basic of information retrieval
Gravitation-Based Model for Information Retrieval

ABSTRACT
This paper proposes GBM (gravitation-based model), a physical model for information retrieval inspired by Newton’s theory of gravitation. A mapping is built in this model from concepts of information retrieval (documents, queries, relevance, etc) to those of physics (mass, distance, radius, attractive force, etc). This model actually provides a new perspective on IR problems. A family of effective term weighting functions can be derived from it, including the well-known BM25 formula. This model has some advantages over most existing ones: First, because it is directly based on basic physical laws, the derived formulas and algorithms can have their explicit physical interpretation. Second, the ranking formulas derived from this model satisfy more intuitive heuristics than most of existing ones, thus have the potential to behave empirically better and to be used safely on various settings. Finally, a new approach for structured document retrieval derived from this model is more reasonable and behaves better than existing ones.
信息检索的基本概念 信息检索的基本流程
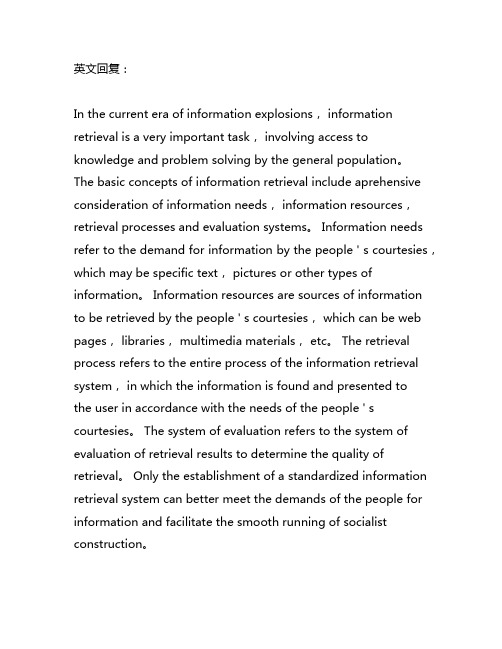
英文回复:In the current era of information explosions, information retrieval is a very important task, involving access to knowledge and problem solving by the general population。
The basic concepts of information retrieval include aprehensive consideration of information needs, information resources,retrieval processes and evaluation systems。
Information needs refer to the demand for information by the people ' s courtesies,which may be specific text, pictures or other types of information。
Information resources are sources of information to be retrieved by the people ' s courtesies, which can be web pages, libraries, multimedia materials, etc。
The retrieval process refers to the entire process of the information retrieval system, in which the information is found and presented tothe user in accordance with the needs of the people ' s courtesies。
我国高校图书馆信息素养教育现状分析
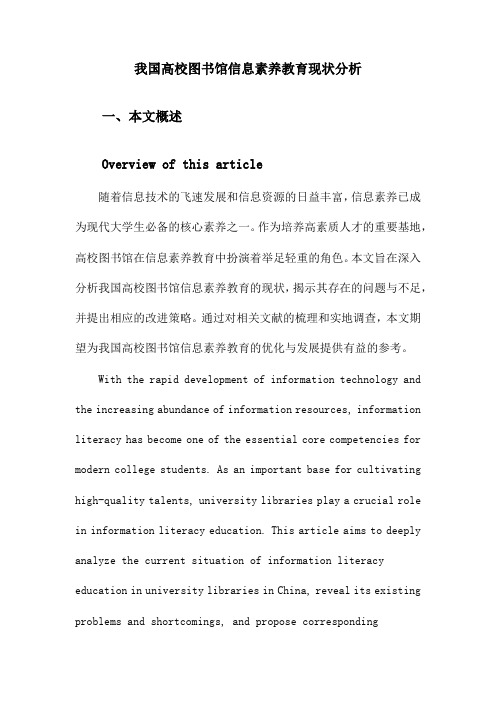
我国高校图书馆信息素养教育现状分析一、本文概述Overview of this article随着信息技术的飞速发展和信息资源的日益丰富,信息素养已成为现代大学生必备的核心素养之一。
作为培养高素质人才的重要基地,高校图书馆在信息素养教育中扮演着举足轻重的角色。
本文旨在深入分析我国高校图书馆信息素养教育的现状,揭示其存在的问题与不足,并提出相应的改进策略。
通过对相关文献的梳理和实地调查,本文期望为我国高校图书馆信息素养教育的优化与发展提供有益的参考。
With the rapid development of information technology and the increasing abundance of information resources, information literacy has become one of the essential core competencies for modern college students. As an important base for cultivating high-quality talents, university libraries play a crucial role in information literacy education. This article aims to deeply analyze the current situation of information literacy education in university libraries in China, reveal its existing problems and shortcomings, and propose correspondingimprovement strategies. Through reviewing relevant literature and conducting field investigations, this article aims to provide useful references for the optimization and development of information literacy education in Chinese university libraries.本文将对信息素养及信息素养教育的概念进行界定,明确其内涵与外延。
EBSCO数据库检索
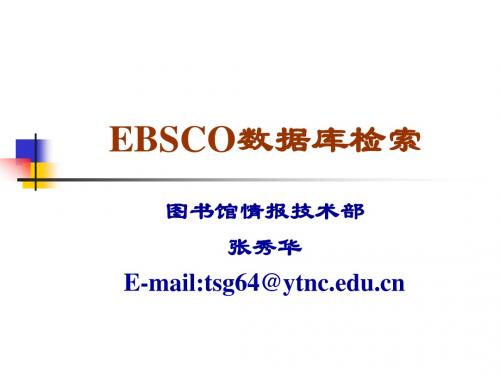
Regional Business News (RBN)
提供覆盖地区性商业出版物的全文,集 成美国所有城市的75种期刊、报纸和新 闻专线 数据库每日更新
World Magazine Bank (WMB)
“世界杂志库” 该库提供包括澳大利亚、新西兰、亚洲、 英国、南非、美国等地出版的250多种英 文期刊全文。
检索结果
收藏夹:数据库检索系统中有一个临时的个人收 藏夹。在一次检索的过程当中,检索者可随时将 需要进一步处理的文章存入收藏夹中,以便检索 完成后集中处理。
收藏夹
在检索结果页面,使用“add”可将选中记录加到收 藏夹。加入完成后,收藏夹显示“Folder has Items”,点 击,可显示所有加入到收藏夹的文献记录。
Academic Search Premier ( ASP)
“学术期刊数据库” 全球最大的多学科学术期刊全文数据库之一。 提供了7876种期刊的文摘和索引;3990种学术期刊的全文 (其中3100多种经同行评议);100多种全文期刊回溯到 1975年或更早。SCI & SSCI收录的核心期刊为993种(全文 有350种)。 几乎覆盖了所有的学术研究领域,包括:社会科学、人文科 学、教育学、语言学、艺术、文学、历史学、法律、军事、 心理学、哲学、工商经济、工程技术、计算机科学、物理学、 化学、医学、生物等。 所提供的许多文献为该数据库所独有,无法在其它数据库中 获得。 资料每日更新。
检索结果
点击检索结果—引文信息
打开PDF全文
全文情况
全文的格式可能有:HTML、XML、 PDF。 有“Linked Full Text”图标时,说明这篇 文章在其它EBSCO 数据库(指当前检索 者拥有使用权的数据库)中有全文。 要浏览PDF 格式的全文,需事先安装 Acrobat Reader 等PDF 浏览器。
APS计算机审核
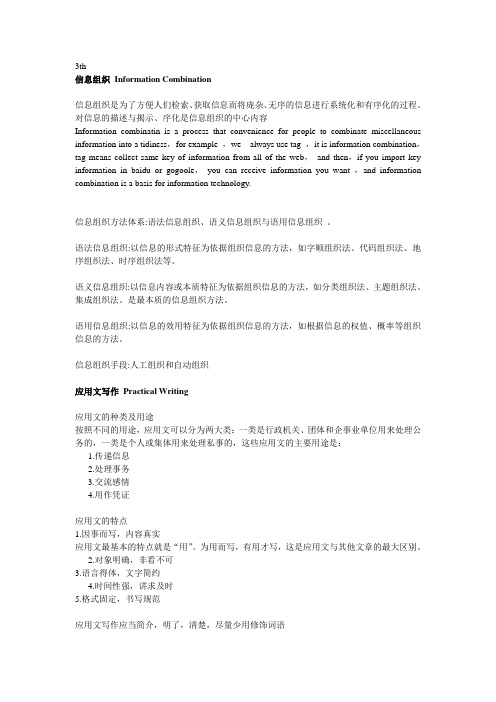
信息组织Information Combination信息组织是为了方便人们检索、获取信息而将庞杂、无序的信息进行系统化和有序化的过程。
对信息的描述与揭示、序化是信息组织的中心内容Information combinatin is a process that convenience for people to combinate miscellaneous information into a tidiness,for example ,we always use tag ,it is information combination,tag means collect same key of information from all of the web,and then,if you import key information in baidu or gogoole,you can receive information you want ,and information combination is a basis for information technology.信息组织方法体系:语法信息组织、语义信息组织与语用信息组织。
语法信息组织:以信息的形式特征为依据组织信息的方法,如字顺组织法、代码组织法、地序组织法、时序组织法等。
语义信息组织:以信息内容或本质特征为依据组织信息的方法,如分类组织法、主题组织法、集成组织法。
是最本质的信息组织方法。
语用信息组织:以信息的效用特征为依据组织信息的方法,如根据信息的权值、概率等组织信息的方法。
信息组织手段:人工组织和自动组织应用文写作Practical Writing应用文的种类及用途按照不同的用途,应用文可以分为两大类:一类是行政机关、团体和企事业单位用来处理公务的,一类是个人或集体用来处理私事的,这些应用文的主要用途是:1.传递信息2.处理事务3.交流感情4.用作凭证应用文的特点1.因事而写,内容真实应用文最基本的特点就是“用”。
Introduction to Information Retrieval
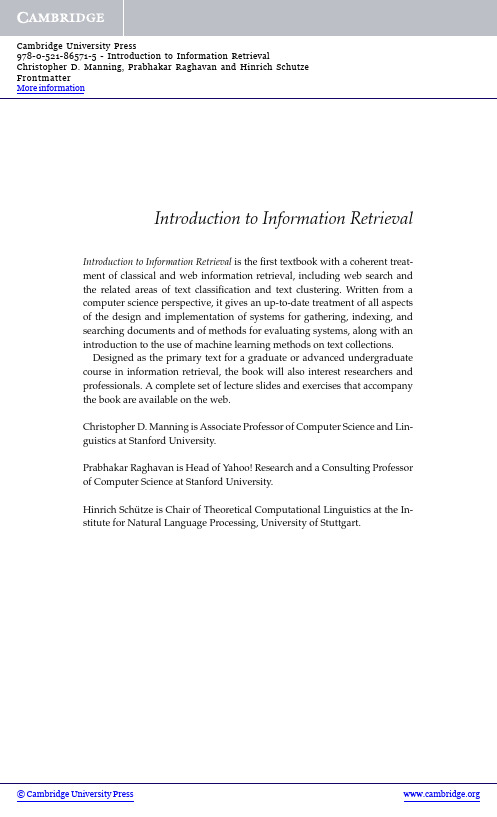
More informationIntroduction to Information Retrieval Introduction to Information Retrieval is thefirst textbook with a coherent treat-ment of classical and web information retrieval,including web search andthe related areas of text classification and text clustering.Written from acomputer science perspective,it gives an up-to-date treatment of all aspectsof the design and implementation of systems for gathering,indexing,andsearching documents and of methods for evaluating systems,along with anintroduction to the use of machine learning methods on text collections.Designed as the primary text for a graduate or advanced undergraduatecourse in information retrieval,the book will also interest researchers andprofessionals.A complete set of lecture slides and exercises that accompanythe book are available on the web.Christopher D.Manning is Associate Professor of Computer Science and Lin-guistics at Stanford University.Prabhakar Raghavan is Head of Yahoo!Research and a Consulting Professorof Computer Science at Stanford University.Hinrich Sch¨utze is Chair of Theoretical Computational Linguistics at the In-stitute for Natural Language Processing,University of Stuttgart.IntroductiontoInformation RetrievalChristopher D.ManningStanford UniversityPrabhakar RaghavanYahoo!ResearchHinrich Sch ¨utzeUniversity ofStuttgartMore informationMore informationcambridge university pressCambridge,New York,Melbourne,Madrid,Cape Town,Singapore,S˜a o Paulo,DelhiCambridge University Press32Avenue of the Americas,New York,NY10013-2473,USAInformation on this title:/9780521865715C Cambridge University Press2008This publication is in copyright.Subject to statutory exceptionand to the provisions of relevant collective licensing agreements,no reproduction of any part may take place withoutthe written permission of Cambridge University Press.First published2008Printed in the United States of AmericaA catalog record for this publication is available from the British Library.Library of Congress Cataloging in Publication dataManning,Christopher D.Introduction to information retrieval/Christopher D.Manning,PrabhakarRaghavan,Hinrich Sch¨utze.p.cm.Includes bibliographical references and index.ISBN978-0-521-86571-5(hardback)1.Text processing(Computer science)rmation retrieval.3.Documentclustering. 