Entropies of complex networks with hierarchically constrained topologies
吴恩达提示词系列解读

吴恩达提示词系列解读摘要:1.吴恩达简介2.提示词系列的背景和意义3.深度学习提示词解读4.强化学习提示词解读5.计算机视觉提示词解读6.自然语言处理提示词解读7.总结正文:吴恩达,全球知名的AI专家,拥有丰富的学术和产业经验,他的一系列提示词为广大AI学习者提供了宝贵的指导。
本文将针对吴恩达提示词系列进行解读,以期帮助大家更好地理解和学习AI技术。
1.吴恩达简介吴恩达,Andrew Ng,曾是斯坦福大学的人工智能教授,后来创立了Google Brain项目,并成为了百度首席科学家。
他一直致力于推动AI技术的发展和应用,尤其是在深度学习和强化学习领域。
2.提示词系列的背景和意义吴恩达提示词系列是他对AI领域的重要观点和思考的总结,涵盖了深度学习、强化学习、计算机视觉、自然语言处理等多个领域。
这些提示词对于AI学习者来说,具有很高的参考价值,可以帮助我们更好地理解AI技术的发展趋势和应用方向。
3.深度学习提示词解读吴恩达的深度学习提示词主要包括“神经网络”、“反向传播”、“卷积神经网络”、“循环神经网络”等。
这些提示词概括了深度学习的核心概念和技术,对于理解深度学习的基本原理和应用至关重要。
4.强化学习提示词解读吴恩达的强化学习提示词主要包括“智能体”、“环境”、“状态”、“动作”、“奖励”等。
这些提示词揭示了强化学习的本质,即智能体如何在环境中通过选择动作来获得奖励,从而实现学习。
5.计算机视觉提示词解读吴恩达的计算机视觉提示词主要包括“图像分类”、“目标检测”、“语义分割”等。
这些提示词代表了计算机视觉的主要任务,对于我们理解和应用计算机视觉技术具有重要意义。
6.自然语言处理提示词解读吴恩达的自然语言处理提示词主要包括“词向量”、“序列到序列模型”、“注意力机制”等。
这些提示词概括了自然语言处理的核心技术,对于我们理解和应用自然语言处理技术具有重要价值。
术语对应的学科名词解释

术语对应的学科名词解释在我们的日常生活和学习中,我们经常会遇到各种各样的术语。
这些术语代表着特定学科中的概念或理论,是学术交流和专业研究的重要工具。
本文将通过一些常见的术语,为读者解释这些术语所对应的学科名词。
1. 熵(Entropy)熵是热力学中的一个重要概念,它代表了一个系统的无序程度。
在物理学中,熵被用来描述能量分布的混乱程度。
而在信息论中,熵则表示信息的不确定性或信息的平均信息量。
因此,熵这个术语既可在物理学中使用,也可在信息科学中使用。
2. 主观性(Subjectivity)主观性是哲学和心理学中常见的概念。
它指的是个体的主观经验和观点,与客观事实相对立。
主观性认为,每个人的认知和感受都是独特而个体化的,因此无法被客观的标准或规则所完全捕捉和测量。
主观性在哲学和心理学领域内有广泛的应用和研究。
3. 多样性(Diversity)多样性是生物学和社会科学领域中的一个重要概念。
在生物学中,多样性描述了一个生态系统或物种群体中的种类和数量多样性。
而在社会科学中,多样性则指代不同文化、民族、性别、背景等方面的差异和多元化。
多样性这个术语在不同学科中的具体定义和应用有所不同,但都强调了不同元素的多样性和丰富性。
4. 可持续发展(Sustainable Development)可持续发展是环境学和经济学中的一个重要概念。
它强调了人类社会的发展需求与生态环境的保护之间的平衡。
可持续发展要求我们在满足当前需求的同时,不损害未来世代的需求和资源。
该概念涉及到经济、社会和环境等多个领域,因此它的学科背景非常广泛。
5. 数据挖掘(Data Mining)数据挖掘是计算机科学和统计学中的一个术语。
它指的是通过从大量数据中提取模式、关联和知识等信息来发现隐藏在数据中的有价值的信息。
数据挖掘可以用于商业、科学和决策等领域,帮助我们了解数据背后的规律和趋势,从而做出更准确的预测和决策。
6. 经典条件作用(Classical Conditioning)经典条件作用是心理学中的一个重要概念,也被称为条件反射。
andrew ng的讲义中文
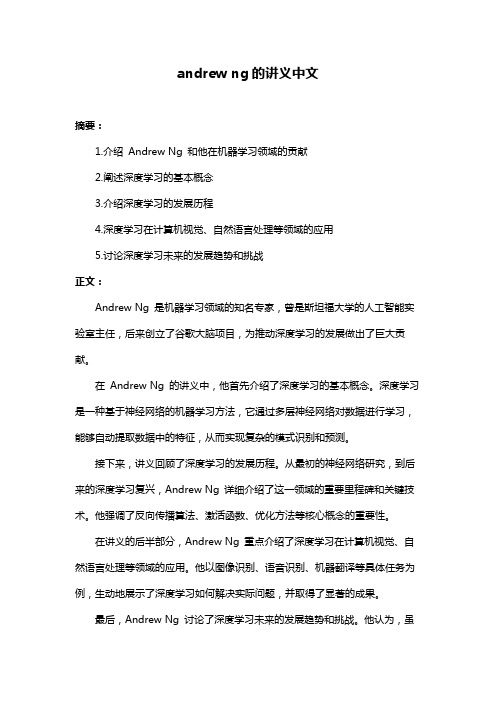
andrew ng的讲义中文
摘要:
1.介绍Andrew Ng 和他在机器学习领域的贡献
2.阐述深度学习的基本概念
3.介绍深度学习的发展历程
4.深度学习在计算机视觉、自然语言处理等领域的应用
5.讨论深度学习未来的发展趋势和挑战
正文:
Andrew Ng 是机器学习领域的知名专家,曾是斯坦福大学的人工智能实验室主任,后来创立了谷歌大脑项目,为推动深度学习的发展做出了巨大贡献。
在Andrew Ng 的讲义中,他首先介绍了深度学习的基本概念。
深度学习是一种基于神经网络的机器学习方法,它通过多层神经网络对数据进行学习,能够自动提取数据中的特征,从而实现复杂的模式识别和预测。
接下来,讲义回顾了深度学习的发展历程。
从最初的神经网络研究,到后来的深度学习复兴,Andrew Ng 详细介绍了这一领域的重要里程碑和关键技术。
他强调了反向传播算法、激活函数、优化方法等核心概念的重要性。
在讲义的后半部分,Andrew Ng 重点介绍了深度学习在计算机视觉、自然语言处理等领域的应用。
他以图像识别、语音识别、机器翻译等具体任务为例,生动地展示了深度学习如何解决实际问题,并取得了显著的成果。
最后,Andrew Ng 讨论了深度学习未来的发展趋势和挑战。
他认为,虽
然深度学习在许多领域取得了成功,但仍然存在一些问题,如模型的可解释性、数据依赖性等。
他呼吁研究人员继续探索新的方法,以解决这些问题,进一步推动深度学习的发展。
人工智能与深度学习技术考试 选择题 60题
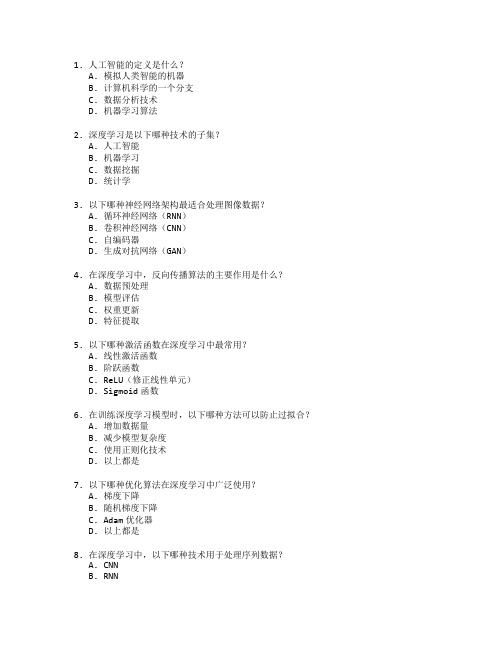
1. 人工智能的定义是什么?A. 模拟人类智能的机器B. 计算机科学的一个分支C. 数据分析技术D. 机器学习算法2. 深度学习是以下哪种技术的子集?A. 人工智能B. 机器学习C. 数据挖掘D. 统计学3. 以下哪种神经网络架构最适合处理图像数据?A. 循环神经网络(RNN)B. 卷积神经网络(CNN)C. 自编码器D. 生成对抗网络(GAN)4. 在深度学习中,反向传播算法的主要作用是什么?A. 数据预处理B. 模型评估C. 权重更新D. 特征提取5. 以下哪种激活函数在深度学习中最常用?A. 线性激活函数B. 阶跃函数C. ReLU(修正线性单元)D. Sigmoid函数6. 在训练深度学习模型时,以下哪种方法可以防止过拟合?A. 增加数据量B. 减少模型复杂度C. 使用正则化技术D. 以上都是7. 以下哪种优化算法在深度学习中广泛使用?A. 梯度下降B. 随机梯度下降C. Adam优化器D. 以上都是8. 在深度学习中,以下哪种技术用于处理序列数据?A. CNNB. RNNC. GAND. 自编码器9. 以下哪种技术用于生成新的数据样本?A. GANB. CNNC. RNND. 自编码器10. 在深度学习中,以下哪种技术用于无监督学习?A. 强化学习B. 自编码器C. CNND. RNN11. 以下哪种技术用于提高模型的泛化能力?A. 数据增强B. 模型集成C. 迁移学习D. 以上都是12. 在深度学习中,以下哪种技术用于处理半监督学习问题?A. 自编码器B. GANC. 迁移学习D. 强化学习13. 以下哪种技术用于处理强化学习问题?A. CNNB. RNNC. GAND. 强化学习算法14. 在深度学习中,以下哪种技术用于处理多任务学习问题?A. 迁移学习B. 模型集成C. 多任务学习D. 强化学习15. 以下哪种技术用于处理小样本学习问题?A. 迁移学习B. 元学习C. 强化学习D. 自编码器16. 在深度学习中,以下哪种技术用于处理对抗性攻击问题?A. 对抗训练B. 模型集成C. 数据增强D. 迁移学习17. 以下哪种技术用于处理模型解释性问题?A. 对抗训练B. 模型集成C. 解释性技术D. 数据增强18. 在深度学习中,以下哪种技术用于处理模型压缩问题?A. 模型剪枝B. 量化C. 知识蒸馏D. 以上都是19. 以下哪种技术用于处理模型部署问题?A. 模型剪枝B. 量化C. 知识蒸馏D. 模型部署技术20. 在深度学习中,以下哪种技术用于处理模型监控问题?A. 