ABSTRACT
英语摘要写法
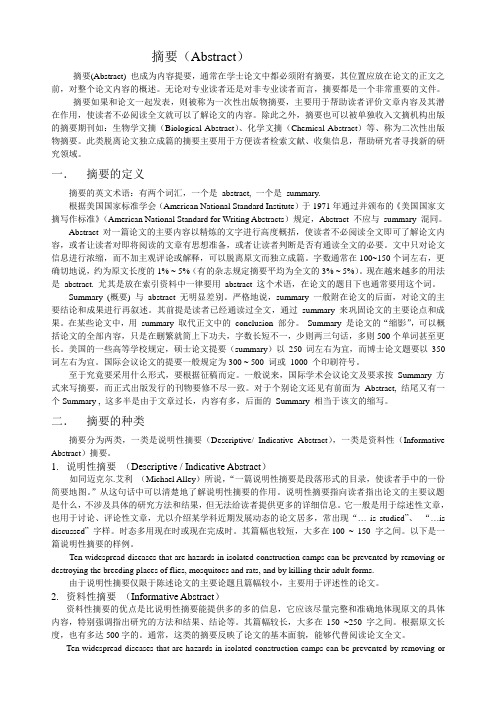
摘要(Abstract)摘要(Abstract) 也成为内容提要,通常在学士论文中都必须附有摘要,其位置应放在论文的正文之前,对整个论文内容的概述。
无论对专业读者还是对非专业读者而言,摘要都是一个非常重要的文件。
摘要如果和论文一起发表,则被称为一次性出版物摘要,主要用于帮助读者评价文章内容及其潜在作用,使读者不必阅读全文就可以了解论文的内容。
除此之外,摘要也可以被单独收入文摘机构出版的摘要期刊如:生物学文摘(Biological Abstract)、化学文摘(Chemical Abstract)等、称为二次性出版物摘要。
此类脱离论文独立成篇的摘要主要用于方便读者检索文献、收集信息,帮助研究者寻找新的研究领域。
一.摘要的定义摘要的英文术语:有两个词汇,一个是abstract, 一个是summary.根据美国国家标准学会(American National Standard Institute)于1971年通过并颁布的《美国国家文摘写作标准》(American National Standard for Writing Abstracts)规定,Abstract 不应与summary 混同。
Abstract 对一篇论文的主要内容以精炼的文字进行高度概括,使读者不必阅读全文即可了解论文内容,或者让读者对即将阅读的文章有思想准备,或者让读者判断是否有通读全文的必要。
文中只对论文信息进行浓缩,而不加主观评论或解释,可以脱离原文而独立成篇。
字数通常在100~150个词左右,更确切地说,约为原文长度的1% ~ 5%(有的杂志规定摘要平均为全文的3% ~ 5%)。
现在越来越多的用法是abstract. 尤其是放在索引资料中一律要用abstract 这个术语,在论文的题目下也通常要用这个词。
Summary (概要) 与abstract 无明显差别。
严格地说,summary 一般附在论文的后面,对论文的主要结论和成果进行再叙述。
4个步骤教你如何写好abstract部分
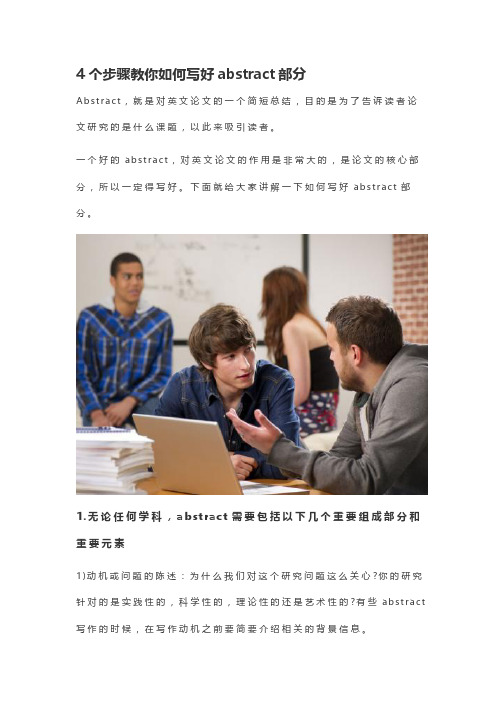
4个步骤教你如何写好abstract部分A b s t r a c t,就是对英文论文的一个简短总结,目的是为了告诉读者论文研究的是什么课题,以此来吸引读者。
一个好的a b s t r a c t,对英文论文的作用是非常大的,是论文的核心部分,所以一定得写好。
下面就给大家讲解一下如何写好a b s t r a c t部分。
1.无论任何学科,a b s t rac t需要包括以下几个重要组成部分和重要元素1)动机或问题的陈述:为什么我们对这个研究问题这么关心?你的研究针对的是实践性的,科学性的,理论性的还是艺术性的?有些a b s t r a c t 写作的时候,在写作动机之前要简要介绍相关的背景信息。
2)措施/流程/解决方法以及调查范围:为了得到相关的结果,你做了什么?3)结果/发现/产品:在上述的调查研究完成后,你学会了什么?发现了什么?创造了什么?4)结论/影响:你得出的哪些结论会产生更大的影响?然而,需要注意的是,根据不同的学科,要注意相关的可变性因素。
2.a b s t rac t中的语法特点和要求1)注意到细微的变化在陈述主要目标和调查范围的时候,多采用陈述性语言。
2)采用合适的方法描述方法的时候多使用过去时态。
要注意时态的一致性。
如果有必要,要按照时间的顺序进行排列。
3)注意结果的呈现方式只需要呈现结果即可。
以过去时态呈现出来。
4)注意结论的可靠性陈述个人观点。
在陈述主要结论的时候要使用现在时。
不要表现出试探性的倾向。
3.a b s t rac t s的类型a b s t r a c t s分为两种类型,i n f o r m a t i o n a l a b s t r a c t s和d e s c r i p t i v ea b s t r a c t s。
I n f o r m a t i o n a l A b s t r a c t s--针对r e p o r t s的相关内容进行沟通。
Abstract写作常用句型及句式
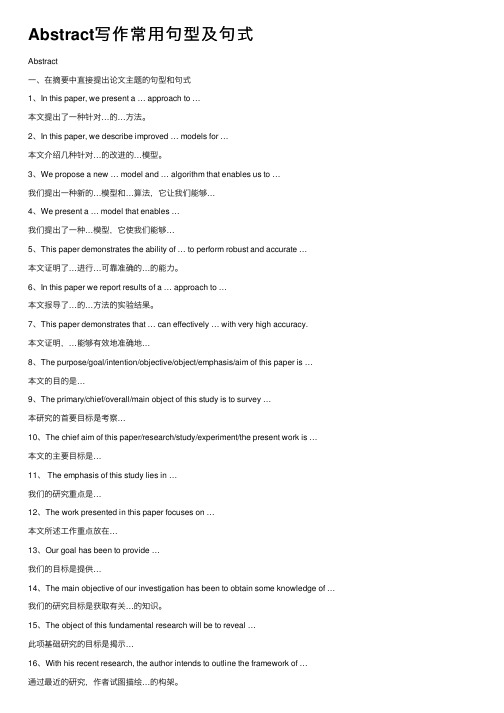
Abstract写作常⽤句型及句式Abstract⼀、在摘要中直接提出论⽂主题的句型和句式1、In this paper, we present a … approach to …本⽂提出了⼀种针对…的…⽅法。
2、In this paper, we describe improved … models for …本⽂介绍⼏种针对…的改进的…模型。
3、We propose a new … model and … algorithm that enables us to …我们提出⼀种新的…模型和…算法,它让我们能够…4、We present a … model that enables …我们提出了⼀种…模型,它使我们能够…5、This paper demonstrates the ability of … to perform robust and accurate …本⽂证明了…进⾏…可靠准确的…的能⼒。
6、In this paper we report results of a … approach to …本⽂报导了…的…⽅法的实验结果。
7、This paper demonstrates that … can effectively … with very high accuracy.本⽂证明,…能够有效地准确地…8、The purpose/goal/intention/objective/object/emphasis/aim of this paper is …本⽂的⽬的是…9、The primary/chief/overall/main object of this study is to survey …本研究的⾸要⽬标是考察…10、The chief aim of this paper/research/study/experiment/the present work is …本⽂的主要⽬标是…11、 The emphasis of this study lies in …我们的研究重点是…12、The work presented in this paper focuses on …本⽂所述⼯作重点放在…13、Our goal has been to provide …我们的⽬标是提供…14、The main objective of our investigation has been to obtain some knowledge of …我们的研究⽬标是获取有关…的知识。
考研英语:词汇abstract的中文翻译解析
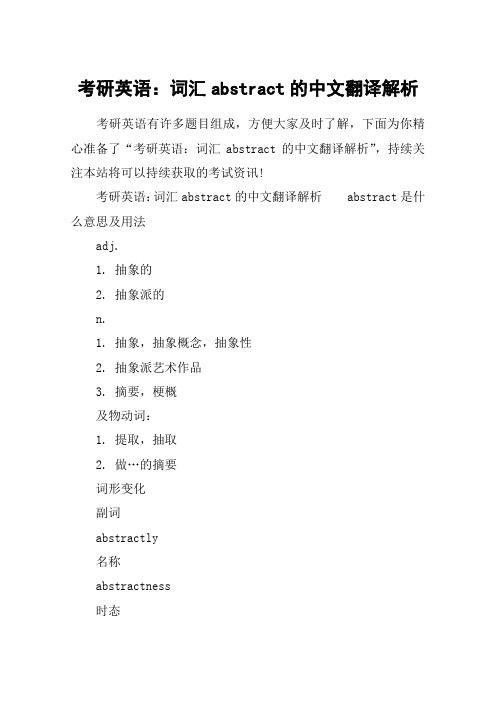
