线性代数项目-mathematics
mathematica求解矩阵方程
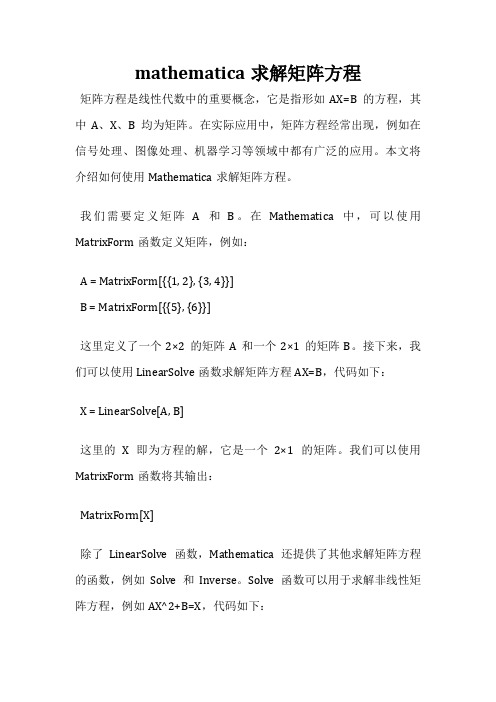
mathematica求解矩阵方程矩阵方程是线性代数中的重要概念,它是指形如AX=B的方程,其中A、X、B均为矩阵。
在实际应用中,矩阵方程经常出现,例如在信号处理、图像处理、机器学习等领域中都有广泛的应用。
本文将介绍如何使用Mathematica求解矩阵方程。
我们需要定义矩阵A和B。
在Mathematica中,可以使用MatrixForm函数定义矩阵,例如:A = MatrixForm[{{1, 2}, {3, 4}}]B = MatrixForm[{{5}, {6}}]这里定义了一个2×2的矩阵A和一个2×1的矩阵B。
接下来,我们可以使用LinearSolve函数求解矩阵方程AX=B,代码如下:X = LinearSolve[A, B]这里的X即为方程的解,它是一个2×1的矩阵。
我们可以使用MatrixForm函数将其输出:MatrixForm[X]除了LinearSolve函数,Mathematica还提供了其他求解矩阵方程的函数,例如Solve和Inverse。
Solve函数可以用于求解非线性矩阵方程,例如AX^2+B=X,代码如下:X = Solve[A.X^2 + B == X, X]这里的X是一个列表,包含了方程的所有解。
Inverse函数可以用于求解可逆矩阵方程,例如AX=B,代码如下:X = Inverse[A].B这里的X即为方程的解,它与使用LinearSolve函数求解的结果相同。
除了求解矩阵方程,Mathematica还可以进行矩阵运算、矩阵分解、矩阵求导等操作,使得矩阵计算变得更加方便和高效。
例如,我们可以使用MatrixPower函数求解矩阵的幂,代码如下:A^2这里的结果是一个2×2的矩阵,即A的平方。
我们还可以使用Eigensystem函数求解矩阵的特征值和特征向量,代码如下:Eigensystem[A]这里的结果是一个列表,包含了矩阵A的特征值和特征向量。
mathematica 行向量 列向量 矩阵
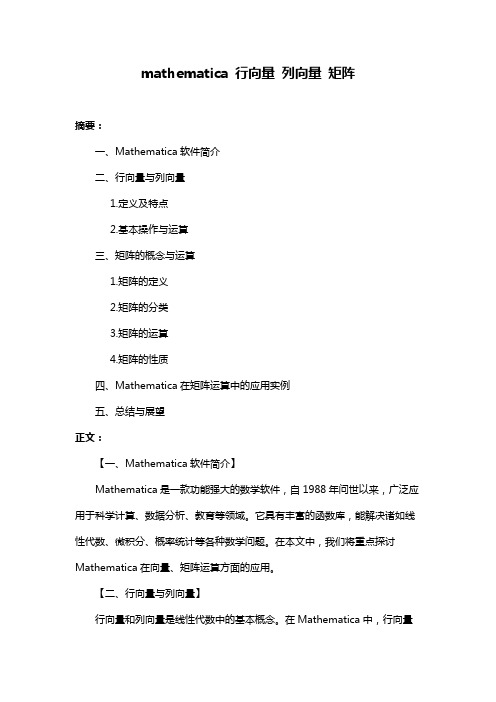
mathematica 行向量列向量矩阵摘要:一、Mathematica软件简介二、行向量与列向量1.定义及特点2.基本操作与运算三、矩阵的概念与运算1.矩阵的定义2.矩阵的分类3.矩阵的运算4.矩阵的性质四、Mathematica在矩阵运算中的应用实例五、总结与展望正文:【一、Mathematica软件简介】Mathematica是一款功能强大的数学软件,自1988年问世以来,广泛应用于科学计算、数据分析、教育等领域。
它具有丰富的函数库,能解决诸如线性代数、微积分、概率统计等各种数学问题。
在本文中,我们将重点探讨Mathematica在向量、矩阵运算方面的应用。
【二、行向量与列向量】行向量和列向量是线性代数中的基本概念。
在Mathematica中,行向量和列向量分别表示为rows和columns。
【1.定义及特点】行向量:一个由n个元素组成的1×n矩阵,其中n为自然数。
行向量有n 个分量,分别表示该向量在各个方向上的分量值。
列向量:一个由n个元素组成的n×1矩阵,其中n为自然数。
列向量有n 个分量,分别表示该向量在每个方向上的分量值。
【2.基本操作与运算】在Mathematica中,行向量和列向量的基本操作与运算主要包括以下几点:1.加法:两个向量相加,结果为一个新向量,其元素为两个向量对应分量之和。
2.减法:两个向量相减,结果为一个新向量,其元素为两个向量对应分量之差。
3.数乘:向量与实数相乘,结果为一个新向量,其元素为原向量对应分量乘以实数。
4.标量积:两个向量的标量积为一个实数,等于两个向量对应分量的乘积之和。
5.向量积:两个向量的向量积为一个新向量,其分量依次为两个向量对应分量的向量积。
【三、矩阵的概念与运算】矩阵是线性代数中的核心概念,它可以看作是一个由行向量或列向量组成的矩形阵列。
在Mathematica中,矩阵表示为一个二维数组。
【1.矩阵的定义】矩阵是一个m×n的矩阵,其中m表示矩阵的行数,n表示矩阵的列数。
Mathematica教程用Mathematica求解线性代数基本问题

Module[{x,y,...},body] Module[{x=x0,y=y0,…},body] lhs:=Module[vars,rhs/:cond] Block[{x,y,... },body] Block[{x=x0,y=y0,…},bddy]
具有局部变量x,y… 的模块
具有初始值的局部变 量的模块 rhs和cond共享局部 变量 运用局部值x,y, …计 算body 给x,y,..赋初始值
Do循环结构
Do[expr,{i,imax}] 循环计算expr,以步长1,i从 1增加到imax
循环计算expr,以步长di,i Do[expr,{i,imin,imax,di}] 从imin增加到imax Do[expr,{n}] 循环计算expr n次
•
•
•
键入
t0={{1,2,3},{4,5,6}}
则得到一个名为t0的2行3列的矩阵。
• 2、也可利用工具栏或菜单输入矩阵 • 点击工具栏上的矩阵输入的工具,就会得到一个 二行二列的矩阵输入框,若不是二行二列的矩阵, 可通过按Ctrl+Enter键增加一行,按Ctrl+,键增加 一列,用鼠标选定一行(或一列),按Del键可删 除一行(或一列)。通过这样的操作,就可输入任 意一个矩阵。下面的图演示了这个过程。
Out[5]=True
ln[6]:=TrueQ[x==y]
Out[6]=false
用“===”可直接测试两个表达式的等同性:
In[7]:=x===y
Out[7]:=False
一般情况下,“===”返回值为真(True)或假(False), 而“==”为符号形式输出,表示一个符号等式。 在 特殊情况下可用“===”测试一个表达式的 结构,而用“==”测试数学上的等同性。
数学 的单词
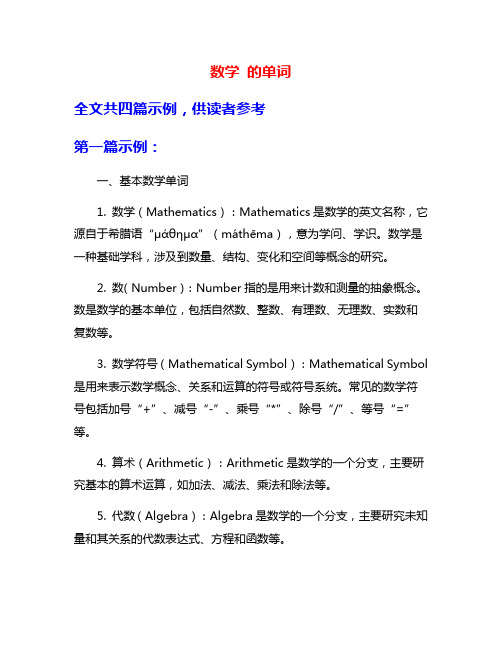
数学的单词全文共四篇示例,供读者参考第一篇示例:一、基本数学单词1. 数学(Mathematics):Mathematics是数学的英文名称,它源自于希腊语“μάθημα”(máthēma),意为学问、学识。
