计量经济学(英文PPT)Chapter 21 Time Series Econometrics ⅠStationarity Unit roots and Cointegration
合集下载
计量经济学(英文版)精品PPT课件
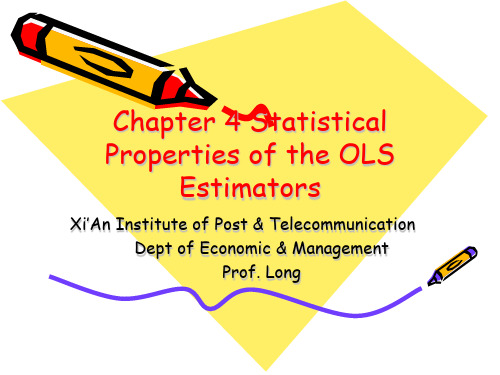
(4.3a)
Expand and multiply top and bottom by n:
b2
=
nSxiyi - Sxi Syi nSxi2-(Sxi) 2
(4.3b)
Variance of b2
4.12
Given that both yi and ei have variance s2,
the variance of the estimator b2 is:
4. cov(ei,ej) = cov(yi,yj) = 0 5. xt c for every observation
6. et~N(0,s 2) <=> yt~N(b1+ b2xt,
The population parameters b1 and b2 4.4 are unknown population constants.
b2
+
nSxiEei - Sxi SEei nSxi2-(Sxi) 2
Since Eei = 0, then Eb2 = b2 .
An Unbiased Estimator
4.8
The result Eb2 = b2 means that the distribution of b2 is centered at b2.
4.6
The Expected Values of b1 and b2
The least squares formulas (estimators) in the simple regression case:
b2 =
nSxiyi - Sxi Syi nSxi22 -(Sxi) 2
b1 = y - b2x
计量经济学ppt课件
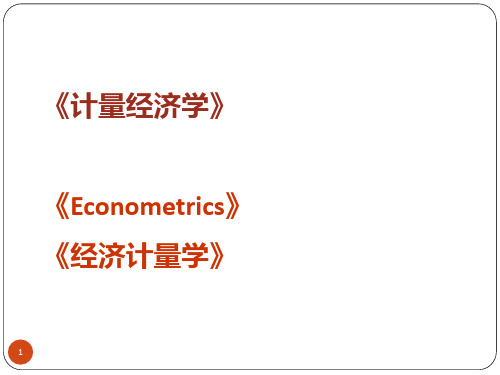
5
⒊ 课程说明
⑴ 教学目的
经济学是一门科学,实证的方法,尤其是数量 分析方法是经济学研究的基本方法论。通过该门课 程教学,使学生掌握计量经济学的基本理论与方法, 并能够建立实用的计量经济学应用模型。
⑵ 先修课程
中级微观经济学、中级宏观经济学、经济统计 学、微积分、线性代数、概率论与数理统计、应用 数理统计
14
△ 在经济学科中占据极重要的地位 克莱因(R.Klein):“计量经济学已经在经 济学科中居于最重要的地位”,“在大多数大 学和学院中,计量经济学的讲授已经成为经济 学课程表中最有权威的一部分”。 萨缪尔森(P.Samuelson) :“第二次大战 后的经济学是计量经济学的时代”。
15
二、计量经济学模型
→Ntserver→Lizn 院外:\\166.111.96.50→Lizn
⑸ 教学讨论区
学院主页→分类讨论区→计量经济学讨论区 进入帐号:s004,口令:kc9476
⑹ 答疑时间
8
⑺ 课程内容提纲及学时安排
(总课时:48学时,课内外周学时:3/6)
第一章 绪论
3学时
第二章 单方程计量经济学模型理论与方法 15学时
本课程中的计量经济学模型,就是狭义计量经 济学意义上的经济数学模型。
18
△ 初、中、高级计量经济学
6
⑶ 教材及参考书
《计量经济学》,李子奈,高等教育出版社,2000年7月
《Basic Econometrics》,Damodar N. Gujarrati,2001
《计量经济学—方法与应用》,李子奈,清华大学出版社, 1992年
《高等计量经济学》,李子奈、叶阿忠,清华大学出版社, 2000年