考研英语:词汇abstract的中文翻译解析考研英语有许多题目组成,方便大家及时了解,下面为你精心准备了“考研英语:词汇abstract的中文翻译解析”,持续关注本站将可以持续获取的考试资讯!考研英语:词汇abstract的中文翻译解析abstract是什么意思及用法adj.1. 抽象的2. 抽象派的n.1. 抽象,抽象概念,抽象性2. 抽象派艺术作品3. 摘要,梗概及物动词:1. 提取,抽取2. 做…的摘要词形变化副词abstractly名称abstractness时态abstracted,abstracting,abstracts英语解释not representing or imitating external reality or the objects of naturemake off with belongings of othersdealing with a subject in the abstract without practical purpose or intentionconsider a concept without thinking of a specific example consider abstractly or theoreticallyconsider apart from a particular case or instancegive an abstract (of)a concept or idea not associated with any specific instancea sketchy summary of the main points of an argument or theoryexisting only in the mind separated from embodiment 例句She beheaded me, and flung my head into abstract space 她切下了我的头颅,把它扔进抽象的空间。
abstract谐音法记忆
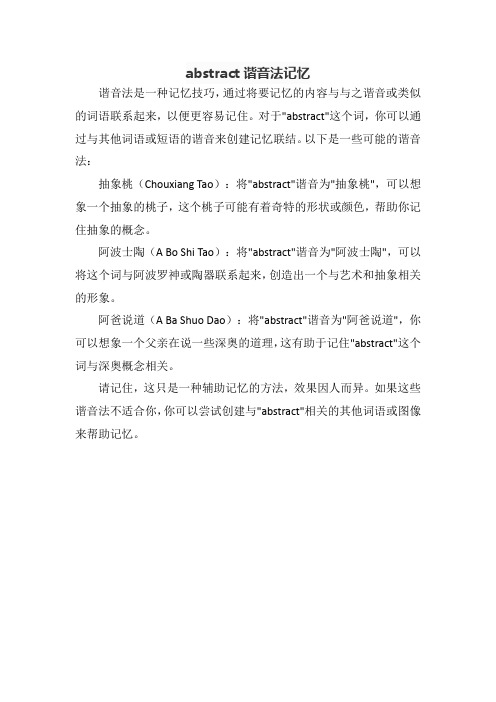
abstract谐音法记忆
谐音法是一种记忆技巧,通过将要记忆的内容与与之谐音或类似的词语联系起来,以便更容易记住。
对于"abstract"这个词,你可以通过与其他词语或短语的谐音来创建记忆联结。
以下是一些可能的谐音法:
抽象桃(Chouxiang Tao):将"abstract"谐音为"抽象桃",可以想象一个抽象的桃子,这个桃子可能有着奇特的形状或颜色,帮助你记住抽象的概念。
阿波士陶(A Bo Shi Tao):将"abstract"谐音为"阿波士陶",可以将这个词与阿波罗神或陶器联系起来,创造出一个与艺术和抽象相关的形象。
阿爸说道(A Ba Shuo Dao):将"abstract"谐音为"阿爸说道",你可以想象一个父亲在说一些深奥的道理,这有助于记住"abstract"这个词与深奥概念相关。
请记住,这只是一种辅助记忆的方法,效果因人而异。
如果这些谐音法不适合你,你可以尝试创建与"abstract"相关的其他词语或图像来帮助记忆。
abstract 的写法
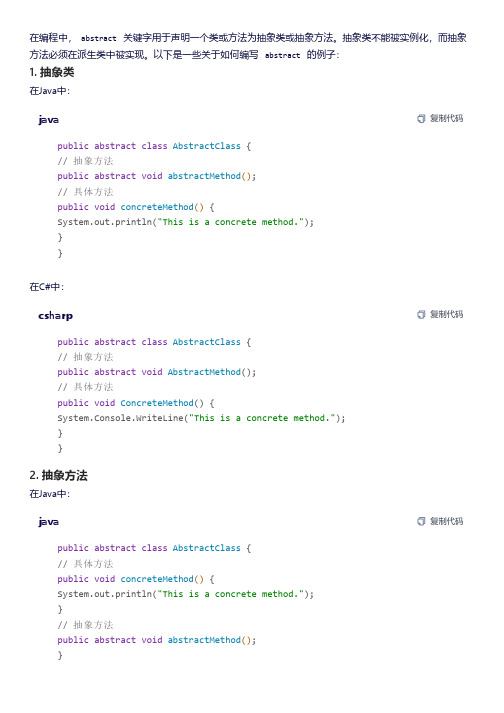
在编程中,abstract关键字用于声明一个类或方法为抽象类或抽象方法。
抽象类不能被实例化,而抽象方法必须在派生类中被实现。
以下是一些关于如何编写abstract的例子:1. 抽象类在Java中:java复制代码public abstract class AbstractClass {// 抽象方法public abstract void abstractMethod();// 具体方法public void concreteMethod() {System.out.println("This is a concrete method.");}}在C#中:csharp复制代码public abstract class AbstractClass {// 抽象方法public abstract void AbstractMethod();// 具体方法public void ConcreteMethod() {System.Console.WriteLine("This is a concrete method.");}}2. 抽象方法在Java中:java复制代码public abstract class AbstractClass {// 具体方法public void concreteMethod() {System.out.println("This is a concrete method.");}// 抽象方法public abstract void abstractMethod();}在C#中:csharp复制代码public abstract class AbstractClass {// 具体方法public void ConcreteMethod() {System.Console.WriteLine("This is a concrete method.");}// 抽象方法public abstract void AbstractMethod();}注意点:抽象类不能被实例化。
abstract 造句
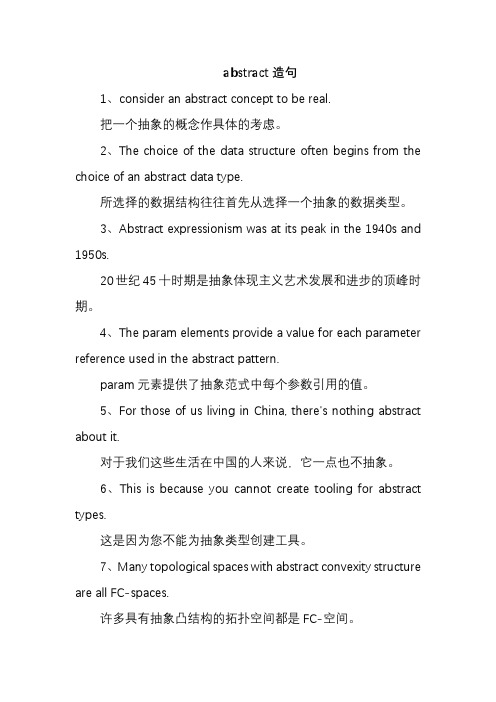
abstract造句1、consider an abstract concept to be real.把一个抽象的概念作具体的考虑。
2、The choice of the data structure often begins from the choice of an abstract data type.所选择的数据结构往往首先从选择一个抽象的数据类型。
3、Abstract expressionism was at its peak in the 1940s and 1950s.20世纪45十时期是抽象体现主义艺术发展和进步的顶峰时期。
4、The param elements provide a value for each parameter reference used in the abstract pattern.param元素提供了抽象范式中每个参数引用的值。
5、For those of us living in China, there's nothing abstract about it.对于我们这些生活在中国的人来说,它一点也不抽象。
6、This is because you cannot create tooling for abstract types.这是因为您不能为抽象类型创建工具。
7、Many topological spaces with abstract convexity structure are all FC-spaces.许多具有抽象凸结构的拓扑空间都是FC-空间。
8、Platonism began the West’s pursuit of abstract truth from the times of ancient Greece.从古希腊时代起,柏拉图主义便开始了西方式的对抽象真理的追求。
9、It used abstract art and often childish language to ridicule the absurdity of the modern world.它使用抽象的艺术和幼稚的语言嘲弄了现代世界的荒谬。
Abstract常用表达和句式
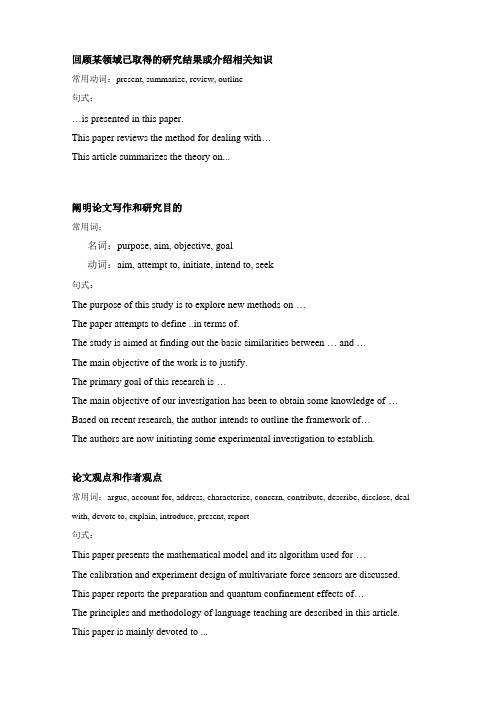
回顾某领域已取得的研究结果或介绍相关知识常用动词:present, summarize, review, outline句式:…is presented in this paper.This paper reviews the method for dealing with…This article summarizes the theory on...阐明论文写作和研究目的常用词:名词:purpose, aim, objective, goal动词:aim, attempt to, initiate, intend to, seek句式:The purpose of this study is to explore new methods on …The paper attempts to define ..in terms of.The study is aimed at finding out the basic similarities between … and …The main objective of the work is to justify.The primary goal of this research is …The main objective of our investigation has been to obtain some knowledge of …Based on recent research, the author intends to outline the framework of…The authors are now initiating some experimental investigation to establish.