模型剪枝B. 量化C. 知识蒸馏D. 模型监控技术21. 以下哪种技术用于处理模型更新问题?A. 模型剪枝B. 量化C. 知识蒸馏D. 模型更新技术22. 在深度学习中,以下哪种技术用于处理模型维护问题?A. 模型剪枝B. 量化C. 知识蒸馏D. 模型维护技术23. 以下哪种技术用于处理模型优化问题?A. 模型剪枝B. 量化C. 知识蒸馏D. 模型优化技术24. 在深度学习中,以下哪种技术用于处理模型调试问题?A. 模型剪枝B. 量化C. 知识蒸馏D. 模型调试技术25. 以下哪种技术用于处理模型测试问题?A. 模型剪枝B. 量化C. 知识蒸馏D. 模型测试技术26. 在深度学习中,以下哪种技术用于处理模型验证问题?A. 模型剪枝B. 量化C. 知识蒸馏D. 模型验证技术27. 以下哪种技术用于处理模型评估问题?A. 模型剪枝B. 量化C. 知识蒸馏D. 模型评估技术28. 在深度学习中,以下哪种技术用于处理模型选择问题?A. 模型剪枝B. 量化C. 知识蒸馏D. 模型选择技术29. 以下哪种技术用于处理模型构建问题?A. 模型剪枝B. 量化C. 知识蒸馏D. 模型构建技术30. 在深度学习中,以下哪种技术用于处理模型设计问题?A. 模型剪枝B. 量化C. 知识蒸馏D. 模型设计技术31. 以下哪种技术用于处理模型训练问题?A. 模型剪枝B. 量化C. 知识蒸馏D. 模型训练技术32. 在深度学习中,以下哪种技术用于处理模型调优问题?A. 模型剪枝B. 量化C. 知识蒸馏D. 模型调优技术33. 以下哪种技术用于处理模型改进问题?A. 模型剪枝B. 量化C. 知识蒸馏D. 模型改进技术34. 在深度学习中,以下哪种技术用于处理模型升级问题?A. 模型剪枝B. 量化C. 知识蒸馏D. 模型升级技术35. 以下哪种技术用于处理模型扩展问题?A. 模型剪枝B. 量化C. 知识蒸馏D. 模型扩展技术36. 在深度学习中,以下哪种技术用于处理模型集成问题?A. 模型剪枝B. 量化C. 知识蒸馏D. 模型集成技术37. 以下哪种技术用于处理模型融合问题?A. 模型剪枝B. 量化C. 知识蒸馏D. 模型融合技术38. 在深度学习中,以下哪种技术用于处理模型组合问题?A. 模型剪枝B. 量化C. 知识蒸馏D. 模型组合技术39. 以下哪种技术用于处理模型协同问题?A. 模型剪枝B. 量化C. 知识蒸馏D. 模型协同技术40. 在深度学习中,以下哪种技术用于处理模型协作问题?A. 模型剪枝B. 量化C. 知识蒸馏D. 模型协作技术41. 以下哪种技术用于处理模型共享问题?A. 模型剪枝B. 量化C. 知识蒸馏D. 模型共享技术42. 在深度学习中,以下哪种技术用于处理模型分发问题?A. 模型剪枝B. 量化C. 知识蒸馏D. 模型分发技术43. 以下哪种技术用于处理模型部署问题?A. 模型剪枝B. 量化C. 知识蒸馏D. 模型部署技术44. 在深度学习中,以下哪种技术用于处理模型运行问题?A. 模型剪枝B. 量化C. 知识蒸馏D. 模型运行技术45. 以下哪种技术用于处理模型执行问题?A. 模型剪枝B. 量化C. 知识蒸馏D. 模型执行技术46. 在深度学习中,以下哪种技术用于处理模型应用问题?A. 模型剪枝B. 量化C. 知识蒸馏D. 模型应用技术47. 以下哪种技术用于处理模型实施问题?A. 模型剪枝B. 量化C. 知识蒸馏D. 模型实施技术48. 在深度学习中,以下哪种技术用于处理模型实现问题?A. 模型剪枝B. 量化C. 知识蒸馏D. 模型实现技术49. 以下哪种技术用于处理模型开发问题?A. 模型剪枝B. 量化C. 知识蒸馏D. 模型开发技术50. 在深度学习中,以下哪种技术用于处理模型设计问题?A. 模型剪枝B. 量化C. 知识蒸馏D. 模型设计技术51. 以下哪种技术用于处理模型构建问题?A. 模型剪枝B. 量化C. 知识蒸馏D. 模型构建技术52. 在深度学习中,以下哪种技术用于处理模型训练问题?A. 模型剪枝B. 量化C. 知识蒸馏D. 模型训练技术53. 以下哪种技术用于处理模型调优问题?A. 模型剪枝B. 量化C. 知识蒸馏D. 模型调优技术54. 在深度学习中,以下哪种技术用于处理模型改进问题?A. 模型剪枝B. 量化C. 知识蒸馏D. 模型改进技术55. 以下哪种技术用于处理模型升级问题?A. 模型剪枝B. 量化C. 知识蒸馏D. 模型升级技术56. 在深度学习中,以下哪种技术用于处理模型扩展问题?A. 模型剪枝B. 量化C. 知识蒸馏D. 模型扩展技术57. 以下哪种技术用于处理模型集成问题?A. 模型剪枝B. 量化C. 知识蒸馏D. 模型集成技术58. 在深度学习中,以下哪种技术用于处理模型融合问题?A. 模型剪枝B. 量化C. 知识蒸馏D. 模型融合技术59. 以下哪种技术用于处理模型组合问题?A. 模型剪枝B. 量化C. 知识蒸馏D. 模型组合技术60. 在深度学习中,以下哪种技术用于处理模型协同问题?A. 模型剪枝B. 量化C. 知识蒸馏D. 模型协同技术答案2. B3. B4. C5. C6. D7. D8. B9. A10. B11. D12. C13. D14. C15. B16. A17. C18. D19. D20. D21. D22. D23. D24. D25. D26. D27. D28. D29. D30. D31. D32. D33. D34. D35. D36. D37. D38. D39. D40. D41. D42. D43. D44. D45. D46. D47. D48. D49. D50. D52. D53. D54. D55. D56. D57. D58. D59. D60. D。
人工智能导论课后习题答案
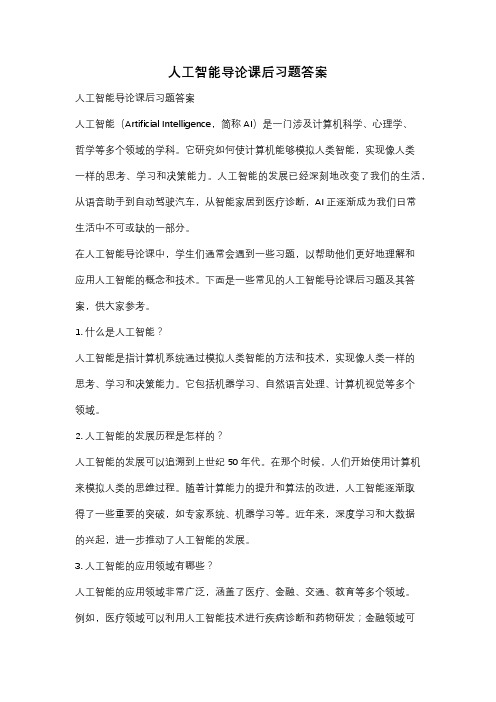
人工智能导论课后习题答案人工智能导论课后习题答案人工智能(Artificial Intelligence,简称AI)是一门涉及计算机科学、心理学、哲学等多个领域的学科。
它研究如何使计算机能够模拟人类智能,实现像人类一样的思考、学习和决策能力。
人工智能的发展已经深刻地改变了我们的生活,从语音助手到自动驾驶汽车,从智能家居到医疗诊断,AI正逐渐成为我们日常生活中不可或缺的一部分。
在人工智能导论课中,学生们通常会遇到一些习题,以帮助他们更好地理解和应用人工智能的概念和技术。
下面是一些常见的人工智能导论课后习题及其答案,供大家参考。
1. 什么是人工智能?人工智能是指计算机系统通过模拟人类智能的方法和技术,实现像人类一样的思考、学习和决策能力。
它包括机器学习、自然语言处理、计算机视觉等多个领域。
2. 人工智能的发展历程是怎样的?人工智能的发展可以追溯到上世纪50年代。
在那个时候,人们开始使用计算机来模拟人类的思维过程。
随着计算能力的提升和算法的改进,人工智能逐渐取得了一些重要的突破,如专家系统、机器学习等。
近年来,深度学习和大数据的兴起,进一步推动了人工智能的发展。
3. 人工智能的应用领域有哪些?人工智能的应用领域非常广泛,涵盖了医疗、金融、交通、教育等多个领域。
例如,医疗领域可以利用人工智能技术进行疾病诊断和药物研发;金融领域可以利用人工智能技术进行风险评估和投资决策;交通领域可以利用人工智能技术实现自动驾驶等。
4. 机器学习是什么?机器学习是一种人工智能的分支,它研究如何使计算机能够从数据中学习,并根据学习到的知识进行决策和预测。
机器学习可以分为监督学习、无监督学习和强化学习等不同类型。
5. 什么是深度学习?深度学习是机器学习的一种方法,它模拟人脑神经网络的结构和功能,通过多层神经网络进行学习和决策。
深度学习在计算机视觉、自然语言处理等领域取得了很多重要的突破,如图像识别、语音识别等。
6. 人工智能是否会取代人类工作?人工智能在某些领域已经取得了很大的进展,但目前还不具备完全取代人类工作的能力。
概括convnext
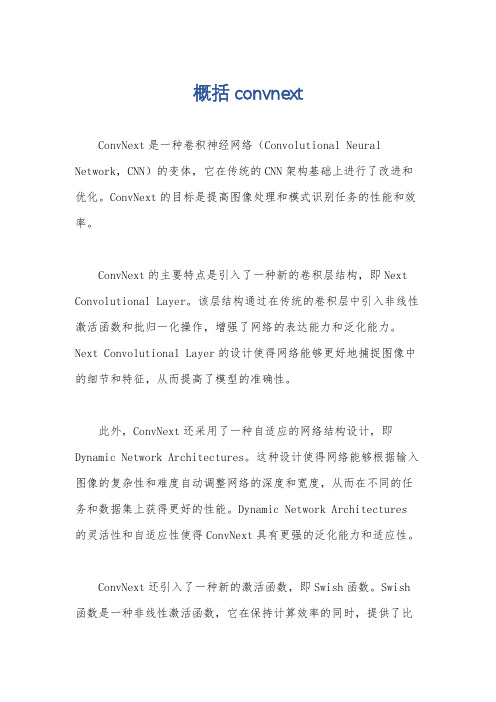
概括convnextConvNext是一种卷积神经网络(Convolutional Neural Network,CNN)的变体,它在传统的CNN架构基础上进行了改进和优化。
ConvNext的目标是提高图像处理和模式识别任务的性能和效率。
ConvNext的主要特点是引入了一种新的卷积层结构,即Next Convolutional Layer。
