Abstract
英语摘要写法
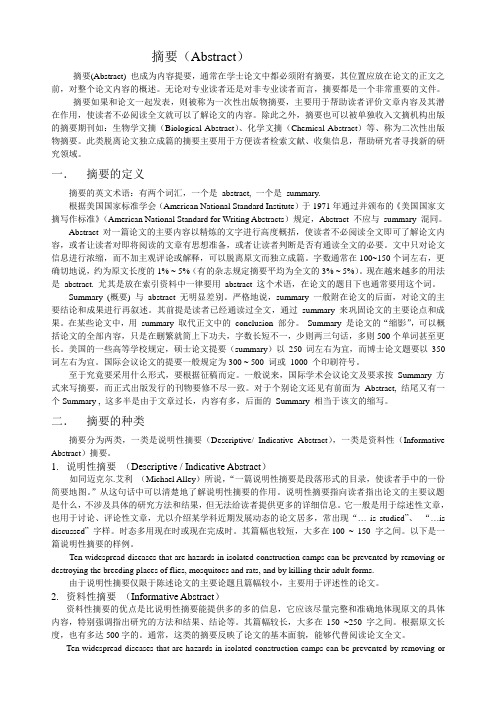
摘要(Abstract)摘要(Abstract) 也成为内容提要,通常在学士论文中都必须附有摘要,其位置应放在论文的正文之前,对整个论文内容的概述。
无论对专业读者还是对非专业读者而言,摘要都是一个非常重要的文件。
摘要如果和论文一起发表,则被称为一次性出版物摘要,主要用于帮助读者评价文章内容及其潜在作用,使读者不必阅读全文就可以了解论文的内容。
除此之外,摘要也可以被单独收入文摘机构出版的摘要期刊如:生物学文摘(Biological Abstract)、化学文摘(Chemical Abstract)等、称为二次性出版物摘要。
此类脱离论文独立成篇的摘要主要用于方便读者检索文献、收集信息,帮助研究者寻找新的研究领域。
一.摘要的定义摘要的英文术语:有两个词汇,一个是abstract, 一个是summary.根据美国国家标准学会(American National Standard Institute)于1971年通过并颁布的《美国国家文摘写作标准》(American National Standard for Writing Abstracts)规定,Abstract 不应与summary 混同。
Abstract 对一篇论文的主要内容以精炼的文字进行高度概括,使读者不必阅读全文即可了解论文内容,或者让读者对即将阅读的文章有思想准备,或者让读者判断是否有通读全文的必要。
文中只对论文信息进行浓缩,而不加主观评论或解释,可以脱离原文而独立成篇。
字数通常在100~150个词左右,更确切地说,约为原文长度的1% ~ 5%(有的杂志规定摘要平均为全文的3% ~ 5%)。
现在越来越多的用法是abstract. 尤其是放在索引资料中一律要用abstract 这个术语,在论文的题目下也通常要用这个词。
Summary (概要) 与abstract 无明显差别。
严格地说,summary 一般附在论文的后面,对论文的主要结论和成果进行再叙述。
4个步骤教你如何写好abstract部分
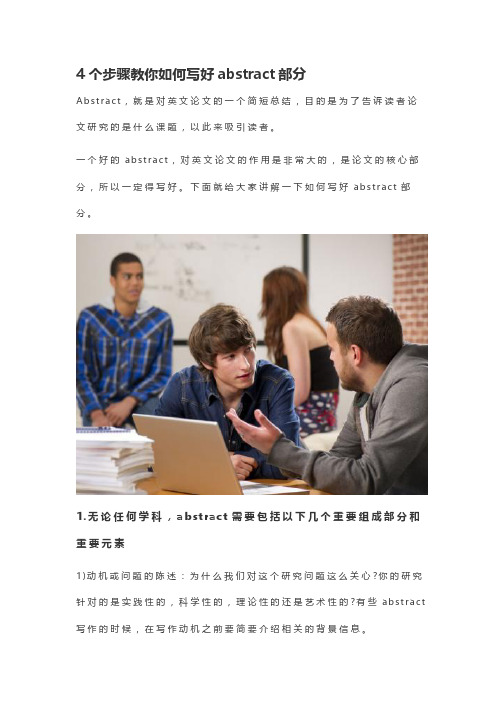
4个步骤教你如何写好abstract部分A b s t r a c t,就是对英文论文的一个简短总结,目的是为了告诉读者论文研究的是什么课题,以此来吸引读者。
一个好的a b s t r a c t,对英文论文的作用是非常大的,是论文的核心部分,所以一定得写好。
下面就给大家讲解一下如何写好a b s t r a c t部分。
1.无论任何学科,a b s t rac t需要包括以下几个重要组成部分和重要元素1)动机或问题的陈述:为什么我们对这个研究问题这么关心?你的研究针对的是实践性的,科学性的,理论性的还是艺术性的?有些a b s t r a c t 写作的时候,在写作动机之前要简要介绍相关的背景信息。
2)措施/流程/解决方法以及调查范围:为了得到相关的结果,你做了什么?3)结果/发现/产品:在上述的调查研究完成后,你学会了什么?发现了什么?创造了什么?4)结论/影响:你得出的哪些结论会产生更大的影响?然而,需要注意的是,根据不同的学科,要注意相关的可变性因素。
2.a b s t rac t中的语法特点和要求1)注意到细微的变化在陈述主要目标和调查范围的时候,多采用陈述性语言。
2)采用合适的方法描述方法的时候多使用过去时态。
要注意时态的一致性。
如果有必要,要按照时间的顺序进行排列。
3)注意结果的呈现方式只需要呈现结果即可。
以过去时态呈现出来。
4)注意结论的可靠性陈述个人观点。
在陈述主要结论的时候要使用现在时。
不要表现出试探性的倾向。
3.a b s t rac t s的类型a b s t r a c t s分为两种类型,i n f o r m a t i o n a l a b s t r a c t s和d e s c r i p t i v ea b s t r a c t s。
I n f o r m a t i o n a l A b s t r a c t s--针对r e p o r t s的相关内容进行沟通。
Abstract写作常用句型及句式
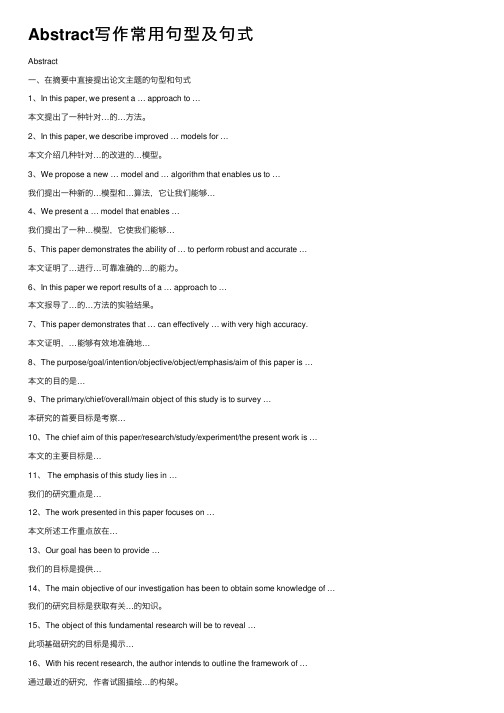
Abstract写作常⽤句型及句式Abstract⼀、在摘要中直接提出论⽂主题的句型和句式1、In this paper, we present a … approach to …本⽂提出了⼀种针对…的…⽅法。
2、In this paper, we describe improved … models for …本⽂介绍⼏种针对…的改进的…模型。
3、We propose a new … model and … algorithm that enables us to …我们提出⼀种新的…模型和…算法,它让我们能够…4、We present a … model that enables …我们提出了⼀种…模型,它使我们能够…5、This paper demonstrates the ability of … to perform robust and accurate …本⽂证明了…进⾏…可靠准确的…的能⼒。
6、In this paper we report results of a … approach to …本⽂报导了…的…⽅法的实验结果。
7、This paper demonstrates that … can effectively … with very high accuracy.本⽂证明,…能够有效地准确地…8、The purpose/goal/intention/objective/object/emphasis/aim of this paper is …本⽂的⽬的是…9、The primary/chief/overall/main object of this study is to survey …本研究的⾸要⽬标是考察…10、The chief aim of this paper/research/study/experiment/the present work is …本⽂的主要⽬标是…11、 The emphasis of this study lies in …我们的研究重点是…12、The work presented in this paper focuses on …本⽂所述⼯作重点放在…13、Our goal has been to provide …我们的⽬标是提供…14、The main objective of our investigation has been to obtain some knowledge of …我们的研究⽬标是获取有关…的知识。
考研英语:词汇abstract的中文翻译解析
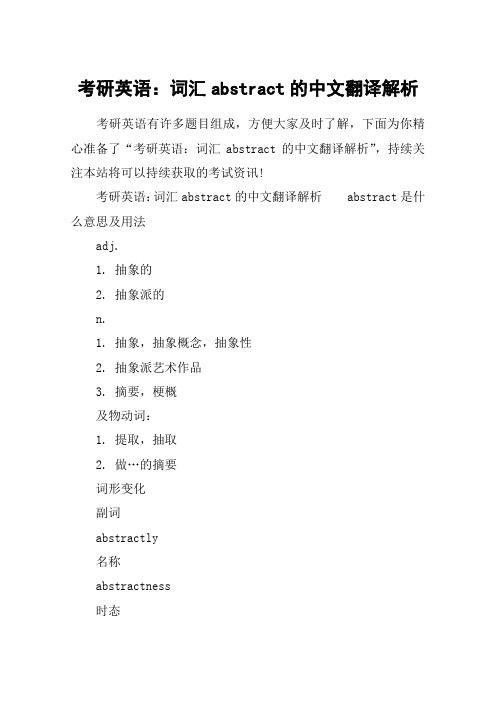