论文观点和作者观点常用词:argue, account for, address, characterize, concern, contribute, describe, disclose, deal with, devote to, explain, introduce, present, report句式:This paper presents the mathematical model and its algorithm used for …The calibration and experiment design of multivariate force sensors are discussed. This paper reports the preparation and quantum confinement effects of…The principles and methodology of language teaching are described in this article. This paper is mainly devoted to ...介绍研究过程和研究范围常用词:过程:analyze, consider, discuss, examine, study, investigate, state, propose 范围:contain, cover, include, outline, scope, field, domain句式:The characteristic of …was investigated.The paper analyzes the possibility of ...We study the one-step-synthesis method for …in this paper.This article discusses the method of calculation of …The principle of constructing … is proposedThis paper states the reasons for...This study identifies some procedures for …This article outlines the preliminary process of …The scope of the study covers.The study includes.The paper contains the specific topic on …介绍计算、测量常用词:calculate, compute, determine, estimate, measure, work out句式:This paper determines the proper temperature for …The cooling rate was calculated by means of.The rational rage of power is measured by …In the paper, we measured the orientation and estimated parameter for ...The author worked out the probability of ...The author has computed equilibrium constant K and …阐明论证常用词:confirm, demonstrate, find, identify, indicate, monitor, note, observe, point out, prove, provide句式:The initial particles are found to be …It is found that the amorphous silicon nitride show a tendency in...It is noted that …can be found in …The result provides a sound basis for …The study of those properties indicate…The experimental results demonstrate that…The effects of …were observed and monitored.说明试验过程常用词:experiment, test, sample句式:The samples of pyroelectric ceramics (电释热陶瓷)亚©[© collected by …We sampled the blood and urine of …The blood screening test for the AIDS antibody has been carried out on…We experimented on the sintering property(流延特性)of …The new protocol architecture for distributed multimedia systems has been tested in …介绍应用、用途常用词:application, use及其动词形式句式:In this paper, the czochralski crystal growth method has been applied in ……technique is used to …The application of the new design is to develop and maintain …展示研究结果常用词:result, cause, increase, lessen, as a result, result in, arrive at句式:As a result we have got pure particle of ...The finding of our research on methodologies in …is….The results of calculation show that the minimum velocity arrives at...The relationship between …and ...is characterized by …The room temperature resistivity is lessened to …介绍结论常用词:conclude, summary, to sum up, lead to, in conclusion, conclusion句式:It is concluded that the absorption spectra of two kinds of particles include... We concluded that …It is concluded that...The conclusion of our research is …On the basis of …,the following conclusion can be drawn …Finally, a summary is given of …To sum up, we have revealed …Our argument proceeds in …The research has led to the discovery of …进行评述句式:There are hardly any data about …Middle management is considered as the go-between of …The shapes and locations of these inclusions are believed to be related to …The finding is acknowledged as essential to ...Existing methods are not sufficient for ...It is difficult to improve the therapy under the conditions of ...The disproportion of age groups will unfortunately lead to …The improper use of methods would seriously influence the performance of …The subject will deepen the understanding of …However, it does not mean that there is no limitation of ...It is well-known that in the field of .., there are still difficulties and challenges. Environmental protection has become the most important concern of …推荐和建议常用词:propose, suggest, recommend句式:The calculation suggests that…Bulk silk is proposed to be the alternative of ordinary silk because ...The finite element method is recommended to …提出进一步研究的可能性常用词:demand, desirable, expect, necessary, necessity, need, require, requirement 句式:Another term of the …need addressing because…However, the development of MRI is absolutely necessary for …To establish a .. .model continues to be a major concern for ...The underway measurement of sea surface temperature has made it necessary to... ..requires more work on …More concern about the blood cleaning point out the need for …There is a growing demand for …There is a surge in the use of …Although there is already an efficient procedure, more study is still needed.突出论文重点句式:The development of…is the primary concern of this paper.Particular attention is paid on the cultivation of …Interface structure is emphasized in the article because …This paper concentrates on the effects of …The chief consideration is …。
- 1、下载文档前请自行甄别文档内容的完整性,平台不提供额外的编辑、内容补充、找答案等附加服务。
- 2、"仅部分预览"的文档,不可在线预览部分如存在完整性等问题,可反馈申请退款(可完整预览的文档不适用该条件!)。
- 3、如文档侵犯您的权益,请联系客服反馈,我们会尽快为您处理(人工客服工作时间:9:00-18:30)。
Intelligent Agent-based Approach to Sales Operations at E-storesMansour AbdoliDoctorate CandidateIndustrial and Management Systems Engineering University of Nebraska-Lincolnmansour@Bashar Al-SalimDoctorate StudentIndustrial and Management Systems Engineering University of Nebraska-LincolnBashar@ABSTRACTIn our paper, we consider the application of Intelligent Agents in supporting the operations in an Internet-based store (e-store). We consider and discuss different opportunities for employing Intelligent Agents to improve the performance of an e-store's operations, including sales, forecasting demand and supporting order fulfillment. We provide a framework for the application of such agents, show available sources of information, and discuss challenging issues in modeling learning and decision processes for agents.KeywordsIntelligent Agent Application, Internet-based Stores, E-stores, Sales Operations, Sales Support Operations. INTRODUCTIONInformation is considered to be the key element in achieving a successful business in today's competitive marketplace. It has been understood that integration of information systems is the first step for the full utilization of information value. The need for integrating information systems within an organization has led to the birth and growth of Enterprise Resource Planning (ERP) applications. On the other hand, the ever-growing interest of businesses in supply chain management necessitates the external integration of information.The high speed of today's transactions and