该层结构通过在传统的卷积层中引入非线性激活函数和批归一化操作,增强了网络的表达能力和泛化能力。
Next Convolutional Layer的设计使得网络能够更好地捕捉图像中的细节和特征,从而提高了模型的准确性。
此外,ConvNext还采用了一种自适应的网络结构设计,即Dynamic Network Architectures。
这种设计使得网络能够根据输入图像的复杂性和难度自动调整网络的深度和宽度,从而在不同的任务和数据集上获得更好的性能。
Dynamic Network Architectures 的灵活性和自适应性使得ConvNext具有更强的泛化能力和适应性。
ConvNext还引入了一种新的激活函数,即Swish函数。
Swish 函数是一种非线性激活函数,它在保持计算效率的同时,提供了比传统的激活函数(如ReLU)更好的激活特性,从而进一步提高了网络的性能。
总结起来,ConvNext是一种基于卷积神经网络的改进型架构,通过引入Next Convolutional Layer、Dynamic Network Architectures和Swish函数等新的设计和技术,提高了网络的表达能力、泛化能力和性能。
这些改进使得ConvNext在图像处理和模式识别任务中具有更好的效果和应用潜力。
人工智能导论_哈尔滨工程大学中国大学mooc课后章节答案期末考试题库2023年

人工智能导论_哈尔滨工程大学中国大学mooc课后章节答案期末考试题库2023年1.下列不属于two-stage检测算法的是:()参考答案:YOLO2.下列对强化学习特点说法错误的是:()参考答案:反馈及时迅速3.下列哪个选项不属于进行迁移学习的原因:()参考答案:大数据与强计算之间的矛盾4.人工智能技术解决了传统机器博弈理论的困难?()参考答案:围棋具有巨大的搜索空间_盘面评估与博弈树搜索紧密相关_高层次的围棋知识也很难归纳5.对于深度学习说法正确的是:()参考答案:通过构建多隐层的模型和海量训练数据,来学习更有用的特征,从而最终提升分类或预测的准确性_“深度模型”是手段,“特征学习”是目的_强调了模型结构的深度,通常有5-10多层的隐层节点_与人工规则构造特征的方法相比,利用大数据来学习特征,更能够刻画数据的丰富内在信息6.支持向量机是最受欢迎、讨论最为广泛的机器学习分类方法之一。
这种方法适用于高维空间(特征向量中有许多特征),并且可以有效地用于小型数据集。
参考答案:正确7.朴素贝叶斯的一个有趣的特征是,它适用于非常大的数据集。
参考答案:错误8.直接策略的RL直接优化目标函数,对策略进行参数化表示,与值函数相比,策略化参数的方法更简单,更容易收敛。
参考答案:正确9.随着人工智能的技术不断地发展,现如今机器也可以创造出令人惊叹的艺术画作,但暂时没能写出完整的小说。
参考答案:错误10.用人工智能技术学习的服装设计风格,建立一套自动给服装线稿添加配色、材质纹理的算法,可以在几秒之内生成任意数量的颜色材质搭配方案,帮助服装设计师更好更快的抓住潮流趋势。
参考答案:正确11.以下关于感知机模型说法错误的是()参考答案:感知机能够求解异或问题12.以下有关BP网络说法错误的是()参考答案:BP网络是一种前馈网络,其隐单元必须分层,又称为多层前馈网络13.思维的本质是人脑有意识的对客体的反映,这个过程构成了人类认识的高级阶段。
基于改进随机游走的复杂网络节点重要性评估
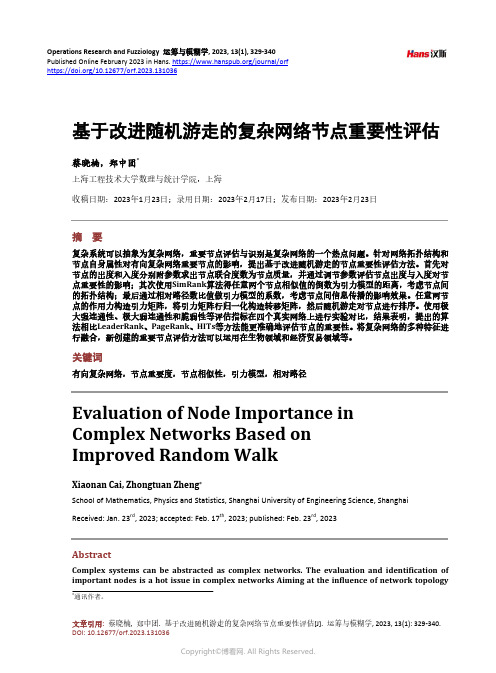
Operations Research and Fuzziology 运筹与模糊学, 2023, 13(1), 329-340 Published Online February 2023 in Hans. https:///journal/orf https:///10.12677/orf.2023.131036基于改进随机游走的复杂网络节点重要性评估蔡晓楠,郑中团*上海工程技术大学数理与统计学院,上海收稿日期:2023年1月23日;录用日期:2023年2月17日;发布日期:2023年2月23日摘要复杂系统可以抽象为复杂网络,重要节点评估与识别是复杂网络的一个热点问题。
针对网络拓扑结构和节点自身属性对有向复杂网络重要节点的影响,提出基于改进随机游走的节点重要性评估方法。
首先对节点的出度和入度分别附参数求出节点联合度数为节点质量,并通过调节参数评估节点出度与入度对节点重要性的影响;其次使用SimRank 算法得任意两个节点相似值的倒数为引力模型的距离,考虑节点间的拓扑结构;最后通过相对路径数比值做引力模型的系数,考虑节点间信息传播的影响效果。
任意两节点的作用力构造引力矩阵,将引力矩阵行归一化构造转移矩阵,然后随机游走对节点进行排序。
使用极大强连通性、极大弱连通性和脆弱性等评估指标在四个真实网络上进行实验对比,结果表明,提出的算法相比LeaderRank 、PageRank 、HITs 等方法能更准确地评估节点的重要性。
将复杂网络的多种特征进行融合,新创建的重要节点评估方法可以运用在生物领域和经济贸易领域等。
关键词有向复杂网络,节点重要度,节点相似性,引力模型,相对路径Evaluation of Node Importance in Complex Networks Based on Improved Random WalkXiaonan Cai, Zhongtuan Zheng *School of Mathematics, Physics and Statistics, Shanghai University of Engineering Science, Shanghai Received: Jan. 23rd, 2023; accepted: Feb. 17th, 2023; published: Feb. 23rd, 2023AbstractComplex systems can be abstracted as complex networks. The evaluation and identification of important nodes is a hot issue in complex networks Aiming at the influence of network topology*通讯作者。
- 1、下载文档前请自行甄别文档内容的完整性,平台不提供额外的编辑、内容补充、找答案等附加服务。
- 2、"仅部分预览"的文档,不可在线预览部分如存在完整性等问题,可反馈申请退款(可完整预览的文档不适用该条件!)。
- 3、如文档侵犯您的权益,请联系客服反馈,我们会尽快为您处理(人工客服工作时间:9:00-18:30)。
a r X i v :0803.1247v 1 [c o n d -m a t .d i s -n n ] 8 M a r 2008Entropies of complex networks with hierarchically constrainedtopologiesGinestra Bianconi 1,Anthony C.C.Coolen 2,3,Conrad J.Perez Vicente 41Abdus Salam International Center for Theoretical Physics,Strada Costiera 11,34014Trieste,Italy2Department of Mathematics,King’s College London,The Strand,London WC2R 2LS,United Kingdom3Randall Division of Cell and Molecular Biophysics,Kings College London,New Hunts House,London SE11UL,United Kingdom4Departament de F´ısica Fonamental,Facultat de F´ısica,Universitat de Barcelona,08028Barcelona,SpainAbstractThe entropy of a hierarchical network topology in an ensemble of sparse random networks,with ”hidden variables”associated to its nodes,is the