《计量经济学—理论、方法与模型》,唐国兴,复旦大学出 版社,1988年
⒊ 课程说明
⑴ 教学目的
经济学是一门科学,实证的方法,尤其是数量 分析方法是经济学研究的基本方法论。通过该门课 程教学,使学生掌握计量经济学的基本理论与方法, 并能够建立实用的计量经济学应用模型。
⑵ 先修课程
中级微观经济学、中级宏观经济学、经济统计 学、微积分、线性代数、概率论与数理统计、应用 数理统计
14
△ 在经济学科中占据极重要的地位 克莱因(R.Klein):“计量经济学已经在经 济学科中居于最重要的地位”,“在大多数大 学和学院中,计量经济学的讲授已经成为经济 学课程表中最有权威的一部分”。 萨缪尔森(P.Samuelson) :“第二次大战 后的经济学是计量经济学的时代”。
15
二、计量经济学模型
→Ntserver→Lizn 院外:\\166.111.96.50→Lizn
⑸ 教学讨论区
学院主页→分类讨论区→计量经济学讨论区 进入帐号:s004,口令:kc9476
⑹ 答疑时间
8
⑺ 课程内容提纲及学时安排
(总课时:48学时,课内外周学时:3/6)
第一章 绪论
3学时
第二章 单方程计量经济学模型理论与方法 15学时
本课程中的计量经济学模型,就是狭义计量经 济学意义上的经济数学模型。
18
△ 初、中、高级计量经济学
6
⑶ 教材及参考书
《计量经济学》,李子奈,高等教育出版社,2000年7月
《Basic Econometrics》,Damodar N. Gujarrati,2001
《计量经济学—方法与应用》,李子奈,清华大学出版社, 1992年
《高等计量经济学》,李子奈、叶阿忠,清华大学出版社, 2000年
《计量经济学—理论、方法与模型》,唐国兴,复旦大学出 版社,1988年
《计量经济学简介》PPT课件

D
R
T=
(0.68) (5.32)
(1.58)
R2= 0.73 调整的精选RP2P=T 0.68
F=20.18
12
➢ 关于随机扰动项
1. 引进的必要性:
(1)经济行为具有随机性;
(2)设定模型时省略了很多因素;
(3)取样本时也会有测量误差。
2. 构成:
(1)省略误差:x的 次要的解释变量必须扔掉
1
2D 3 R
精选PPT
11
三、计量经济模型的建立(续)
(4)引进扰动项(下一页有解释)
C Y W 1 2D 3 R
理论上的经济计量模型
(二)收集数据:比如时序数据1973~1991年(t=19),
单位:亿元
(三)模型估计
(1)估计方法:比如 OLS
C ˆ Y W (2)估计式: 0 .0 0 3 0 .8 1 2 0 .1 3 8
不可观测的变量也得省掉
可观测不可定量化的省掉
未认识到的变量
f的:数学形式设定中导出的误差
(2)测量误差:观测误差、统计数据归并时的误差。
精选PPT
13
三、经济计量模型的建立(续)
(四)模型检验
(1)经济合理性检验:
比如YD和WR的系数是否在(0,1)之间 (2)古典统计检验:R2,T, F检验
(五)模型应用
人、企业等观测单位本身具有而我们又观测不 到的特性
精选PPT
18
例: 一个两年的面板数据格式如下
Obsno city year y x1 x2 x3
1
1 1986 . . .
.
2
1 1990 . . .
.
3
计量经济学(英文PPT)Chapter 22 TIME SERIES CONOMETRICS FORECASTING
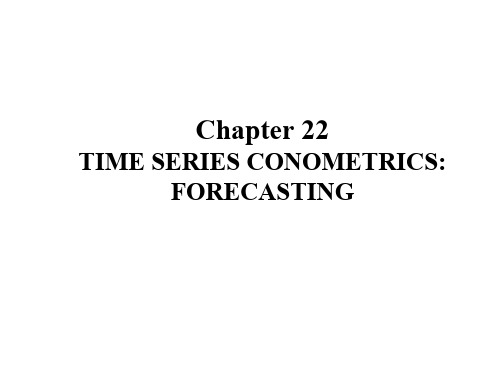
1.Single Exponential Smoothing 2.Holt’s Linear Method 3.Holt winter’s Method
and their variations.