考研英语:词汇abstract的中文翻译解析考研英语有许多题目组成,方便大家及时了解,下面为你精心准备了“考研英语:词汇abstract的中文翻译解析”,持续关注本站将可以持续获取的考试资讯!考研英语:词汇abstract的中文翻译解析abstract是什么意思及用法adj.1. 抽象的2. 抽象派的n.1. 抽象,抽象概念,抽象性2. 抽象派艺术作品3. 摘要,梗概及物动词:1. 提取,抽取2. 做…的摘要词形变化副词abstractly名称abstractness时态abstracted,abstracting,abstracts英语解释not representing or imitating external reality or the objects of naturemake off with belongings of othersdealing with a subject in the abstract without practical purpose or intentionconsider a concept without thinking of a specific example consider abstractly or theoreticallyconsider apart from a particular case or instancegive an abstract (of)a concept or idea not associated with any specific instancea sketchy summary of the main points of an argument or theoryexisting only in the mind separated from embodiment 例句She beheaded me, and flung my head into abstract space 她切下了我的头颅,把它扔进抽象的空间。
学术英语写作Unit-5----Abstract
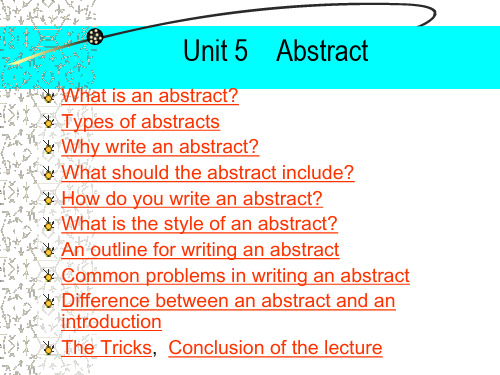
Informative abstraห้องสมุดไป่ตู้ts
An informative abstract provides detail about the substance of a piece of writing because readers will sometimes rely on the abstract alone for information. Informative abstracts typically follow this format:
Unit 5 Abstract
What is an abstract? Types of abstracts Why write an abstract? What should the abstract include? How do you write an abstract? What is the style of an abstract? An outline for writing an abstract Common problems in writing an abstract Difference between an abstract and an introduction The Tricks, Conclusion of the lecture
3. evaluative abstracts: comment on the worth of the original are included.
Difference between descriptive abstracts and informative abstracts
abstract谐音法记忆
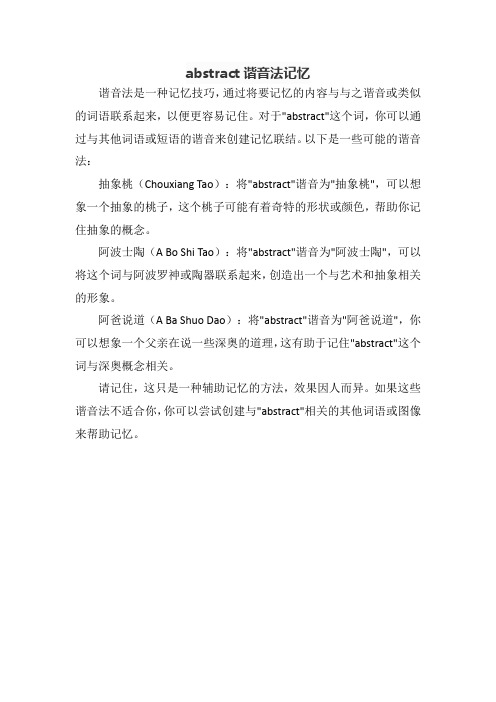
abstract谐音法记忆
谐音法是一种记忆技巧,通过将要记忆的内容与与之谐音或类似的词语联系起来,以便更容易记住。
对于"abstract"这个词,你可以通过与其他词语或短语的谐音来创建记忆联结。
以下是一些可能的谐音法:
抽象桃(Chouxiang Tao):将"abstract"谐音为"抽象桃",可以想象一个抽象的桃子,这个桃子可能有着奇特的形状或颜色,帮助你记住抽象的概念。
阿波士陶(A Bo Shi Tao):将"abstract"谐音为"阿波士陶",可以将这个词与阿波罗神或陶器联系起来,创造出一个与艺术和抽象相关的形象。
阿爸说道(A Ba Shuo Dao):将"abstract"谐音为"阿爸说道",你可以想象一个父亲在说一些深奥的道理,这有助于记住"abstract"这个词与深奥概念相关。
请记住,这只是一种辅助记忆的方法,效果因人而异。
如果这些谐音法不适合你,你可以尝试创建与"abstract"相关的其他词语或图像来帮助记忆。
abstract 的写法
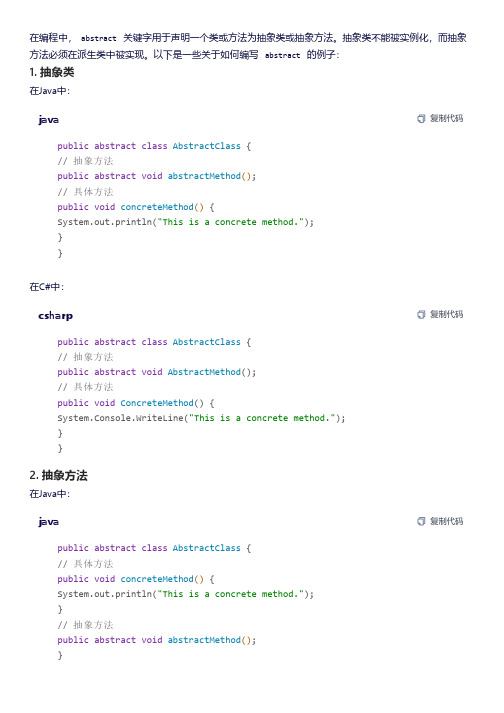
在编程中,abstract关键字用于声明一个类或方法为抽象类或抽象方法。
抽象类不能被实例化,而抽象方法必须在派生类中被实现。
以下是一些关于如何编写abstract的例子:1. 抽象类在Java中:java复制代码public abstract class AbstractClass {// 抽象方法public abstract void abstractMethod();// 具体方法public void concreteMethod() {System.out.println("This is a concrete method.");}}在C#中:csharp复制代码public abstract class AbstractClass {// 抽象方法public abstract void AbstractMethod();// 具体方法public void ConcreteMethod() {System.Console.WriteLine("This is a concrete method.");}}2. 抽象方法在Java中:java复制代码public abstract class AbstractClass {// 具体方法public void concreteMethod() {System.out.println("This is a concrete method.");}// 抽象方法public abstract void abstractMethod();}在C#中:csharp复制代码public abstract class AbstractClass {// 具体方法public void ConcreteMethod() {System.Console.WriteLine("This is a concrete method.");}// 抽象方法public abstract void AbstractMethod();}注意点:抽象类不能被实例化。
abstract 造句
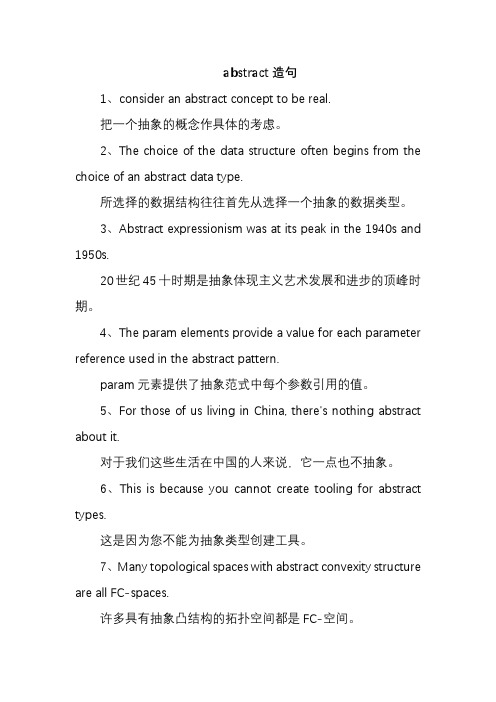
abstract造句1、consider an abstract concept to be real.把一个抽象的概念作具体的考虑。
2、The choice of the data structure often begins from the choice of an abstract data type.所选择的数据结构往往首先从选择一个抽象的数据类型。
3、Abstract expressionism was at its peak in the 1940s and 1950s.20世纪45十时期是抽象体现主义艺术发展和进步的顶峰时期。
4、The param elements provide a value for each parameter reference used in the abstract pattern.param元素提供了抽象范式中每个参数引用的值。
5、For those of us living in China, there's nothing abstract about it.对于我们这些生活在中国的人来说,它一点也不抽象。