log-likelihood that a given network topology is present in the chosen ensemble.We obtain a general formula for this entropy,which has a clear interpretation in some simple limiting cases.The results provide new keys with which to solve the general problem of “fitting”a given network with an appropriate ensemble of random networks.PACS numbers:89.75.Hc,89.75.Fb,75.10.NrI.INTRODUCTIONThe entropy is a key concept in information theory[1]and in the theory of dynamical systems[2].In information theory,the problem of inference of a probability distribution on the basis offinite number of independent observations is usually addressed using the maximum likelihood principle or via the minimization of the Kullback-Leibler distance be-tween the given(empirical)distribution and the inferred one.Recently,several studies have extended the tools of information theory along these lines in order to measure the perfor-mance offiltering procedures of correlation matrices in the case of multivariate data[3,4]. In the framework of graph theory the large deviations of the ensemble of random Erd¨o s and Renyi graphs where derived by studying the free energies of statistical mechanics models defined on them[5,6].There is now increased interest,in the community of complex net-works[7,8,9],in the definition of entropy measures that are related with the networks’topological structure[10]or with diffusion processes defined on them[11].The inference problem applied to complex networks can be formulated as the identification of the ensemble of networks which retains the essential structural characteristics and complexity of a given real network realization.The identification of this ensemble,is an activefield of research. One aims tofit a given specific network with a suitable network ensemble that retains some information on its structure.Newman has proposed this approach tofind the community structure in a given network[12].Later,this method has been extended to define ensembles of networks that have other topological characteristics in common with the real network, such as the degree sequence and/or the degree correlations.As we add further features that a desired ensemble is to have in common with a given real network,we effectively consider ensembles with decreasing cardinality.The cardinality of an ensemble of networks with a given topology has attracted the attention of the graph theory community[13,14,15],and more recently also of the statistical mechanics community[10].In this paper we evaluate the entropy of a given hierarchical topology in a”canonical”or”hidden variable”ensemble,i.e.we calculate the normalized logarithm of the probability that a given topology appears in this ensemble.By hierarchical topology we will mean the set of the generalized degrees of the nodes,defined as the sequence k i=(k1i,k2i,...,k L i)of the number of nodes at distance1,2,...,L from the node i.The”canonical”or“hidden variable”[16,17,18,19,20]ensembles are generalization of the G(N,p)ensemble for het-erogeneous nodes.The hetereogeneity of the nodes is described in terms of some”hidden variables”x i,defined on each node i of the network,and the probability p ij of a link between a node i and a node j is not p as in G(N,p)but it is a general function Q(x i,x j)of the hidden variables at i and j nodes.These ensembles correspond to networks which satisfy soft constraints,for example the degree of a node is notfixed,but only the average degree of each node isfixed,allowing for Poissonianfluctuations.We derive a general formula for the entropy of a given topology