Middleware Support for Data Mining and Knowledge Discovery in Large-scale Distributed Infor
(完整word版)物联网技术导论模拟试题汇总(含答案)

《物联网技术导论》模拟试卷汇总一、填空题:(红色字体及下划横线为填空的答案)1.物联网就是“物物相连的互联网”。
具有两层含义:第一,物联网的核心和基础仍然是互联网,是在互联网基础上的延伸和扩展的网络;第二,其用户端延伸和扩展到了任何物品与物品之间进行信息交换和通信。
(5个空)2.吸引了百万人关注的剑桥大学特洛伊计算机实验室的咖啡壶事件发生在1991年。
(1个空)3.物联网的战略意义体现主要体现在经济价值、社会价值、国家安全及科技发展需求。
(4个空)4.物联网的主要应用领域有:工业与自动化控制、智能物流、智能交通、智能电网、智能医疗、智能农业、智能环保、国防军事、金融与服务、智能家具等。
(10个空)5.物联网定义是指通过射频识别(RFID)、红外感应器、全球地位系统、激光扫描器等信息传感设备,按约定的协议。
把任何物体与互联网连接起来,进行信息交换和通信,以实现智能化识别、定位、跟踪、监控和管理的一种网络。
(5个空)6.物联网至少应该具备三个关键特征:一是,各类终端实现“全面感知”;二是,电信网、互联网等融合实现“可靠传输”;三是,云计算等技术对海量数据“智慧处理”。
(3个空)7.RFID即射频识别,常用的工作频段和工作特点按下表分类,并对表格中的空挡给予填写(12个空)8.传感器是把非电学物理量转换成易于测量、传输、处理的电学量(如电压、电流、电容等)的一种元件。
常见的传感器包括温度、光电、压力、湿度、加速度、霍尔磁性传感器等。
(7个空)9.当今移动通信处于2G、3G、4G 三代技术并存,2G主要代表是GSM和CDMA;3G主要代表是中国TD-SCDMA、欧洲WCDMA、美国cdma2000和全球微波互联接入(WiMAX)、4G主要代表是LTE和全球微波互联接入(WiMAX)。
(8个空)10.典型的短距离无线通信网络协议有WiFi (802.11,Wireless Fidelity,无线局域网)、蓝牙(802.15.1协议)、紫蜂ZigBee(802.15.4协议)、IrDA(Infrared Data Association,红外线数据协会)无线协议及WSN(wireless sensor network,无线传感器网络)等无线低速网络技术。
数据挖掘导论英文版
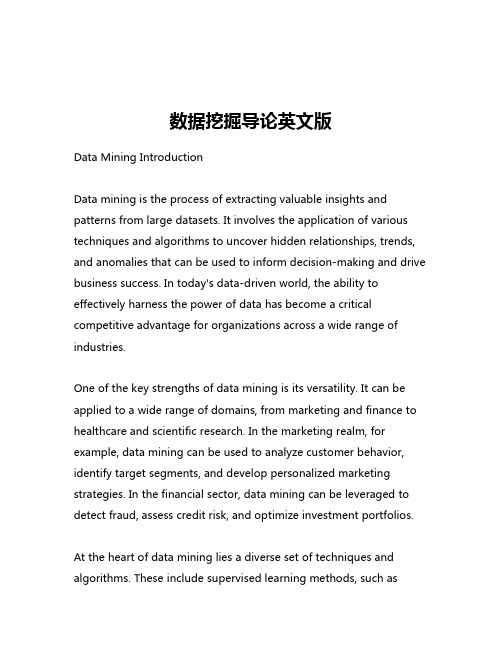
数据挖掘导论英文版Data Mining IntroductionData mining is the process of extracting valuable insights and patterns from large datasets. It involves the application of various techniques and algorithms to uncover hidden relationships, trends, and anomalies that can be used to inform decision-making and drive business success. In today's data-driven world, the ability to effectively harness the power of data has become a critical competitive advantage for organizations across a wide range of industries.One of the key strengths of data mining is its versatility. It can be applied to a wide range of domains, from marketing and finance to healthcare and scientific research. In the marketing realm, for example, data mining can be used to analyze customer behavior, identify target segments, and develop personalized marketing strategies. In the financial sector, data mining can be leveraged to detect fraud, assess credit risk, and optimize investment portfolios.At the heart of data mining lies a diverse set of techniques and algorithms. These include supervised learning methods, such asregression and classification, which can be used to predict outcomes based on known patterns in the data. Unsupervised learning techniques, such as clustering and association rule mining, can be employed to uncover hidden structures and relationships within datasets. Additionally, advanced algorithms like neural networks and decision trees have proven to be highly effective in tackling complex, non-linear problems.The process of data mining typically involves several key steps, each of which plays a crucial role in extracting meaningful insights from the data. The first step is data preparation, which involves cleaning, transforming, and integrating the raw data into a format that can be effectively analyzed. This step is particularly important, as the quality and accuracy of the input data can significantly impact the reliability of the final results.Once the data is prepared, the next step is to select the appropriate data mining techniques and algorithms to apply. This requires a deep understanding of the problem at hand, as well as the strengths and limitations of the available tools. Depending on the specific goals of the analysis, the data mining practitioner may choose to employ a combination of techniques, each of which can provide unique insights and perspectives.The next phase is the actual data mining process, where the selectedalgorithms are applied to the prepared data. This can involve complex mathematical and statistical calculations, as well as the use of specialized software and computing resources. The results of this process may include the identification of patterns, trends, and relationships within the data, as well as the development of predictive models and other data-driven insights.Once the data mining process is complete, the final step is to interpret and communicate the findings. This involves translating the technical results into actionable insights that can be easily understood by stakeholders, such as business leaders, policymakers, or scientific researchers. Effective communication of data mining results is crucial, as it enables decision-makers to make informed choices and take appropriate actions based on the insights gained.One of the most exciting aspects of data mining is its continuous evolution and the emergence of new techniques and technologies. As the volume and complexity of data continue to grow, the need for more sophisticated and powerful data mining tools and algorithms has become increasingly pressing. Advances in areas such as machine learning, deep learning, and big data processing have opened up new frontiers in data mining, enabling practitioners to tackle increasingly complex problems and extract even more valuable insights from the data.In conclusion, data mining is a powerful and versatile tool that has the potential to transform the way we approach a wide range of challenges and opportunities. By leveraging the power of data and the latest analytical techniques, organizations can gain a deeper understanding of their operations, customers, and markets, and make more informed, data-driven decisions that drive sustainable growth and success. As the field of data mining continues to evolve, it is clear that it will play an increasingly crucial role in shaping the future of business, science, and society as a whole.。