We will not discuss them in this chapter.
§22.1 APPROACHES TO ECONOMIC
Step3.Diagnostic checking. That is, test whether the chosen model fits
the data reasonably well. Choosing the right model needs not only
the science,but also considerable skills and the art.
The prerequisite of using BJ methodology to model an ARMA process,is that we must have either a stationary time series or a time series that is stationary after one or more differencings.
In short,a moving average process is simply a linear combination of white noise error term.
Autoregressive and Moving Average (ARMA) Process
It is quite likely that Y has characteristics of both
form knowledge directly perceived through the senses
and their variations.
We will not discuss them in this chapter.
§22.1 APPROACHES TO ECONOMIC
Step3.Diagnostic checking. That is, test whether the chosen model fits
the data reasonably well. Choosing the right model needs not only
the science,but also considerable skills and the art.
The prerequisite of using BJ methodology to model an ARMA process,is that we must have either a stationary time series or a time series that is stationary after one or more differencings.
In short,a moving average process is simply a linear combination of white noise error term.
Autoregressive and Moving Average (ARMA) Process
It is quite likely that Y has characteristics of both
form knowledge directly perceived through the senses
计量经济学第六章-PPT课件
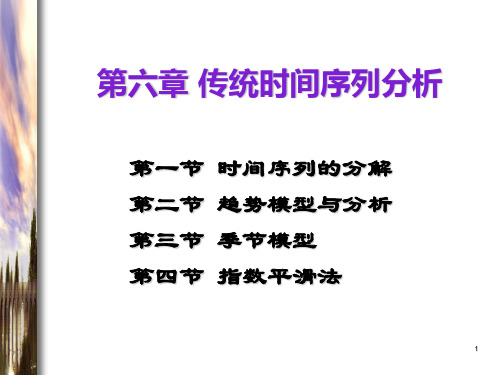
若模型有三个未知数,将数据三等分,分别求出 每部分的和,代入方程,得到三个方程,解方程 组可获得三个参数的估计值 10
模型的参数估计(续1)
参数的非线性最小二乘估计(第五章)
非线性模型可利用NLS进行参数的精确估计
首先,用param命令对参数赋初值 其次,输入方程,对模型进行估计
11
考虑选择指数曲线模型
2000000
1500000
1000000
500000
0 72 74 76 78 80 Y 82 84 YF 86 88 90 92
9
模型的参数估计
参数的最小二乘估计
常用的各类趋势模型参数估计仍常用OLS 其中,自变量为时间t
参数的三和值法(第五章)
若选用有增长上限的曲线趋势模型,当增长 上限事先不能确定时,可采用三和值法 基本思想
1961-1981年我国搪瓷面盆销售量数据如下 根据其变化,试以Gompertz曲线作为预测模型
由于增长上限L事先无法得知,参数估计可用NLS 在精确估计前,选择三和值法获得参数的初值 模型取对数转换成修正指数曲线 t ˆ y log L b log a log t
计算各段和值 根据参数计算公式计算参数值
产品市场生命周期
进入期 成长期 成熟期 衰退期
20
产品生命周期分析(续1)
f(t)
饱和点
进 成长期 入 期
成熟期 后 期 前 期
衰退期
t
21
产品生命周期分析(续2)
产品市场生命周期的各个阶段与某些趋势 模型存在大致的对应关系
《计量经济学入门》PPT课件
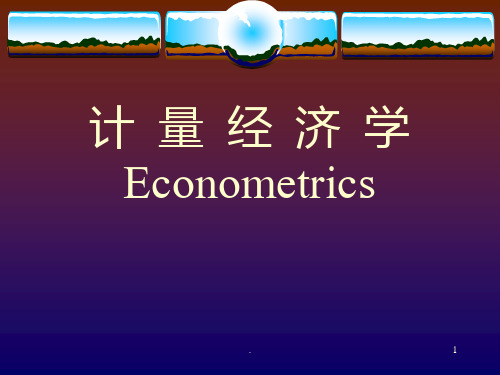
Q i 0 1 P i 2 P 0 i 3 Y i 4 T i u i
其中
Q i ——某种商品需求量;
.