数学是一种基础学科,涉及到数量、结构、变化和空间等概念的研究。
2. 数(Number):Number指的是用来计数和测量的抽象概念。
数是数学的基本单位,包括自然数、整数、有理数、无理数、实数和复数等。
3. 数学符号(Mathematical Symbol):Mathematical Symbol 是用来表示数学概念、关系和运算的符号或符号系统。
常见的数学符号包括加号“+”、减号“-”、乘号“*”、除号“/”、等号“=”等。
4. 算术(Arithmetic):Arithmetic是数学的一个分支,主要研究基本的算术运算,如加法、减法、乘法和除法等。
5. 代数(Algebra):Algebra是数学的一个分支,主要研究未知量和其关系的代数表达式、方程和函数等。
6. 几何(Geometry):Geometry是数学的一个分支,主要研究空间形状、大小、位置关系和变化等几何概念。
7. 微积分(Calculus):Calculus是数学的一个分支,主要研究变化率、极限、积分和微分等连续变量的数学概念。
8. 概率论(Probability):Probability是数学的一个分支,主要研究随机事件发生的可能性和规律等概率性问题。
9. 统计学(Statistics):Statistics是数学的一个分支,主要研究数据的收集、分析、解释和预测等统计性问题。
1. 加法(Addition):Addition指的是将两个或多个数相加的运算,如2+3=5。
2. 减法(Subtraction):Subtraction指的是将一个数从另一个数中减去的运算,如4-2=2。
5. 平方(Square):Square指的是一个数的平方,如3的平方是9。
Mathematica的主要功能

3、数
Mathematica 以符号运算为主,这与一些语言有所不同,例如源自, e, 2 ,3
2 等符号表示准确数,近似数用带小数点的数表示,例如
1.2,2.3*10^5 等。Mathematica 中求近似值以及近似值的精度控制
函数为函数“N”,其调用格式如下:
N[表达式] 计算表达式的近似值,具有机器规定的精度(16 位有 效数字),但是按标准输出只显示前 6 位有效数字
每次运行结束后,Mathematica 会自动在输入的式子前面加上 “In[n]:=”(n 表示输入命令的序列号),在输出的答案前面加上 “Out[n]=”(n 表示输出结果的序列号),以便分清输入和输出并 自动加上编号。可以用“%”表示前一个输出的内容,“%%” 表 示倒数第 2 个输出的内容,依此类推,“% n”表示第 n 个(即 Out[n])输出的内容。也就是说 Mathematica 输出的内容被系统 记忆,它们可以像其它变量一样在后面的计算中引用。
四、编程基础
1、自定义函数
前面介绍了 Mathematica 本身自带的内置函数,下面我们以实 例来说明定义函数的方法。例如,要定义函数 f (x) ex (sin x 1) ln x2 , 我们只要键入命令 f[x_]:=Exp[x]*(Sin[x]+1)+Log[x^2] 运行即可。
注意:在函数的自变量后面有一个下划线“_”,这表示 x 为自变量, 可以把 x 代入为任何的值进行计算;等号前面的有个冒号,表示定 义函数。同样可以定义多变量函数。定义了函数 f[x]后,可以直接 地调用 f[x]来进行符号数学运算(例如积分、微分等)
三、基本代数运算
下面介绍一些实现基本代数运算的函数,用于变换数学表达式、解 方程和解不等式。Mathematica 具有强大的符号运算功能,下面列 举的函数均可代入具有字母的表达式进行计算,得到精确解。
mathematics矩阵运算
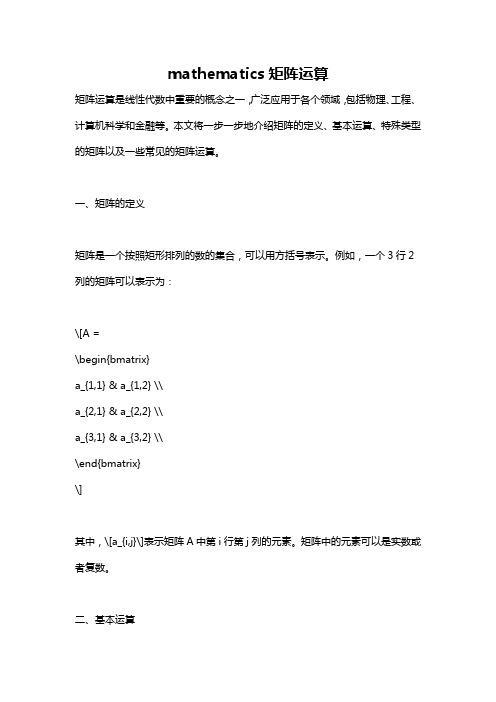
mathematics矩阵运算矩阵运算是线性代数中重要的概念之一,广泛应用于各个领域,包括物理、工程、计算机科学和金融等。
本文将一步一步地介绍矩阵的定义、基本运算、特殊类型的矩阵以及一些常见的矩阵运算。
一、矩阵的定义矩阵是一个按照矩形排列的数的集合,可以用方括号表示。
例如,一个3行2列的矩阵可以表示为:\[A =\begin{bmatrix}a_{1,1} & a_{1,2} \\a_{2,1} & a_{2,2} \\a_{3,1} & a_{3,2} \\\end{bmatrix}\]其中,\[a_{i,j}\]表示矩阵A中第i行第j列的元素。
矩阵中的元素可以是实数或者复数。
二、基本运算1. 矩阵的加法和减法:两个相同大小的矩阵可以进行加法和减法运算。
对应位置上的元素相加或相减,得到的结果矩阵具有相同的大小。
例如,对于两个3行2列的矩阵\[A\]和\[B\],它们的和\[A + B\]可以表示为:\[A + B =\begin{bmatrix}a_{1,1}+b_{1,1} & a_{1,2}+b_{1,2} \\a_{2,1}+b_{2,1} & a_{2,2}+b_{2,2} \\a_{3,1}+b_{3,1} & a_{3,2}+b_{3,2} \\\end{bmatrix}\]2. 矩阵的标量乘法:矩阵可以与一个实数或者复数进行乘法运算,我们称之为标量乘法。
将矩阵中的每一个元素与标量相乘,得到的结果矩阵具有相同的大小。
例如,对于一个3行2列的矩阵\[A\]和一个标量\[k\],它们的乘积\[k \cdot A\]可以表示为:\[k \cdot A =\begin{bmatrix}k \cdot a_{1,1} & k \cdot a_{1,2} \\k \cdot a_{2,1} & k \cdot a_{2,2} \\k \cdot a_{3,1} & k \cdot a_{3,2} \\\end{bmatrix}\]3. 矩阵的乘法:矩阵的乘法是定义在两个矩阵之间的运算,它不同于矩阵加法和减法。
线性代数(linearalgebra)
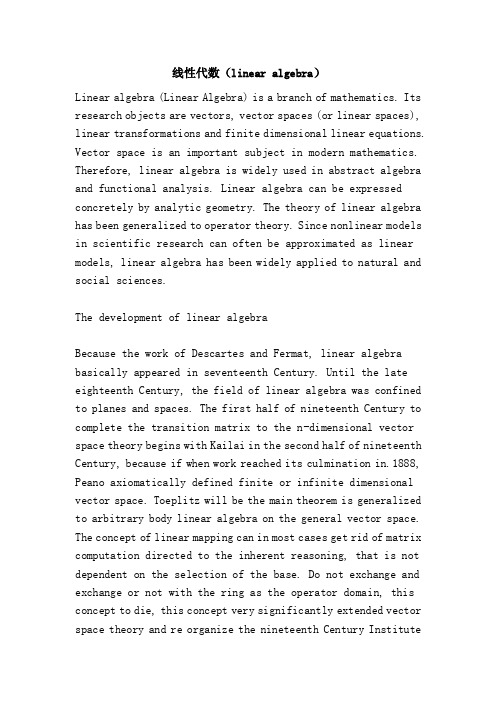