in a”canonical”ensemble using ideas and methods from the study of diluted combinatorial optimization problems and statistical mechanical systems on sparse networks[21,22,23,24,25,26,27,28,29,30,31, 32,33,34,35,36,37,38,39,40,41].In the simple case where we study the likelihood of a degree distribution of a network belonging to the chosen ensemble the entropy is found to be the Kullback-Leibler distance between the probability distribution of the degrees and the expected probability of the typical topology of the network.The paper is structured as follows:in section II we introduce the definition of the problem, in section III we provide the asymptotic entropy expression of the network topology in a given ensemble,in section IV we study the form that the entropy takes in special and relevant cases,and the conclusions are presented in section V.II.FORMULATION OF THE PROBLEM AND DEFINITIONSTo model the essential properties of a real network it is useful to think of it as an instance of an ensemble of networks.The ensemble can be either”microcanonical”or”canonical”depending on whether the networks in the ensemble are subject to hard or soft constraints. The main example of what we call a”microcanonical”ensemble is G(N,M)where the number of links isfixed to be exactly M,and the main example of”canonical”ensemble is G(N,p)in which only the average number of links M =pN(N−1)/2isfixed.These ensembles can be generalized to ensembles of random graphs with a given degree sequence and with a given hidden variable distribution.In this paper we will calculate the entropy of a given network topology(defined in terms of its hierarchical structure)in a general ”canonical”ensemble.This entropy is defined as the probability that the given network topology is found in the”canonical”network ensemble under consideration.A.“Canonical”ensemblesWe consider networks characterized by N nodes(or‘sites’)labeled i=1,...,N,and a symmetric matrix c with entries c ij∈{0,1}that specify whether(c ij=1)or not(c ij=0)nodes i and j are connected.We choose c ii=0for all i.We write the set of all such undirected networks as G={0,1}1N Q(x i,x j)δcij,1+(1−cthemselves,or by giving the collective generalized degree statistics,conditioned on the values of the hidden variables,i.e.P(k|x,c)=P(k1,...,k L|c)=1log c∈G W(c) iδk i,k i(c)(5)N1constrain statistics:ΩL[P]=log k1...k N k,xδ P(k|x)− iδk,k iδ[x−x i]N(6)but absent from (5).We evaluate (5,6)by writing each Kronecker δand each δ-functionin integral form.Upon defining the short-hands k 0i =1for all i ,and ωi ·k i = L ℓ=1ωℓi k ℓi ,expression (3)allows us to simplify the term δk ,k i (c )toδk i ,k i (c )=π−πd ωie i ωi ·k iN logπ−π id ωie i ωi ·k iNlogk ,xd ˆP (k |x )e iN ˆP (k |x )P (k |x )N lim ∆→0log k ,xd ˆP (k |x )e iN ∆ˆP (k |x )P (k |x )(2π)LD [{ω,k }](10)The core of the problem is apparently to calculate the function D [{ω,k }]in (8),whichinvolves the introduction of a measure W (ω,k ,x |{ω,k })=N −1i δk ,k i δ[x −x i ]δ[ω−ωi ]:D [{ω,k }]= i<j1+c2cNdxdx ′Q (x,x ′)π−πd ωd ω′kk ′W (ω,k ,x |...)