4.Semantic Web.I.Raghavan,Prabhakar.II.Sch¨utze,Hinrich.III.Title.QA76.9.T48M262008025.04–dc222008001257ISBN978-0-521-86571-5hardbackCambridge University Press has no responsibility forthe persistence or accuracy of URLs for external orthird-party Internet Web sites referred to in this publicationand does not guarantee that any content on suchWeb sites is,or will remain,accurate or appropriate.More informationContentsTable of Notation page xiPreface xv1Boolean retrieval11.1An example information retrieval problem31.2Afirst take at building an inverted index61.3Processing Boolean queries91.4The extended Boolean model versus ranked retrieval131.5References and further reading162The term vocabulary and postings lists182.1Document delineation and character sequence decoding182.2Determining the vocabulary of terms212.3Faster postings list intersection via skip pointers332.4Positional postings and phrase queries362.5References and further reading433Dictionaries and tolerant retrieval453.1Search structures for dictionaries453.2Wildcard queries483.3Spelling correction523.4Phonetic correction583.5References and further reading594Index construction614.1Hardware basics624.2Blocked sort-based indexing634.3Single-pass in-memory indexing664.4Distributed indexing684.5Dynamic indexing71vMore informationvi Contents4.6Other types of indexes734.7References and further reading765Index compression785.1Statistical properties of terms in information retrieval795.2Dictionary compression825.3Postingsfile compression875.4References and further reading976Scoring,term weighting,and the vector space model1006.1Parametric and zone indexes1016.2Term frequency and weighting1076.3The vector space model for scoring1106.4Variant tf–idf functions1166.5References and further reading1227Computing scores in a complete search system1247.1Efficient scoring and ranking1247.2Components of an information retrieval system1327.3Vector space scoring and query operator interaction1367.4References and further reading1378Evaluation in information retrieval1398.1Information retrieval system evaluation1408.2Standard test collections1418.3Evaluation of unranked retrieval sets1428.4Evaluation of ranked retrieval results1458.5Assessing relevance1518.6A broader perspective:System quality and userutility1548.7Results snippets1578.8References and further reading1599Relevance feedback and query expansion1629.1Relevance feedback and pseudo relevancefeedback1639.2Global methods for query reformulation1739.3References and further reading17710XML retrieval17810.1Basic XML concepts18010.2Challenges in XML retrieval18310.3A vector space model for XML retrieval18810.4Evaluation of XML retrieval192More informationContents vii10.5Text-centric versus data-centric XML retrieval19610.6References and further reading19811Probabilistic information retrieval20111.1Review of basic probability theory20211.2The probability ranking principle20311.3The binary independence model20411.4An appraisal and some extensions21211.5References and further reading21612Language models for information retrieval21812.1Language models21812.2The query likelihood model22312.3Language modeling versus other approachesin information retrieval22912.4Extended language modeling approaches23012.5References and further reading23213Text classification and Naive Bayes23413.1The text classification problem23713.2Naive Bayes text classification23813.3The Bernoulli model24313.4Properties of Naive Bayes24513.5Feature selection25113.6Evaluation of text classification25813.7References and further reading26414Vector space classification26614.1Document representations and measures of relatednessin vector spaces26714.2Rocchio classification26914.3k nearest neighbor27314.4Linear versus nonlinear classifiers27714.5Classification with more than two classes28114.6The bias–variance tradeoff28414.7References and further reading29115Support vector machines and machine learning on documents29315.1Support vector machines:The linearly separable case29415.2Extensions to the support vector machine model30015.3Issues in the classification of text documents30715.4Machine-learning methods in ad hoc information retrieval31415.5References and further reading318More informationviii Contents16Flat clustering32116.1Clustering in information retrieval32216.2Problem statement32616.3Evaluation of clustering32716.4K-means33116.5Model-based clustering33816.6References and further reading34317Hierarchical clustering34617.1Hierarchical agglomerative clustering34717.2Single-link and complete-link clustering35017.3Group-average agglomerative clustering35617.4Centroid clustering35817.5Optimality of hierarchical agglomerativeclustering36017.6Divisive clustering36217.7Cluster labeling36317.8Implementation notes36517.9References and further reading36718Matrix decompositions and latent semantic indexing36918.1Linear algebra review36918.2Term–document matrices and singular valuedecompositions37318.3Low-rank approximations37618.4Latent semantic indexing37818.5References and further reading38319Web search basics38519.1Background and history38519.2Web characteristics38719.3Advertising as the economic model39219.4The search user experience39519.5Index size and estimation39619.6Near-duplicates and shingling40019.7References and further reading40420Web crawling and indexes40520.1Overview40520.2Crawling40620.3Distributing indexes41520.4Connectivity servers41620.5References and further reading419More informationContents ix21Link analysis42121.1The Web as a graph42221.2PageRank42421.3Hubs and authorities43321.4References and further reading439Bibliography441Index469More informationTable