical EngineeringSchool of EngineeringIndustry QuestionnaireModelling Issues and Practice Across the Product andProcess Life CycleApril 2005Prof Ian T CameronChemical EngineeringSchool of EngineeringThe University of QueenslandBrisbane, Australia 4072Email: itc@.auMODELLING ACROSS THE PRODUCT/PROCESS LIFECYCLEA Questionnaire on Issues and PracticePurpose: This questionnaire is gathering industry data on current modelling practice and key issues faced by corporations that use a range of modelling and simulation systems as decision making tools.Use of data: The data will be collated and analyzed. The consolidated responses will be sent back to all respondents in a way that will not identify individuals. The general results will be presented in the open literature as a means of informing the widest possible audience regarding the challenges for future developments in modelling methodologies, tools and practices.Anonymity: All responses are treated anonymously.Instructions: Please mark your responses with a cross in the appropriate location. There are 3 Parts and a total of 25 questions taking about 20 to 30 minutes of your time.Please send the completed questionnaire by email to Ian Cameron, The University of Queensland at: itc@.au before April 30, 2005.I appreciate you taking the time to respond to this questionnaire!Part 1: GENERAL BUSINESS ENVIRONMENT1.What are your industry or business sectors?2.What role does your work group play in the overall corporation?3.What is the general educational background of the group?4.What is the average modelling experience within the group?5.How many people in your group are primarily engaged in modelling?There are ……. people out of a total number of …… people involved in modelling. Part 2: GENERAL MODELLING ISSUESFor the purposes of this questionnaire, modelling is defined as the activity of developing or using mathematical forms to describe the behaviour of a system and then using that model for simulation or other purposes.1.How much of the group’s time is spent in doing modelling work?2.Which of the following life cycle phases do you address in your modelling work?3.For the particular life cycle phases you address, what types of modelling do youundertake?ment on the way modelling and simulation takes place.5.If you collaborate, then what are the main mechanisms supporting collaboration?ment on the reasons for undertaking modelling and simulation in the lifecycle phases you work on.7.What are the principal tools you use for modelling and simulation?8.Who are the “customers” of your modelling and simulation activities?9.How are the modell ing outcomes communicated to the “customer”?ment on the quality and communication of the modelling outcomes11.What if anything restricts the use of modelling in your organization?Part 3: SPECIFIC MODELLING ISSUES AND PRACTICEment on basic modelling practice.ment on the conceptualization phase of modelling.ment on the documentation aspects of the modelling or simulation youundertake.ment on the informational aspects of modelling or simulation.ment on the validation practices for modelling and simulation.ment on the model building and solution phase.ment on the quality of the modelling outcomes.ment on the modelling experience.ment on the value and risks associated with modellingAny further written comments you might like to make:11。
加强炼镁传热效率的研究进展
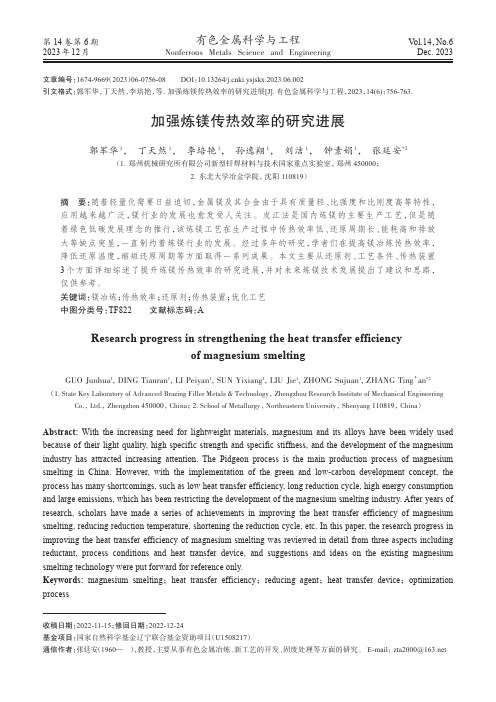
第14卷第6期2023年12月有色金属科学与工程Nonferrous Metals Science and EngineeringVol.14,No.6Dec. 2023加强炼镁传热效率的研究进展郭军华1, 丁天然1, 李培艳1, 孙逸翔1, 刘洁1, 钟素娟1, 张廷安*2(1.郑州机械研究所有限公司新型钎焊材料与技术国家重点实验室, 郑州 450000;2.东北大学冶金学院, 沈阳 110819)摘要:随着轻量化需要日益迫切,金属镁及其合金由于具有质量轻、比强度和比刚度高等特性,应用越来越广泛,镁行业的发展也愈发受人关注。
皮江法是国内炼镁的主要生产工艺,但是随着绿色低碳发展理念的推行,该炼镁工艺在生产过程中传热效率低、还原周期长、能耗高和排放大等缺点突显,一直制约着炼镁行业的发展。
经过多年的研究,学者们在提高镁冶炼传热效率,降低还原温度,缩短还原周期等方面取得一系列成果。
本文主要从还原剂、工艺条件、传热装置3个方面详细综述了提升炼镁传热效率的研究进展,并对未来炼镁技术发展提出了建议和思路,仅供参考。