线性代数(linear algebra)Linear algebra (Linear Algebra) is a branch of mathematics. Its research objects are vectors, vector spaces (or linear spaces), linear transformations and finite dimensional linear equations. Vector space is an important subject in modern mathematics. Therefore, linear algebra is widely used in abstract algebra and functional analysis. Linear algebra can be expressed concretely by analytic geometry. The theory of linear algebra has been generalized to operator theory. Since nonlinear models in scientific research can often be approximated as linear models, linear algebra has been widely applied to natural and social sciences.The development of linear algebraBecause the work of Descartes and Fermat, linear algebra basically appeared in seventeenth Century. Until the late eighteenth Century, the field of linear algebra was confined to planes and spaces. The first half of nineteenth Century to complete the transition matrix to the n-dimensional vector space theory begins with Kailai in the second half of nineteenth Century, because if when work reached its culmination in.1888, Peano axiomatically defined finite or infinite dimensional vector space. Toeplitz will be the main theorem is generalized to arbitrary body linear algebra on the general vector space. The concept of linear mapping can in most cases get rid of matrix computation directed to the inherent reasoning, that is not dependent on the selection of the base. Do not exchange and exchange or not with the ring as the operator domain, this concept to die, this concept very significantly extended vector space theory and re organize the nineteenth Century Instituteof the.The word "algebra" appeared relatively late in China, in the Qing Dynasty when the incoming China, it was translated into "Alj Bala", until 1859, the Qing Dynasty famous mathematician, translator Li Shanlan translated it as "algebra", still in use.The status of linear algebraLinear algebra is a subject that discusses matrix theory and finite dimensional vector spaces combined with matrices and their linear transformation theory.The main theory is mature in nineteenth Century, and the first cornerstone (the solution of two or three Yuan linear equations) appeared as early as two thousand years ago (see in our ancient mathematical masterpiece "nine chapters arithmetic").The linear algebra has many important applications in mathematics, mechanics, physics and technology, so it has important place in various branches of algebra;In the computer today, computer graphics, computer aided design, cryptography, virtual reality and so on are all part of the theory and algorithm of linear algebra;.Between geometric and algebraic methods embodied in the concept of the subject of the connection from the axiomatic method on the abstract concept and rigorous logic reasoning, cleverly summed up, to strengthen people's training in mathematics, science and intelligent gain is