of NotationSymbol Page Meaningγ90γcodeγ237Classification or clustering function:γ(d)is d’s classor cluster237Supervised learning method in Chapters13and14:(D)is the classification functionγlearned fromtraining set Dλ370Eigenvalueµ(.)269Centroid of a class(in Rocchio classification)or acluster(in K-means and centroid clustering)105Training exampleσ374Singular value(·)10A tight bound on the complexity of an algorithmω,ωk328Cluster in clustering328Clustering or set of clusters{ω1,...,ωK}arg maxf(x)164The value of x for which f reaches its maximumxarg minf(x)164The value of x for which f reaches its minimumxc,c j237Class or category in classificationcf t82The collection frequency of term t(the total numberof times the term appears in the document collection) C237Set{c1,...,c J}of all classesC248A random variable that takes as values members ofCC369Term–document matrixd4Index of the d th document in the collection Dd65A documentd, q163Document vector,query vectorD326Set{d1,...,d N}of all documentsD c269Set of documents that is in class cD237Set{ d1,c1 ,..., d N,c N }of all labeled documents inChapters13–15xiMore informationxii Table of Notationdf t108The document frequency of term t(the total numberof documents in the collection the term appears in) H91EntropyH M93M th harmonic numberI(X;Y)252Mutual information of random variables X and Yidf t108Inverse document frequency of term tJ237Number of classesk267Top k items from a set,e.g.,k nearest neighbors inkNN,top k retrieved documents,top k selected fea-tures from the vocabulary Vk50Sequence of k charactersK326Number of clustersL d214Length of document d(in tokens)L a242Length of the test document(or application docu-ment)in tokensL ave64Average length of a document(in tokens)M4Size of the vocabulary(|V|)M a242Size of the vocabulary of the test document(or appli-cation document)M ave71Average size of the vocabulary in a document in thecollectionM d218Language model for document dN4Number of documents in the retrieval or training col-lectionN c240Number of documents in class cN(ω)275Number of times the eventωoccurredO(·)10A bound on the complexity of an algorithmO(·)203The odds of an eventP142PrecisionP(·)202ProbabilityP425Transition probability matrixq55A queryR143Recalls i53A strings i103Boolean values for zone scoringsim(d1,d2)111Similarity score for documents d1,d2T40Total number of tokens in the document collectionT ct240Number of occurrences of word t in documents ofclass ct4Index of the t th term in the vocabulary Vt56A term in the vocabularytf t,d107The term frequency of term t in document d(the totalnumber of occurrences of t in d)More informationTable of Notation xiiiU t246Random variable taking values0(term t is present)and1(t is not present)V190Vocabulary of terms{t1,...,t M}in a collection(a.k.a.the lexicon)v(d)111Length-normalized document vectorV(d)110Vector of document d,not length normalizedwf t,d115Weight of term t in document dw103A weight,for example,for zones or termsw T x=b269Hyperplane; w is the normal vector of the hyperplaneand w i component i of wx204Term incidence vector x=(x1,...,x M);more gener-ally:document feature representationX246Random variable taking values in V,the vocabulary(e.g.,at a given position k in a document)X237Document space in text classification|A|56Set cardinality:the number of members of set A|S|370Determinant of the square matrix S|s i|53Length in characters of string s i| x|128Length of vector x| x− y|121Euclidean distance of x and y(which is the length of( x− y))More informationPrefaceAs recently as the1990s,studies showed that most people preferred gettinginformation from other people rather than from information retrieval(IR)systems.Of course,in that time period,most people also used human travelagents to book their travel.However,during the last decade,relentless opti-mization of information retrieval effectiveness has driven web search enginesto new quality levels at which most people are satisfied most of the time,andweb search has become a standard and often preferred source of informationfinding.For example,the2004Pew Internet Survey(Fallows2004)foundthat“92%of Internet users say the Internet is a good place to go for gettingeveryday information.”To the surprise of many,thefield of information re-trieval has moved from being a primarily academic discipline to being thebasis underlying most people’s preferred means of information access.Thisbook presents the scientific underpinnings of thisfield,at a level accessibleto graduate students as well as advanced undergraduates.Information retrieval did not begin with the Web.In response to variouschallenges of providing information access,thefield of IR evolved to giveprincipled approaches to searching various forms of content.Thefield be-gan with scientific publications and library records but soon spread to otherforms of content,particularly those of information professionals,such asjournalists,lawyers,and doctors.Much of the scientific research on IR hasoccurred in these contexts,and much of the continued practice of IR dealswith providing access to unstructured information in various corporate andgovernmental domains,and this work forms much of the foundation of ourbook.Nevertheless,in recent years,a principal driver of innovation has been theWorld Wide Web,unleashing publication at the scale of tens of millions ofcontent creators.This explosion of published information would be moot ifthe information could not be found,annotated,and analyzed so that eachuser can quicklyfind information that is both relevant and comprehensivefor their needs.By the late1990s,many people felt that continuing to in-dex the whole Web would rapidly become impossible,due to the Web’sxvMore informationxvi Prefaceexponential growth in size.But major scientific innovations,superb engi-neering,the rapidly declining price of computer hardware,and the rise ofa commercial underpinning for web search have all conspired to power to-day’s major search engines,which are able to provide high-quality resultswithin subsecond response times for hundreds of millions of searches a dayover billions of web pages.Book organization and course developmentThis book is the result of a series of courses we have taught at Stanford Uni-versity and at the University of Stuttgart,in a range of durations includinga single quarter,one semester,and two quarters.These courses were aimedat early stage graduate students in computer science,but we