×W (ω′,k ′,x ′|...)e−i P L ℓ=1[ωℓk ′ℓ−1+ω′ℓk ℓ−1]−1N/2π],resulting inD [{ω,k }]={dW d ˆW}e iNR π−πd ωdxPk ˆW (ω,k ,x )W (ω,k ,x )+O (N0)×e12cN −iP i ˆW (ωi ,k i ,x i )(12)Now only the last line contains microscopic variables,and it factorizes fully over the nodes of the network.Upon inserting(12)into(9)and(10)this allows us to evaluate both expressions for N→∞via steepest descent integration over the distributions W(ω,k,x),leading toΩL[{k}]=extr{W,ˆW}Ψ1[{W,ˆW}](13)ΩL[P]=extr{W,ˆW,ˆP}Ψ2[{W,ˆW,ˆP}](14)with the functionsΨ1[{W,ˆW}]=i π−πdωdx kˆW(ω,k,x)W(ω,k,x)+Φ[{W}]+ dx p(x) k P(k|x)log π−πdω(2π)L k e i[ω·k−ˆP(k|x)/p(x)−ˆW(ω,k,x)](16) whereΦ[{W}]=−12c dxdx′Q(x,x′) π−πdωdω′ kk′W(ω,k,x)W(ω′,k′,x′)×e−i P Lℓ=1[ωℓk′ℓ−1+ω′ℓkℓ−1](17)It will be convenient to introduce new functions Q(k|x)=exp[−iˆP(k|x)/p(x)]and V(ω,k,x)=exp[−iˆW(ω,k,x)]so that our saddle-point equations simplify toΩL[{k}]=extr{V,W}˜Ψ1[{V,W}](18)ΩL[P]=extr{Q,V,W}˜Ψ2[{Q,V,W}](19)with the functions˜Ψ1[{V,W}]=Φ[{W}]− π−πdωdx k W(ω,k,x)log V(ω,k,x)+ dx p(x) k P(k|x)log π−πdω˜Ψ[{Q,V,W}]=Φ[{W}]− π−πdωdx k W(ω,k,x)log V(ω,k,x)2− dx p(x) k P(k|x)log Q(k|x)+ dx p(x)log k Q(k|x) π−πdωπ−πdω′V(ω′,k,x)e iω′·k(23) For˜Ψ2[...](referring to ensembles with constrained distributions of generalized degrees) these are found to be the following:log V(ω,k,x)=c dx′Q(x,x′) π−πdω′ k′W(ω′,k′,x′)e−i P Lℓ=1[ωℓk′ℓ−1+ω′ℓkℓ−1](24)p(x)Q(k|x)V(ω,k,x)e iω·kW(ω,k,x)=k′Q(k′|x) π−πdωV(ω,k′,x)e iω·k′(26) The last equation is easily solved,viz.P(k|x)Q(k|x)=×π−πd ω′V (ω′,k ′,x ′)e i ω′·k ′−iP Lℓ=1[ωℓk ′ℓ−1+ω′ℓk ℓ−1]π−πd ωexp i ω·k ′+c ξ′∈I N L γ(k ′,ξ′,x ′)e −i ω·ξ′ The two integrals over ωin the latter fraction can be done.Both are of the formI (k ,k ′,x )= π−πd ωexp i ω·k +c ξ′∈I N Lγ(k ′,ξ′,x ′)e −i ω·ξ′ =(2π)Lm ≥0c m m ! ξ1...ξm ∈I NL m n =1γ(k ′,ξn ,x ′) L ℓ=1δk ′ℓ,k ℓ−1+P n ≤m ξn ℓm ! ξ1...ξm ∈I N Lm n =1γ(k ′,ξn ,x ′) δk ′,P n ≤m ξn=δξ1,1 dx ′p (x ′)Q (x,x ′)k ′k ′1 ξ1...ξk ′1 k ′1n =1γ(k ′,ξn ,x ′) δk ′,Pn ≤k ′1ξn(33)where we use the conventions that [ m n =1u n ]m =0≡1,[ mn =1u n ]m =0≡0,and [ξ1...ξm u (ξ1,...,ξm )]m =0≡1.If L =1we have k =→k and ξ→1,so γ(k ,ξ,x )→γ(k,x ).This describes the situation where the degrees are not generalized,but measure as usual onlythe number of direct links per node.Here our equation forγ(...)simplifies drastically to L=1:γ(k,x)= dx′p(x′)Q(x,x′) k′k′P(k′|x′)cγ(x′) k kP(k|x′)(35) If L>1we can manipulate at most some further Kroneckerδs,and thefinal form is thereforeγ(k,ξ,x)=ξ2δξ1,1ξ1...ξξ2 ξ2n=1γ((ξ2,...,ξL,k′),ξn,x′) δk′,P n≤ξ2ξn L L−1ℓ=1δξℓ+1,P n≤ξ2ξnℓC.Simplification of the asymptotic entropy formulasAt this stage we insert our previous results for the kernels{V,W,Q}into(18,19,20,21) to arrive at more explicit expressions for the asymptotic entropies,which will only involve the functionγ(k,ξ,x)of(36).Thefirst step is to substitute expression(27)into(21).This leads,in combination with the fact that at the relevant saddle-points the kernels{V,W} obey identical equations for the two cases(constrained generalized degrees versus constrained statistics of generalized degrees),to a simple and natural relation between our two entropies:lim N→∞ΩL[P]=limN→∞ΩL[{k}]− dx p(x) k P(k|x)log P(k|x)(37)The extra freedom to construct microscopic network realizations in the case where we only constrain the generalized degree distribution,as opposed to constraining the actual values of the generalized degrees,is measured by the Shannon entropy of the imposed distribution P(k|x).So we only need to express lim N→∞ΩL[{k}]in terms of the functionγ(k,ξ,x).We first note that at the relevant saddle-point the functionΦ[{W}](17)takes the valueΦ[{W}]=−12 dxπ−πdωkW(ω,k,x)log V(ω,k,x)(38)Insertion into(20),followed by elimination of W(ω,k,x)via(23),leads us to limN→∞ΩL[{k}]= dx p(x) k P(k|x)log π−πdω2c−1π−πd ωV(ω,k ,x )e i ω·k(39)The final step is the elimination of V (ω,k ,x )via (29),followed by integration over ω,using the property that γ(k ,ξ,x )=0unless ξ1=1:π−πd ωm !ξ1...ξm n ≤mγ(k ,ξn,x ) δk ,Pn ≤mξn =δk ,0+c k 1θ[k 1−1k 1! ξ1...ξk 1 n ≤k 1 γ(k ,ξn ,x ) δk ,Pn ≤k 1ξn (40)π−πd ω(m −1)! ξ1...