13
P i——该商品的价格 ;
P0 i ——可替代商品的价格;
Y i ——消费者收入 ;
T i ——消费者偏好; u i ——影响商品需求量的其他因素和随机因素
0 ~ 4 ——需求函数的回.归系数。
14
参考书目
基础书: 高等数学、西方经济学、 概率论与数理统计
专业书: 1、《经济计量学》(第四版),张保法 编著,经济科学出版社,2000年版。 2、《计量经济学—理论、方法与模型》, 唐国兴,复旦大学出版. 社,1988年版。 15
❖ 3、《计量经济学》(第三版),李子奈,高等 教育出版社,2010年3月版。
的变化情况。 ❖ 截面数据的时间是固定的。
.
26
GDP growth rate:
平面数据 年份 中国 美国
(Panel Data) 1994 11.8 4.08
❖ 平面数据是 时间序列数据
1995 10.5 2.7 1996 9.6 3.61 1997 8.8 4.47
与截面数据的 1998 7.8 4.32
2001.1
8.1
2001.2
7.9
2001.3
7.6
2001.4
7.3
2002.1
7.6
2002.2
8.0
2002.3
7.9
2002.4
8.0
2003.1
9.9
2003.2
. 8.2
25
截面数据 (Cross-Sectional Data)
❖ 截面数据又俗称横向数据,是一批发生在同 一时间 截面上的调查数据。研究某个时点上
其中
Q i ——某种商品需求量;
.
13
P i——该商品的价格 ;
P0 i ——可替代商品的价格;
Y i ——消费者收入 ;
T i ——消费者偏好; u i ——影响商品需求量的其他因素和随机因素
0 ~ 4 ——需求函数的回.归系数。
14
参考书目
基础书: 高等数学、西方经济学、 概率论与数理统计
专业书: 1、《经济计量学》(第四版),张保法 编著,经济科学出版社,2000年版。 2、《计量经济学—理论、方法与模型》, 唐国兴,复旦大学出版. 社,1988年版。 15
❖ 3、《计量经济学》(第三版),李子奈,高等 教育出版社,2010年3月版。
的变化情况。 ❖ 截面数据的时间是固定的。
.
26
GDP growth rate:
平面数据 年份 中国 美国
(Panel Data) 1994 11.8 4.08
❖ 平面数据是 时间序列数据
1995 10.5 2.7 1996 9.6 3.61 1997 8.8 4.47
与截面数据的 1998 7.8 4.32
2001.1
8.1
2001.2
7.9
2001.3
7.6
2001.4
7.3
2002.1
7.6
2002.2
8.0
2002.3
7.9
2002.4
8.0
2003.1
9.9
2003.2
. 8.2
25
截面数据 (Cross-Sectional Data)
❖ 截面数据又俗称横向数据,是一批发生在同 一时间 截面上的调查数据。研究某个时点上
2024版计量经济学(很好用的完整)ppt课件
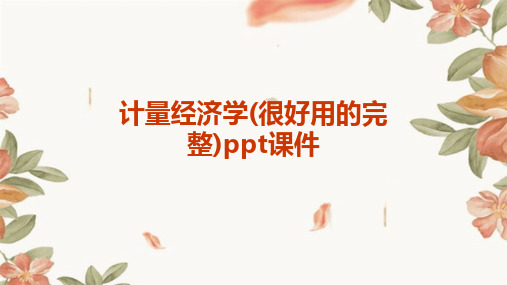
贝叶斯计量经济学的定义
基于贝叶斯定理和概率分布理论进行计量分析的经济学分支。
贝叶斯先验分布的设定
根据历史数据、专家经验等因素设定参数的先验分布,作为后续推 断的基础。
贝叶斯计量模型的估计方法
包括马尔科夫链蒙特卡罗方法、变分贝叶斯方法等,用于估计模型 参数和进行统计推断。
机器学习在计量经济学中应用
机器学习算法在计量经济学中的应用场景
广义线性模型介绍
1
定义
广义线性模型是一类用于回归分析的统计 模型,它扩展了线性模型的框架,允许响 应变量遵循非正态分布,并且可以通过一 个链接函数与解释变量建立线性关系。
2
组成
广义线性模型由三部分组成——随机成分、 系统成分和链接函数。随机成分指定响应 变量的分布类型和参数,系统成分描述解 释变量与响应变量之间的线性关系,链接 函数则将随机成分和系统成分连接起来。
06
计量经济学软件应用
EViews软件介绍及操作指南
01
EViews软件概述
EViews是一款功能强大的计量 经济学软件,广泛应用于数据 分析、模型估计和预测等领域。
02
数据导入与预处理