数据挖掘与数据分析,数据可视化试题
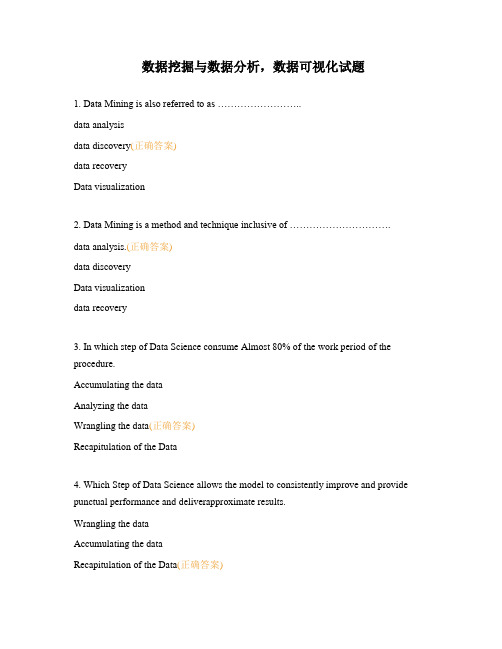
数据挖掘与数据分析,数据可视化试题1. Data Mining is also referred to as ……………………..data analysisdata discovery(正确答案)data recoveryData visualization2. Data Mining is a method and technique inclusive of …………………………. data analysis.(正确答案)data discoveryData visualizationdata recovery3. In which step of Data Science consume Almost 80% of the work period of the procedure.Accumulating the dataAnalyzing the dataWrangling the data(正确答案)Recapitulation of the Data4. Which Step of Data Science allows the model to consistently improve and provide punctual performance and deliverapproximate results.Wrangling the dataAccumulating the dataRecapitulation of the Data(正确答案)Analyzing the data5. Which tool of Data Science is robust machine learning library, which allows the implementation of deep learning ?algorithms. STableauD3.jsApache SparkTensorFlow(正确答案)6. What is the main aim of Data Mining ?to obtain data from a less number of sources and to transform it into a more useful version of itself.to obtain data from a less number of sources and to transform it into a less useful version of itself.to obtain data from a great number of sources and to transform it into a less useful version of itself.to obtain data from a great number of sources and to transform it into a more useful version of itself.(正确答案)7. In which step of data mining the irrelevant patterns are eliminated to avoid cluttering ? Cleaning the data(正确答案)Evaluating the dataConversion of the dataIntegration of data8. Data Science t is mainly used for ………………. purposes. Data mining is mainly used for ……………………. purposes.scientific,business(正确答案)business,scientificscientific,scientificNone9. Pandas ………………... is a one dimensional labeled array capable of holding data of any type (integer, string, float, python objects, etc.).Series(正确答案)FramePanelNone10. How many principal components Pandas DataFrame consists of ?4213(正确答案)11. Important data structure of pandas is/are ___________SeriesData FrameBoth(正确答案)None of the above12. Which of the following command is used to install pandas?pip install pandas(正确答案)install pandaspip pandasNone of the above13. Which of the following function/method help to create Series? series()Series()(正确答案)createSeries()None of the above14. NumPY stands for?Numbering PythonNumber In PythonNumerical Python(正确答案)None Of the above15. Which of the following is not correct sub-packages of SciPy? scipy.integratescipy.source(正确答案)scipy.interpolatescipy.signal16. How to import Constants Package in SciPy?import scipy.constantsfrom scipy.constants(正确答案)import scipy.constants.packagefrom scipy.constants.package17. ………………….. involveslooking at and describing the data set from different angles and then summarizing it ?Data FrameData VisualizationEDA(正确答案)All of the above18. what involves the preparation of data sets for analysis by removing irregularities in the data so that these irregularities do not affect further steps in the process of data analysis and machine learning model building ?Data AnalysisEDA(正确答案)Data FrameNone of the above19. What is not Utility of EDA ?Maximize the insight in the data setDetect outliers and anomaliesVisualization of dataTest underlying assumptions(正确答案)20. what can hamper the further steps in the machine learning model building process If not performed properly ?Recapitulation of the DataAccumulating the dataEDA(正确答案)None of the above21. Which plot for EDA to check the dependency between two variables ? HistogramsScatter plots(正确答案)MapsTime series plots22. What function will tell you the top records in the data set?shapehead(正确答案)showall of the aboce23. what type of data is useful for internal policymaking and business strategy building for an organization ?public dataprivate data(正确答案)bothNone of the above24. The ………… function can “fill in” NA valueswith non-null data ?headfillna(正确答案)shapeall of the above25. If you want to simply exclude the missing values, then what function along with the axis argument will be use?fillnareplacedropna(正确答案)isnull26. Which of the following attribute of DataFrame is used to display data type of each column in DataFrame?DtypesDTypesdtypes(正确答案)datatypes27. Which of the following function is used to load the data from the CSV file into a DataFrame?read.csv()readcsv()read_csv()(正确答案)Read_csv()28. how to Display first row of dataframe ‘DF’ ?print(DF.head(1))print(DF[0 : 1])print(DF.iloc[0 : 1])All of the above(正确答案)29. Spread function is known as ................ in spreadsheets ?pivotunpivot(正确答案)castorder30. ................. extract a subset of rows from a data fram based on logical conditions ? renamefilter(正确答案)setsubset31. We can shift the DataFrame’s index by a certain number of periods usingthe …………. Method ?melt()merge()tail()shift()(正确答案)32. We can join melted DataFrames into one Analytical Base Table using the ……….. function.join()append()merge()(正确答案)truncate()33. What methos is used to concatenate datasets along an axis ?concatenate()concat()(正确答案)add()merge()34. Rows can be …………….. if the number of missing values is insignificant, as thiswould not impact the overall analysis results.deleted(正确答案)updatedaddedall35. There is a specific reason behind the missing value.What stands for Missing not at randomMCARMARMNAR(正确答案)None of the above36. While plotting data, some values of one variable may not lie beyond the expectedrange, but when you plot the data with some other variable, these values may lie far from the expected value.Identify the type of outliers?Univariate outliersMultivariate outliers(正确答案)ManyVariate outlinersNone of the above37. if numeric values are stored as strings, then it would not be possible to calculatemetrics such as mean, median, etc.Then what type of data cleaning exercises you will perform ?Convert incorrect data types:(正确答案)Correct the values that lie beyond the rangeCorrect the values not belonging in the listFix incorrect structure:38. Rows that are not required in the analysis. E.g ifobservations before or after a particular date only are required for analysis.What steps we will do when perform data filering ?Deduplicate Data/Remove duplicateddataFilter rows tokeep only therelevant data.(正确答案)Filter columns Pick columnsrelevant toanalysisBring the datatogether, Groupby required keys,aggregate therest39. you need to…………... the data in order to get what you need for your analysis. searchlengthorderfilter(正确答案)40. Write the output of the following ?>>> import pandas as pd >>> series1 =pd.Series([10,20,30])>>> print(series1)0 101 202 30dtype: int64(正确答案)102030dtype: int640 1 2 dtype: int64None of the above41. What will be output for the following code?import numpy as np a = np.array([1, 2, 3], dtype = complex) print a[[ 1.+0.j, 2.+0.j, 3.+0.j]][ 1.+0.j]Error[ 1.+0.j, 2.+0.j, 3.+0.j](正确答案)42. What will be output for the following code?import numpy as np a =np.array([1,2,3]) print a[[1, 2, 3]][1][1, 2, 3](正确答案)Error43. What will be output for the following code?import numpy as np dt = dt =np.dtype('i4') print dtint32(正确答案)int64int128int1644. What will be output for the following code?import numpy as np dt =np.dtype([('age',np.int8)]) a = np.array([(10,),(20,),(30,)], dtype = dt)print a['age'][[10 20 30]][10 20 30](正确答案)[10]Error45. We can add a new row to a DataFrame using the _____________ methodrloc[ ]iloc[ ]loc[ ](正确答案)None of the above46. Function _____ can be used to drop missing values.fillna()isnull()dropna()(正确答案)delna()47. The function to perform pivoting with dataframes having duplicate values is _____ ? pivot(unique = True)pivot()pivot_table(unique = True)pivot_table()(正确答案)48. A technique, which when performed on a dataframe, rearranges the data from rows and columns in a report form, is called _____ ?summarisingreportinggroupingpivoting(正确答案)49. Normal Distribution is symmetric is about ___________ ?VarianceMean(正确答案)Standard deviationCovariance50. Write a statement to display “Amount” as x-axis label. (consider plt as an alias name of matplotlib.pyplot)bel(“Amount”)plt.xlabel(“Amount”)(正确答案)plt.xlabel(Amount)None of the above51. Fill in the blank in the given code, if we want to plot a line chart for values of list ‘a’ vs values of list ‘b’.a = [1, 2, 3, 4, 5]b = [10, 20, 30, 40, 50]import matplotlib.pyplot as pltplt.plot __________(a, b)(正确答案)(b, a)[a, b]None of the above52. #Loading the datasetimport seaborn as snstips =sns.load_dataset("tips")tips.head()In this code what is tips ?plotdataset name(正确答案)paletteNone of the above53. Visualization can make sense of information by helping to find relationships in the data and support (or disproving) ideas about the dataAnalyzeRelationShip(正确答案)AccessiblePrecise54. In which option provides A detailed data analysis tool that has an easy-to-use tool interface and graphical designoptions for visuals.Jupyter NotebookSisenseTableau DesktopMATLAB(正确答案)55. Consider a bank having thousands of ATMs across China. In every transaction, Many variables are recorded.Which among the following are not fact variables.Transaction charge amountWithdrawal amountAccount balance after withdrawalATM ID(正确答案)56. Which module of matplotlib library is required for plotting of graph?plotmatplotpyplot(正确答案)None of the above57. Write a statement to display “Amount” as x-axis label. (consider plt as an alias name of matplotlib.pyplot)bel(“Amount”)plt.xlabel(“Amount”)(正确答案)plt.xlabel(Amount)None of the above58. What will happen when you pass ‘h’ as as a value to orient parameter of the barplot function?It will make the orientation vertical.It will make the orientation horizontal.