have also hadenrollment from upper-class computer science undergraduates,as well asstudents from law,medical informatics,statistics,linguistics,and various en-gineering disciplines.The key design principle for this book,therefore,wasto cover what we believe to be important in a one-term graduate course onIR.An additional principle is to build each chapter around material that webelieve can be covered in a single lecture of75to90minutes.Thefirst eight chapters of the book are devoted to the basics of informationretrieval and in particular the heart of search engines;we consider this ma-terial to be core to any course on information retrieval.Chapter1introducesinverted indexes and shows how simple Boolean queries can be processedusing such indexes.Chapter2builds on this introduction by detailing themanner in which documents are preprocessed before indexing and by dis-cussing how inverted indexes are augmented in various ways for function-ality and speed.Chapter3discusses search structures for dictionaries andhow to process queries that have spelling errors and other imprecise matchesto the vocabulary in the document collection being searched.Chapter4de-scribes a number of algorithms for constructing the inverted index from atext collection with particular attention to highly scalable and distributed al-gorithms that can be applied to very large collections.Chapter5covers tech-niques for compressing dictionaries and inverted indexes.These techniquesare critical for achieving subsecond response times to user queries in largesearch engines.The indexes and queries considered in Chapters1through5only deal with Boolean retrieval,in which a document either matches a queryor does not.A desire to measure the extent to which a document matches aquery,or the score of a document for a query,motivates the development ofterm weighting and the computation of scores in Chapters6and7,leadingto the idea of a list of documents that are rank-ordered for a query.Chapter8focuses on the evaluation of an information retrieval system based on therelevance of the documents it retrieves,allowing us to compare the relativeMore informationPreface xviiperformances of different systems on benchmark document collections andqueries.Chapters9through21build on the foundation of thefirst eight chaptersto cover a variety of more advanced topics.Chapter9discusses methods bywhich retrieval can be enhanced through the use of techniques like relevancefeedback and query expansion,which aim at increasing the likelihood of re-trieving relevant documents.Chapter10considers IR from documents thatare structured with markup languages like XML and HTML.We treat struc-tured retrieval by reducing it to the vector space scoring methods developedin Chapter6.Chapters11and12invoke probability theory to compute scoresfor documents on queries.Chapter11develops traditional probabilistic IR,which provides a framework for computing the probability of relevance ofa document,given a set of query terms.This probability may then be usedas a score in ranking.Chapter12illustrates an alternative,wherein,for eachdocument in a collection,we build a language model from which one canestimate a probability that the language model generates a given query.Thisprobability is another quantity with which we can rank-order documents.Chapters13through18give a treatment of various forms of machine learn-ing and numerical methods in information retrieval.Chapters13through15treat the problem of classifying documents into a set of known categories,given a set of documents along with the classes they belong to.Chapter13motivates statistical classification as one of the key technologies needed fora successful search engine;introduces Naive Bayes,a conceptually simpleand efficient text classification method;and outlines the standard method-ology for evaluating text classifiers.Chapter14employs the vector spacemodel from Chapter6and introduces two classification methods,Rocchioand k nearest neighbor(kNN),that operate on document vectors.It alsopresents the bias-variance tradeoff as an important characterization of learn-ing problems that provides criteria for selecting an appropriate method for atext classification problem.Chapter15introduces support vector machines,which many researchers currently view as the most effective text classifica-tion method.We also develop connections in this chapter between the prob-lem of classification and seemingly disparate topics such as the induction ofscoring functions from a set of training examples.Chapters16,17,and18consider the problem of inducing clusters of relateddocuments from a collection.In Chapter16,wefirst give an overview of anumber of important applications of clustering in IR.We then describe twoflat clustering algorithms:the K-means algorithm,an efficient and widelyused document clustering method,and the expectation-maximization al-gorithm,which is computationally more expensive,but also moreflexible.Chapter17motivates the need for hierarchically structured clusterings(in-stead offlat clusterings)in many applications in IR and introduces a numberof clustering algorithms that produce a hierarchy of clusters.The chapterMore informationxviii Prefacealso addresses the difficult problem of automatically computing labels forclusters.Chapter18develops methods from linear algebra that constitutean extension of clustering and also offer intriguing prospects for algebraicmethods in IR,which have been pursued in the approach of latent semanticindexing.Chapters19through21treat the problem of web search.We give in Chap-ter19a summary of the basic challenges in web search,together with a setof techniques that are pervasive in web information retrieval.Next,Chap-ter20describes the architecture and requirements of a basic web crawler.Finally,Chapter21considers the power of link analysis in web search,usingin the process several methods from linear algebra and advanced probabilitytheory.This book is not comprehensive in covering all topics related to IR.Wehave put aside a number of topics,which we deemed outside the scope ofwhat we wished to cover in an introduction to IR class.Nevertheless,forpeople interested in these topics,we provide the following pointers to mainlytextbook coverage:Cross-language IR Grossman and Frieder2004,ch.4,and Oard andDorr1996.Image and multimedia IR Grossman and Frieder2004,ch.4;Baeza-Yates and Ribeiro-Neto1999,ch.6;Baeza-Yates and Ribeiro-Neto1999,ch.11;Baeza-Yates