very useful;And with the development of science, we should not only study the relationship between the individual variables, but also further study the relationship between multiple variables, all kinds of practical problems in most cases can be linearized, and because of the development of the computer, the linearized problem can be calculated, linear algebra is a powerful tool to solve these problems.Basic introduction to linear algebraLinear algebra originated from the study of two-dimensional and three-dimensional Cartesian coordinate systems. Here, a vector is a line segment with a direction that is represented by both length and direction. Thus vectors can be used to represent physical quantities, such as force, or to add and multiply scalar quantities. This is the first example of a real vector space.Modern linear algebra has been extended to study arbitrary or infinite dimensional spaces. A vector space of dimension n is called n-dimensional space. In two-dimensional andthree-dimensional space, most useful conclusions can be extended to these high-dimensional spaces. Although many people do not easily imagine vectors in n-dimensional space, such vectors (i.e., n tuples) are very useful for representing data. Since n is a tuple, and the vector is an ordered list of n elements, most people can effectively generalize and manipulate data in this framework. For example, in economics, 8 dimensional vectors can be used to represent the gross national product (GNP) of 8 countries. When all the nationalorder (such as scheduled, China, the United States, Britain, France, Germany, Spain, India, Australia), you can use the vector (V1, V2, V3, V4, V5, V6, V7, V8) showed that these countries a year each GNP. Here, each country's GNP are in their respective positions.As a purely abstract concept used in proving theorems, vector spaces (linear spaces) are part of abstract algebra and have been well integrated into this field. Some notable examples are: irreversible linear maps or groups of matrices, rings of linear mappings in vector spaces. Linear algebra also plays an important role in mathematical analysis,Especially in vector analysis, higher order derivatives are described, and tensor product and commutative mapping are studied.A vector space is defined on a domain, such as a real or complex domain. Linear operators map the elements of a linear space into another linear space (or in the same linear space), and maintain the consistency of addition and scalar multiplication in the vector space. The set of all such transformations is itself a vector space. If a basis of linear space is determined, all linear transformations can be expressed as a table, called matrix. Further studies of matrix properties