关键词:镁冶炼;传热效率;还原剂;传热装置;优化工艺中图分类号:TF822 文献标志码:AResearch progress in strengthening the heat transfer efficiencyof magnesium smeltingGUO Junhua 1, DING Tianran 1, LI Peiyan 1, SUN Yixiang 1, LIU Jie 1, ZHONG Sujuan 1, ZHANG Ting ’an *2(1. State Key Laboratory of Advanced Brazing Filler Metals & Technology , Zhengzhou Research Institute of Mechanical EngineeringCo., Ltd., Zhengzhou 450000, China ; 2. School of Metallurgy , Northeastern University , Shenyang 110819, China )Abstract: With the increasing need for lightweight materials, magnesium and its alloys have been widely used because of their light quality, high specific strength and specific stiffness, and the development of the magnesium industry has attracted increasing attention. The Pidgeon process is the main production process of magnesium smelting in China. However, with the implementation of the green and low-carbon development concept, the process has many shortcomings, such as low heat transfer efficiency, long reduction cycle, high energy consumption and large emissions, which has been restricting the development of the magnesium smelting industry. After years of research, scholars have made a series of achievements in improving the heat transfer efficiency of magnesium smelting, reducing reduction temperature, shortening the reduction cycle, etc. In this paper, the research progress in improving the heat transfer efficiency of magnesium smelting was reviewed in detail from three aspects including reductant, process conditions and heat transfer device, and suggestions and ideas on the existing magnesium smelting technology were put forward for reference only.Keywords: magnesium smelting ; heat transfer efficiency ; reducing agent ; heat transfer device ; optimization process收稿日期:2022-11-15;修回日期:2022-12-24基金项目:国家自然科学基金辽宁联合基金资助项目(U1508217)通信作者:张廷安(1960— ),教授,主要从事有色金属冶炼、新工艺的开发、固废处理等方面的研究。
- 1、下载文档前请自行甄别文档内容的完整性,平台不提供额外的编辑、内容补充、找答案等附加服务。
- 2、"仅部分预览"的文档,不可在线预览部分如存在完整性等问题,可反馈申请退款(可完整预览的文档不适用该条件!)。
- 3、如文档侵犯您的权益,请联系客服反馈,我们会尽快为您处理(人工客服工作时间:9:00-18:30)。
Measuring and Modelling the Group Membership in theInternetJun-Hong Cui1,Michalis Faloutsos3,Dario Maggiorini4,Mario Gerla2,Khaled Boussetta2 Emails:jcui@,michalis@,dario@dico.unimi.it,gerla@,boukha@ 1Computer Science&Engineering Department,University of Connecticut,Storrs,CT060292Computer Science Department,University of California,Los Angeles,CA900953Computer Science&Engineering,University of California,Riverside,CA925214Computer Science Department,University of Milan,via Comelico39,I-20135,Milano,ItalyABSTRACTIn this paper,we measure and model the distribution of multicast group members.Multicast research has traditionally been plagued by a lack of real data and an absence of a systematic simulation methodology.Although temporal group properties have received some attention,the location of group members has not been mea-sured and modelled.However,the placement of members can have significant impact on the design and evaluation of multicast schemes and protocols as shown in previous studies.In our work,we iden-tify properties of members that reflect their spatial clustering and the correlation among them(such as participation probability,and pairwise correlation).Then,we obtain values for these properties by monitoring the membership of network games and large audio-video broadcasts from IETF and NASA.Finally,we provide a com-prehensive model that can generate realistic groups.We evaluate our model against the measured data with excellent results.A re-alistic group membership model can help us improve the effective-ness of simulations and guide the design of group-communication protocols.Categories and Subject DescriptorsC.2.2[Computer-Communication Networks]:Network Protocols—ApplicationsGeneral TermsAlgorithms,Measurement,Performance,ExperimentationKeywordsGroup Membership,Member Clustering,Skewed Distribution,Pair-wise Correlation,Maximum Entropy1.INTRODUCTIONWhere should the members be located in a multicast simulation? This is the question that lies in the heart of this work.Multicast Permission to make digital or hard copies of all or part of this work for personal or classroom use is granted without fee provided that copies are not made or distributed for profit or commercial advantage and that copies bear this notice and the full citation on thefirst page.To copy otherwise,to republish,to post on servers or to redistribute to lists,requires prior specific permission and/or a fee.IMC’03,October27–29,2003,Miami Beach,Florida,USA.Copyright2003ACM1-58113-773-7/03/0010...