and Ribeiro-Neto1999,ch.12;del Bimbo1999;Lew2001;and Smeulders et al.2000.Speech retrieval Coden et al.2002.Music retrieval Downie2006and /.User interfaces for IR Baeza-Yates and Ribeiro-Neto1999,ch.10.Parallel and peer-to-peer IR Grossman and Frieder2004,ch.7;Baeza-Yates and Ribeiro-Neto1999,ch.9;and Aberer2001.Digital libraries Baeza-Yates and Ribeiro-Neto1999,ch.15,and Lesk2004.Information science perspective Korfhage1997;Meadow et al.1999;and Ingwersen and J¨a rvelin2005.Logic-based approaches to IR van Rijsbergen1989.Natural language processing techniques Manning and Sch¨utze1999;Jurafsky and Martin2008;and Lewis and Jones1996.PrerequisitesIntroductory courses in data structures and algorithms,in linear algebra,andin probability theory suffice as prerequisites for all twenty-one chapters.Wenow give more detail for the benefit of readers and instructors who wish totailor their reading to some of the chapters.More informationPreface xixChapters1through5assume as prerequisite a basic course in algorithmsand data structures.Chapters6and7require,in addition,a knowledge ofbasic linear algebra,including vectors and dot products.No additional pre-requisites are assumed until Chapter11,for which a basic course in prob-ability theory is required;Section11.1gives a quick review of the conceptsnecessary in Chapters11,12,and13.Chapter15assumes that the readeris familiar with the notion of nonlinear optimization,although the chaptermay be read without detailed knowledge of algorithms for nonlinear op-timization.Chapter18demands afirst course in linear algebra,includingfamiliarity with the notions of matrix rank and eigenvectors;a brief reviewis given in Section18.1.The knowledge of eigenvalues and eigenvectors isalso necessary in Chapter21.Book layout✎Worked examples in the text appear with a pencil sign next to them in theleft margin.Advanced or difficult material appears in sections or subsections ✄with a question mark.The level of difficulty of exercises is indicated as easy indicated with scissors in the margin.Exercises are marked in the margin?[ ],medium[ ],or difficult[ ].AcknowledgmentsThe authors thank Cambridge University Press for allowing us to make thedraft book available online,which facilitated much of the feedback we havereceived while writing the book.We also thank Lauren Cowles,who has beenan outstanding editor,providing several rounds of comments on each chap-ter;on matters of style,organization,and coverage;as well as detailed com-ments on the subject matter of the book.To the extent that we have achievedour goals in writing this book,she deserves an important part of the credit.We are very grateful to the many people who have given us comments,suggestions,and corrections based on draft versions of this book.We thankfor providing various corrections and comments:Cheryl Aasheim,Josh At-tenberg,Luc B´e langer,Tom Breuel,Daniel Burckhardt,Georg Buscher,Fa-zli Can,Dinquan Chen,Ernest Davis,Pedro Domingos,Rodrigo PanchiniakFernandes,Paolo Ferragina,Norbert Fuhr,Vignesh Ganapathy,Elmer Gar-duno,Xiubo Geng,David Gondek,Sergio Govoni,Corinna Habets,BenHandy,Donna Harman,Benjamin Haskell,Thomas H¨uhn,Deepak Jain,Ralf Jankowitsch,Dinakar Jayarajan,Vinay Kakade,Mei Kobayashi,Wes-sel Kraaij,Rick Lafleur,Florian Laws,Hang Li,David Mann,Ennio Masi,Frank McCown,Paul McNamee,Sven Meyer zu Eissen,Alexander Murzaku,Gonzalo Navarro,Scott Olsson,Daniel Paiva,Tao Qin,Megha Raghavan,More informationxx PrefaceGhulam Raza,Michal Rosen-Zvi,Klaus Rothenh¨a usler,Kenyu L.Run-ner,Alexander Salamanca,Grigory Sapunov,Tobias Scheffer,Nico Schlae-fer,Evgeny Shadchnev,Ian Soboroff,Benno Stein,Marcin Sydow,AndrewTurner,Jason Utt,Huey Vo,Travis Wade,Mike Walsh,Changliang Wang,Renjing Wang,and Thomas Zeume.Many people gave us detailed feedback on individual chapters,eitherat our request or through their own initiative.For this,we’re particularlygrateful to James Allan,Omar Alonso,Ismail Sengor Altingovde,Vo NgocAnh,Roi Blanco,Eric Breck,Eric Brown,Mark Carman,Carlos Castillo,Junghoo Cho,Aron Culotta,Doug Cutting,Meghana Deodhar,Susan Du-mais,Johannes F¨urnkranz,Andreas Heß,Djoerd Hiemstra,David Hull,Thorsten Joachims,Siddharth Jonathan J.B.,Jaap Kamps,Mounia Lal-mas,Amy Langville,Nicholas Lester,Dave Lewis,Stephen Liu,DanielLowd,Yosi Mass,Jeff Michels,Alessandro Moschitti,Amir Najmi,MarcNajork,Giorgio Maria Di Nunzio,Paul Ogilvie,Priyank Patel,Jan Peder-sen,Kathryn Pedings,Vassilis Plachouras,Daniel Ramage,Stefan Riezler,Michael Schiehlen,Helmut Schmid,Falk Nicolas Scholer,Sabine Schulteim Walde,Fabrizio Sebastiani,Sarabjeet Singh,Alexander Strehl,John Tait,Shivakumar Vaithyanathan,Ellen Voorhees,Gerhard Weikum,Dawid Weiss,Yiming Yang,Yisong Yue,Jian Zhang,and Justin Zobel.Andfinally there were a few reviewers who absolutely stood out in termsof the quality and quantity of comments that they provided.We thank themfor their significant impact on the content and structure of the book.We ex-press our gratitude to Pavel Berkhin,Stefan B¨uttcher,Jamie Callan,ByronDom,Torsten Suel,and Andrew Trotman.Parts of the initial drafts of Chapters13,14,and15were based on slidesthat were generously provided by Ray Mooney.Although the material hasgone through extensive revisions,we gratefully acknowledge Ray’s contri-bution to the three chapters in general and to the description of the timecomplexities of text classification algorithms in particular.The above is unfortunately an incomplete list;we are still in the process ofincorporating feedback we have received.And,like all opinionated authors,we did not always heed the advice that was so freely given.The publishedversions of the chapters remain solely the responsibility of the authors.The authors thank Stanford University and the University of Stuttgart forproviding a stimulating academic environment for discussing ideas and theopportunity to teach courses from which this book arose and in which itscontents were refined.CM thanks his family for the many hours they’ve lethim spend working on this book and hopes he’ll have a bit more free time onweekends next year.PR thanks his family for their patient support throughthe writing of this book and is also grateful to Yahoo!Inc.for providing afertile environment in which to work on this book.HS would like to thankhis parents,family,and friends for their support while writing this book.。
4-信息获取系统评价Retrieval Evaluation
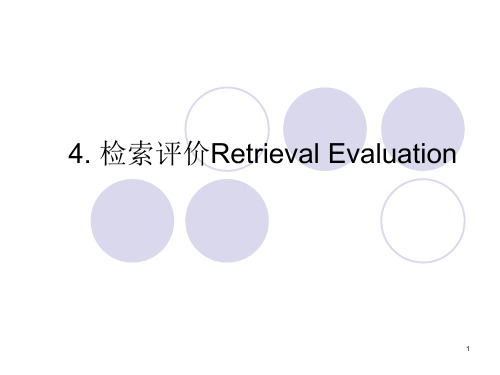
怎样的正确率和召回率表示是一个好的搜索系统?