6、This is because you cannot create tooling for abstract types.这是因为您不能为抽象类型创建工具。
7、Many topological spaces with abstract convexity structure are all FC-spaces.许多具有抽象凸结构的拓扑空间都是FC-空间。
8、Platonism began the West’s pursuit of abstract truth from the times of ancient Greece.从古希腊时代起,柏拉图主义便开始了西方式的对抽象真理的追求。
9、It used abstract art and often childish language to ridicule the absurdity of the modern world.它使用抽象的艺术和幼稚的语言嘲弄了现代世界的荒谬。
- 1、下载文档前请自行甄别文档内容的完整性,平台不提供额外的编辑、内容补充、找答案等附加服务。
- 2、"仅部分预览"的文档,不可在线预览部分如存在完整性等问题,可反馈申请退款(可完整预览的文档不适用该条件!)。
- 3、如文档侵犯您的权益,请联系客服反馈,我们会尽快为您处理(人工客服工作时间:9:00-18:30)。
Ideal Observers for Detecting Motion: Correspondence NoiseHongjing Lu Department of Psychology,UCLA Los Angeles,CA90095 hongjing@Alan Yuille Department of Statistics,UCLA Los Angeles,CA90095 yuille@AbstractWe derive a Bayesian Ideal Observer(BIO)for detecting motion andsolving the correspondence problem.We obtain Barlow and Tripathy’sclassic model as an approximation.Our psychophysical experimentsshow that the trends of human performance are similar to the BayesianIdeal,but overall human performance is far worse.We investigate waysto degrade the Bayesian Ideal but show that even extreme degradationsdo not approach human performance.Instead we propose that humansperform motion tasks using generic,general purpose,models of motion.We perform more psychophysical experiments which are consistent withhumans using a Slow-and-Smooth model and which rule out an alterna-tive model using Slowness.1IntroductionIdeal Observers give fundamental limits for performing visual tasks(somewhat similar to Shannon’s limits on information transfer).They give benchmarks against which to evaluate human performance.This enables us to determine objectively what visual tasks humans are good at,and may help point the way to underlying neuronal mechanisms.For a recent review,see[1].In an influential paper,Barlow and Tripathy[2]tested the ability of human subjects to detect dots moving coherently in a background of random dots.They derived an“ideal observer”model using techniques from Signal Detection theory[3].They showed that their model predicted the trends of the human performance as properties of the stimuli changed,but that humans performed far worse than their model.They argued that degrading their model, by lowering the spatial resolution,would give predictions closer to human performance. Barlow and Tripathy’s model has generated considerable interest,see[4,5,6,7].We formulate this motion problem in terms of Bayesian Decision Theory and derive a Bayesian Ideal Observer(BIO)model.We describe why Barlow and Tripathy’s(BT)model is not fully ideal,show that it can be obtained as an approximation to the BIO,and deter-mine conditions under which it is a good approximation.We perform psychophysical ex-periments under a range of conditions and show that the trends of human subjects are more similar to those of the BIO.We investigate whether degrading the Bayesian Ideal enables us to reach human performance,and conclude that it does not(without implausibly largedeformations).We comment that Barlow and Tripathy’s degradation model is implausible due to the nature of the approximations used.Instead we show that a generic motion detection model which uses a slow-and-smooth assumption about the motion field [8,9]gives similar performance to