1010_Analytical_data_interpretation_and_treatment_分析数据解释及处理
SPSS术语中英文对照
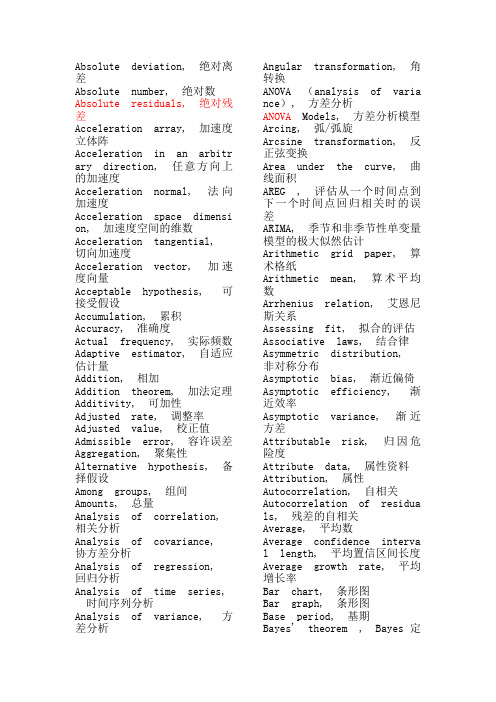
Absolute deviation, 绝对离差Absolute number, 绝对数Absolute residuals, 绝对残差Acceleration array, 加速度立体阵Acceleration in an arbitrary direction, 任意方向上的加速度Acceleration normal, 法向加速度Acceleration space dimension , 加速度空间的维数Acceleration tangential, 切向加速度Acceleration vector, 加速度向量Acceptable hypothesis, 可接受假设Accumulation, 累积Accuracy, 准确度Actual frequency, 实际频数Adaptive estimator, 自适应估计量Addition, 相加Addition theorem, 加法定理Additivity, 可加性Adjusted rate, 调整率Adjusted value, 校正值Admissible error, 容许误差Aggregation, 聚集性Alternative hypothesis, 备择假设Among groups, 组间Amounts, 总量Analysis of correlation, 相关分析Analysis of covariance, 协方差分析Analysis of regression, 回归分析Analysis of time series, 时间序列分析Analysis of variance, 方差分析Angular transformation, 角转换ANOVA (analysis of variance ), 方差分析ANOVA Models, 方差分析模型Arcing, 弧/弧旋Arcsine transformation, 反正弦变换Area under the curve, 曲线面积AREG , 评估从一个时间点到下一个时间点回归相关时的误差ARIMA, 季节和非季节性单变量模型的极大似然估计Arithmetic grid paper, 算术格纸Arithmetic mean, 算术平均数Arrhenius relation, 艾恩尼斯关系Assessing fit, 拟合的评估Associative laws, 结合律Asymmetric distribution, 非对称分布Asymptotic bias, 渐近偏倚Asymptotic efficiency, 渐近效率Asymptotic variance, 渐近方差Attributable risk, 归因危险度Attribute data, 属性资料Attribution, 属性Autocorrelation, 自相关Autocorrelation of residuals , 残差的自相关Average, 平均数Average confidence interval length, 平均置信区间长度Average growth rate, 平均增长率Bar chart, 条形图Bar graph, 条形图Base period, 基期Bayes' theorem , Bayes定理Bell-shaped curve, 钟形曲线Bernoulli distribution, 伯努力分布Best-trim estimator, 最好切尾估计量Bias, 偏性Binary logistic regression, 二元逻辑斯蒂回归Binomial distribution, 二项分布Bisquare, 双平方Bivariate Correlate, 二变量相关Bivariate normal distributio n, 双变量正态分布Bivariate normal population,双变量正态总体Biweight interval, 双权区间Biweight M-estimator, 双权M 估计量Block, 区组/配伍组BMDP(Biomedical computer pro grams), BMDP统计软件包Boxplots, 箱线图/箱尾图Breakdown bound, 崩溃界/崩溃点Canonical correlation, 典型相关Caption, 纵标目Case-control study, 病例对照研究Categorical variable, 分类变量Catenary, 悬链线Cauchy distribution, 柯西分布Cause-and-effect relationshi p, 因果关系Cell, 单元Censoring, 终检Center of symmetry, 对称中心Centering and scaling, 中心化和定标Central tendency, 集中趋势Central value, 中心值CHAID -χ2 Automatic Interac tion Detector, 卡方自动交互检测Chance, 机遇Chance error, 随机误差Chance variable, 随机变量Characteristic equation, 特征方程Characteristic root, 特征根Characteristic vector, 特征向量Chebshev criterion of fit, 拟合的切比雪夫准则Chernoff faces, 切尔诺夫脸谱图Chi-square test, 卡方检验/χ2检验Choleskey decomposition, 乔洛斯基分解Circle chart, 圆图Class interval, 组距Class mid-value, 组中值Class upper limit, 组上限Classified variable, 分类变量Cluster analysis, 聚类分析Cluster sampling, 整群抽样Code, 代码Coded data, 编码数据Coding, 编码Coefficient of contingency, 列联系数Coefficient of determination , 决定系数Coefficient of multiple corr elation, 多重相关系数Coefficient of partial corre lation, 偏相关系数Coefficient of production-mo ment correlation, 积差相关系数Coefficient of rank correlat ion, 等级相关系数Coefficient of regression, 回归系数Coefficient of skewness, 偏度系数Coefficient of variation, 变异系数Cohort study, 队列研究Column, 列Column effect, 列效应Column factor, 列因素Combination pool, 合并Combinative table, 组合表Common factor, 共性因子Common regression coefficien t, 公共回归系数Common value, 共同值Common variance, 公共方差Common variation, 公共变异Communality variance, 共性方差Comparability, 可比性Comparison of bathes, 批比较Comparison value, 比较值Compartment model, 分部模型Compassion, 伸缩Complement of an event, 补事件Complete association, 完全正相关Complete dissociation, 完全不相关Complete statistics, 完备统计量Completely randomized design , 完全随机化设计Composite event, 联合事件Composite events, 复合事件Concavity, 凹性Conditional expectation, 条件期望Conditional likelihood, 条件似然Conditional probability, 条件概率Conditionally linear, 依条件线性Confidence interval, 置信区间Confidence limit, 置信限Confidence lower limit, 置信下限Confidence upper limit, 置信上限Confirmatory Factor Analysis , 验证性因子分析Confirmatory research, 证实性实验研究Confounding factor, 混杂因素Conjoint, 联合分析Consistency, 相合性Consistency check, 一致性检验Consistent asymptotically no rmal estimate, 相合渐近正态估计Consistent estimate, 相合估计Constrained nonlinear regres sion, 受约束非线性回归Constraint, 约束Contaminated distribution, 污染分布Contaminated Gausssian, 污染高斯分布Contaminated normal distribu tion, 污染正态分布Contamination, 污染Contamination model, 污染模型Contingency table, 列联表Contour, 边界线Contribution rate, 贡献率Control, 对照Controlled experiments, 对照实验Conventional depth, 常规深度Convolution, 卷积Corrected factor, 校正因子Corrected mean, 校正均值Correction coefficient, 校正系数Correctness, 正确性Correlation coefficient, 相关系数Correlation index, 相关指数Correspondence, 对应Counting, 