好或不好是相对的,没有绝对的值
为什么在增大召回率的时候经常导致降低正确率? 为了尽可能不漏掉,系统可能会多检出一些文档, 这些文档往往是不相关的,于是导致整体正确率 下降 正确率和召回率往往是一对矛盾,需要权衡
20
理想化的IR系统
最理想的系统对所有查询都有P=1, R=1 可能吗?为什么?
6
与什么相关?
用户的信息需求
问题? 查询请求?
关于相关性最终的测试是
用户发现信息有用 用户能够用信息解决问题 用户发现通过检索学到了他之前所不知道 的一些东西
7
相关性判断Relevance Judgment
从用户的角度进行判断
检索到的文档从多大程度上满足了用户的需求 检索到的文档有多有用 如果文档有关但没什么用
16
正确率和召回率
两个指标分别衡量了系统的某个方面,但是为比较带来了 难度,究竟哪个系统好?
解决方法:单一指标,将两个指标融成一个指标
两个指标都是基于集合进行计算,并没有考虑序的作用
举例:两个系统,对某个查询,返回的相关文档数目一样都是10, 但是第一个系统是前10条结果,后一个系统是最后10条结果。显 然,第一个系统优。但是根据上面基于集合的计算,显然两者指 标一样。 解决方法:引入序的作用
Query 3 0.45/0.5 0.4/0.5 0.5/ 0.7
Query 4 0.3/0.6 0.3/0.7 0.3/0.8
Query 5 0.1/ 0.8 0.2/0.8 0.2/ 0.9
30
P 1.0
用P-R 图比较不同系统
System A System B
0.5
信息素养(Informationliteracy)

信息素养(Information literacy)"信息素养 (information literacy)" 的本质是全球信息化需要人们具备的一种基本能力.信息素养这一概念是信息产业协会主席保罗·泽考斯基于1974年在美国提出的.简单的定义来自1989年美国图书馆学会 (american library association, ala), 它包括: 能够判断什么时候需要信息, 并且懂得如何去获取信息, 如何去评价和有效利用所需的信息.这样的看法目前已形成一种共识.除美国外, 一些西方发达国家的观点大同小异, acrl提出的高校信息素养标准, 在美国已被广泛认可和接收, 英国、澳大利亚等根据国情, 稍有补充和修改.编辑本段定义信息素养 (information literacy) 更确切的名称应该是信息文化(information literacy) 信息素养是一种基本能力信息素养是一种对信息社会的适应能力.美国教育技术ceo论坛2001年第4季度报告提出21世纪的能力素质, 包括基本学习技能 (指读、写、算) 、信息素养、创新思维能力、人际交往与合作精神、实践能力.信息素养是其中一个方面, 它涉及信息的意识、信息的能力和信息的应用. 信息素养是一种综合能力信息素养涉及各方面的知识, 是一个特殊的、涵盖面很宽的能力, 它包含人文的、技术的、经济的、法律的诸多因素, 和许多学科有着紧密的联系.信息技术支持信息素养, 通晓信息技术强调对技术的理解、认识和使用技能.而信息素养的重点是内容、传播、分析, 包括信息检索以及评价, 涉及更宽的方面.它是一种了解、搜集、评估和利用信息的知识结构, 既需要通过熟练的信息技术, 也需要通过完善的调查方法、通过鉴别和推理来完成.信息素养是一种信息能力, 信息技术是它的一种工具.编辑本段九大信息素养标准1998年, 美国图书馆协会和教育传播协会制定了学生学习的九大信息素养标准, 概括了信息素养的具体内容. 标准一: 具有信息素养的学生能够有效地和高效地获取信息. 标准二: 具有信息素养的学生能够熟练地和批判地评价信息. 标准三: 具有信息素养的学生能够有精确地、创造性地使用信息. 标准四: 作为一个独立学习者的学生具有信息素养, 并能探求与个人兴趣有关的信息. 标准五: 作为一个独立学习者的学生具有信息素养, 并能欣赏作品和其他对信息进行创造性表达的内容. 标准六: 作为一个独立学习者的学生具有信息素养, 并能力争在信息查询和知识创新中做得最好. 标准七: 对学习社区和社会有积极贡献的学生具有信息素养, 并能认识信息对民主化社会的重要性. 标准八: 对学习社区和社会有积极贡献的学生具有信息素养, 并能实行与信息和信息技术相关的符合伦理道德的行为. 标准九: 对学习社区和社会有积极贡献的学生具有信息素养, 并能积极参与小组的活动探求和创建信息.编辑本段信息素养的内涵(一) 信息文化常识信息系统是由硬件、软件与人三个要素组成的一个整体, 三个要素之间必须十分协调地工作, 才能充分发挥信息系统的效能, 达到预期目标. 硬件是对信息系统的所有物理的实际设施的通称, 包括信息存储设备, 信息传输设备, 信息输入、输出设备以及信息处理设备等几类.The information storage device. Information needs to be stored before spreading, such as for video recording equipment, sound and image information stored in the computer memory storage and data are stored in program information storage device. According to the information organization mode, information storage devices can be divided into internal storage device.According to the information organization mode, information storage devices can be divided into internal memory, storage devices, storage devices, random sequence. The information input and output devices. The input device includes character input device, position input device, image information input device, voice information input device, a variety of sensors. Output devices include visual output device. Information processing equipment. The function of the most comprehensive and powerful computer. Information transmission equipment, network has become an important information transmission equipment in people's social lives. The software is control and indicating how the hardware information collection, information processing, information storage, information dissemination and information tasks for information technology systems. Operating system, a software system used for resource management of the computer system. Software tools, including maintenance tools and common tools. Software development tools, such as a variety of programming languages, media management tools, information browsing tools. Application software refers to various information system software designed to do different jobs. Who is the most important factor in the information system, the coordination of information systems is a very important work, as an important part of information literacy, information and knowledge is the indispensable content. As an information literate person, should understand: basic knowledge of information technology (all terms, all kinds of technology and information technology, information technology development history and trends); working principle of information system (Digital principle, algorithm and program, data, information dissemination principle); and each information structure system components (hardware, software, system); and the impactof information technology (advantages and limitations of the use of information technology etc.); knowledge of law and morality is related to information technology.(two) the information consciousness and emotionTo have the information literacy, undoubtedly need to learn to use information technology. But not necessarily familiar with information technology. Moreover, with the development of high technology, information technology is to become popular partners for the development, operation is more simple, reliable and timely information to provide all kinds of convenience for the people. Therefore, modern people's information literacy level, first depends on the information consciousness and emotion. The information consciousness and emotion mainly includes: to face the challenges of information technology, information technology is not afraid of; with a positive attitude of learning to operate a variety of information tools; understand the source of information and often use information tools; can quickly and acutely capture all kinds of information, and willing to take the information technology as the basic means of work; that the role and value of information the limitations and negative effects of information technology so as to correctly deal with various information; identity and abide by the information exchanges in a variety of moral norms and conventions.(three) information skillsAccording to the information of education experts, teachers and students in modern society should have six information skills:determine the information task to judge exactly the problem, and to determine the specific information associated with the problem. The decision of information strategy in decision may need to be included in the information which is useful information resources. Information retrieval strategy began to implement the query strategy. This part skills include: the use of information access tools, organization of each part of the arrangement of information materials and textbooks, and decided to search for online resources strategy. Choose to use information in the seized information, through interaction of listening, looking and reading behavior and information, to decide which information is helpful to solve the problem, and can extract the required records. Copy and reference information. Comprehensive information refers to the information re combined and packaged into different forms to meet the different task requirements. General can be very simple, can also be very complex.The evaluation