human subjects under a range of experimental conditions.A simpler approach using a slowness assumption alone does not match new experimental data that we present.We conclude that human observers are not ideal,in the sense that they do not perform inference using the model that the experimenter has chosen to generate the data,but may instead use a general purpose model perhaps adapted to the motion statistics of natural images.2Bayes Decision Theory and Ideal ObserversWe now give the basic elements of Bayes Decision Theory.The input data is D and we seek to estimate a binary state W (e.g.coherent or incoherent motion,horizon-tal motion to right or to left).We assume models P (D |W )and P (W ).We define a decision rule α(D )and a loss function L (α(I ),W )=1−δα(D ),W .The risk is R (α)= D,W L (α(D ),W )P (D |W )P (W ).Optimal performance is given by the Bayes rule:α∗=arg min R (α).The fundamental limits are given by Bayes Risk:R ∗=R (α∗).Bayes risk is the best performance that can be achieved.It corresponds to ideal performance.Barlow and Tripathy’s (BT)model does not achieve Bayes risk.This is because they used simplification to derive it using concepts from Signal Detection theory (SDT).SDT is es-sentially the application of Bayes Decision Theory to the task of signal detection but,for historical reasons,SDT restricts itself to a limited class of probability models and is unable to capture the complexity of the motion problem.3Experimental Setup and Correspondence NoiseWe now give the details of Barlow and Tripathy’s stimuli,their model,and their experi-ments.The stimuli consist of two image frames with N dots in each frame.The dots in the first frame are at random positions.For coherent stimuli,see figure (1),a proportion CN of dots move coherently left or right horizontally with a fixed translation motion with dis-placement T .The remaining N (1−C )dots in the second frame are generated at random.For incoherent stimuli,the dots in both frames are generated at random.Estimating motion for these stimuli requires solving the correspondence problem to match dots between frames.For coherent motion,the noise dots act as correspondence noise and make the matching harder,see the rightmost panel in figure (1).Barlow and Tripathy perform two types of binary forced choice experiments.In detection experiments ,the task is to determine whether the stimuli is coherent or incoherent motion.For discrimination experiments ,the goal is to determine if the motion is to the right or the left.The experiments are performed by adjusting the fraction C of coherently moving dots until the human subject’s performance is at threshold (i.e.75percent correct).Barlow and Tripathy’s (BT)model gives the proportion of dots at threshold to be C θ=1/√Q −N where Q is the size of the image lattice.This is approximately 1/√Q (because N <<Q )and so is independent of the density of dots.Barlow and Tripathy compare the thresholds of the human subjects with those of their model for a range of experimental conditions which we will discuss in later sections.Figure1:The left three panels show coherent stimuli with N=20,C=0.1,N=20,C= 0.5and N=20,C=1.0respectively.The closed and open circles denote dots in thefirst and second frame respectively.The arrows show the motion of those dots which are moving