计数Counts, 计数/频数Covariance, 协方差Covariant, 共变Cox Regression, Cox回归Criteria for fitting, 拟合准则Criteria of least squares, 最小二乘准则Critical ratio, 临界比Critical region, 拒绝域Critical value, 临界值Cross-over design, 交叉设计Cross-section analysis, 横断面分析Cross-section survey, 横断面调查Crosstabs , 交叉表Cross-tabulation table, 复合表Cube root, 立方根Cumulative distribution func tion, 分布函数Cumulative probability, 累计概率Curvature, 曲率/弯曲Curvature, 曲率Curve fit , 曲线拟和Curve fitting, 曲线拟合Curvilinear regression, 曲线回归Curvilinear relation, 曲线关系Cut-and-try method, 尝试法Cycle, 周期Cyclist, 周期性D test, D检验Data acquisition, 资料收集Data bank, 数据库Data capacity, 数据容量Data deficiencies, 数据缺乏Data handling, 数据处理Data manipulation, 数据处理Data processing, 数据处理Data reduction, 数据缩减Data set, 数据集Data sources, 数据来源Data transformation, 数据变换Data validity, 数据有效性Data-in, 数据输入Data-out, 数据输出Dead time, 停滞期Degree of freedom, 自由度Degree of precision, 精密度Degree of reliability, 可靠性程度Degression, 递减Density function, 密度函数Density of data points, 数据点的密度Dependent variable, 应变量/依变量/因变量Dependent variable, 因变量Depth, 深度Derivative matrix, 导数矩阵Derivative-free methods, 无导数方法Design, 设计Determinacy, 确定性Determinant, 行列式Determinant, 决定因素Deviation, 离差Deviation from average, 离均差Diagnostic plot, 诊断图Dichotomous variable, 二分变量Differential equation, 微分方程Direct standardization, 直接标准化法Discrete variable, 离散型变量DISCRIMINANT, 判断Discriminant analysis, 判别分析Discriminant coefficient, 判别系数Discriminant function, 判别值Dispersion, 散布/分散度Disproportional, 不成比例的Disproportionate sub-class n umbers, 不成比例次级组含量Distribution free, 分布无关性/免分布Distribution shape, 分布形状Distribution-free method, 任意分布法Distributive laws, 分配律Disturbance, 随机扰动项Dose response curve, 剂量反应曲线Double blind method, 双盲法Double blind trial, 双盲试验Double exponential distribut ion, 双指数分布Double logarithmic, 双对数Downward rank, 降秩Dual-space plot, 对偶空间图DUD, 无导数方法Duncan's new multiple range method, 新复极差法/Duncan新法Effect, 实验效应Eigenvalue, 特征值Eigenvector, 特征向量Ellipse, 椭圆Empirical distribution, 经验分布Empirical probability, 经验概率单位Enumeration data, 计数资料Equal sun-class number, 相等次级组含量Equally likely, 等可能Equivariance, 同变性Error, 误差/错误Error of estimate, 估计误差Error type I, 第一类错误Error type II, 第二类错误Estimand, 被估量Estimated error mean squares , 估计误差均方Estimated error sum of squar es, 估计误差平方和Euclidean distance, 欧式距离Event, 事件Event, 事件Exceptional data point, 异常数据点Expectation plane, 期望平面Expectation surface, 期望曲面Expected values, 期望值Experiment, 实验Experimental sampling, 试验抽样Experimental unit, 试验单位Explanatory variable, 说明变量Exploratory data analysis, 探索性数据分析Explore Summarize, 探索-摘要Exponential curve, 指数曲线Exponential growth, 指数式增长EXSMOOTH, 指数平滑方法Extended fit, 扩充拟合Extra parameter, 附加参数Extrapolation, 外推法Extreme observation, 末端观测值Extremes, 极端值/极值F distribution, F分布F test, F检验Factor, 因素/因子Factor analysis, 因子分析Factor Analysis, 因子分析Factor score, 因子得分Factorial, 阶乘Factorial design, 析因试验设计False negative, 假阴性False negative error, 假阴性错误Family of distributions, 分布族Family of estimators, 估计量族Fanning, 扇面Fatality rate, 病死率Field investigation, 现场调查Field survey, 现场调查Finite population, 有限总体Finite-sample, 有限样本First derivative, 一阶导数First principal component, 第一主成分First quartile, 第一四分位数Fisher information, 费雪信息量Fitted value, 拟合值Fitting a curve, 曲线拟合Fixed base, 定基Fluctuation, 随机起伏Forecast, 预测Four fold table, 四格表Fourth, 四分点Fraction blow, 左侧比率Fractional error, 相对误差Frequency, 频率Frequency polygon, 频数多边图Frontier point, 界限点Function relationship, 泛函关系Gamma distribution, 伽玛分布Gauss increment, 高斯增量Gaussian distribution, 高斯分布/正态分布Gauss-Newton increment, 高斯-牛顿增量General census, 全面普查GENLOG (Generalized liner mo dels), 广义线性模型Geometric mean, 几何平均数Gini's mean difference, 基尼均差GLM (General liner models), 一般线性模型Goodness of fit, 拟和优度/配合度Gradient of determinant, 行列式的梯度Graeco-Latin square, 希腊拉丁方Grand mean, 总均值Gross errors, 重大错误Gross-error sensitivity, 大错敏感度Group averages, 分组平均Grouped data, 分组资料Guessed mean, 假定平均数Half-life, 半衰期Hampel M-estimators, 汉佩尔M估计量Happenstance, 偶然事件Harmonic mean, 调和均数Hazard function, 风险均数Hazard rate, 风险率Heading, 标目Heavy-tailed distribution, 重尾分布Hessian array, 海森立体阵Heterogeneity, 不同质Heterogeneity of variance, 方差不齐Hierarchical classification,组内分组Hierarchical clustering meth od, 系统聚类法High-leverage point, 高杠杆率点HILOGLINEAR, 多维列联表的层次对数线性模型Hinge, 折叶点Histogram, 直方图Historical cohort study, 历史性队列研究Holes, 空洞HOMALS, 多重响应分析Homogeneity of variance, 方差齐性Homogeneity test, 齐性检验Huber M-estimators, 休伯M估计量Hyperbola, 双曲线Hypothesis testing, 假设检验Hypothetical universe, 假设总体Impossible event, 不可能事件Independence, 独立性Independent variable, 自变量Index, 指标/指数Indirect standardization, 