information refers to answer questions to determine the implementation of information problem solving process effectiveness and efficiency. In the evaluation of efficiency also need to consider the value of the time spent in activities, and the estimated time required to complete the task which is right.The information literacy training of EditorsThe information technology education includes two aspects, one is the education of information technology, the two is the integration of information technology course and other courses. Today, to improve teenagers' information literacy has becomea core element of the infiltration of quality education. It is necessary to put forward new requirements to the teachers, which opened in the course of information technology at the same time, to actively explore the ideas and methods of the integration of information technology and other courses, the application of modern information technology in the classroom, the information technology curriculum into other courses to cultivate students' information literacy through school education channel. Therefore, teachers should do the following. To the cultivation of information literacy into contact with organic materials, cognitive tools, network and the development of a variety of learning and teaching resources. The information presented in the form of diversification to form students' demand for information, search, evaluation, training students' ability of effective use, and create a form to convey information, and thus expand the students' understanding of the essence of information. Adhere to the development of the students. Don't pay too much attention to knowledge learning, and should be concerned about how to guide students to use information technology tools to solve problems, especially by the learning and teaching of information technology combine to allow students to acquire knowledge and technology as information processing, to solve the problem and service tools. At the same time, teachers should be concerned about the emotional development of students, not because of the intervention of information technology while ignoring the direct dialogue and communication with the students. In cultivating students' information literacy at the same time, but also pay attention to the development of students' information literacy and is closely related to the "media literacy", "computer literacy" and "visual literacy" and"artistic quality" and "digital literacy", in order to improve students' comprehensive quality to adapt to the information needs of the times. Information literacy education is to cultivate students' innovative spirit and practical ability as the core. Therefore, in the information technology course, must be based on independent learning and collaborative learning environment, students, active learning, curriculum designers and teachers become mentors students, make students really become the subject of study. Teachers can use the network and multimedia technology, the construction of information rich and reflective, conducive to learning environment and tools of students' autonomous learning, cooperative learning and inquiry learning, to develop students' autonomous learning strategies, allow students to freely explore, greatly promote the development of their critical and creative thinking. In our country, according to the actual situation of the domestic education, students' information literacy training mainly in the following five aspects. (1) the love of life, will have access to new information, can take the initiative from life practice continue to find and explore new information. (2) with the basic scientific and cultural knowledge, can more easily carry out the discrimination and analysis of the information obtained, correctly evaluate. (3) can flexibly control information, a good grasp of the information, select the skills to. (4) the use of information, to express personal thoughts and ideas effectively, and willing to share different opinions or information with others. (5) no matter what the situation, they can confidently use all kinds of information to solve the problem, there is a strong sense of innovation and enterprising spirit.The eight section mainly displays the ability to editInformation literacy is the ability of mainly the following 8 aspects: (1) the use of information tools: can skillfully use a variety of information tools, especially Internet communication tools; (2): access to information can effectively collect all kinds of learning materials and information according to their own learning objectives, be proficient in reading, discussion, visit, visit experiment and retrieval method for retrieving information;(3): information processing to the information collected by induction and classification, memory, identification, selection, comprehensive analysis, abstraction and expression;(4) generating information: Based on information collected, accurate, comprehensive, and fulfill the overview required to express information, make it simple clear, easy and full of personality characteristics; (5) the creation of information based on the interaction of several collect information on the burst of creative thinking sparks, producing new information, so as to create new information, achieve ultimate goal of collecting information; (6) play the benefit of information: good use of acceptance to solve the problem of information, so that information to maximize the social and economic benefits;(7): the information and information collaboration tools as information across time and space, "zero distance" communication and cooperation Intermediary, make it become the extension of efficient means of their own, establish harmonious relationship with the outside world; (8) the immune information: the vast information resources are uneven in quality needs to have the correct outlook on life, values, and discriminationability and self-control, self-discipline and self adjustment ability, can consciously resist the erosion and interference and eliminate waste information and harmful information, and improve the ethical quality accord with the information era。
- 1、下载文档前请自行甄别文档内容的完整性,平台不提供额外的编辑、内容补充、找答案等附加服务。
- 2、"仅部分预览"的文档,不可在线预览部分如存在完整性等问题,可反馈申请退款(可完整预览的文档不适用该条件!)。
- 3、如文档侵犯您的权益,请联系客服反馈,我们会尽快为您处理(人工客服工作时间:9:00-18:30)。
•
分类检索语言
•
分类检索语言是指用分类号表达各种概念,并将 各种概念以学科性质为主加以划分和系统排列的 检索语言。
分类检索语言又称为分类法,《中国图书馆分类 法》、《中国科学院图书馆图书分类法》、《中 国人民大学图书馆图书分类法》、《国际十进分 类法》、《杜威十进分类法》、《国际专利分类 表》。
•
主题检索语言
•
主题语言,也称主题法,是一种用语词标识处理 原始信息、组织主题检索工具或检索系统的检索 语言。主题语言又分为标题词语言、单元词语言、 关键词语言和叙词语言。
由于主题检索语言直接以代表主题概念的主题词 作为信息标识,以主题事物为中心,将与该主题 事物有关的所有信息集中在一起,从而可以检索 出各学科论述同一主题事物的所有信息。
Contributor Source Date Language Resources type Relation Format Coverage Identifier Rights
个人知识管理建议
• • • • • •
•
• •
越早越好 越广越好 越精越好 越长越好 越细致越好 越浓越好 越专业越好 越懒越好 ……
截词检索
•
1.