coherently.Correspondence noise is illustrated by the far right panel showing that a dot in thefirst frame has many candidate matches in the second frame.4The Bayesian Ideal ModelWe now compute the Bayes rule and Bayes risk by taking into account exactly how the data is generated.We denote the dot positions in thefirst and second frame by D={x i:i= 1,...,N},{y a:a=1,...,N}.We define correspondence variables V ia:V ia=1if x i→y a,V ia=0otherwise.The generative model for the data is given by:P(D|Coh,T)= V ia P({y a}|{x i},{V ia},T)P({V ia})P({x i})coherent,P(D|Incoh)=P({y a})P({x i}),incoherent.(1) The prior distributions for the dot positions P({x i}),P({y a})allow all configurations ofthe dots to be equally likely.They are therefore of form P({x i})=P({y a})=(Q−N)!Q! where Q is the number of lattice points.The model P({y a}|{x i},{V ia},T)for coher-ent motion is P({y a}|{x i},{V ia},T)=(Q−N)!(Q−CN)! ia(δy a,x i+T)V ia.We set the priors P({V ia}to be the uniform distribution.There is a constraint ia V ia=CN(since only CN dots move coherently).This gives:P(D|Incoh)=(Q−N)!Q!(Q−N)!Q!,P(D|Coh,T)={(N−CN)!(N)!(N−CN)!(N)!}2(CN)!V iaia(δya+T,x i)V ia.These can be simplified further by observing that V ia ia(δy a,x i+T)V ia=Ψ!(Ψ−M)!M!,whereΨis the total number of matches–i.e.the number of dots in thefirst frame that have a corresponding dot at displacement T in the second frame(this includes“fake”matches due to change alignment of noise dots in the two frames).The Bayes rule for performing the tasks are given by testing the log-likelihood ratios:(i)log P(D|Incoh)P(D|Coh,T)for detection(i.e.coherent versus incoherent),and(ii)log P(D|Coh,−T)P(D|Coh,T)fordiscrimination(i.e.motion to right or to left).For detection,the log-likelihood ratio is a function ofΨ.For discrimination,the log-likelihood ratio is a function of the number of matches to the rightΨr and to the leftΨl.It is straightforward to calculate the Bayes risk and determine coherence thresholds.We can rederive Barlow and Tripathy’s model as an approximation to the Bayesian Ideal.They make two approximations:(i)they model the distribution ofψas Binomial,(ii)theyuse d′.Both approximations are very good near threshold,except for small N.The use ofd′can be justified if P(Ψ|Coh,T)and P(Ψ|Incoh)are Gaussians with similar variance. This is true for large N=1000and a range of C but not so good for small N=100,seefigure(2).Figure2:We plot P(Ψ|Coh,T)and P(Ψ|Incoh),shown as P(Ψ|C)and P(Ψ|N)re-spectively,for a range of N and C.One of Barlow and Tripathy’s two approximations arejustified if the distributions are Gaussian with the same variance.This is true for large N(left two panels)but fails for small N(right two panels).Note that human thresholds areroughly30times higher than for BIO(the scales on graphs differ).We computed the coherence threshold for the BIO and the BT models for N=100to N=1000,see the second and fourth panels infigure(3).As described earlier,the BT threshold is approximately independent of the number N of dots.Our computations showed that the BIO threshold is also roughly constant except for small N(this is not surprising in light of figure(2).This motivated psychophysics experiments to determine how humans performed for small N(this range of dots was not explored in Barlow and Tripathy’s