间接标准化法Individual, 个体Inference band, 推断带Infinite population, 无限总体Infinitely great, 无穷大Infinitely small, 无穷小Influence curve, 影响曲线Information capacity, 信息容量Initial condition, 初始条件Initial estimate, 初始估计值Initial level, 最初水平Interaction, 交互作用Interaction terms, 交互作用项Intercept, 截距Interpolation, 内插法Interquartile range, 四分位距Interval estimation, 区间估计Intervals of equal probabili ty, 等概率区间Intrinsic curvature, 固有曲率Invariance, 不变性Inverse matrix, 逆矩阵Inverse probability, 逆概率Inverse sine transformation,反正弦变换Iteration, 迭代Jacobian determinant, 雅可比行列式Joint distribution function,分布函数Joint probability, 联合概率Joint probability distributi on, 联合概率分布K means method, 逐步聚类法Kaplan-Meier, 评估事件的时间长度Kaplan-Merier chart, Kaplan-Merier图Kendall's rank correlation, Kendall等级相关Kinetic, 动力学Kolmogorov-Smirnove test, 柯尔莫哥洛夫-斯米尔诺夫检验Kruskal and Wallis test, Kru skal及Wallis检验/多样本的秩和检验/H检验Kurtosis, 峰度Lack of fit, 失拟Ladder of powers, 幂阶梯Lag, 滞后Large sample, 大样本Large sample test, 大样本检验Latin square, 拉丁方Latin square design, 拉丁方设计Leakage, 泄漏Least favorable configuratio n, 最不利构形Least favorable distribution , 最不利分布Least significant difference , 最小显著差法Least square method, 最小二乘法Least-absolute-residuals est imates, 最小绝对残差估计Least-absolute-residuals fit , 最小绝对残差拟合Least-absolute-residuals lin e, 最小绝对残差线Legend, 图例L-estimator, L估计量L-estimator of location, 位置L估计量L-estimator of scale, 尺度L 估计量Level, 水平Life expectance, 预期期望寿命Life table, 寿命表Life table method, 生命表法Light-tailed distribution, 轻尾分布Likelihood function, 似然函数Likelihood ratio, 似然比line graph, 线图Linear correlation, 直线相关Linear equation, 线性方程Linear programming, 线性规划Linear regression, 直线回归Linear Regression, 线性回归Linear trend, 线性趋势Loading, 载荷Location and scale equivaria nce, 位置尺度同变性Location equivariance, 位置同变性Location invariance, 位置不变性Location scale family, 位置尺度族Log rank test, 时序检验Logarithmic curve, 对数曲线Logarithmic normal distribut ion, 对数正态分布Logarithmic scale, 对数尺度Logarithmic transformation, 对数变换Logic check, 逻辑检查Logistic distribution, 逻辑斯特分布Logit transformation, Logit 转换LOGLINEAR, 多维列联表通用模型Lognormal distribution, 对数正态分布Lost function, 损失函数Low correlation, 低度相关Lower limit, 下限Lowest-attained variance, 最小可达方差LSD, 最小显著差法的简称Lurking variable, 潜在变量Main effect, 主效应Major heading, 主辞标目Marginal density function, 边缘密度函数Marginal probability, 边缘概率Marginal probability distrib ution, 边缘概率分布Matched data, 配对资料Matched distribution, 匹配过分布Matching of distribution, 分布的匹配Matching of transformation, 变换的匹配Mathematical expectation, 数学期望Mathematical model, 数学模型Maximum L-estimator, 极大极小L 估计量Maximum likelihood method, 最大似然法Mean, 均数Mean squares between groups,组间均方Mean squares within group, 组内均方Means (Compare means), 均值-均值比较Median, 中位数Median effective dose, 半数效量Median lethal dose, 半数致死量Median polish, 中位数平滑Median test, 中位数检验Minimal sufficient statistic , 最小充分统计量Minimum distance estimation,最小距离估计Minimum effective dose, 最小有效量Minimum lethal dose, 最小致死量Minimum variance estimator, 最小方差估计量MINITAB, 统计软件包Minor heading, 宾词标目Missing data, 缺失值Model specification, 模型的确定Modeling Statistics , 模型统计Models for outliers, 离群值模型Modifying the model, 模型的修正Modulus of continuity, 连续性模Morbidity, 发病率Most favorable configuration , 最有利构形Multidimensional Scaling (AS CAL), 多维尺度/多维标度Multinomial Logistic Regress ion , 多项逻辑斯蒂回归Multiple comparison, 多重比较Multiple correlation , 复相关Multiple covariance, 多元协方差Multiple linear regression, 多元线性回归Multiple response , 多重选项Multiple solutions, 多解Multiplication theorem, 乘法定理Multiresponse, 多元响应Multi-stage sampling, 多阶段抽样Multivariate T distribution,多元T分布Mutual exclusive, 互不相容Mutual independence, 互相独立Natural boundary, 自然边界Natural dead, 自然死亡Natural zero, 自然零Negative correlation, 负相关Negative linear correlation,负线性相关Negatively skewed, 负偏Newman-Keuls method, q检验NK method, q检验No statistical significance,无统计意义Nominal variable, 名义变量Nonconstancy of variability,变异的非定常性Nonlinear regression, 非线性相关Nonparametric statistics, 非参数统计Nonparametric test, 非参数检验Nonparametric tests, 非参数检验Normal deviate, 正态离差Normal distribution, 正态分布Normal equation, 正规方程组Normal ranges, 正常范围Normal value, 正常值Nuisance parameter, 多余参数/讨厌参数Null hypothesis, 无效假设Numerical variable, 数值变量Objective function, 目标函数Observation unit, 观察单位Observed value, 观察值One sided test, 单侧检验One-way analysis of variance , 单因素方差分析Oneway ANOVA , 单因素方差分析Open sequential trial, 开放型序贯设计Optrim, 优切尾Optrim efficiency, 优切尾效率Order statistics, 顺序统计量Ordered categories, 有序分类Ordinal logistic regression , 序数逻辑斯蒂回归Ordinal variable, 有序变量Orthogonal basis, 正交基Orthogonal design, 正交试验设计Orthogonality conditions, 正交条件ORTHOPLAN, 正交设计Outlier cutoffs, 