$5.00.research can greatly benefit from realistic models and a systematic evaluation methodology([7][25][10][21][8][23][22][26][30] [14]).Despite the significant breakthroughs in modelling the traf-fic and the topology of the Internet,there has been little progress in multicast modelling.As a result,the design and evaluation of multicast protocols is based on commonly accepted but often un-proven assumptions.For example,the majority of simulation stud-ies assumes that the users are uniformly distributed in the network. In this paper,we challenge this assumption and study the spatial properties of group members,such as clustering and correlation.A realistic and systematic membership model can have signifi-cant impact on the design and development of multicast protocols. Spatial information can help us address the scalability issues,which has always been a major concern in multicasting.Similarly,reli-able multicast protocols need spatial information in order tofine-tune their performance or even evaluate their viability.Further-more,spatial properties of a group with common interest members transcend the scope of IP multicast.Group communications is an undeniable necessity independently of the specifics of the technol-ogy that is used to support it.For example,web caching or applica-tion level multicast protocols can and should consider the member locality.Only recently,properties of group membership have received some attention,but the spatial properties have not been adequately measured and modelled.Several studies show the importance of the spatial distribution of members[30][14][21].However,there does not exist a generative model for such a distribution,which is partly due to unavailability of real data.In more detail,there have been several studies on the temporal group properties[4][15].In addition,several studies examine the scaling properties of multi-cast trees[10][21][8][9]and the aggregatability of multicast state [22][26][30][14].Philips et al.[21]conclude that the affinity and disaffinity of members can affect the size of the multicast tree significantly.Thaler et al.[26]and Fei et al.[14]observe that the location of members has significant influence on the performance of their state reduction schemes.In this paper,we study the distribution of group members focus-ing on their clustering and correlation.A distinguishing point of our work is that we use extensive measurements to understand the real distributions and develop a powerful model to generate realis-tic distributions.Our contributions can be grouped into two main thrusts.I.Real data analysis.We measure and analyze the member-ship of net games and large audio-video broadcasts from IETF and NASA(over the MBONE).We quantify properties of the mem-bership focusing on:a)the clustering,b)the distribution of theparticipation,and c)the distribution of the pairwise correlation of members or clusters in a group.We observe that the MBONE mul-ticast and gaming groups exhibit differences,which suggests the need for aflexible model to capture both.In our clustering analy-sis,we use the seminal approach of network-aware clustering[16]. More specifically,we make the following observations.1.MBONE multicast members:The group members are highlyclustered and the clusters exhibit strong pairwise correlations in their participation. game members:The clustering is much less pronouncedand there does not seem to be a strong correlation between users.Interestingly,we observe a very strong daily periodic-ity.II.GEM:A model for generating realistic groups.We de-velop GE neralized M embership model(GEM)that can generate realistic member distributions.These distributions are given as in-put parameters to the model,enabling users to match the desired distribution.The main innovation of the model is the capability to match pairwise participation probabilities.To achieve this,we use the Maximum Entropy method[31],which,in an under-defined system,chooses the solution with maximum“randomness”or en-tropy.As a result,GEM can simulate the following membership behavior:1.Uniform distribution,which is the typical but not always re-alistic distribution.2.Skewed participation distribution without pairwise correla-tions.3.Skewed participation distribution with pairwise correlations. We validate our model with very positive results.We are able to generate groups whose statistical behavior matches very well the real distributions.Modelling location of users with common interests.The analy-sis and the framework presented here can be of interest even out-side the multicast community.Applications with multiple recipi-ents such as web caching and streaming multimedia are also inter-ested in the location of users([6][29]).We provide our data and our model to the community with