(正确答案)It will make line graphNone of the above59. what is the name of the function to display Parameters available are viewed .set_style()axes_style()(正确答案)despine()show_style()60. In stacked barplot, subgroups are displayed as bars on top of each other. How many parameters barplot() functionhave to draw stacked bars?OneTwoNone(正确答案)three61. In Line Chart or Line Plot which parameter is an object determining how to draw the markers for differentlevels of the style variable.?x.yhuemarkers(正确答案)legend62. …………………..similar to Box Plot but with a rotated plot on each side, giving more information about the density estimate on the y axis.Pie ChartLine ChartViolin Chart(正确答案)None63. By default plot() function plots a ________________HistogramBar graphLine chart(正确答案)Pie chart64. ____________ are column-charts, where each column represents a range of values, and the height of a column corresponds to how many values are in that range.Bar graphHistograms(正确答案)Line chartpie chart65. The ________ project builds on top of pandas and matplotlib to provide easy plotting of data.yhatSeaborn(正确答案)VincentPychart66. A palette means a ________.. surface on which a painter arranges and mixed paints. circlerectangularflat(正确答案)all67. The default theme of the plotwill be ________?Darkgrid(正确答案)WhitegridDarkTicks68. Outliers should be treated after investigating data and drawing insights from a dataset.在调查数据并从数据集中得出见解后,应对异常值进行处理。
信息系统项目管理师、系统集成项目管理工程师考试常见英语单词汇总
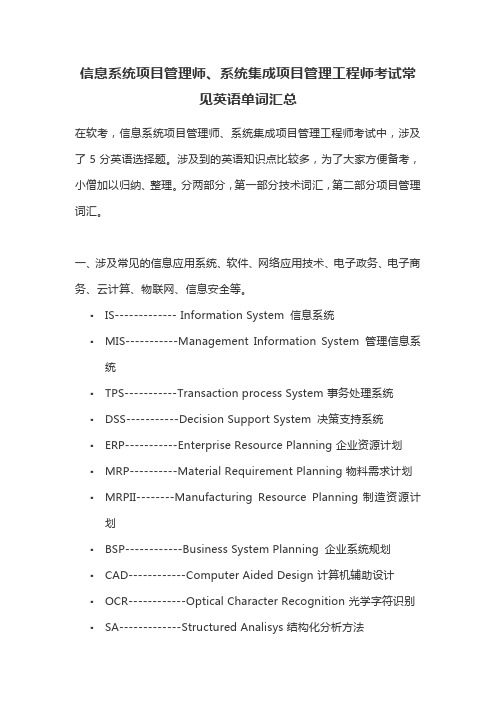
信息系统项目管理师、系统集成项目管理工程师考试常见英语单词汇总在软考,信息系统项目管理师、系统集成项目管理工程师考试中,涉及了5分英语选择题。
涉及到的英语知识点比较多,为了大家方便备考,小僧加以归纳、整理。
分两部分,第一部分技术词汇,第二部分项目管理词汇。
一、涉及常见的信息应用系统、软件、网络应用技术、电子政务、电子商务、云计算、物联网、信息安全等。
▪IS------------- Information System 信息系统▪MIS-----------Management Information System 管理信息系统▪TPS-----------Transaction process System 事务处理系统▪DSS-----------Decision Support System 决策支持系统▪ERP-----------Enterprise Resource Planning 企业资源计划▪MRP----------Material Requirement Planning 物料需求计划▪MRPII--------Manufacturing Resource Planning 制造资源计划▪BSP------------Business System Planning 企业系统规划▪CAD------------Computer Aided Design 计算机辅助设计▪OCR------------Optical Character Recognition 光学字符识别▪SA-------------Structured Analisys 结构化分析方法▪OOA----------Object-Oriented Analysis 面向对象分析方法▪OOD----------Object-Oriented Design 面向对象设计▪OOP----------Object Oriented Programming 面向对象编程▪DFD----------Data Flow Diagram 数据流图▪DD------------Data Dictionary 数据字典▪E-R-----------Entity Relationship Diagram E-R图▪OLAP--------On-Line Analytical Processing 在线联机分析处理▪OLTP--------On-Line Transaction Processing 联机事物处理系统▪EDI-----------Electronic Data Interchange 电子数据交换▪CRM---------Customer Relationship Management 客户关系管理▪SCM----------Supply Chain Management 供应链管理▪Data Mining----------数据挖掘▪Data Warehouse----------数据仓库▪Database----------数据库▪Data Mart----------数据集市▪ITIL----------Information Technology Infrastructure Library 信息技术基础架构库▪ITSM----------IT Service Management IT服务管理▪Message----------消息▪Communication-----------消息通信▪UML----------Unified Modeling Language 统一建模语言▪Use case diagram----------用例图▪Class diagram----------类图▪Object diagram----------对象图▪Component diagram----------构件图▪Deployment diagram----------部署图▪State diagram----------状态图▪Sequence diagram----------序列图▪Collaboration diagram----------协作图▪Activity diagram----------活动图▪C/S---------- Client/Server 客户机/服务器▪B/S---------- Browser/Server 浏览器/服务器▪SOA----------Service Oriented Architecture 面向服务的体系结构▪Middleware----------中间件▪RPC----------Remote Procedure Call 远程过程调用▪Web Services----------Web服务▪SOAP----------Simple Object Access Protocol 简单对象访问协议▪WSDL----------Web Services Description Language Web服务描述语言▪UDDI----------Universal Description Discovery and Integration 通用描述、发现与集成服务▪XML-----------Extensible Markup Language 可扩展标记语言▪HTML----------Hypertext Markup Language 超文本标记语言▪Component----------构建▪Containe----------容器▪WorkFlow----------工作流▪WFMS---------- Workflow Management System 工作流管理系统▪CORBA---------- Common Object Request Broker Architecture 公共对象请求代理体系结构▪OMG---------- Object Management Group 对象管理组织▪DCOM-----------Distributed Component Object Model 分布式构件对象模型▪API-----------Application Programming Interface 应用程序编程接口▪Graphical User Interface-----------图形用户界面▪Logic View----------逻辑视图▪Development View----------开发视图▪Module View----------模块视图▪Process View----------进程视图▪Physical View----------物理视图▪Attribute----------属性▪Object----------对象▪Class----------类▪Inheritance----------继承▪Dependency----------依赖▪Generalization----------泛化▪Aggregation----------聚合▪Composite----------组合▪Association----------关联▪function---------函数▪template---------模板▪LAN---------- Local Area Network 局域网▪Ethernet----------以太网▪Token King----------令牌环网▪WAN----------- Wide Area Network 广域网▪Proxy----------代理▪Server----------服务器▪Workstation----------工作站▪Bridge-----------网桥▪Router----------路由器▪Gateway----------网关▪OSI----------Open System Interconnect 开放式互联系统▪Physical Layer----------物理层▪Datalink Layer----------数据链路层▪Network Layer----------网络层▪Transport Layer----------传输层▪Session Layer----------会话层▪Presentation Layer---------表示层▪Application Layer---------应用层▪Virus---------病毒▪Firewall---------防火墙▪Directory structure--------- 目录结构▪TCP----------Transmission Control Protocol 传输控制协议▪UDP----------User Datagram Protocol 用户数据包协议▪ARP----------Address Resolution Protocol 地址解析协议▪URL----------Uniform Resource Locator 统一资源定位器▪FTP----------File Transfer Protocol 文件传输协议▪DHCP----------Dynamic Host Configuration Protocol 动态主机设置协议▪PPTP---------- Point to Point Tunneling Protocol 点对点协议▪ATM----------Asynchronous Transfer Mode 异步传输模式▪DAS----------Direct-Attached Storage 直接连接存储▪NAS---------- Network Attached Storage 网络连接存储▪SAN---------- Storage Area Network 存储区域网络▪PDS----------Premises Distribution System 综合布线系统▪Work Area Subsystem---------- 工作区子系统▪Horizontal Backbone Subsystem---------- 水平干线子系统▪Administrator Subsystem---------- 设备间子系统▪Backbone Subsystem---------- 垂直干线子系统▪Campus Backbone Subsystem---------- 楼宇▪Equipment Room Subsystem----------设备间子系统▪SQA----------Software Quality Assurance软件质量保证▪Performance----------性能▪Reliability----------可靠性▪Availability----------可用性▪Security----------安全性▪Modifiability----------可修改性▪Maintainability----------可维护性▪Extendibility----------可扩展性▪Reassemble----------结构重组▪Portability---------可移植性▪Functionality--------功能性▪FDMA----------Frequency Division Multiple Access 频分多址▪WDMA---------Wave Division Multiple Access 频分多址▪TDMA----------Time Division Multiple Access 时分多址▪CDMA----------Code Division Multiple Access 码分多址▪ADSL----------Asymmetric Digital Subscriber Line 非对称数字用户环境▪HDSL----------High-speed Digital Subscriber Line 高速率数字用户体验▪VDSL----------Very-high-bit-rate Digital Subscriber loop 甚高速数字用户环境。