介绍如何在EViews中导入数据、 进行数据清洗和预处理等操作。
03
模型估计与检验
详细讲解EViews中线性回归模 型、时间序列模型等模型的估 计方法,以及模型的检验和诊 断。
THANKS
包括变量选择、模型诊断、预测等。
监督学习在计量经济学中的应用
通过训练数据集学习模型,然后利用测试数据集评估模型性能。
非监督学习在计量经济学中的应用
通过聚类、降维等技术发现数据中的潜在结构和模式。
深度学习在计量经济学中的应用
计量经济学ppt第一章
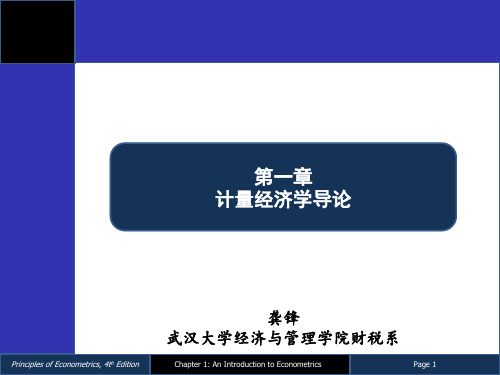
1.2 What is Econometrics About
◆计量经济学家时常被指责为:使用大铁锤去砸开花 生,却对数据不足以及成功运用这些技术所需的但却 不可靠的许多假设熟视无睹。
“计量经济理论就像仔细斟酌过的法国食谱,清楚、精确地 说明了混合调味料需要调几次,需要多少克拉的香料,以及在恰 好474度下需要多少毫秒烘烤混合物。可是,当统计学的”厨师“ 转向原材料时,却发现没有仙人掌水果的核,因此用几块哈密瓜 代替;当食谱要求采用粉条时他却用麦片;他还用绿色胡椒代替 咖喱,用鹌鹑蛋代替海龟蛋,还用一罐松脂油代替1883的 Chalifougnac。”(Valavanis,1959)
Page 5
1.1 什么是计量经济学
Principles of Econometrics, 4th Edition
Chapter 1: An Introduction to Econometrics
Page 6
1.1.1 计量经济学的概念
计量经济学( Econometrics):是经济理论、统计学和数学 的结合。
原因之三:
新的检验要求新的计量经济学方法,从
而催生新的理论的诞生。 这也提示我们,在学习计量经济学时,应回到经济学 之中,应与经济现实相结合,对感兴趣的经济理论或假
设进行检验。
Principles of Econometrics, 4th Edition
Chapter 1: An Introduction to Econometrics Page 15
Principles of Econometrics, 4th Edition
Chapter 1: An Introduction to Econometrics Page 10
- 1、下载文档前请自行甄别文档内容的完整性,平台不提供额外的编辑、内容补充、找答案等附加服务。
- 2、"仅部分预览"的文档,不可在线预览部分如存在完整性等问题,可反馈申请退款(可完整预览的文档不适用该条件!)。
- 3、如文档侵犯您的权益,请联系客服反馈,我们会尽快为您处理(人工客服工作时间:9:00-18:30)。
6
k
k
0
covariance at lag k variance
obviously when k 0, 1 0
1 1 k
plot against k, the graph we obtain is known as the population correlogram k
• Returning to the example given in figure 21.8,the value of the Q statistic up to lag 25 is about 793,the LB statistic is about 891,both are highly significant, the probability of obtaining such a high
• We can rewrite the functions above as,
Yt ( 1)Yt1 ut
(21.9.1)
• or,
• Yt Yt 1 ut
(-1.96*0.1066,1.96*0.1066) or (0.2089,0.2089)
10
• In figure 21.8,the left two lines of dots represent the 95% confidence interval.
the joint hypothesis test of k H0 : all the k are simultaneo usly equal to zero.