ξm n ≤mγ(k ,ξn ,x ) δk ,Pn ≤m ξn =c k 1θ[k 1−1(k 1−1)! ξ1...ξk 1n ≤k1γ(k ,ξn ,x ) δk ,P n ≤k 1ξn (41)So one arrives at the compact result,where we have used the fact that if k 1=0then k ℓ=0for all ℓ(which follows from the definition of the generalized degrees):lim N →∞ΩL [{k }]=k 1P (k 1)log πc (k 1)(42)+dx p (x )kP (k |x )logξ1...ξk 1n ≤k 1γ(k ,ξn,x )δk ,Pn ≤k 1ξn with the average-c Poissonian degree distribution πc (k )=c k e −c /k !.If we introduce the Fourier transforms of γ(k ,ω,x )defined asγ(k ,ω,x )=ξγ(k ,ξ,x )e i ω·ξ(43)we can write the entropy (42)equivalently aslim N →∞ΩL [{k }]= dx p (x )kP (k |x )log d ωe −i ω·ξ+cγ(k ,ω,x )πcγ(k ,ω,x )(k 1)IV.APPLICATIONS OF THE GENERAL THEORYA.Regular random graphsOurfirst application domain is that of r-regular degree distribution P(k|x)=δk,k(r), with k(r)=(r,r2,...,r L).Here one can solve(36)explicitly:γ(k,ξ,x)=ξ2δξ1,1ξ1...ξk1(x′) r n=1γ(k(r),ξn,x′) Lℓ=1δrℓ,P n≤rξnℓThe solution is seen to be of the formγ(k,ξ,x)=γ(k,x)δξ,(1,r,r2,...,r L−1),and independent of k L.Insertion of this form into the above equation then givesγ(k,x)=rγ(k(r),x′)(45)We conclude thatγ(k,ξ,x)=γ(x) L−1 ℓ=1δkℓ,rℓ L ℓ=1δξℓ,rℓ−1 (46) whereγ(x)is the solution ofγ(x)=rγ(x′)(47)For the entropies(37,42)one thenfindslim N→∞ΩL[P]=limN→∞ΩL[{k}]=logπc(r)+r dx p(x)logγ(x)(48)As expected,the two entropies are identical(since for regular graphs there is no entropy contribution from degree permutations)and independent of L(since upon specifying that the degrees are r-regular,the full distributions P(k|x)are uniquely specified for any L).In the special case Q(x,x′)=1of uncorrelated degrees the above solution simplifies further. Nowγ(x)=2r log(r/c)(49)B.The case L =1For L =1we have already simplified our formula for the function γ(k ,ξ,x )to relation (35)forasimplefunction γ(x ).We can do the same for expression (42)for the entropy,which giveslim N →∞Ω1[{k }]=kP (k )log πc (k )+dx p (x )k P (k |x )log γk (x )(50)lim N →∞Ω1[P ]=kP (k )log πc (k )−dx p (x )kP (k |x )log[P (k |x )/γk (x )](51)with πc (k )=c k e −c /k !,and where γ(x )is to be solved fromγ(x )= dx ′p (x ′)Q (x,x ′)k =kkP (k ).Expression (50)now becomes Q (x,x ′)=1:lim N →∞Ω1[{k }]=kP (k )log πc (k )+1k log(2k/c )(53)So,if one also choosesp (x )k (x ),withk (x )=kkP (k |x )thendx ′Q (x,x ′)f (x ′)=λf (x ),f (x )=λ2dx p (x )k (x )log[λp (x )k (x )/c ]= k P(k)logπc(k)+12k= dx p(x)k(x)).Let us next discuss some example kernels Q(x,x′)for which γ(x)can be solved explicitly,either directly,or via the above procedure based on using eigenfunctions of Q(.,.):•First example:Here we assume Q(x,x′)to be such that the conditional connectivities k(x)= k kP(k|x)are the typical ones for the ensemble(1),which implies thatk(x)=c dx′Q(x,x′)p(x′)(56) In this case(52)has the solutionγ(x)=k(x)/c,which leads to the following simple expression for the entropies:Ω1[{k}]= dx p(x) k P(k|x)logπk(x)(57) limN→∞limΩ1[P]=− dx p(x) k P(k|x)log[P(k|x)/πk(x)](58) N→∞This indicates that in this case the entropy lim N→∞Ω1[P]takes the form of an integral over p(x)of the Kullback-Leibler distance between the probabilities P(k|x)and the Possion distributionπk(x).We note that for the hidden variable model the typical degree distribution of the nodes with hidden variable x is indeedπk(x)[20].•Second example:1Q(x,x′)=a0+a1δ(x−x′),k(x)=1−a1 dx p2(x)+1c log 1−a1 1−1dx p2(x)+12•Third example:Q (x,x ′)=g (x )+g (x ′)g 2 0+g (x )22 1−1dx φ(x ),and with g (x )≥0for allx ∈[−1,1].Here one finds the solutionγ(x )=1cg 2 0 g 2 0+g (x ),λ= g 0+dx p (x )g (x )(63)C.The case L =2Here we have to find first the solution of (36),which now reduces to γ((k 1,k 2),(1,ξ),x )=ξξ1...