the vast amount of information available due to the integration impose a challenge to decision makers in the operations level. A viable approach to processing and decision-making in today's demanding environment is the use of Intelligent Agents (IA's). Intelligent agents are software programs that perceive the environment through their sensors, make autonomous decisions, and affect their environment through their actuators. An agent rationale invokes actions that best satisfy a set of predefined goals (for a formal definition of an agent, see for example Luck and d'Inverno, 2001). Application and implementation of IA’s have been the focus of many studies. Applications of IA's have been considered in various areas including e-commerce (Guttma, Moukas and Maes, 1998; Rahwan, Kowalczyk and Pham, 2001; Ye, Liu and Moukas, 2001) and supply chain management (Mehra and Nissen, 1998; Shen, Ulieru, Norrie and Kremer, 1999; Shen and Norrie, 1998; Swaminathan, Smith and Sadeh, 1998). The two main concerns of these application studies are to identify the agent's goal set and its decision models. Implementation studies, on the other hand, focus on the structure of agents, communication between agents, and security issues (Da Silva, Choren and de Lucena, 2004; Guan and Zhu, 2002; Marques, Silva and Silva, 1999).Abdoli and Choobineh (Abdoli and Choobineh, 2003) provide a conceptual framework for implementing a sales agent at Internet-based stores (e-stores). They also discuss the challenging issues in modeling the agent including customer identification and sales tactic selection. In this paper, we extend their work in two directions. First, we provide a more detailed plan for implementing the sales-agent. We consider a two-level structure consisting of a master sales agent(MSA) and many personal sales agents(PSA). PSAs are reactive agents that interact with visitors, preprocess visitors’ s urfing activities, and make final decisions regarding the use of sales tactics. The MSA is a proactive agent that decides on the use of system-wide tactics based on available information including those provided by PSAs. We also extend the work of Abdoli and Choobineh by introducing two other supporting agents for sales operations, namely,demand-forecasting agent (DFA) and o rder-fulfillment agent (OFA).The rest of the paper is organized as follows. In the next section, important supporting operations for sales in e-stores are discussed. A general framework for application of IA's for those supporting operations is provided. Moreover, challenging modeling issues are discussed and some modeling approaches are suggested. The paper concludes with a summary of research and future directions.E-STORE OPERATIONSSales and sales-supporting operations of e-stores are often more challenging than those operations of their brick-and-mortar counterparts. In the following, we discuss the challenges that e-stores face with respect to sales and sales support operations such as demand forecast and order fulfillment.By making various storefronts easily accessible, the Internet has made the shopping experience more efficient for many customers. The ease of access to e-stores via the Internet has increased the number of online customers, thus driving the growth of Internet storefronts (e-stores). The growth of e-stores, consequently, lowers the search cost (the cost of comparing services/products) for