Introduction to Data Mining

Introduction to Data MiningData mining is a process of extracting useful information from large datasets by using various statistical and machine learning techniques. It is a crucial part of the field of data science and plays a key role in helping businesses make informed decisions based on data-driven insights.One of the main goals of data mining is to discover patterns and relationships within data that can be used to make predictions or identify trends. This can help businesses improve their marketing strategies, optimize their operations, and better understand their customers. By analyzing large amounts of data, data mining algorithms can uncover hidden patterns that may not be immediately apparent to human analysts.There are several different techniques that are commonly used in data mining, including classification, clustering, association rule mining, and anomaly detection. Classification involves categorizing data points into different classes based on their attributes, while clustering groups similar data points together. Association rule mining identifies relationships between different variables, and anomaly detection detects outliers or unusual patterns in the data.In order to apply data mining techniques effectively, it is important to have a solid understanding of statistics, machine learning, and data analytics. Data mining professionals must be able to preprocess data, select appropriate algorithms, and interpret the results of their analyses. They must also be able to communicate their findings effectively to stakeholders in order to drive business decisions.Data mining is used in a wide range of industries, including finance, healthcare, retail, and telecommunications. In finance, data mining is used to detect fraudulent transactions and predict market trends. In healthcare, it is used to analyze patient data and improve treatment outcomes. In retail, it is used to optimize inventory management and personalize marketing campaigns. In telecommunications, it is used to analyze network performance and customer behavior.Overall, data mining is a powerful tool that can help businesses gain valuable insights from their data and make more informed decisions. By leveraging the latest advances in machine learning and data analytics, organizations can stay competitive in today's data-driven world. Whether you are a data scientist, analyst, or business leader, understanding the principles of data mining can help you unlock the potential of your data and drive success in your organization.。
1. Data Mining – Practical Machine Learning Tools and Techniques with Java
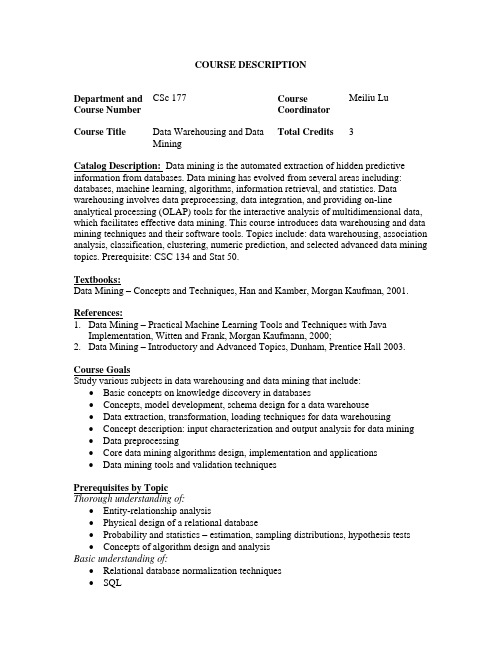
COURSE DESCRIPTIONDepartment and Course Number CSc 177 CourseCoordinatorMeiliu LuCourse Title Data Warehousing and DataMiningTotal Credits 3Catalog Description: Data mining is the automated extraction of hidden predictive information from databases. Data mining has evolved from several areas including: databases, machine learning, algorithms, information retrieval, and statistics. Data warehousing involves data preprocessing, data integration, and providing on-line analytical processing (OLAP) tools for the interactive analysis of multidimensional data, which facilitates effective data mining. This course introduces data warehousing and data mining techniques and their software tools. Topics include: data warehousing, association analysis, classification, clustering, numeric prediction, and selected advanced data mining topics. Prerequisite: CSC 134 and Stat 50.Textbooks:Data Mining – Concepts and Techniques, Han and Kamber, Morgan Kaufman, 2001.References:1.Data Mining – Practical Machine Learning Tools and Techniques with JavaImplementation, Witten and Frank, Morgan Kaufmann, 2000;2.Data Mining – Introductory and Advanced Topics, Dunham, Prentice Hall 2003.Course GoalsStudy various subjects in data warehousing and data mining that include: •Basic concepts on knowledge discovery in databases•Concepts, model development, schema design for a data warehouse•Data extraction, transformation, loading techniques for data warehousing•Concept description: input characterization and output analysis for data mining •Data preprocessing•Core data mining algorithms design, implementation and applications•Data mining tools and validation techniquesPrerequisites by TopicThorough understanding of:•Entity-relationship analysis•Physical design of a relational database•Probability and statistics – estimation, sampling