?computer可以检索到 computer、 minicomputer、microcomputer 等结果。 computer?可以检索到 computer、 computers、computerize、 computerized、computerization 等结果。 ?computer?可以检索到 computer、minicomputer、 microcomputer、computers、 computerize、computerized、 computerization等结果。 defen*e可以同时检索出 defense和defence。
boolean operators 1
• •
• •
•
逻辑与 用于限定检索结果,缩小 检索范围,增强检索的专 指度,提高信息查准率。 逻辑运算符:AND 、* 。 逻辑表达式:A AND B 或 写成A * B 。 检索语义:被检索的文献 记录中同时含有A 和B 两 个概念。
boolean operators 2
•
关键词语言
•
关键词语言是直接选用文献信息中的自然 语言作基本词汇,并将那些能够揭示文献 信息题名或主题意旨的关键性自然语词作 为关键词进行标引的一种检索语言。
7.检索评价
评价指标: • 查全率(检全率)
检出相关文献量 检全率 100% 系统中相关文献总量
•
查准率(检准率)
检出相关文献量 检准率 100% 检出文献总量
software, tools
1.rss, google reader 2.email 3.search engine 4.website 1.tags 2.folders 3.cloud storage 4.note
1.分享 2.blog 3.microblog 4.generate 5.papers 6.……
search it yourself
• •
位置检索 加权检索
4.如何确定信息源
图书 2. 期刊 3. 专业数据 4. 网络信息
1.
• • • • • • •
如何选择?
•
逛网站 找类似 发现最适合 理解网站 理解网络 理解互联网 理解信息的形态
5.信息检索过程
1. 2.
分析研究课题
制定检索需求描述
Query Interpreted
Results Ranked
Index searched Items retrieved
2.检索者应具备的素质
information literacy
①专业素质 ②信息意识 ③计算机能力 ④学习能力 ⑤信息道德
3.信息检索技术
1.布尔逻辑检索 2.截词检索 3.字段检索 4.位置检索 5.加权检索
信息检索
李俊
libiun+iloveyou@
信息检索基础知识
1. 信息检索的内涵
2. 检索者应具备的素质
3. 信息检索技术 4. 确定信息源
5. 信息检索过程
6. 检索语言 7. 检索评价
/~hearst/irbook/
3.
4.
调整检索策略
索取原始文献
ห้องสมุดไป่ตู้
分析研究课题
•
明确以下问题:
1.
2.
3.
4.
分析客体的主要内容以及所涉及的知识点 明确所需要的文献种类、语种、年代以及文 献量。 明确对查新、查准、查全的指标要求以及侧 重点。 确定所需要的文献应该具备的内外部特征。
•
课题检索的类型:
• • •
查全型:开题报告、综述等 查准型:在具体细微的专业问题方面的研究 动态型:新技术、新理论的研究
•
查新型:同类研究项目比较
6.检索语言
•
用来描述文献特征和表达检索提问的一种专门语言,是用于 文献标引和检索提问的约定语言。 在信息存贮过程中,使用检索语言描述信息的内容特征和 外表特征,从而形成文献标识; 在信息检索过程中,使用检索语言描述检索提问,从而形 成提问标识。
•
•
•
当提问标识与文献标识完全匹配或部分匹配时,需要的信 息就被检索出来了。
•
•
history
信息著录,标引 Standard Metatags
•
The Dublin Core (/)
15 common items to use in labeling any web document
Title Creator Subject Description Publisher
•
•
•
boolean operators 4
•
• • • •
逻辑异或
用于表示结果信息 排除。 逻辑运算符是:xor 逻辑表达式为:A xor B 。 语义表示:被检文献 为包含逻辑 A ,也包含逻辑B ,但不包含同 时含有A 和B 的信息。用逻辑与、 或、非表达则为(A or B )not (A and B) 。
1.信息检索的内涵
•
信息检索的三要素
• • •
•
广义
• •
信息需求者 检索技术 信息源
•
information storage information retrieval
狭义
•
information retrieval
information storage
• • • • •
信息采集 信息著录 信息标引 信息整序 信息结构规范 信息存储的自动化 信息检索接口创建
预备概念
• • • •
•
• •
•
• •
book journal digital library paper database query, retrieval internet website information knowledge
• • • • • •
•
•
search engine web application desktop application mobile application software language cloud computing Google
•
•
逻辑或
用于并列概念的组配。使用逻辑 或可以扩大检索范围,提高信息 查全率。 逻辑运算表示符:OR、 + 。 逻辑表达式:A OR B、A + B 。 语义表达:被检文献中含有A 或 含有B 以及两词概念都包含。
•
• •
boolean operators 3
•
•
逻辑非
用于排除含有不需要概念的信 息,可缩小所检索信息的范围。 逻辑运算符是:NOT 、- 。 逻辑表达式为:A NOT B 、A B。 语义表示:被检文献中含有A 而不含有B 概念的文献。
评价指标:
•漏检率
漏检相关文献量 漏检率 100% 系统中相关文献总量
•误检率
误检文献量 误检率 100% 检出文献总量
• • •
逛逛
•
•
•
截词检索就是用截断的词 的一个局部进行的检索, 是检索词与数据库所存储 信息字符的部分一致性匹 配检索。 截词检索的作用在于扩大 检索范围。 按截断的位置截词技术有 后截断、前截断、前后截 断和中间截断四种类型。 常用的截词符号有? 、$ 、 * 等。
2.
3.
4.
Fields
• •
•
字段检索 是指定检索词在记录中某一具体的字段中出现可 以提高检索的效率。 被指定的字段也称检索入口,在数据库的复杂检 索或高级检索中多提供几个字段供用户同时选用。
1.阅读 2.整理 3.总结归纳 4.理解创新 5.……
information retrieval
1. 2.
3. 4. 5.
6.
7.
用户确定需求 用检索界面定制 条件 信息源接收请求 信息源建立结果 返回信息 用户接收信息 again
the process of search engine
Query entered