离群值截断点Outliers, 极端值OVERALS , 多组变量的非线性正规相关Overshoot, 迭代过度Paired design, 配对设计Paired sample, 配对样本Pairwise slopes, 成对斜率Parabola, 抛物线Parallel tests, 平行试验Parameter, 参数Parametric statistics, 参数统计Parametric test, 参数检验Partial correlation, 偏相关Partial regression, 偏回归Partial sorting, 偏排序Partials residuals, 偏残差Pattern, 模式Pearson curves, 皮尔逊曲线Peeling, 退层Percent bar graph, 百分条形图Percentage, 百分比Percentile, 百分位数Percentile curves, 百分位曲线Periodicity, 周期性Permutation, 排列P-estimator, P估计量Pie graph, 饼图Pitman estimator, 皮特曼估计量Pivot, 枢轴量Planar, 平坦Planar assumption, 平面的假设PLANCARDS, 生成试验的计划卡Point estimation, 点估计Poisson distribution, 泊松分布Polishing, 平滑Polled standard deviation, 合并标准差Polled variance, 合并方差Polygon, 多边图Polynomial, 多项式Polynomial curve, 多项式曲线Population, 总体Population attributable risk , 人群归因危险度Positive correlation, 正相关Positively skewed, 正偏Posterior distribution, 后验分布Power of a test, 检验效能Precision, 精密度Predicted value, 预测值Preliminary analysis, 预备性分析Principal component analysis , 主成分分析Prior distribution, 先验分布Prior probability, 先验概率Probabilistic model, 概率模型probability, 概率Probability density, 概率密度Product moment, 乘积矩/协方差Profile trace, 截面迹图Proportion, 比/构成比Proportion allocation in str atified random sampling, 按比例分层随机抽样Proportionate, 成比例Proportionate sub-class numb ers, 成比例次级组含量Prospective study, 前瞻性调查Proximities, 亲近性Pseudo F test, 近似F检验Pseudo model, 近似模型Pseudosigma, 伪标准差Purposive sampling, 有目的抽样QR decomposition, QR分解Quadratic approximation, 二次近似Qualitative classification, 属性分类Qualitative method, 定性方法Quantile-quantile plot, 分位数-分位数图/Q-Q图Quantitative analysis, 定量分析Quartile, 四分位数Quick Cluster, 快速聚类Radix sort, 基数排序Random allocation, 随机化分组Random blocks design, 随机区组设计Random event, 随机事件Randomization, 随机化Range, 极差/全距Rank correlation, 等级相关Rank sum test, 秩和检验Rank test, 秩检验Ranked data, 等级资料Rate, 比率Ratio, 比例Raw data, 原始资料Raw residual, 原始残差Rayleigh's test, 雷氏检验Rayleigh's Z, 雷氏Z值Reciprocal, 倒数Reciprocal transformation, 倒数变换Recording, 记录Redescending estimators, 回降估计量Reducing dimensions, 降维Re-expression, 重新表达Reference set, 标准组Region of acceptance, 接受域Regression coefficient, 回归系数Regression sum of square, 回归平方和Rejection point, 拒绝点Relative dispersion, 相对离散度Relative number, 相对数Reliability, 可靠性Reparametrization, 重新设置参数Replication, 重复Report Summaries, 报告摘要Residual sum of square, 剩余平方和Resistance, 耐抗性Resistant line, 耐抗线Resistant technique, 耐抗技术R-estimator of location, 位置R估计量R-estimator of scale, 尺度R 估计量Retrospective study, 回顾性调查Ridge trace, 岭迹Ridit analysis, Ridit分析Rotation, 旋转Rounding, 舍入Row, 行Row effects, 行效应Row factor, 行因素RXC table, RXC表Sample, 样本Sample regression coefficien t, 样本回归系数Sample size, 样本量Sample standard deviation, 样本标准差Sampling error, 抽样误差SAS(Statistical analysis sys tem ), SAS统计软件包Scale, 尺度/量表Scatter diagram, 散点图Schematic plot, 示意图/简图Score test, 计分检验Screening, 筛检SEASON, 季节分析Second derivative, 二阶导数Second principal component, 第二主成分SEM (Structural equation mod eling), 结构化方程模型Semi-logarithmic graph, 半对数图Semi-logarithmic paper, 半对数格纸Sensitivity curve, 敏感度曲线Sequential analysis, 贯序分析Sequential data set, 顺序数据集Sequential design, 贯序设计Sequential method, 贯序法Sequential test, 贯序检验法Serial tests, 系列试验Short-cut method, 简捷法Sigmoid curve, S形曲线Sign function, 正负号函数Sign test, 符号检验Signed rank, 符号秩Significance test, 显著性检验Significant figure, 有效数字Simple cluster sampling, 简单整群抽样Simple correlation, 简单相关Simple random sampling, 简单随机抽样Simple regression, 简单回归simple table, 简单表Sine estimator, 正弦估计量Single-valued estimate, 单值估计Singular matrix, 奇异矩阵Skewed distribution, 偏斜分布Skewness, 偏度Slash distribution, 斜线分布Slope, 斜率Smirnov test, 斯米尔诺夫检验Source of variation, 变异来源Spearman rank correlation, 斯皮尔曼等级相关Specific factor, 特殊因子Specific factor variance, 特殊因子方差Spectra , 频谱Spherical distribution, 球型正态分布Spread, 展布SPSS(Statistical package for the social science), SPSS 统计软件包Spurious correlation, 假性相关Square root transformation, 平方根变换Stabilizing variance, 稳定方差Standard deviation, 标准差Standard error, 标准误Standard error of difference , 差别的标准误Standard error of estimate, 标准估计误差Standard error of rate, 率的标准误Standard normal distribution , 标准正态分布Standardization, 标准化Starting value, 起始值Statistic, 统计量Statistical control, 统计控制Statistical graph, 统计图Statistical inference, 统计推断Statistical table, 统计表Steepest descent, 最速下降法Stem and leaf display, 茎叶图Step factor, 步长因子Stepwise regression, 逐步回归Storage, 存Strata, 层(复数)Stratified sampling, 分层抽样Stratified sampling, 分层抽样Strength, 强度Stringency, 严密性Structural relationship, 结构关系Studentized residual, 学生化残差/t化残差Sub-class numbers, 次级组含量Subdividing, 分割Sufficient statistic, 充分统计量Sum