and matrix algorithms (including determinants and eigenvectors) are also considered part of linear algebra.We can simply say that the linear problems in Mathematics - those that exhibit linear problems - are most likely to be solved. For example, differential calculus studies the problemof linear approximation of functions. In practice, the difference between a nonlinear problem and a nonlinear one is very important.The linear algebra method refers to the problem of using a linear viewpoint to describe it and to describe it in the language of linear algebra and to solve it (when necessary) by using matrix operations. This is one of the most important applications in mathematics and engineering.Some useful theoremsEvery linear space has a base.The nonzero matrix n for a row of N rows A, if there is a matrix B that makes AB = BA = I (I is the unit matrix), then A is nonsingular matrix.A matrix is nonsingular if and only if its determinant is not zero.A matrix is nonsingular if and only if the linear transformation it represents is a automorphism.A matrix is semi positive if and only if each of its eigenvalues is greater than or equal to zero.A matrix is positive if and only if each of its eigenvalues is greater than zero.Generalizations and related topicsLinear algebra is a successful theory, and its method has been applied to other branches of mathematics.The theory of modulus is to study the substitution of scalar domains in linear algebra by ring substitution.Multilinear algebra transforms the "multivariable" problem of mapping into the problem of each variable, resulting in the concept of tensor.In the spectral theory of operators, by using mathematical analysis, infinite dimensional matrices can be controlled.All of these areas have very large technical difficulties.Basic contents of linear algebra in Chinese UniversitiesFirst, the nature and tasks of the courseThe course of linear algebra is an important basic theory course required by students of science and Engineering in universities and colleges. It is widely used in every field of science and technology. Especially today, with the development and popularization of computer, linear algebra has become the basic theory knowledge and important mathematical tool for engineering students. Linear algebra is to train thehigh-quality specialized personnel needed for the socialist modernization construction of our country. Through the study of this course, we should make students get:1 determinant2, matrix3. The correlation of vectors and the rank of matrices4 、 linear equations5, similar matrix and two typeAnd other basic concepts, basic theories and basic operational skills, and lay the necessary mathematical foundation for further courses and