Internet shoppers. Finally, the ease of use and lower search costs have reduced the likelihood of customers being loyal to a particular store. The combination of the above factors has made it challenging for e-store owners to attract visitors and convert them into buyers and loyal customers. This disadvantage of e-stores is more pronounced when considering the lack of human contact. The success of stores depends partly on the performance of their salespersons who take full advantage of their face-to-face contact with customers. In e-stores, however, the face-to-face contact is not applicable. E-stores can use visual/audio technology to imitate the face-to-face contact in brick-and-mortar stores; nonetheless, the use of such technology is not popular. This is most likely due to the large number of customers, low buyer-to-visitor ratio, and limited resources (e.g. bandwidth required to support visual/audio communications).The lack of physical boundary –the main difference between e-stores and brick-and-mortar stores– has also affected the operations routine in e-stores. In brick-and-mortar stores, most customer orders are fulfilled at the store. In e-stores, however, customers are invited from geologically dispersed areas and orders most often need to be shipped to them. This leads to certain advantages and disadvantages for e-stores. The advantages include having the ability to choose a providing source from a larger set of providers, and providing the opportunity to avoid the costs of holding inventories; practices which are supported and encouraged in e-commerce literature. Nonetheless, having to make order fulfillment decisions based on single customer orders (rather than forecasts of store-wide sales) increases the burden of decision makers. In order to maintain a low (or zero) inventory, e-stores need to have a more precise understanding of customers’ demands and need to be able to react to sudden shifts in customers’ i nterests. These consequently increase the risk of failure in on-time delivery and customer dissatisfaction.The application of IA’s provides a plausible approach to challenges that e-stores face. IA’s can use information available to e-stores to: 1) assist customers with recommendations and persuade them to make a purchase using promotions, 2) improve order fulfillment by initiating proper shipping and handling transactions, and 3) support planning operations through frequent and accurate forecasting demands. In the following section we provide a conceptual framework for implementing three groups of IA's that can satisfy the above applications.APPLICATION OF IA’S AT E-STORES: A FRAMEWORKFigure 1 shows our general framework for the application of three groups of IA’s that can improve the performance of the e-stores’ o perations. Our framework presents the interconnections and flow of information between agents (circles) and informative nodes (ovals) in e-stores. In our framework, bold arrows distinguish the outflow of information from agents. Different types of agents communicate with each other directly or indirectly (through informative nodes). This framework initiates a decision support system that interacts with customers in the storefront and provides informational support to supply-end operations. In the rest of this section, a brief introduction to informative nodes in the framework is provided, followed by a separate presentation