experiments). All our data points are from300trials using QUEST,so errors bars are so small that we do not include them.We performed the detection and discrimination tasks with translation motion T=16(asin Barlow and Tripathy).For detection and discrimation,the human subject’s thresholdsshowed similar trends to the thresholds for BIO and BT.But human performance at smallN are more consistent with BIO,seefigure(3).Figure3:The left two panels show detection thresholds–human subjects(far left)and BIOand BT thresholds(left).The right two panels show discrimination thresholds–humansubjects(right)and BIO and BT(far right).But probably the most striking aspect offigure(3)is how poorly humans perform comparedto the models.The thresholds for BIO are always higher than those for BT,but thesedifferences are almost negligible compared to the differences with the human subjects.Theexperiments also show that the human subject trends differ from the models at large N.But these are extreme conditions where there are dots on most points on the image lattice.5Degradating the Ideal Observer ModelsWe now degrade the Bayes Ideal model to see if we can obtain human performance.We consider two mechanisms:(A)Humans do not know the precise value of the motion transla-tion T .(B)Humans have poor spatial uncertainty.We will also combine both mechanisms.For (A),we model lack of knowledge of the velocity T by summing over different motions.We generate the stimuli as before from P (D |Incoh)or P (D |Coh ,T ),but we make the decision by thresholding:logTP (D |Coh ,T )P (T )P (D |Incoh).For (B),we model lack of spatial resolution by replacing P ({y a }|{x i },{V ia },T )=(Q −N )!(Q −CN )! ia V ia δy a ,x i +t by P ({y a }|{x i },{V ia },T )=(Q −N )!(Q −CN )!ia V ia f W (y a ,x i +t ).Here W is the width of a spatial window,so that f W (a,b )=1/W 2,if |a −b |<W ;f W (a,b )=0,otherwise .Our calculations,see figure (4),show that neither (A)nor (B)not their combination are sufficient to account for the poor performance of human ck of knowledge of the correct motion (and consequently summing over several models)does little to de-grade performance.Decreasing spatial resolution does degrade performance but even huge degradations are insufficient to reach human levels.Barlow and Tripathy [2]argue that they can degrade their model to reach human performance but the degradations are huge and they occur in conditions (e.g.N =50or N =100)where their model is not a good approximation to the true Bayesian Ideal Observer.Figure 4:Comparing the degraded models to human performance.We use a log-log plot because the differences between humans and model thresholds is very large.6Slowness and Slow-and-SmoothWe now consider an alternative explanation for why human performance differs so greatly from the Bayesian Ideal Observer.Perhaps human subjects do not use the ideal model (which is only known to the designer of the experiments)and instead use a general purpose motion model.We now consider two possible models:(i)a slowness model,and (ii)a slow and smooth model.Figure 5:The coherence threshold as a function of N for different translation motions T .From left to right,human subject (HL),human subject (RK),2DNN (shown for T =16only),and 1DNN.In the two right panels we have drawn the average human performance for