the hope that it can be part of a realistic and systematic evaluation methodology for this kind of research([1]).The rest of this paper is organized as follows.Section2gives some background on multicast group modelling.Section3lists the spatial properties of group members.Section4quantifies the spatial group properties using real data from the MBONE and net games.Section5describes our powerful group membership model. In Section6,we validate the capabilities of our model.Finally,we conclude our work in section7.2.BACKGROUNDIn this section,we give some background on multicast group modelling and related efforts.The properties of multicast group be-havior can be classified into two categories:spatial and temporal properties.Spatial properties consider the distribution of multicast group members in the network.Temporal properties concentrate on the distribution of inter-arrival time and life time of group mem-bers,in other words,the group member dynamics.In the following, we give an overview of the related work on the modelling of multi-cast group behavior.The majority of multicast research assumes simplifying assump-tions on the distribution of members in the network.Protocol devel-opers assume almost always that users are uniformly distributed in the network(such as[27],[28],[5],[17],[13],and[10],etc.).This is partly due to the unavailability of real data.On the other hand, it is interesting to observe that skewed distributions have been ob-served in multiple aspects of communication networks from traffic behavior[18][20]to preferences for content[11]and peer-to-peer networks[19].There have been some studies on the temporal group properties, such as[4]and[15].[4]measured and studied the member arrival interval and membership duration for MBONE.It also showed that, for multicast sessions on MBONE,an exponential function works well for the member inter-arrival time of all type of sessions,while for membership duration time,an exponential function works well for short sessions,but a Zipf[32]distribution works well for longer sessions.[15]conducted a follow-on study for net games.The au-thors found that player duration timefits an exponential distribu-tion,while inter-arrival timefits a heavy-tailed distribution for net game sessions.Several studies examining the scaling properties of multicast trees ([10][21][8][9][23])and the aggregatability of multicast state ([22][26][30][14])show that the spatial properties do matter in multicast research.In their seminal work,Chuang and Sirbu[10] discovered that the scaling of the tree cost follows power law with respect to the group size,assuming that group members are uni-formly distributed throughout the network.Philips et al.gave an explanation of the Chuang and Sirbu scaling law in[21].They also considered member affinity1,and concluded that,for afixed num-ber of members,affinity can significantly affect the size of the de-livery tree.These two works mainly concentrate on multicast effi-ciency(the gain of multicast vs unicast).Besides defining a metric to measure multicast efficiency,Chalmers and Almeroth([8][9]) also examined the shape of the multicast trees through measure-ments from MBONE,basically focusing on the the distribution and frequency of the degree of in-tree nodes,the depth of receivers,and the node class distribution.In this work,Chalmers and Almeroth also indicate that the multicast efficiency can be affected by the member clustering.The distribution of the group members affects our ability to ag-gregate the multicast state significantly.State aggregation has been the goal of several research efforts([22],[26],and[14]).These pa-pers proposed different state reduction schemes,and showed that group spatial properties,such as clustering of members,correlation between members,affects the performance of their approaches.In [30],Wong et al.did a comprehensive analysis of multicast state scalability considering network topology,group density,cluster-ing/affinity of members and inter-group correlation.They conclude that application-driven membership has significant impact on mul-ticast state distribution and concentration.3.CHARACTERISTICS OF THE GROUPMEMBERSHIPIn this section,we identify and define several properties of group membership,which we quantify through measurements in the next section.For simplicity,we refer to the hosts or routers in the Inter-net as“nodes”or“network