of each agent’s operation.Informative NodesInformative nodes represent the IA's perception of the environment. These nodes contain pre-existing information in the system and/or knowledge learned and created by other agents. Pre-existing information is extracted from available information to e-stores that include database systems and websites’ s erver log-files. Table 1 provides the list informative nodes used in our framework. A brief introduction to each node is followed.Figure 1. The general framework for application of IA's in e-stores.Informative Nodes Pre-ExistingInformationLearnedInformationClickstream√√Customer Behavior√Active Orders√Sales History√Product Information√√Tactics & Strategies√Adjusted Tactics√Table 1. List of informative nodes and their generating sources.ClickstreamCustomers' visiting actions are recorded in server log-files and can be used for estimating customer visiting characteristics,including visiting intention and purchasing interest. The sequence of actions taken by a customer is commonly called clickstream and usually is extracted from server log-files. In our framework, we consider the option that personal sales agents (PSAs) update clickstream node directly.Customer BehaviorThis node represents the agent’s perception of the customer's visiting intention and shopping interest. The intention and visiting objective of customers are not observable. Nonetheless, agents can use available information (e.g. clickstream,demographic data, and purchase history) to learn about customers' interests and intentions. Table 2 shows some details of available information (Demographic data can be supplied by Customer Relationship Management database which is not shown in our framework). The master sales agent (MSA) can use this information to update the customer behavior rmation TypeFields Page Viewing Time Number of Pages Visited Type of Pages VisitedServer Log-Files(Click-stream)The Sequence of Actions TakenAge GenderIncomeCustomer Relationship Management(Demographic data)LocationLast Purchase Date Last Purchased AmountSales(Purchase History)Sales Tactic UsedTable 2. Examples of customer-related information available to e-stores .Active OrdersThis node provides the list of outstanding orders made by customers. This information is usually held by the sales database.In our framework, PSAs can update this node; however, no learning process is required.Sales History“Sales History” s imply includes the history of sales made by each customer. This information is usually held by the sales database. In our framework, order-fulfillment agent (OFA) can update this node; however, no learning process is required.Product InformationThis node includes the inventory status of products as well as their specifications and relevance (functional relationship).Inventory status is easily accessible from the inventory database, but not all product relationships are readily available. The MSA can use clickstream to identify (learn) unobservable (non-transparent) relationships between products. Such approaches have been used for recommending products based on previous customer's preferences. For example, provides visitors with a list of recommended books based on the purchase history of customers with similar interests. Table 3 shows a list of product related information.Tactics and StrategiesThis node provides both goals and action sets required for applications