comparision.The slowness model is partly motivated by Ullman’s minimal mapping theory[10]and partly by the design of practical computer vision tracking systems.This model solves the correspondence problem by simply matching a dot in thefirst frame to the closest dot in the second frame.We consider a2D nearest neighbour model(2DNN)and a1D nearest neighbour model(1DNN),for which the matching is constrained to be in horizontal directions only.After the motion has been calculated we perform a log-likelihood test to solve the discrimination and detection tasks.This enables us to calculate coherence thresholds,seefigure(5).Both1DNN and2DNN predict that correspondence will be easy for small translation motions even when the number of dots is very large.This motivates a new class of experiments where we vary the translation motion.Our experiments show that1DNN and2DNN are poorfits to human performance.Human performance thresholds are relatively insensitive to the number N of dots and the trans-lation motion T,see the two left panels infigure(5).By contrast,the1DNN and2DNN thresholds are either far lower than humans for small N or far higher at large N with a transition that depends on T.We conclude that the1DNN and2DNN models do not match human performance.Figure6:The motionflows from Slow-and-Smooth for N=100as functions of C and T.From left to right,C=0.1,C=0.2,C=0.3,C=0.5.From top to bottom, T=4,T=8,T=16.The closed and open circles denote dots in thefirst and second frame respectively.The arrows indicate the motionflow specified by the Slow-and-Smooth model.We now consider the Slow-and-Smooth model[8,9]which has been shown to account for a range of motion phenomena.We use a formulation[8]that was specifically designed for dealing with the correspondence problem.This gives a model of form P(V,v|{x i},{y a})=(1/Z)e−E[V,v]/T m,whereE[V,v]=Ni=1Na=1V ia(y a−x i−v(x i))2+λ||Lv||2+ζNi=1V i0,(2)L is an operator that penalizes slow-and-smooth motion and depends on a paramtersσ,see Yuille and Grzywacz for details[8].We impose the constraint that N i=a V ia=1,∀i, which enforces that each point i in thefirst frame is either unmatched,if V i0=1,or is matched to a point a in the second frame.We implemented this model using an EM algorithm to estimate the motionfield v(x)that maximizes P(v|{x i},{y a})= V P(V,v|{x i},{y a}).The parameter settings are T m= 0.001,λ=0.5,ζ=0.01,σ=0.2236.(The size of the units of length are normalized by the size of the image).The size ofσdetermines the spatial scale of the interaction between dots[8].This parameter settings estimate correct motion directions in the condition that all dots move coherently,C=1.0.The following results,seefigure(6),show that for100dots(N=100)the results of the slow-and-smooth model are similar to those of the human subjects for a range of different translation motions.Slow-and-Smooth starts giving coherence thresholds between C=0.2 and C=0.3consistent with human performance.Lower thresholds occurred for slower coherent translations in agreement with human performance.Slow-and-Smooth also gives thresholds similar to human performance when we alter the number N of dots,seefigure(7).Once again,Slow-and-Smooth starts giving the correct horizontal motion between c=0.2and c=0.3.Figure7:The motionfields of Slow-and-Smooth