nodes”.1.Member Clustering:Clustering captures the proximity ofthe group members.We are interested in the proximity from 1Member affinity means the members are likely to cluster together, while disaffinity means that they tend to spread out.a networking point of view,and we use the network-awareclustering method[16]in our measurement.Earlier studies proposed models to capture the clustering of group members([26],[30]).However,these studies do not provide measurements of the clustering in the Internet.Note that the metrics we present below can refer to a node or a cluster.We will use the term“cluster”,since a node isa cluster of size1.In addition,we focus on clusters in ouranalysis.2.Group Participation Probability:Different clusters havedifferent probabilities in participating in multicast groups: some clusters are more likely to be part of a group.The uni-form distribution of participation is a special case where all clusters have the same probability.Multiple Group Participation:If we have many groups,we define the participation probability of a cluster as the ratio of groups that the cluster joins.Time-based Participation:For a single but long-lived group, the participation probability can be defined as the percentage of time that a cluster is part of the group.Wefind this defini-tion particularly attractive,since our data is often limited in the number of groups.It should be noted that,in our analysis, we use this definition.Fei et al.[14]proposed a node-weighted model to incorpo-rate the difference among network nodes,where each node is assigned a weight representing the probability for that node to be in a group.3.Pairwise Correlation in Group Participation:This metriccaptures the joint probability that two clusters are members of a group.The intuition is that common-interest or related users(e.g.friends)will probably share more than one groups.More specifically,we quantify the pairwise correlation be-tween two clusters as follows.Given two clusters C i andC j,we denote the participation probabilities of cluster C iand C j as p i and p j respectively,and the joint participation probability of C i and C j is denoted by p i,j.The correlation coefficient between C i and C j,coef(i,j),is the normalized covariance between C i and C j([24]):coef(i,j)=(p i,j−p i×p j)i i×j j.(1)Multiple Group Pairwise Correlation:In the presence ofmany groups,we can use the multiple group participationprobability to compute pairwise correlation.Time-based Pairwise Correlation:In this work,we mea-sured and analyzed single but long lived sessions(from MBONE and net games).Thus we can use the time-based participa-tion probability that we defined above to compute time-basedpairwise correlation.In the literature,there has been some effort to model the pair-wise correlation.In[26],a two-dimensional array of ran-domly allocated correlation probabilities is used.In[30],theauthors simulated the correlation implicitly by encouragingthe members of sets of nodes to join the same group,onceone of the nodes of the set has joined.We did notfind any previous studies which use real data to verify and quantify the spatial properties.In addition,no previous effort has provided a comprehensive model for all of the above properties of group membership,as we do here.4.MEMBERSHIP FEATURES MEASUREDFROM MBONE AND NET GAMESIn this section,we measure the properties of multicast group membership in real applications.First,we use data from NASA and IETF broadcasts over the MBONE,which are single-source large-scale application.Second,we measure the membership at net games,which are multiple-source interactive application.The MBONE is an overlay network on the Internet,and it has served asa testbed for multicast researchers games is one of the most popular multiple-source applications.Though most of net games are implemented using multiple unicasts,we are interestedin the membership behavior(or spatial group properties),which is independent of the underlying implementation.4.1Measurement MethodologyMBONE.We use data sets provided by Chalmers and Almeroth from University of Santa Barbara([8][9]).The data sets are di-vided into two groups:real data sets and cumulative data sets, which are summarized in Table1and Table2separately.The real data sets include IETF43-A,IETF43-V and NASA,and the cumulative data sets include UCSB-2000,UCSB-2001,Gatech-2001and UOregon-2001.For the details