of products, 积和Sum of squares, 离差平方和Sum of squares about regress ion, 回归平方和Sum of squares between group s, 组间平方和Sum of squares of partial re gression, 偏回归平方和Sure event, 必然事件Survey, 调查Survival, 生存分析Survival rate, 生存率Suspended root gram, 悬吊根图Symmetry, 对称Systematic error, 系统误差Systematic sampling, 系统抽样Tags, 标签Tail area, 尾部面积Tail length, 尾长Tail weight, 尾重Tangent line, 切线Target distribution, 目标分布Taylor series, 泰勒级数Tendency of dispersion, 离散趋势Testing of hypotheses, 假设检验Theoretical frequency, 理论频数Time series, 时间序列Tolerance interval, 容忍区间Tolerance lower limit, 容忍下限Tolerance upper limit, 容忍上限Torsion, 扰率Total sum of square, 总平方和Total variation, 总变异Transformation, 转换Treatment, 处理Trend, 趋势Trend of percentage, 百分比趋势Trial, 试验Trial and error method, 试错法Tuning constant, 细调常数Two sided test, 双向检验Two-stage least squares, 二阶最小平方Two-stage sampling, 二阶段抽样Two-tailed test, 双侧检验Two-way analysis of variance , 双因素方差分析Two-way table, 双向表Type I error, 一类错误/α错误Type II error, 二类错误/β错误UMVU, 方差一致最小无偏估计简称Unbiased estimate, 无偏估计Unconstrained nonlinear regr ession , 无约束非线性回归Unequal subclass number, 不等次级组含量Ungrouped data, 不分组资料Uniform coordinate, 均匀坐标Uniform distribution, 均匀分布Uniformly minimum variance u nbiased estimate, 方差一致最小无偏估计Unit, 单元Unordered categories, 无序分类Upper limit, 上限Upward rank, 升秩Vague concept, 模糊概念Validity, 有效性VARCOMP (Variance component estimation), 方差元素估计Variability, 变异性Variable, 变量Variance, 方差Variation, 变异Varimax orthogonal rotation,方差最大正交旋转Volume of distribution, 容积W test, W检验Weibull distribution, 威布尔分布Weight, 权数Weighted Chi-square test, 加权卡方检验/Cochran检验Weighted linear regression m ethod, 加权直线回归Weighted mean, 加权平均数Weighted mean square, 加权平均方差Weighted sum of square, 加权平方和Weighting coefficient, 权重系数Weighting method, 加权法W-estimation, W估计量W-estimation of location, 位置W估计量Width, 宽度Wilcoxon paired test, 威斯康星配对法/配对符号秩和检验Wild point, 野点/狂点Wild value, 野值/狂值Winsorized mean, 缩尾均值Withdraw, 失访Youden's index, 尤登指数Z test, Z检验Zero correlation, 零相关Z-transformation, Z变换Summarize菜单项数值分析过程Frequencies子菜单项单变量的频数分布统计Descriptives子菜单项单变量的描述统计Explore子菜单项指定变量的综合描述统计Crosstabs子菜单项双变量或多变量的各水平组合的频数分布统计Compare Mean菜单项均值比较分析过程Means子菜单项单变量的综合描述统计Independent Sample T test子菜单项独立样本的T检验Paired Sample T test子菜单项配对样本的T检验One-Way ANOVA子菜单项一维方差分析(单变量方差分析)ANOVA Models菜单项多元方差分析过程Simple Factorial子菜单项因子设计的方差分析General Factorial子菜单项一般方差分析Multivariate子菜单项双因变量或多因变量的方差分析Repeated Factorial子菜单项因变量均值校验Correlate菜单项相关分析Bivariate子菜单项Pearson积矩相关矩阵和Kendall、Spearman非参数相关分析Partial子菜单项双变量相关分析Distance子菜单项相似性、非相似性分析Regression菜单项回归分析Liner子菜单项线性回归分析Logistic子菜单项二分变量回归分析(逻辑回归分析)Probit子菜单项概率分析Nonlinear子菜单项非线性回归分析Weight Estimation子菜单项不同权数的线性回归分析2-stage Least Squares子菜单项二阶最小平方回归分析Loglinear菜单项对数线性回归分析General子菜单项一般对数线性回归分析Hierarchical子菜单项多维交叉变量对数回归分析Logit子菜单项单因变量多自变量回归分析Classify菜单项聚类和判别分析K-means Cluster子菜单项指定分类数聚类分析Hierarchical Cluster子菜单项未知分类数聚类分析Discriminent子菜单项聚类判别函数分析Data Reduction菜单项降维、简化数据过程Factor子菜单项因子分析Correspondence Analysis子菜单项对应表(交叉表)分析Homogeneity Analysis子菜单项多重对应分析Nonlinear Components子菜单项非线性成分分析OVERALS子菜单项非线性典则相关分析Scale菜单项Reliability Ananlysis子菜单项加性等级的项目分析Multidimensional Scaling子菜单项多维等级分析Nonparametric Tests菜单项Chi-Square子菜单项相对比例假设检验Binomial子菜单项特定时间发生概率检验Run子菜单项随即序列检验1-Sample Kolmogorov Smirnov子菜单项样本分布检验2-Independent Samples子菜单项双不相关组分布分析K Independent Samples子菜单项多不相关组分布分析2 Related Samples子菜单项双相关变量分布分析McNemar' test子菜单项相关样本比例变化分析K Related Samples子菜单项相关变量分布分析Cocharn's Q test子菜单项二分变量均数检验Kendall's W子菜单项一致性判定。
机器学习设计知识测试 选择题 53题

1. 在机器学习中,监督学习的主要目标是:A) 从无标签数据中学习B) 从有标签数据中学习C) 优化模型的复杂度D) 减少计算资源的使用2. 下列哪种算法属于无监督学习?A) 线性回归B) 决策树C) 聚类分析D) 支持向量机3. 在机器学习模型评估中,交叉验证的主要目的是:A) 增加模型复杂度B) 减少数据集大小C) 评估模型的泛化能力D) 提高训练速度4. 下列哪项不是特征选择的方法?A) 主成分分析(PCA)B) 递归特征消除(RFE)C) 网格搜索(Grid Search)D) 方差阈值(Variance Threshold)5. 在深度学习中,卷积神经网络(CNN)主要用于:A) 文本分析B) 图像识别C) 声音处理D) 推荐系统6. 下列哪种激活函数在神经网络中最为常用?A) 线性激活函数B) 阶跃激活函数C) ReLUD) 双曲正切函数7. 在机器学习中,过拟合通常是由于以下哪种情况引起的?A) 模型过于简单B) 数据量过大C) 模型过于复杂D) 数据预处理不当8. 下列哪项技术用于处理类别不平衡问题?A) 数据增强B) 重采样C) 特征选择D) 模型集成9. 在自然语言处理(NLP)中,词嵌入的主要目的是:A) 提高计算效率B) 减少词汇量C) 捕捉词之间的语义关系D) 增加文本长度10. 下列哪种算法不属于集成学习方法?A) 随机森林B) AdaBoostC) 梯度提升机(GBM)D) 逻辑回归11. 在机器学习中,ROC曲线用于评估:A) 模型的准确性B) 模型的复杂度C) 模型的泛化能力D) 分类模型的性能12. 下列哪项不是数据预处理的步骤?A) 缺失值处理B) 特征缩放C) 模型训练D) 数据标准化13. 在机器学习中,L1正则化主要用于:A) 减少模型复杂度B) 增加特征数量C) 特征选择D) 提高模型精度14. 下列哪种方法可以用于处理时间序列数据?A) 主成分分析(PCA)B) 线性回归C) ARIMA模型D) 决策树15. 在机器学习中,Bagging和Boosting的主要区别在于:A) 数据处理方式B) 模型复杂度C) 样本使用方式D) 特征选择方法16. 下列哪种算法适用于推荐系统?A) K-均值聚类B) 协同过滤C) 逻辑回归D) 随机森林17. 在机器学习中,A/B测试主要用于:A) 模型选择B) 特征工程C) 模型评估D) 用户体验优化18. 下列哪种方法可以用于处理缺失数据?A) 删除含有缺失值的样本B) 使用均值填充C) 使用中位数填充D) 以上都是19. 