for T=16as a function of c and N. From left to right,C=0.1,C=0.2,C=0.3,C=0.5.From top to bottom,N= 50,N=100,N=1000.Same conventions as for previousfigure.7SummaryWe defined a Bayes Ideal Observer(BIO)for correspondence noise and showed that Bar-low and Tripathy’s(BT)model[2]can be obtained as an approximation.We performed psychophysical experiments which showed that the trends of human performance were more similar to those of BIO(when it differed from BT).We attempted to account for human’s poor performance(compared to BIO)by allowing for degradations of the model such as poor spatial resolution and uncertainty about the precise translation velocity.We concluded that these degradation had to be implausibly large to account for the poorness of human performance.We noted that Barlow and Tripathy’s degradation model[2]takes them into a regime where their model is a bad approximation to the BIO.Instead,we in-vestigated the possibility that human observers perform these motion tasks using generic probability models for motion possibly adapted to the statistics of motion in the natural world.Further psychophysical experiments showed that human performance was inconsis-tent with a model than prefers slow motion.But human performance was consistent with the Slow-and-Smooth model[8,9].We conclude with two metapoints.Firstly,it is possible to design ideal observer models for complex stimuli using techniques from Bayes decision theory.There is no need to restrict oneself to the traditional models described in classic signal detection books such as Green and Swets[3].Secondly,human performance at visual tasks may be based on generic models,such as Slow-and-Smooth,rather than the ideal models for the experimental tasks (known only to the experimenter).AcknowledgementsWe thank Zili Liu for helpful discussions.We gratefully acknowledge funding support from the American Association of University Women(HL),NSF0413214and W.M.Keck Foundation(ALY). References[1]Geisler,W.S.(2002)“Ideal Observer Analysis”.In L.Chalupa and J.Werner(Eds).The Visual Neuroscienes.Boston.MIT Press.825-837.[2]Barlow,H.,and Tripathy,S.P.(1997)Correspondence noise and signal pooling in the detection of coherent visual motion.Journal of Neuroscience,17(20),7954-7966.[3]Green,D.M.,and Swets,J.A.(1966)Signal detection theory and psychophysics.New York: Wiley.[4]Morrone,M.C.,Burr,D.C.,and Vaina,L.M.(1995)Two stages of visual processing for radial and circular motion.Nature,376(6540),507-509.[5]Neri,P.,Morrone,M.C.,and Burr,D.C.(1998)Seeing biological motion.Nature,395(6705), 894-896.[6]Song,Y.,and Perona,P.(2000)A computational model for motion detection and direction dis-crimination in humans.IEEE computer society workshop on Human Motion,Austin,Texas.[7]Wallace,J.M and Mamassian,P.(2004)The efficiency of depth discrimination for non-transparent and transparent stereoscopic surfaces.Vision Research,44,2253-2267.[8]Yuille,A.L.and Grzywacz,N.M.(1988)A computational theory for the perception of coherent visual motion.Nature,333,71-74,[9]Weiss,Y.,and Adelson,E.H.(1998)Slow and smooth:A Bayesian theory for the combination of local motion signals in human vision Technical Report1624.Massachusetts Institute of Technology.[10]Ullman,S.(1979)The interpretation of Visual Motion.MIT Press,Cambridge,MA,1979.。