of measurement on MBONE, please see references[8]and[9].One thing deserving more de-scription is the generation of cumulative data sets:multicast paths are traced using a number of sources(UCSB,Georgia Tech,and Univ.of Oregon)for a series of22,000IP addresses that were known to have participated in multicast groups over a two years period,June’97-June’99.In these data sets,although most of the traces were collected recently and reflect the latest multicast infras-tructure,the group members represent a relatively random sample taken from the older MBONE.Due to the limited number of real data sets,we use cumulative data sets to get an intuition of how the size of groups affects the property of member clustering.Net Games.For net games,we use the QStat tool[3]to collect data.QStat is a program designed to poll and display the status of game servers.Some game servers offer a querying mechanism, which can retrieve some specific information,such as the number of players,players’nicknames,IP addresses,and scores,and the time that each player has been connected,etc.To analyze cluster-ing of members,we need members’IP addresses(the reason will be clarified in Section4.2).Not all games,however,provide play-ers’IP addresses.Quake I is one of the fewer games that allow this.Thus,we choose Quake I though it is a little bit old game. Using QStat,we measured70Quake game servers(obtained froma master server)forfive days(across a weekend),and the servers are polled every one minute.We select the10most popular servers (5of them providing IP addresses of players)for our analysis,and the selected game servers are illustrated in Table3.From each data set of MBONE and net games(note that,one data set corresponds to one multicast session or group),we sample the group membership at regular interval(1minute).Each sample of group membership is composed of members with IP address or player ID for some net games data sets(in which IP address of play-ers are not provided).To give some intuition of the data sets,we plot some examples chosen from real MBONE data and net games data in Fig.1,Fig.2,Fig.3,and Fig.4.In all thesefigures,theX-axis is the time,and the Y-axis represents the number of mem-bers(receivers for MBONE or players for net games).We can see clearly how the number of members changes with the time.Table1:MBONE Real Group Data SetsReceiversName Description Trace Period Total Maximum Average IETF43-A43rd IETF Meeting Audio Dec.7-11,199********.72 IETF43-V43rd IETF Meeting Video Dec.7-11,199********.59 NASA NASA Shuttle Launch Feb.14-21,1999626240.33Table2:MBONE cumulative Group Data SetsReceiversName Description Trace Period Total Maximum Average SYNTH-1UC Santa Barbara Jan.6-10,20001,8711653805.94 SYNTH-2Georgia Tech Jul.12-25,20011,4971497958.17 SYNTH-3University of Oregon Dec.18-19,20011,0191019492.45 SYNTH-4UC Santa Barbara Dec.19-22,20011,0181018474.35Table3:Netgames Group DataSetsPlayersName Game Server Meassurement Period Total Maximum Average May14-18,20023528 1.71 May14-18,200226511 1.89 QS-3195.147.246.71May14-18,200223411 1.72 May14-18,200215810 2.22 QS-5zoologi38.zoologi.su.se May14-18,200239111 2.34 QS-6200.230.198.53:26004May14-18,2002119810 3.31 May14-18,200243716 4.04 May14-18,200241713 3.67 QS-9200.230.198.53:26001May14-18,200212988 3.50 QS-10209.48.106.170May14-18,2002604158.12MBONE multicast:decelerating increase and “black-out”phases.In Fig.1and Fig.2,we see that the IETF broadcast in-creases close to monotonically but with decreasing rate of increase.We also notice some short periods (there is also a big period for IETF Video)in which the number of members drop suddenly and then rise again.One possible explanation is the network instabil-ity:either the tree was torn down and rebuilt or the measurements got lost.Another possible reason is that these might correspond to breaks of the IETF meeting,such as lunch time.Fig.3shows the sampled data sets for NASA broadcast.We see that NASA broad-cast has smaller number of drop periods than IETF broadcast.One reason to explain this is that,unlike IETF meeting,NASA shuttle lunch is a more continuous event.The big drop period can be ex-plained by some break of network connection or some unexpected and uninteresting event.Net games:membership is strongly periodic.Fig.4shows very interesting behavior of net games (Quake)players:in each day,there is a big spike in user participation.Moreover,there