在机器学习中,偏差-方差权衡主要关注:A) 模型的复杂度B) 数据集的大小C) 模型的泛化能力D) 特征的数量20. 下列哪种算法属于强化学习?A) Q-学习B) 线性回归C) 决策树D) 支持向量机21. 在机器学习中,特征工程的主要目的是:A) 减少数据量B) 增加模型复杂度C) 提高模型性能D) 简化数据处理22. 下列哪种方法可以用于处理多分类问题?A) 一对多(One-vs-All)B) 一对一(One-vs-One)C) 层次聚类D) 以上都是23. 在机器学习中,交叉熵损失函数主要用于:A) 回归问题B) 分类问题C) 聚类问题D) 强化学习24. 下列哪种算法不属于深度学习?A) 卷积神经网络(CNN)B) 循环神经网络(RNN)C) 随机森林D) 长短期记忆网络(LSTM)25. 在机器学习中,梯度下降算法的主要目的是:A) 减少特征数量B) 优化模型参数C) 增加数据量D) 提高计算速度26. 下列哪种方法可以用于处理文本数据?A) 词袋模型(Bag of Words)B) TF-IDFC) 词嵌入D) 以上都是27. 在机器学习中,正则化的主要目的是:A) 减少特征数量B) 防止过拟合C) 增加数据量D) 提高计算速度28. 下列哪种算法适用于异常检测?A) 线性回归B) 决策树C) 支持向量机D) 孤立森林(Isolation Forest)29. 在机器学习中,集成学习的主要目的是:A) 提高单个模型的性能B) 结合多个模型的优势C) 减少数据量D) 增加模型复杂度30. 下列哪种方法可以用于处理高维数据?A) 主成分分析(PCA)B) 特征选择C) 特征提取D) 以上都是31. 在机器学习中,K-均值聚类的主要目的是:A) 分类B) 回归C) 聚类D) 预测32. 下列哪种算法适用于时间序列预测?A) 线性回归B) ARIMA模型C) 决策树D) 支持向量机33. 在机器学习中,网格搜索(Grid Search)主要用于:A) 特征选择B) 模型选择C) 数据预处理D) 模型评估34. 下列哪种方法可以用于处理类别特征?A) 独热编码(One-Hot Encoding)B) 标签编码(Label Encoding)C) 特征哈希(Feature Hashing)D) 以上都是35. 在机器学习中,AUC-ROC曲线的主要用途是:A) 评估分类模型的性能B) 评估回归模型的性能C) 评估聚类模型的性能D) 评估强化学习模型的性能36. 下列哪种算法不属于监督学习?A) 线性回归B) 决策树C) 聚类分析D) 支持向量机37. 在机器学习中,特征缩放的主要目的是:A) 减少特征数量B) 提高模型性能C) 增加数据量D) 简化数据处理38. 下列哪种方法可以用于处理文本分类问题?A) 词袋模型(Bag of Words)B) TF-IDFC) 词嵌入D) 以上都是39. 在机器学习中,决策树的主要优点是:A) 易于理解和解释B) 计算效率高C) 对缺失值不敏感D) 以上都是40. 下列哪种算法适用于图像分割?A) 卷积神经网络(CNN)B) 循环神经网络(RNN)C) 随机森林D) 支持向量机41. 在机器学习中,L2正则化主要用于:A) 减少模型复杂度B) 增加特征数量C) 特征选择D) 提高模型精度42. 下列哪种方法可以用于处理时间序列数据的季节性?A) 移动平均B) 季节分解C) 差分D) 以上都是43. 在机器学习中,Bagging的主要目的是:A) 减少模型的方差B) 减少模型的偏差C) 增加数据量D) 提高计算速度44. 下列哪种算法适用于序列数据处理?A) 卷积神经网络(CNN)B) 循环神经网络(RNN)C) 随机森林D) 支持向量机45. 在机器学习中,AdaBoost的主要目的是:A) 减少模型的方差B) 减少模型的偏差C) 增加数据量D) 提高计算速度46. 下列哪种方法可以用于处理文本数据的情感分析?A) 词袋模型(Bag of Words)B) TF-IDFC) 词嵌入D) 以上都是47. 在机器学习中,支持向量机(SVM)的主要优点是:A) 适用于高维数据B) 计算效率高C) 对缺失值不敏感D) 以上都是48. 下列哪种算法适用于推荐系统中的用户行为分析?A) 协同过滤B) 内容过滤C) 混合过滤D) 以上都是49. 在机器学习中,交叉验证的主要类型包括:A) K-折交叉验证B) 留一法交叉验证C) 随机划分交叉验证D) 以上都是50. 下列哪种方法可以用于处理图像数据?A) 卷积神经网络(CNN)B) 循环神经网络(RNN)C) 随机森林D) 支持向量机51. 在机器学习中,梯度提升机(GBM)的主要优点是:A) 适用于高维数据B) 计算效率高C) 对缺失值不敏感D) 以上都是52. 下列哪种算法适用于异常检测中的离群点检测?A) 线性回归B) 决策树C) 支持向量机D) 孤立森林(Isolation Forest)53. 在机器学习中,特征提取的主要目的是:A) 减少特征数量B) 提高模型性能C) 增加数据量D) 简化数据处理答案:1. B2. C3. C4. C5. B6. C7. C8. B9. C10. D11. D12. C13. C14. C15. C16. B17. D18. D19. C20. A21. C22. D23. B24. C25. B26. D27. B28. D29. B30. D31. C32. B33. B34. D35. A36. C37. B38. D39. D40. A41. A42. D43. A44. B45. B46. D47. A48. D49. D50. A51. D52. D53. B。
Molecular Computing
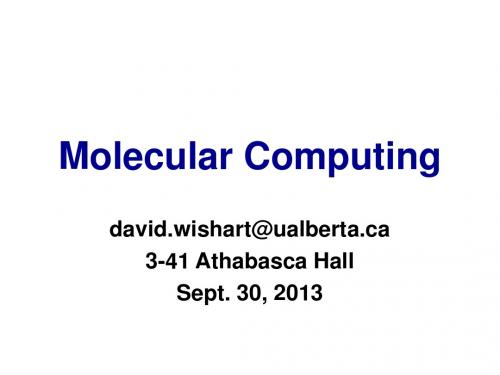
Computing With DNA
Leonard Adleman**
• • • • • Mathematician, computer scientist, boxer Specialized in cryptography (RSA, 1983) Invented the term computer virus (1984) Became intrigued by “real” viruses (HIV) Published a paper on HIV in 1993 and decided to learn molecular biology • Came up with DNA computing (1994) while studying “Molecular Biology of the Gene” • Member of NAS, won Turing prize in 2002
Principles of PCR
Gels & PCR
Not Exactly Routine “Calculations”
Advantages of DNA Computing
• With bases spaced at 0.35 nm along DNA, data density is 400,000 Gbits/cm compared to 3 Gbits/cm in typical high performance hard drive • 1 gram of DNA can hold about 1x1014 MB of data • A test tube of DNA can contain trillions of strands. Each operation on a test tube of DNA is carried out on all strands in the tube in parallel • Adleman figured his computer was running 2 x 1019 operations per joule
双重机器学习代码
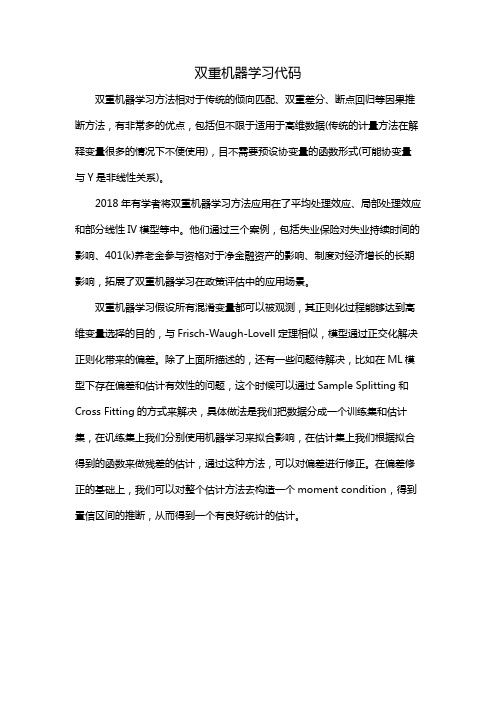
双重机器学习代码
双重机器学习方法相对于传统的倾向匹配、双重差分、断点回归等因果推断方法,有非常多的优点,包括但不限于适用于高维数据(传统的计量方法在解释变量很多的情况下不便使用),目不需要预设协变量的函数形式(可能协变量与Y是非线性关系)。
2018年有学者将双重机器学习方法应用在了平均处理效应、局部处理效应和部分线性IV模型等中。
他们通过三个案例,包括失业保险对失业持续时间的影响、401(k)养老金参与资格对于净金融资产的影响、制度对经济增长的长期影响,拓展了双重机器学习在政策评估中的应用场景。
双重机器学习假设所有混淆变量都可以被观测,其正则化过程能够达到高维变量选择的目的,与Frisch-Waugh-Lovell定理相似,模型通过正交化解决正则化带来的偏差。
除了上面所描述的,还有一些问题待解决,比如在ML模型下存在偏差和估计有效性的问题,这个时候可以通过Sample Splitting和Cross Fitting的方式来解决,具体做法是我们把数据分成一个训练集和估计集,在讥练集上我们分别使用机器学习来拟合影响,在估计集上我们根据拟合得到的函数来做残差的估计,通过这种方法,可以对偏差进行修正。
在偏差修正的基础上,我们可以对整个估计方法去构造一个moment condition,得到置信区间的推断,从而得到一个有良好统计的估计。
USP1010_分析数据解析与处理_CN
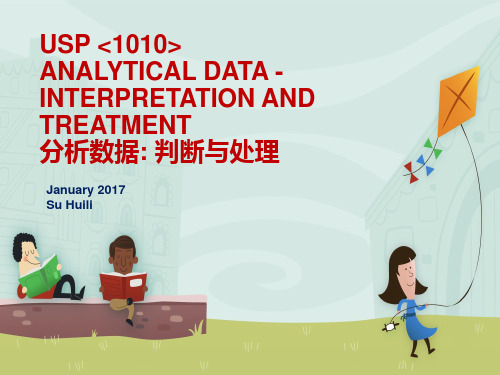
检测原则与变异
如: 变量呈正态分布, 但右边拖尾更长. 此类分布 通常可以进行对数转换, 使之更呈正态. 另一个方法就是使用”分布不拘”或”非参数”统 计法, 这些方法不需要集合形状为正态分布. 若需要建立平均值或平均值差异的置信区间, 根 据中心极限定理central limit theorem, 正态假 设就显得不重要了
• 有效的取样是评估总体质量特性的重要步骤; 取 样目的是提供代表性数据(样品数据),以评估总体 特性. • 如何取样与样品数据有关 • 随机取样是合适的取样方法. 必须随机, 独立取 样, 确保生成的数据能够有效评估总体特性. • 非随机或”方便”的样本存在偏见性评估可能性 的风险
实验室必备的规范与原则:
出现异常值时, 必须进行系统的实验室调查,甚至 工艺调查, 从而找出产生异常结果的原因.
异常结果
调查异常结果必须考虑以下因素, 至少包括
人员差错 仪器差错 计算错误 产品或包材缺陷
若确定原因与产品或包材无关, 则可以对原样进行复验; 可能的话, 对新样品进行检验.