further knowledge of mathematics.While imparting knowledge through various teaching links gradually cultivate students with abstract thinking ability, logical reasoning ability, spatial imagination ability and self-learning ability, but also pay special attention to cultivate students with good operation ability and comprehensive use of the knowledge to the ability to analyze and solve problems.Two, the content of the course teaching, basic requirements and class allocation(1) teaching content1 determinant(1) definition of order n determinant(2) the nature of determinant(3) the calculation of the determinant is carried out in rows (columns)(4) the Clem rule for solving linear equations2, matrix(1) the concept of matrix, unit matrix, diagonal matrix, symmetric matrix(2) linear operations, multiplication operations, transpose operations and laws of matrices(3) inverse matrix concept and its properties, and inverse matrix with adjoint matrix(4) the operation of partitioned matrices3 vector(1) the concept of n-dimensional vectors(2) the linear correlation, linear independence definition and related theorems of vector groups, and the judgement of linear correlation(3) the maximal independent group of vectors and the rank of vectors(4) the concept of rank of matrix(5) elementary transformation of matrix, rank and inverse matrix of matrix by elementary transformation(6) n-dimensional vector spaces and subspaces, bases, dimensions, coordinates of vectors4 、 linear equations(1) the necessary and sufficient conditions for the existence of nonzero solutions of homogeneous linear equations and the necessary and sufficient conditions for the existence of solutions of nonhomogeneous linear equations(2) the fundamental solution, the general solution and the solution structure of the system of linear equations(3) the condition and judgement of the solution of nonhomogeneous linear equations and the solution of the system of equations(4) finding the general solution of linear equations by elementary row transformation5, similar matrix and two type(1) eigenvalues and eigenvectors of matrices and their solutions(2) similarity matrix and its properties(3) the necessary and sufficient conditions and methods of diagonalization of matrices(4) similar diagonal matrices of real symmetric matrices(5) two type and its matrix representation(6) the method of linearly independent vector group orthogonal normalization(7) the concept and property of orthogonal transformation and orthogonal matrix(8) orthogonal transformation is used as the standard shape of the two type(9) the canonical form of quadratic form and two form of two type are formulated by formula(10) the inertia theorem, the rank of the two type, the positive definite