• This can be done by using the Q statistic developed by Box and Pierce,
m
Q n k k 1
• Where n is the sample size, and m is the lag length.
11
• Q is approximately (in large sample) distributed as chi-square distribution with m df. If Q ,df 2 we can reject the
§ 21.9 The Unit Root Test
• Consider the model as below:
Y Y u t
t 1
t
(21.3.4)
Where ut is a random error term with zero mean, constant v ariance and is serially uncorrelat ed. That is, ut is a white noise. It is an AR(1) model, if the coefficien t of Y t-1 is 1, then Y t has the unit root problem, that is, the time series Y t is nonstation ary.
4
Stochastic time series Yt has following properties:
Mean E(Yt)
Variance var(Yt) E(Yt - )2 2 Covariance k E[(Yt )(Y t k )] where k,the covariance at lag k, or autocovariance , is the
• For example, in figure 21.8, n=88,the variance is 1/88, and the
standard error is 1 0.1066 88
9
ˆ k
~
N (0, 1 ) n
(21.8.5)
The 95% confidence interval for k is
3
Example : pollen swimming in the water is a Ito process. stationary stochastic process: If a random process’s mean and variance are constant over
time and the value of covariance between the two time periods only depends on the distance or lag between the two time periods and not the actual time at which the covariance is computed, then it is stationary. actually, such process is known as weakly stationary stochastic process or wide sense stochastic process
ˆk
(Yt Y )(Ytk Y ) n
(21.8.2)
The sample variance
ˆ0
(Yt Y )2 n
(21.8.3)
The sample autocorrelation function is
ˆ k
ˆk ˆ0
(21.8.4)
8
• In Figure 21.6,we have show this correlogram up to 30 lags. Bartlett has show that if a time series is purely random, that is ,it exhibits white noise, its sample autocorrelation coefficients are approximately normally distributed with zero mean and variance equal to 1n,n is the sample size.
first step in the analysis of any time series, getting a overall impression.
2
§21.3 Stochastic Processes
Any time series data can be seen as a result generated by stochastic or random process; a concrete set of data can be seen as a particular realization of the underlying stochastic process, which is akin to the relationship between population and sample.
17
• So if we do the regression, we will get
• Yt Yt 1 ut
(21.4.1)
• And then if we find that is in fact 1,we can
say the variance Yt has one unit root.
• A time series with an unit root is a random walk (time series). Random walk is an example of nonstationary time series.
14
• Assume that:
E(ut) , E(ut )2 2 , and each ut are serially uncorrelat ed. So time series Yt is a random walk, if : Y t Yt1 ut (utis the random stock)
16
• Why ? How can we show that ?
var(Y ) E(Yt E(Yt ))2 E(Yt t)2 E( ut t)2 E((ut ))2 E[(u1 ) (u2 ) ut ]2 E(u1 )2 E(u2 )2 E(ut )2 (E(ut )(us ) 0) t 2
Generally, we only have a realization of a stochastic process,
we can only compute the sample autocorrelation function k
7
• The sample covariance at lag k
covariance between the values of Yt and Y t k,that is , between two Y values k period apart
k=0, covariance is v time serial is stationary, its mean 、variance and covariance are constant over time.
12
• An improvement of the Box-Pierce Q statistic is the LB
statistic developed by Ljung-Box.