distributions, hypothesis tests •Concepts of algorithm design and analysisBasic understanding of:•Relational database normalization techniques•SQLExposure to:•Bayesian theory•RegressionMajor Topics Covered in the Course1.Introduction to the process of knowledge discovery in databases2.Basic concepts of data warehousing and data mining3.Data preprocessing techniques: selection, extraction, transformation, loading4.Data warehouse design and implementation: multidimensional data model, casestudy using Oracle technology5.Machine learning schemes in data mining: finding and describing structure patterns(models) in data, informing future decisionsrmation theory and statistics in data mining: from entropy to regression7.Data mining core algorithms: statistical modeling, classification, clustering,association analysis8.Credibility: evaluating what has been leaned from training data and predictingmodel performance on new data, evaluation methods, and evaluation metrics9.Weka: a set of commonly used machine learning algorithms implemented in Javafor data mining10.C5 and Cubist: Decision tree and model tree based data mining tools11.Selected advance topics based on students’ interests such as: web mining, textmining, statistical learning12.Case studies of real data mining applications (paper survey and invited speaker) Laboratory Projects1.Design and implement a data warehouse database (4 weeks)2.Explore extraction, transformation, loading tasks in data warehousing (1 week)3.Explore data mining tools and algorithms implementation (3 weeks)4.Design and implement data mining application (3 weeks)Expected OutcomesThorough understanding of:•Process and tasks for Knowledge discovery in databases.•Differences between a data warehouses OLAP and operational databases OLTP.•Multidimensional data model design and development.•Techniques for data extraction, transformation, and loading.•Machine learning schemes in data mining.•Mining association rules (Apriori).•Classification and prediction (Statistical based: Naïve Bayes, regression trees and model trees; Distance based: KNN, Decision tree based: 1R, ID3, CART;Covering algorithm: Prism).•Cluster analysis (Hierarchical algorithms: single link, average link, and complete link; Partitional algorithms: MST, K-means; Probability based algorithm: EM).•Use of data mining tools: C5, Cubist, Weka.Basic understanding of:•Data warehouse architecture.•Information theory and statistics in data mining.•Credibility analysis and performance evaluation.Exposure to:•Mining complex types of data: multimedia, spatial, and temporal•Statistical learning theory•Support vector machine and ANNEstimated CSAB Category ContentCORE ADVANCED CORE ADVANCEDDataStructures .2Algorithms 1 Computer Org & ArchitectureSoftwareDesign .5 Concepts ofProgrammingLanguages .2Oral and Written Communications1.Three written reports (term project proposal, research paper review, and termproject report).2.Two oral presentations (10 minutes for paper review and 15-20 minutes for termproject).Social and Ethical IssuesNo significant component.Theoretical Content1.Data warehouse schema and data cube computation.rmation theory and statistics in data mining.3.Data mining algorithms and their output model performance prediction.4.Evaluation metrics (confusion matrix, cost matrix, F measure, ROC curve). Analysis and Design1.Design of a data warehouse.2.Design of a process of ETL (Extraction, Transformation, Loading).3.Design of a data mining application.4.Analysis of performance of a data warehouse.5.Analysis and comparison of data mining schema.CSC 17711-04。
计算机科学与技术学院申请博士学位发表学术论文的规定(2008.9上网)
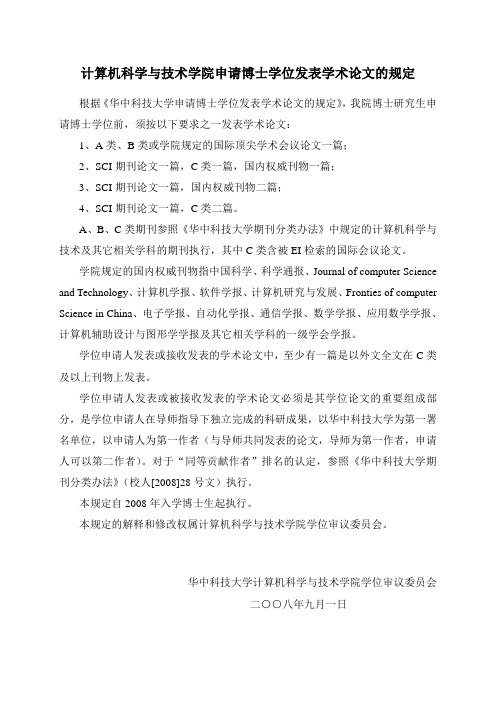
计算机科学与技术学院申请博士学位发表学术论文的规定根据《华中科技大学申请博士学位发表学术论文的规定》,我院博士研究生申请博士学位前,须按以下要求之一发表学术论文:1、A类、B类或学院规定的国际顶尖学术会议论文一篇;2、SCI期刊论文一篇,C类一篇,国内权威刊物一篇;3、SCI期刊论文一篇,国内权威刊物二篇;4、SCI期刊论文一篇,C类二篇。
A、B、C类期刊参照《华中科技大学期刊分类办法》中规定的计算机科学与技术及其它相关学科的期刊执行,其中C类含被EI检索的国际会议论文。
学院规定的国内权威刊物指中国科学、科学通报、Journal of computer Science and Technology、计算机学报、软件学报、计算机研究与发展、Fronties of computer Science in China、电子学报、自动化学报、通信学报、数学学报、应用数学学报、计算机辅助设计与图形学学报及其它相关学科的一级学会学报。
学位申请人发表或接收发表的学术论文中,至少有一篇是以外文全文在C类及以上刊物上发表。
学位申请人发表或被接收发表的学术论文必须是其学位论文的重要组成部分,是学位申请人在导师指导下独立完成的科研成果,以华中科技大学为第一署名单位,以申请人为第一作者(与导师共同发表的论文,导师为第一作者,申请人可以第二作者)。
对于“同等贡献作者”排名的认定,参照《华中科技大学期刊分类办法》(校人[2008]28号文)执行。
本规定自2008年入学博士生起执行。
本规定的解释和修改权属计算机科学与技术学院学位审议委员会。
华中科技大学计算机科学与技术学院学位审议委员会二○○八年九月一日为提高研究生培养质量、提高学术水平、促进国际学术交流,经计算机学院学位审议委员会研究决定,国际顶尖学术会议分为A、B两类,分类如下:一、A类1. International Conference on Architectural Support for ProgrammingLanguages and Operating Systems (ASPLOS)2. ACM Conference on Computer and Communication Security (CCS)3. USENIX Conference on File and Storage Techniques (FAST)4. International Symposium on High Performance ComputerArchitecture (HPCA)5. International Conference on Software Engineering (ICSE)6. International Symposium on Computer Architecture (ISCA)7. USENIX Conference on Operating System and Design (OSDI)8. ACM SIGCOMM Conference (SIGCOMM)9. ACM Annual International ACM SIGIR Conference on Research andDevelopment in Information Retrieval (SIGIR)10. International Conference on Management of Data and Symposium onPrinciples of Database Systems (SIGMOD/PODS)11. ACM Symposium on Operating Systems Principles (SOSP)12. Annual ACM Symposium on Theory of Computing (STOC)13. USENIX Annual Technical Conference (USENIX)14. ACM International Conference on Virtual Execution Environments(VEE)15. International Conference on Very Large Data Bases (VLDB)二、B类1. International Conference on Dependable Systems and Networks (DSN)2. IEEE Symposium on Foundations of Computer Science (FOCS)3. IEEE International Symposium on High Performance DistributedComputing (HPDC)4. International Conference on Distributed Computing Systems (ICDCS)5. International Conference on Data Engineering (ICDE)6. IEEE International Conference on Network Protocols (ICNP)7. ACM International Conference on Supercomputing (ICS)8. International Joint Conference on Artificial Intelligence (IJCAI)9. IEEE Conference on Computer Communications (INFOCOM)10. ACM SIGKDD International Conference on Knowledge Discovery andData Mining (KDD)11. Annual IEEE/ACM International Symposium on Microarchitecture(MICRO)12. ACM/IFIP/USENIX International Middleware Conference (Middleware)13. ACM International Conference on Multimedia (MM)14. ACM International Conference on Mobile Systems, Applications, andServices (MobiSys)15. ACM Conference on Programming Language Design andImplementation (PLDI)16. Annual ACM Symposium on Principles of Distributed Computing(PODC)17. ACM Symposium on Principles of Programming Languages (POPL)18. ACM SIGPLAN Symposium on Principles and Practice of ParallelProgramming (PPoPP)19. IEEE Real-Time Systems Symposium (RTSS)20. Supercomputing (SC'XY) Conference21. ACM Conference on Computer Graphics and Interactive Techniques(SIGGRAPH)22. ACM Conference on Measurement and Modeling of ComputerSystems (SIGMETRICS)23. IEEE Symposium on Security and Privacy (SP)24. Annual ACM Symposium on Parallel Algorithms and Architectures(SPAA)25. International World Wide Web Conference (WWW)华中科技大学计算机科学与技术学院学位审议委员会二○○八年十一月十七日计算机学院资助教师和学生参加顶尖国际学术会议试行办法院办字[2006]06号为了促进计算机学院师生开展国际学术交流,提高学术水平,经第75次学院办公会议研究,并经第2次教授咨询委员会咨询,学院资助教师和在校学生参加顶尖国际学术会议,制定本办法。
数据挖掘data mining 核心专业词汇
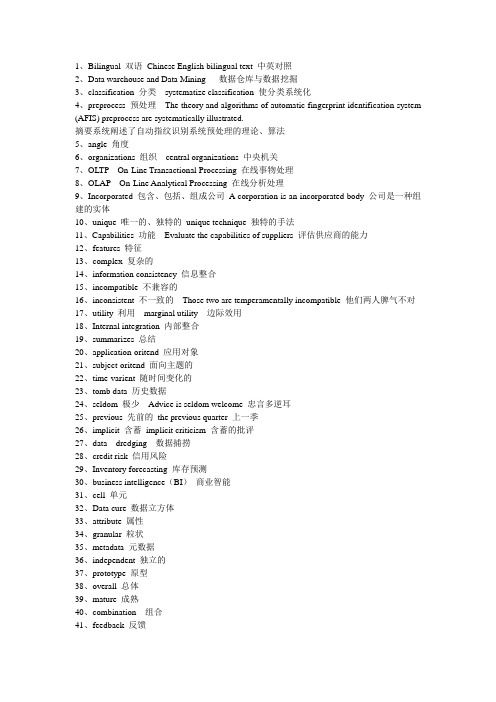
1、Bilingual 双语Chinese English bilingual text 中英对照2、Data warehouse and Data Mining 数据仓库与数据挖掘3、classification 分类systematize classification 使分类系统化4、preprocess 预处理The theory and algorithms of automatic fingerprint identification system (AFIS) preprocess are systematically illustrated.摘要系统阐述了自动指纹识别系统预处理的理论、算法5、angle 角度6、organizations 组织central organizations 中央机关7、OLTP On-Line Transactional Processing 在线事物处理8、OLAP On-Line Analytical Processing 在线分析处理9、Incorporated 包含、包括、组成公司A corporation is an incorporated body 公司是一种组建的实体10、unique 唯一的、独特的unique technique 独特的手法11、Capabilities 功能Evaluate the capabilities of suppliers 评估供应商的能力12、features 特征13、complex 复杂的14、information consistency 信息整合15、incompatible 不兼容的16、inconsistent 不一致的Those two are temperamentally incompatible 他们两人脾气不对17、utility 利用marginal utility 边际效用18、Internal integration 内部整合19、summarizes 总结20、application-oritend 应用对象21、subject-oritend 面向主题的22、time-varient 随时间变化的23、tomb data 历史数据24、seldom 极少Advice is seldom welcome 忠言多逆耳25、previous 先前的the previous quarter 上一季26、implicit 含蓄implicit criticism 含蓄的批评27、data dredging 数据捕捞28、credit risk 信用风险29、Inventory forecasting 库存预测30、business intelligence(BI)商业智能31、cell 单元32、Data cure 数据立方体33、attribute 属性34、granular 粒状35、metadata 元数据36、independent 独立的37、prototype 原型38、overall 总体39、mature 成熟40、combination 组合41、feedback 反馈42、approach 态度43、scope 范围44、specific 特定的45、data mart 数据集市46、dependent 从属的47、motivate 刺激、激励Motivate and withstand higher working pressure个性积极,愿意承受压力.敢于克服困难48、extensive 广泛49、transaction 交易50、suit 诉讼suit pending 案件正在审理中51、isolate 孤立We decided to isolate the patients.我们决定隔离病人52、consolidation 合并So our Party really does need consolidation 所以,我们党确实存在一个整顿的问题53、throughput 吞吐量Design of a Web Site Throughput Analysis SystemWeb网站流量分析系统设计收藏指正54、Knowledge Discovery(KDD)55、non-trivial(有价值的)--Extraction interesting (non-trivial(有价值的), implicit(固有的), previously unknown and potentially useful) patterns or knowledge from huge amounts of data.56、archeology 考古57、alternative 替代58、Statistics 统计、统计学population statistics 人口统计59、feature 特点A facial feature 面貌特征60、concise 简洁a remarkable concise report 一份非常简洁扼要的报告61、issue 发行issue price 发行价格62、heterogeneous (异类的)--Constructed by integrating multiple, heterogeneous (异类的)data sources63、multiple 多种Multiple attachments多实习64、consistent(一贯)、encode(编码)ensure consistency in naming conventions,encoding structures, attribute measures, etc.确保一致性在命名约定,编码结构,属性措施,等等。
- 1、下载文档前请自行甄别文档内容的完整性,平台不提供额外的编辑、内容补充、找答案等附加服务。
- 2、"仅部分预览"的文档,不可在线预览部分如存在完整性等问题,可反馈申请退款(可完整预览的文档不适用该条件!)。
- 3、如文档侵犯您的权益,请联系客服反馈,我们会尽快为您处理(人工客服工作时间:9:00-18:30)。
Middleware Support for Data Mining and Knowledge Discovery in Large-scale Distributed Information Systems
This work has been partially supported by NSF (grant CCR9308344). 1 In the remainder of this paper, we will use the term \Internet" to mean either the Internet or the intranet of a particular enterprise
Azer Bestavros
Abstract