of the two type and their discrimination(two) basic requirements1, understand the definition of order n determinant, will use the definition of simple determinant calculation2, master the basic calculation methods and properties of determinant3, master Clem's law4. Understand the definition of a matrix5, master the matrix operation method and inverse matrix method6. Understanding the concept of vector dependency defines the relevance of the vector by definition7, grasp the method of finding the rank of the matrix, and understand the relation between the rank of the matrix and the correlation of the vector group8, understand the concept of vector space, will seek vector coordinates9. Master the matrix rank and inverse matrix with elementary transformation, and solve the system of linear equations10, master the method of solving linear equations, and know the simple application of linear equations11. Master the method of matrix eigenvalue and eigenvector12. Grasp the concept of similar matrices and the concept of diagonalization of matrices13, master the orthogonal transformation of two times for standard type method14, understand the inertia theorem of the two type, and use thematching method to find the sum of squares of the two type15. Grasp the concept and application of the positive definiteness of the two typeMATLABIt is a programming language and can be used as a teaching software for engineering linear algebra. It has been introduced into many university textbooks at home and abroad.。
mathematica计算n阶行列式

mathematica计算n阶行列式n阶行列式是线性代数中的重要概念,它在矩阵理论、线性方程组的求解以及求解线性变换等方面有着广泛的应用。
在Mathematica 中,我们可以使用Det函数来计算n阶行列式的值。
行列式是一个由元素组成的方阵,其中元素的排列方式对计算结果起到关键作用。
对于一个n阶行列式来说,我们可以通过对第一行(或第一列)展开来计算其值。
展开后的表达式是一个多项式,其中每一项的系数就是对应元素的代数余子式。
在Mathematica中,我们可以使用MatrixForm函数来直观地表示一个矩阵。
例如,对于一个3阶行列式来说,我们可以使用如下代码进行表示:```mathematicaMatrixForm[{{a, b, c}, {d, e, f}, {g, h, i}}]```其中,a、b、c等为矩阵的元素。
这样,我们就可以清晰地看到矩阵的结构,便于进行计算和分析。
接下来,我们将使用Det函数来计算一个n阶行列式的值。
假设我们有一个4阶行列式,可以使用如下代码进行计算:```mathematicaDet[{{a, b, c, d}, {e, f, g, h}, {i, j, k, l}, {m, n, o, p}}]```其中,a、b、c等为矩阵的元素。
运行此代码后,Mathematica将返回计算结果,即该4阶行列式的值。
除了计算n阶行列式的值,Mathematica还提供了其他一些与行列式相关的函数,如Eigenvalues、Eigenvectors等。
这些函数可以用于计算矩阵的特征值和特征向量,从而进一步分析矩阵的性质和行列式的特点。
除了使用Det函数外,我们还可以使用Simplify函数对行列式进行化简。
化简后的行列式可以更好地展示其特点和性质,便于我们进行分析和理解。
在Mathematica中,我们还可以使用Transpose函数对矩阵进行转置。
转置后的矩阵可以改变行列式的排列方式,从而影响行列式的计算结果。
- 1、下载文档前请自行甄别文档内容的完整性,平台不提供额外的编辑、内容补充、找答案等附加服务。
- 2、"仅部分预览"的文档,不可在线预览部分如存在完整性等问题,可反馈申请退款(可完整预览的文档不适用该条件!)。
- 3、如文档侵犯您的权益,请联系客服反馈,我们会尽快为您处理(人工客服工作时间:9:00-18:30)。
大连东软信息学院
12-13学年第2学期《线性代数》课程(单元)项
目研究报告
信心开发12001班
项目一☆☆☆☆☆☆
【项目内容】详细叙述拟完成项目的条件和问题,可配表或图。
假设在比赛中8名选手进行单循环赛,比赛成绩可以用一个8*8矩阵D= (aij)表示,其中矩阵元素规定为:若选手i胜了选手j,则aij=1,否则令aij=0,那么我们得到一个类似于
的矩阵,如何据此对8名选手进行比赛排名?