are more players during the weekend (May 17th and May 18th).This periodicity is natural given the nature of the activity:For a game server,due to the delay constraints of gaming,most of the players come from areas within some range (say,in several hops).Thus the players are more likely active in some relatively fixed period of time in a day.For example,in Fig.4,we see that late night is a very active period for game players in this server.102030405060708090100Dec 07Dec 08Dec 09Dec 10Dec 11Dec 12N u m b e r o f R e c e i v e r sDaysFigure 1:The data set sampled from IETF Meeting Audio (IETF43-A).In the rest of this section,we examine membership properties of the above data.4.2Member ClusteringTo model member clustering,we employ network-aware cluster-ing.Intuitively,two members should be in the same cluster if they are close in terms of network routing.In the Internet,this kind of grouping can be done based on IP addresses.We adopt the method in [16]to identify member clusters using network prefixes,based on information available from BGP routing snapshots (we use the BGP dump tables obtained from [2]).This way,clustered nodes are likely to to be under common administrative control.For details,please see [16].We briefly outline network-aware clustering for completeness.We first extract the network prefixes/netmasks from BGP dump tables and the IP addresses of members from group membership samples,then we classify all the member IP addresses that have the same longest-match prefix into one cluster,which is identi-102030405060708090100Dec 07Dec 08Dec 09Dec 10Dec 11Dec 12N u m b e r o f R e c e i v e r sDaysFigure 2:The data set sampled from IETF Meeting Video (IETF43-V).010203040506070Feb 14Feb 15Feb 16Feb 17Feb 18Feb 19Feb 20Feb 21Feb 22N u m b e r o f R e c e i v e r sDaysFigure 3:The data set sampled from NASA Shuttle Lunch (NASA).1234567891011121314151617May 14May 15May 16May 17May 18May 19N u m b e r o f P l a y e r sDaysFigure 4:The data set sampled from net game server 1(QS-7).fied by the shared prefix.For example,suppose we want to clus-ter the IP addresses 216.123.0.1,216.123.1.5,216.123.16.59,and 216.123.51.87.In the routing table,we find the longest-match pre-fixes are 216.123.0.0/19,216.123.0.0/19,216.123.0.0/19,and 216.123.48.0/21respectively.Then we can classify the first threeIP addresses into a cluster identified by prefix/netmask 216.123.0.0/19and the last one into another cluster identified by 216.123.48.0/21.It should be to noted that other clustering methods,such as network topology based approach,are possible.But network-aware cluster-ing is an easy and effective way for us to do clustering considering the data we have achieved.In later sections,we will show that our analysis and model are not constrained by the clustering method.For each data set,we want to see the number of group mem-bers per cluster.Here,we refer to the number of group members in a cluster as the size of the cluster (or cluster size).For all the group membership samples,we examine the Cumulative Distribu-tion Function (CDF)of the cluster size.The results for data sets from MBONE and net games are shown in Fig.5.Therefore,for a given cluster size in the X-axis,we see how many clusters have at most that size.C D FCluster size IETF video IETF audioNASAGatech 2001UCSB 2000UCSB 2001Univ. OregonNetgamesFigure 5:CDF of cluster size for data sets from MBONE and net games.The upper set of curves are for net game data sets (with 5game servers providing IP addresses of players),the middle set of curves are for MBONE real data sets (IETF43-A,IETF43-A,and NASA),and the lower set of curves are for MBONE cumulative data sets.Group members form clusters with skewed size distribution.Group members are significantly clustered.In Fig.5,we can see three different groups of curves:the upper group for net game data sets (with 5game servers providing IP addresses of players),the middle group for MBONE real data sets (IETF43-A,IETF43-A,and NASA),and the lower group for MBONE cumulative data sets.In each group,the data sets have similar member clustering prop-erty:for example,for MBONE real data sets,more than 20%clus-ters have 2or more group members;for MBONE cumulative data sets (UCSB-2000,UCSB-2001,Gatech-2001and UOregon-2001),they also have similar features:more than 60%clusters have 2or more group members;while for net games,the corresponding group