The explosive growth in availability and use of the Internet demands that we raise the level of common services and introduce new types of higher-level services. These common services would lie between the transport and application levels, hence the term \middleware" pc95], and would provide means for extending commonly available metacomputing services on the Internet1 to support the demands of High Performance Computing (HPC) applications, including those requiring data mining and knowledge discovery services. There are opportunities to create many such middleware components, including caching and replication
2 Middleware Resource Management
performance. While useful for a variety of applications, best e ort Metacomputing is not su cient for applications that are subject to timing and reliability constraints. Examples of such applications include: interactive applications (e.g., battle eld group simulations), time-constrained database queries for real-time applications (e.g. image searห้องสมุดไป่ตู้h for tactical image analysis), and data-mining and knowledge discovery applications that involve temporal data (e.g. stock market modeling and weather prediction). The real-time and reliability constraints imposed by these (and other) applications require responsive rather than merely best-e ort metacomputing. We call such an environment a Responsive Web Computer (RWC). The best-e ort philosophy of current metacomputing platforms is due to the unpredictability of the underlying computing infrastructure, which is due to the inability of applications to control or negotiate the resources they need. Therefore, in order to achieve responsive metacomputing, new middleware services need to be developed to reduce this unpredictabilty and to allow a certain level of commitment when resources are contributed to a metacomputing platform. The Resource Management Interface (RMI) is a middleware service (abstraction) that allows the computational requirements of processes to be matched with the resources available at the disposal of the system through a schedule that satis es the timing and fault-tolerance requirements of these processes. The overall structure of middleware RMI is shown in Figure 1. There are three main services to be supported. The Task Registration Service allows the computational resources needed by, and the performance constraints imposed on a task to be speci ed. The Resource Registration Service allows the computational resources contributed (or leased) to the system to be speci ed. The Resource Management Service provides the admission control and scheduling protocols for managing the registerd resources in accordance with the performance constraints of the registered tasks. To motivate the issues involved in the speci cation of a task to the resource manager, consider a simple situation where a real-time application is to be executed. Furthermore, assume that the real-time application consists of a large set of periodic processes, where each process requires various resources (e.g. CPU cycles, network bandwidth, etc.) As a concrete example, consider a Web agent, which is responsible for monitoring the contents of a particular object (e.g. areal radar map, wheather map, number of objects in a given database, stock quote). Assume that the performance of that agent is constrained so as to report back the results of its operation periodically (say every minute) to another agent (i.e. another RWC task). Furthermore,
1 Introduction
services, brokerage and resource management services, indexing services, remote scripting environments, data typing and structuring primitives, and higher level communication abstractions such as multicast and causal broadcast. Many of these middleware components are crucial for the design of scalable data mining and knowledge discovery systems. In this paper we focus on three such candidate components, namely resource management and brokerage services for responsiveness, information dissemination, and speculative service. We argue that these middleware components have the potential to alleviate the latency and bandwidth requirements of data mining and knowledge discovery systems. In our work, we use the WWW as the underlying distributed computing resource to be managed by these middleware components. First, the WWW o ers an unmatched opportunity to inspect a wide range of distributed object types, structures, and sizes. Second, the WWW is fully deployed in thousands of institutions worldwide, which gives us an unparalleled opportunity to apply our ndings to an already-existing real-world application. Our research in middleware services is aimed at: 1) Improving the predictability of network services through e cent resource management protocols; and 2) Improving the scalability of information retrieval for data mining and knowledge discovery applications through proper partitioning and distribution of data. In the remainder of this paper we overview three speci c middleware components that aim at addressing these issues. Our rst middelware component adds responsiveness (timeliness + fault-tolerance) to the services available in Internet metacomputing environments. FAFNER Col] is an example of such an environment. FAFNER brings resources and expertise from many sites world-wide to solve the problem of factoring RSA-130. The approach that FAFNER uses to harness the Internet resources available at its disposal can be best described as a beste ort approach, which does not o er any guaranteed