【相关知识点】列出完成项目要用到的知识点及方法
知识点:
矩阵的转置
矩阵的乘法
矩阵的加法
方法:
基本的知识点运用
【模型假设与分析】对项目问题进行必要的分析和必要的假设分析:每一行的和等于选手i总共获胜的次数,因此以D的行和作为选拔的标准,
即以矩阵S=De判断,其中容易算出
分量S=(5,3,4,4,2,5,3,2)。
但是容易看出,这个分量并
不能达到我们的目的,因为它不能区分出选手三和选手四,选手一
和选手六,选手二和选手七,选手五和选手八的高低,仅仅由S
不能反映出各队真正的实力名次
假设:
为了清楚的表达这类胜负情况,我们运用如图1所示来说明一个性
质
图一所示有向图可对应于一个矩阵,P=(aij)
其中aij=1表示存在从行标相应点到列标相应点的带箭头的边,即有向边,没有此边则令aij=0
又
其中P2的元素有特定意义,例如P2(1,3)元素为1,由矩阵的乘法运算得:
该元素的几何意义相当于从A点到C点长度为2的路径仅有1条:AC->CC 可以推广的是,对于由n个点构成的有向图,P2的(i,j)素值
表示从顶点i到j长度为2的路径数目。
进一步证明的是Pm的(i,j)元素表示从顶点i到j的长度为m的路径数目
【模型建立】由上述分析建立数学模型
回到竞赛排名的问题,实际上这也是可以表示为一个有向图的,对选手一
和六,选手二和七,选手三和四,选手五和八,仅通过长度为1的路径数目已经无法判别,应在充分考虑对手的强弱因素,减少选手发挥视屏不正常而带来的影响,即避免了强队偶然输给弱队带来名次的大落,又应考虑到弱队超水平发挥后的名次上升,因此以D+D2的行和作为选拔标准比较合理
【模型求解】应用相关知识和方法详细求解数学模型,可使用数学软件,必要时可插入软件程序或者软件求解的截图。
经计算得:
由(D+D2)e算出分量为:
T=(19,12,16,15,9,24,11,10)
【结论及分析】对求解的结论结合实际问题给出合理的解释,并分析结论的优劣,是否可继续改进,给出改进的方向及方法等。
结论:
据此可对8名选手给出排名从高到低分分别是,选手六,选手一,选手三,选手四,选手二,选手七,选手八,选手五
分析:
例如:D2(1,2)=1的实际意义通过战胜1名选手从而达到间接战胜了选手二的效果(具体来说选手一通过战胜选手八,选手八战胜选手二),当然,在同等比较情况下,间接战胜数越大,说明该选手的相对实力越强,例如D2(1,5)=3,D2(6,5)=5,说明同样是对选手五比赛,选手六相对实力超过了选手一,因为选手六战胜了5(选手一的这个数字只有3)名其他选手,这些选手又战胜了选手五,所以最后的总排名,需结合D,D2的行和来判断没命选手的相对实力总和,即根据T=(D+D2)e进行排名,
若仍出现相同元素值无法排名,在以此类推D3,继续增加路径长度计算路径数目:
【心得与体会】列出完成该项目的收获,以及在促进知识学习、能力提升、团队合作方面的心得与体会。
随着当代科学技术,特别是计算机技术的飞速发展,线性代数课程也经历着变革,我们应该适应变革,积极努力做新实际的人才,通过例子和练习将知识融会贯通,逐步培养我们的创新能力和实践能力
【参考文献】
1.田原,沈亦一,线性代数【M】.上海:华东理工大学出版社2007
2.同济大学应用数学系,工程数学线性代数【M】.北京:高等教育出版
社2003
3.袁震东,数学建模法【M】.上海:华东师范大学出版社2003
百度文库:“浅谈线性代数的应用教学策略”。