of CDF curves do not show significant member clustering:about 90%“clusters”have size 1.Cluster size distribution is mainly affected by the group size.We observe that it is primarily the size of the group that affects the range of the distribution.However,the cluster size distribu-tion is similar qualitatively in all three groups of data sets.When comparing the groups of curves,we can conclude that the MBONE cumulative data sets have more significant clustering feature than MBONE real data sets.We attribute this to the larger size of the group.The average number of members for each cumulative data set (from 500to 1000)is much higher than that for real data sets (around 50).The bigger the group is,the more members tend tobe in one cluster and the more significant of the member clustering feature is.As for net games,the feature of member clustering is even less significant:most “clusters”have only one member,which means that Quake players are more likely scattered over the net-work.This observation suggests that probably the gain from some multicast or intelligent caching schemes may not provide signifi-cant benefits in this case.The absence of clustering in the net games can be attributed to many factors.One observation is that the maximum number of players (16in Quake)is controlled by the game servers because of management issues.Thus,the possibility for the members to fall in one cluster becomes smaller.It would be very interesting to examine a net game with a larger user participation,but we were not able to get such data.Another possible explanation may be that the game players are not necessarily from a similar area of the network potentially.This suggests that gaming community is scat-tered,or alternatively,that net games bring together people from significantly different places.The practical implications of member clustering.Understand-ing the clustering properties can help us develop efficient protocols to improve the scalability and the performance of applications.The member clustering captures the proximity of the members in the network especially with the use of network-aware clustering.For example,in a well clustered group,we can potentially develop hi-erarchical protocols that can exploit the spatial distribution of the members like hierarchical multicasting.4.3Group Participation ProbabilityWe find that the participation probability is non-uniform across clusters or nodes.This strongly suggests that the uniform distri-bution used so far for most research is not realistic.In the analysis below,we study the distribution across clusters or nodes that par-ticipate at least once in a multicast group.Clearly,there are clusters or nodes in the network that do not appear in any group.In fact,we expect that these clusters (or nodes)are probably large in number,which reinforces the observation that not all clusters (or nodes)are created equal regarding multicast participation.Note that,given the limited number of groups,we measured the time-based participation probability as defined in the previous sec-tion.We give the CDF of the participation probability of clusters or nodes for MBONE and net games in Fig.6and Fig.7respectively.Given a probability in the X-axis,we can see how many clusters or nodes have at most that probability to participate in the multicast group.MBONE:the cluster participation probability is non-uniform.In Fig.6,we see that the MBONE clusters are not equal in partic-ipating in a group.If the clusters had the same probability p of participating in a group,the CDF of the participation probability would appear as a vertical line at the exact p on the X-axis.The current plot of the CDF shows a roughly linear increase with close to 45degrees slope.This suggests that we have a wide range of participation probabilities:for any value on the X-axis,we can find a cluster with such a participation probability.Net Games:the node participation probability roughly fol-lows uniform distribution.For net games,since the member clus-tering feature is not significant at all (about 90%“clusters”have size 1),we simply analyze the node participation probability which is approximated by the frequency of nodes joining the net game session.Fig.7plots the CDF of the participation probability for net games.We observe that the plot is qualitatively different from the MBONE distribution.For all the Quake servers we examined,more than。