应调查方法的精密度, 准确度, USP标准品, 工艺趋势, 标 准限度;
检测原则与变异
应进行精密度研究, 对分析方法变异性有更好的评价. 可进行中 间精密度研究(包括”组间”与”组内”变异性)和重复性研究(组内 变异性) 中间精密度应能够允许预期的实验条件的变化,如不同化验员, 不 同试剂溶液, 不同天以及不同仪器. 精密度需要重复进行多次检验. 每次检验必须完全独立进行, 以 对不同组分变异性进行准确评估. 此外, 每组检验时, 应进行重复检验, 从而评估重复性. 精密度试验详见附录B
ai工程师面试常见的100道题
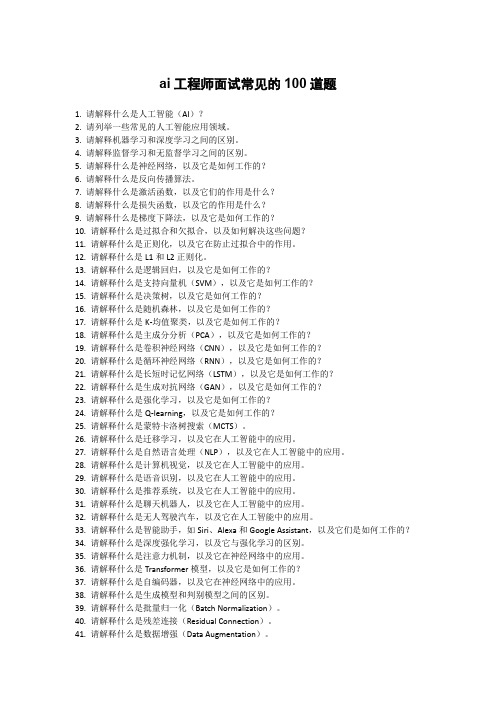
ai工程师面试常见的100道题1. 请解释什么是人工智能(AI)?2. 请列举一些常见的人工智能应用领域。
3. 请解释机器学习和深度学习之间的区别。
4. 请解释监督学习和无监督学习之间的区别。
5. 请解释什么是神经网络,以及它是如何工作的?6. 请解释什么是反向传播算法。
7. 请解释什么是激活函数,以及它们的作用是什么?8. 请解释什么是损失函数,以及它的作用是什么?9. 请解释什么是梯度下降法,以及它是如何工作的?10. 请解释什么是过拟合和欠拟合,以及如何解决这些问题?11. 请解释什么是正则化,以及它在防止过拟合中的作用。
12. 请解释什么是L1和L2正则化。
13. 请解释什么是逻辑回归,以及它是如何工作的?14. 请解释什么是支持向量机(SVM),以及它是如何工作的?15. 请解释什么是决策树,以及它是如何工作的?16. 请解释什么是随机森林,以及它是如何工作的?17. 请解释什么是K-均值聚类,以及它是如何工作的?18. 请解释什么是主成分分析(PCA),以及它是如何工作的?19. 请解释什么是卷积神经网络(CNN),以及它是如何工作的?20. 请解释什么是循环神经网络(RNN),以及它是如何工作的?21. 请解释什么是长短时记忆网络(LSTM),以及它是如何工作的?22. 请解释什么是生成对抗网络(GAN),以及它是如何工作的?23. 请解释什么是强化学习,以及它是如何工作的?24. 请解释什么是Q-learning,以及它是如何工作的?25. 请解释什么是蒙特卡洛树搜索(MCTS)。
26. 请解释什么是迁移学习,以及它在人工智能中的应用。
27. 请解释什么是自然语言处理(NLP),以及它在人工智能中的应用。
28. 请解释什么是计算机视觉,以及它在人工智能中的应用。
29. 请解释什么是语音识别,以及它在人工智能中的应用。
30. 请解释什么是推荐系统,以及它在人工智能中的应用。
31. 请解释什么是聊天机器人,以及它在人工智能中的应用。
利用随机森林构建分类模型,并用十折交叉验证。r语言教程

利用随机森林构建分类模型,并用十折交叉验证。
r语言教程在R语言中,我们可以使用`caret`包中的`train`函数进行模型的训练,并使用`caret`包的`createDataPartition`函数进行十折交叉验证。
以下是使用随机森林构建分类模型的示例代码:首先,确保你已经安装了必要的包。
如果没有,你可以使用以下命令进行安装:```r("caret")("randomForest")```然后,加载这些包:```rlibrary(caret)library(randomForest)接下来,我们需要加载数据。
假设我们有一个名为`data`的数据框,其中包含我们的特征和目标变量:```rdata <- ("your_") 请将"your_"替换为你的数据文件路径```然后,我们将使用`createDataPartition`函数进行十折交叉验证的数据分割:```r(123) 为了结果的可重复性control <- rbind(trainControl(method = "cv", number = 10), 10折交叉验证trainControl(method = "oob") 用于随机森林的外部验证)```接着,我们将使用`train`函数训练我们的模型:(123) 为了结果的可重复性rf_model <- train(target ~ ., data = data, trControl = control, method = "rf") 使用随机森林方法训练模型```最后,我们可以输出模型的详细信息:```rprint(rf_model)```以上代码演示了如何使用随机森林和十折交叉验证在R语言中构建分类模型。
请注意,你可能需要根据自己的数据和需求对代码进行一些调整。
findvariablefeatures函数每个参数的意义 -回复

findvariablefeatures函数每个参数的意义-回复参数1:dataframe数据集(DataFrame),包含要分析的变量。
参数2:target_variable目标变量(str),要分析的特定变量。
参数3:exclude_variables要排除的变量列表(list),不需要分析的变量。
参数4:correlation_threshold相关性阈值(float),用于确定要保留的相关性较强的特征。
参数5:importance_threshold特征重要性阈值(float),用于确定要保留的重要特征。
参数6:n_features要选择的变量的数量(int)。
如果不指定,则选择所有变量。
参数7:random_state随机种子(int),用于复现随机过程。
参数8:categorical_encoding分类变量编码方式(str),用于将分类变量转换为数值变量。
默认为None,即不进行编码。
参数9:feature_selection_method特征选择方法(str),用于选择变量的方法。
默认为"correlation",即通过相关性选择。
参数10:model机器学习模型(object),用于选择变量的方法为"importance"时需要指定一个机器学习模型。
参数11:model_parameters机器学习模型的参数(dict),用于选择变量的方法为"importance"时需要指定机器学习模型的参数。
参数12:scoring评估指标(str),用于选择变量的方法为"importance"时需要指定一个评估指标。
参数13:cv交叉验证折数(int),用于选择变量的方法为"importance"时需要指定交叉验证的折数。
参数14:n_jobs并行执行的作业数(int),默认为1。
如果为-1,则使用所有处理器。
- 1、下载文档前请自行甄别文档内容的完整性,平台不提供额外的编辑、内容补充、找答案等附加服务。
- 2、"仅部分预览"的文档,不可在线预览部分如存在完整性等问题,可反馈申请退款(可完整预览的文档不适用该条件!)。
- 3、如文档侵犯您的权益,请联系客服反馈,我们会尽快为您处理(人工客服工作时间:9:00-18:30)。
1010_Analytical_data_interpretation_and_treatment_分析数据解释及处理<1010>Analytical data interpretation and treatment 分析数据解释及处理IntroductionThis chapter provides information regarding acceptable practices for the analysis and consistent interpretation of data obtained from chemical and other analyses.Basic statistical approaches for evaluating data are described,and the treatment of outliers and comparison of analytical methods are discussed in some detail.序论这一章节提供了对从化学和其他的实验中获得的信息如何进行合理分析和一致解释的信息。
评估数据的基本统计方法,如何处理极端数据及分析方法的比较在一些章节中进行讨论。
NOTE---It should not be inferred that the analysis tools mentioned in this chapter form an exhaustive list .Other ,equallyvalid,statistical methods may be used at the discretion of the manufacture and other users of this chapter.注意---没必要从一个详细的清单中推断这一章节提到的分析工具。
其他等效的统计方法也可能被用于慎密的制造或被其他用户使用。
Assurance of the quality of pharmaceuticals is accomplished by combining a number of practices,including robust formulationdesign,validation,testing of starting materials,in-process testing ,and final-product testing,Each of these practices is dependent onreliable test methods.In the development process ,test procedures are developed and vaildated to ensure that the manufactured products are thoroughly characterized. Final-product testing provides further assurance that the products are consistently safe ,efficacious,and in compliance with their specification.Measurements are inherently variable .The variability of biological tests has long been recognized by the USP,For example ,the need to consider this variability when analyzing biological test data is addressed under Design and Analysis of Biological Assays<111>.The chemical analysis measurements commonly used to analyze pharmaceuticals are also inherently variable,although less so than those of the biological tests.However,in many instances the acceptance criteria are proportionally tighter,and thus ,this smaller allowable variability has to be considered when analyzing data generated using analytical procedures.If the variability of a measurement is not characterized and stated along with the result of the measurement, then the data can only be interpreted in the most limited sense.For example ,stating that the difference between the averages from two laboratories when testing a commom set of samples is 10% has limited interpretation ,in terms of howimportant such a difference is , without knowledge of theintralaboratory variability.This chapter provides direction for scientifically acceptabletreatment and interpretation of data .Statistical tools that may be helpful in the interpretation of analytical data are described.Many descriptive statistics, such as the mean and standard deviation ,are in commom use.Other statistical tools ,such as outlier tests,can be performed using several different,scientifically valid approaches , and examples of these tools and their applications are also included. The framwork within which the results from a compendial test are interpreted is clearly outlined in Test Results,Statistics,and Standards under General Notices and Requirements.Selected references that might be helpful in obtaining additional information on the statistical tools discussed in this chapter are listed in Appendix F at the end of the chapter. USP does not endorse these citations,and they do not represent an exhaustive list. Further information about many of the methods cited in this chapter may also be found in most ststistical textbooks.PREREQUISITE LABORATORY PRACTICES ANDPRINCIPLESThe sound application of statistical principles to laboratory data requires the assumption that such data have been collected in a traceable (i.e.,documents) and unbiased manner.To ensure this ,the following practices are beneficial.Sound Record KeepingLaboratory records are maintained with sufficient detail ,so that other equally qualified analysts can reconstruct the experimental conditions and review the results obtained.When collecting data,the data should generally be obtained with more decimal places than the specification requires and rounded only after final calculations are completed as per the General Notices and Requirements.Sampling