ξξξn =1γ((ξ,k ′),(1,ξn ),x ′)δk ′,P n ≤ξξn(64)We observe that the right-hand side is independent of k 2,so the solution of our equation must have the form γ((k 1,k 2),(1,ξ),x )=γ(k 1,ξ,x ),whereγ(k,ξ,x )=ξξ1...ξξξn =1γ(ξ,ξn ,x ′)δk ′,P n ≤ξξn.(65)The entropy would becomelim N →∞Ω2[{k }]=k 1P (k 1)log πc (k 1)+dx p (x )k 1k 2P (k 1,k 2|x )logξ1...ξk 1n ≤k 1γ(k 1,ξn ,x )δk 2,P n ≤k1ξn(66)Let us limit ourselves to the simplest scenario where there are no degree correlations,i.e.Q (x,x ′)=1.Here we have γ(k,ξ,x )=γ(k,ξ),and we need only the generalized degreestatistics P (k 1,k 2)=dx p (x )P (k 1,k 2|x ).Our formulae thereby reduce toγ(k,ξ)=ξξ1...ξξξn =1γ(ξ,ξn )δk ′,P n ≤ξξn(67)limΩ2[{k}]= k1P(k1)logπc(k1)(68) N→∞+ k1k2P(k1,k2)log ξ1...ξk1 n≤k1γ(k1,ξn) δk2,P n≤k1ξnHere one observes the validity of the following simple relation:k2γ(k1,k2)γ(k2,k1)=k1[3]M.Tummiello,F.Lillo and R.N.Mantegna,Phys.Rev.E76,031123(2007).[4]G.Biroli,J.-P.Bouchaud and M.Potters,arXiv:0710.0802[cond-mat](2007).[5] A.Engel,R.Monasson and A.K.Hartmann,Jour.Stat.Phys.117,387(2004).[6]O.Rivoire,Jour.Stat.Phys.117,453(2004).[7]R.Albert and A.-L.Barab´a si,Rev.Mod.Phys.74,47(2002).[8]S.N.Dorogovtsev,A.Goltsev and J.F.F.Mendes,arXiv:0705.0010[cond-mat](2007).[9]S.Boccaletti,tora,Y.Moreno,M.Chavez and D.U.Hwang,Phys.Rep.424,175(2006).[10]G.Bianconi,Europhys.Lett.81,28005(2008).[11]J.Gomez-Gardenes and tora,arXiv:0712.0278[cond-mat].[12]M.E.J.Newman and E.A.Leicht,PNAS104,9564(2007).[13] E.Bender and E.Rodney Canfield,bin.Theory Ser.A24,296(1978).[14] B.D.McKay,Ars Combin.19A,15(1985).[15] B.Pittel and N.Wormald,bin.Theory Ser.A101,249(2003).[16]J.Park and M.E.J.Newman,Phys.Rev.E70,066146(2004).[17] B.Sodeberg,Phys.Rev.E66,066121(2002).[18] F.Chung and L.Lu,PNAS99,15879(2002).[19]G.Caldarelli,A.Capocci,P.De Los Rios and M.A.Mu˜n oz,Phys.Rev.Lett.89,258702(2002).[20]M.Bogu˜n´a and R.Pastor-Satorras,Phys.Rev.E68,036112(2003).[21]R.Monasson,J.Phys.A31,513(1998).[22]R.Monasson and R.Zecchina,Phys.Rev.E56,1357(1997).[23]R.Monasson and R.Zecchina,J.Phys.A:Math.Gen.31,9209(1998).[24]R.Monasson,R.Zecchina,S.Kirkpatrick,B.Selman and L.Troyansky Nature400,133(1999).[25]M.Weigt and A.K.Hartmann,Phys.Rev.Lett.84,6118(2000).[26]R.Mulet,A.Pagnani,M.Weigt and R.Zecchina,Phys.Rev.Lett.89,268701(2002).[27]M.Leone,A.V`a zquez,A.Vespignani and R.Zecchina,Eur.Phys.Jour.B28,191(2002).[28] B.Wemmenhove and A.C.C.Coolen,J.Phys.A:Math.Gen.36,9617(2003).[29]I.P´e rez-Castillo and N.S.Skantzos,J.Phys.A:Math.Gen.37,9087(2004).[30]I.P´e rez-Castillo,B.Wemmenhove,J.P.L.Hatchett,A.C.C.Coolen,N.S.Skantzos and T.Nikoletopoulos,J.Phys.A:Math.Gen.37,8789(2004).[31]T.Nikoletopoulos T,A.C.C.Coolen,I.P´e rez Castillo,N.S.Skantzos,J.P.L.Hatchett andB.Wemmenhove J.Phys.A:Math.Gen.37,6455(2004).[32]T.Nikoletopoulos and A.C.C.Coolen,J.Phys.A:Math.Gen.37,8433(2004).[33]J.P.L.Hatchett,I.P´e rez-Castillo,A.C.C.Coolen and N.S.Skantzos,Phys.Rev.Lett.95,117204(2005).[34]H.Hansen-Goos and M.Weigt,J.Stat.Mech.Theory Exp.P08001(2005).[35] A.C.C.Coolen,N.S.Skantzos,I.P´e rez Castillo,C.J.P´e rez Vicente,J.P.L.Hatchett,B.Wemmenhove and T.Nikoletopoulos,J.Phys.A:Math.Gen.38,8289(2005).[36]N.S.Skantzos,I.P´e rez Castillo and J.P.L.Hatchett,Phys.Rev.E72,066127(2005).[37]N.S.Skantzos and J.P.L.Hatchett,Physica A381,202(2007).[38] A.Mozeika and A.C.C.Coolen,preprint arXiv:0712.1139(2007).[39]L.Zdeborova and F.Krzakala,Phys.Rev.E76,031131(2007).[40]J.Raymond and D.Saad,J.Phys.A:Math.Gen.40,12315(2007).[41]R.C.Alamino and D.Saad,J.Phys.A:Math.Gen.40,12259(2007).。