LB
n(n
m
2) (
2 k
)
k 1 n k
~
2 m
• The LB statistic has been found to have better small-sample properties than the Q statistic.
k
k
0
covariance at lag k variance
obviously when k 0, 1 0
1 1 k
plot against k, the graph we obtain is known as the population correlogram k
• Returning to the example given in figure 21.8,the value of the Q statistic up to lag 25 is about 793,the LB statistic is about 891,both are highly significant, the probability of obtaining such a high
• We can rewrite the functions above as,
Yt ( 1)Yt1 ut
(21.9.1)
• or,
• Yt Yt 1 ut
(-1.96*0.1066,1.96*0.1066) or (0.2089,0.2089)
10
• In figure 21.8,the left two lines of dots represent the 95% confidence interval.
the joint hypothesis test of k H0 : all the k are simultaneo usly equal to zero.
• This can be done by using the Q statistic developed by Box and Pierce,
m
Q n k k 1
• Where n is the sample size, and m is the lag length.
11
• Q is approximately (in large sample) distributed as chi-square distribution with m df. If Q ,df 2 we can reject the
§ 21.9 The Unit Root Test
• Consider the model as below:
Y Y u t
t 1
t
(21.3.4)
Where ut is a random error term with zero mean, constant v ariance and is serially uncorrelat ed. That is, ut is a white noise. It is an AR(1) model, if the coefficien t of Y t-1 is 1, then Y t has the unit root problem, that is, the time series Y t is nonstation ary.
4
Stochastic time series Yt has following properties:
Mean E(Yt)
Variance var(Yt) E(Yt - )2 2 Covariance k E[(Yt )(Y t k )] where k,the covariance at lag k, or autocovariance , is the
• For example, in figure 21.8, n=88,the variance is 1/88, and the
standard error is 1 0.1066 88
9
ˆ k
~
N (0, 1 ) n
(21.8.5)
The 95% confidence interval for k is
3
Example : pollen swimming in the water is a Ito process. stationary stochastic process: If a random process’s mean and variance are constant over
time and the value of covariance between the two time periods only depends on the distance or lag between the two time periods and not the actual time at which the covariance is computed, then it is stationary. actually, such process is known as weakly stationary stochastic process or wide sense stochastic process
ˆk
(Yt Y )(Ytk Y ) n
(21.8.2)
The sample variance
ˆ0
(Yt Y )2 n
(21.8.3)
The sample autocorrelation function is
ˆ k
ˆk ˆ0
(21.8.4)
8
• In Figure 21.6,we have show this correlogram up to 30 lags. Bartlett has show that if a time series is purely random, that is ,it exhibits white noise, its sample autocorrelation coefficients are approximately normally distributed with zero mean and variance equal to 1n,n is the sample size.
first step in the analysis of any time series, getting a overall impression.
2
§21.3 Stochastic Processes
Any time series data can be seen as a result generated by stochastic or random process; a concrete set of data can be seen as a particular realization of the underlying stochastic process, which is akin to the relationship between population and sample.
17
• So if we do the regression, we will get
• Yt Yt 1 ut
(21.4.1)
• And then if we find that is in fact 1,we can
say the variance Yt has one unit root.
• A time series with an unit root is a random walk (time series). Random walk is an example of nonstationary time series.
14
• Assume that:
E(ut) , E(ut )2 2 , and each ut are serially uncorrelat ed. So time series Yt is a random walk, if : Y t Yt1 ut (utis the random stock)
16
• Why ? How can we show that ?
var(Y ) E(Yt E(Yt ))2 E(Yt t)2 E( ut t)2 E((ut ))2 E[(u1 ) (u2 ) ut ]2 E(u1 )2 E(u2 )2 E(ut )2 (E(ut )(us ) 0) t 2
Generally, we only have a realization of a stochastic process,
we can only compute the sample autocorrelation function k
7
• The sample covariance at lag k
covariance between the values of Yt and Y t k,that is , between two Y values k period apart
k=0, covariance is v time serial is stationary, its mean 、variance and covariance are constant over time.
12
• An improvement of the Box-Pierce Q statistic is the LB
statistic developed by Ljung-Box.
LB
n(n
m
2) (
2 k
)
k 1 n k
~
2 m
• The LB statistic has been found to have better small-sample properties than the Q statistic.