ConsiderationsEffective sampling is an important step in the assessment of aquality attribute of a population . The purpose of sampling is toprovide representative data(the sample) for estimating the properties of the population. How to attain such a sample depends entirely on the question that is to be answered by the sample data . In general,use for random process is considered the most appropriate way of selecting a sample.Indeed ,a random and independent sample is necessary to ensurethat the resulting data produce valid estimates of the properties of the population.Generating a nonrandom or “convenience” sample risks the possibility that the estimates will be biased, The moststraightforward type of random sampling is called sample random sampling, a process inwhich every unit of the population has an equal chance of appearingin the sample. However , sometimes this method of selecting a random sample is not optimal because it cannot guarantee equal representation among factors (i.e.,time ,location,machine) that may influnece thecritical properties of the population. For example,if it requires 12 hours tomanufacture all of the units in a lot and it is vital that the sample be representative of the entire production process , then taking a simple random sample after the production has been completed may not be appropriate because there can be no guarantee that such a sample will contain a similar number of units made from every time period within the 12-hour process. Instead, it is better to take a systematic random sample where by a unit is randomly selected from the production process at systematically selected times or locations(e.g.,sample every 30 minutes from the units produced at that time) to ensure that units taken throughout the entire manufacturing process are included in the sample.Another type of random sampling procedure is needed if ,for example,a product is filled into vials using four different filling machines .In this case it would be important to capture a random sample of vials from each of the filling machines .A stratified random sample, which randomly samples an equalnumber of vials from each of the four filling machines ,wouldsatisfy this requirement . Regardless of the reason for taking a sample(e.g.,batch-release testing ), a sampling plan should be established to provide details on how the sample is to be obtained to ensure that the sample is representative of the entirety of the population and that the resulting data have the required sensitivity . The optimal samplingstrategy will depend on knowledge of the manufacturing and analytical measurement processes.Once the sampling scheme has been defined,it is likely that the sampling will include some element of randomselection . Finally , there must be sufficient sample collected for the original analysis, subsequent verification analyses , and other analyses.Tests discussed in the remainder of this chapter assume that simple random sampling has been performed.Use of Reference StandardsWhere the use of the USP Reference Standard is specified, the USP Reference Standard, or a secondary standard traceable to the USP Reference Standard, is used . Because the assignment of a value to a standard is one of the most important factors that influences theaccurary of an analysis,it is critical that this be done correctly.System Performance VerificationVerifying an acceptable level of performance for an analyticalsystem in routine or continuous use can be a valuable practice . Thismay be accomplished by analyzing a control sample at appropriateintervals , or using other means ,such as ,variation among the standards, backgroud signal-to-noise ratios,etc. Attention to the measuredparameter , such as charting the results obtained by analysis of acontrol sample , can signal a change in performance that requries adjustment of the analytical system.Method ValidationAll methods are appropriately validated as specified underValidation of Compendial Methods <1225>.Methods published in the USP-NF have been validated and meet the Current Good Manufacturing Practices regulatory requirement for validation as established in the Code of Federal Regulations, A validated method may be used totest a new formulation (such as a new product,dosage form, or process intermediate) only after confirming that the new formulation does not interfere with the accurary ,linearity ,or precision of the method.It may not be assumed that a validated method could correctly measure the active ingredient in a formulation that is different from that used in establishing the original validity of the method.MEASUREMENT PRINCIPLES AND VARIATIONAll measurement are, at best, estimates of th e actual (“true”or “accepted”) value for they contain random variability (also referred to as random error) and may also contain systematic variation (bias). Thus,the measured value differs from the actual value because of variability inherent in the measurement .If an array of measurement consists of individual results that are representative of thewhole,statistical methods can be used to estimate informative properties of the entirety, and statistical tests are available to investigate whether it is likely that these properties comply with given requirements . The resulting statistical analyses should address the variability associated with the measurement process as well as that ofthe entity being measured . Statistical measures used to assess the direction and magnitude of these errors include themean,standard deviation , and expressions derived therefrom ,such as the coefficient of variation (CV, also called the relative standard deviation , RSD).The estimated variability can be used calculate confidence intervals for the maen, or measures of variability , and tolerance intervals capturing a specified proportion of the individual measurements.The use of statistical measures must be tempered with goodjudgment , especially with regard to representative sampling. Mostof the statistical measures and tests cited in this chapter rely on the assumptions that the distribution of the entire population is represented by a normal distribution and that the analyzed sample is a representative subset of this population. The normal (or Gaussian) distribution is bell-shaped and symmetric about its center and has certain characteristics that are required for these tests to be valid. If the assumption of a normal distribution for the population is not warranted, then normality can often be achieved (at least approximately) through an approptriate transformation of the measurement values.For example, there exist variables that have distributions with longer right tails than left. Such distributions can often be made approximately normal through a log transformation. An alternative approach would be to use “distribution-free” or“nonparametric”statistical procedures that do not require that the shape of the population be that of a normal distribution . When the objective is to construct a confidence interval for the mean or for the differencebetween。