of MSA and OFA. These sets are provided by the e-store managers. The main goal of the e-stores, usually financial growth, can be interpreted as multiple detailed objectives such as increasing the monetary value of sales and reducing the cost of shipping.The action set includes the possible tactics that can be used to satisfy one or more of the detailed objectives. These tactics may affect the product price, the product specification, and/or customer service.Price discrimination tactics (with long-term life-cycle), and promotional discounts (with short-term life-cycle) affect the product’s price. Up-sizing tactics increase the volume of sales, for example, by adding other products. Also, supportive actions directly target customer satisfaction. Table 4provides some examples for each tactic types.Adjusted TacticsTactics and strategies provide the ground for sales decision support; however, they may represent conflicting directions. For example, reducing shipping costs can be in conflict with increasing customer satisfaction. Also, using some tactics (e.g.reducing the price) can increase the sales of a store and its market share, but it also can reduce the profit margin per sales.Accordingly, using sales tactics is a two-edged sword, and is considered an important issue in marketing and has been extensively studied (for example, see Zeithaml, 1987).The MSA can proactively refine and adjust tactics and objective functions based on the market trends and customer behavior.“Adjusted Tactics” node represents the sales tactics that have been adjusted for use based on the collective interest and intention of all customers at the current time.SourceInformation Type Field On-hand Inventory In-Order Inventory Quantity Rate of consumption (Demand)List Price Replenishment Cost MonetaryInventory Cost I n v e n t o r y D a t a b a s e Transparent AssociationPossible Substitutions Accessories L o g -F i l e s Non-Transparent Association Products frequently bought togetherTable 3. Examples product-related information available to e-stores.Target Sales Tactics ExamplesLoyalty ProgramGroup DiscountVolume DiscountPrice Discriminations Nonlinear PricingSeasonal Sales/ RebatesExcess Inventory SalesP r i c e Promotional Discount Individual DiscountCross SellingUp SellingP r o d u c t Sales Up Sizing BundlingBandwidthTechnical SupportFast/Free DeliveryAdvertisementC u s t o m e r Supportive OffersShelf Presentation Table 4. Examples of sales tactics grouped based on their target.Sales IA'sAbdoli and Choobineh (Abdoli and Choobineh, 2003) proposed the use of a sales agent that utilizes its perception from customer behavior and its knowledge of product specifications to adjust a set of given sales tactics. The agent uses the adjusted sales tactics to provide a customer with promotions and offers for improving the sales performance of the e-store (e.g. increasing the probability of purchase). Here we present a multi-agent approach with the same objective. Our approach considers the following two agents: 1) a Master sales agent (MSA) that provides periodic system-wide adjustments to sales tactics (e.g. setting price modifications or seasonal sales) based on the recent behavior of all customers; and 2) a group of Personal sales agents (PSAs) that select sales tactics by comparing online actions of customers to suggestions provided by MSA. We consider MSA to be a proactive agent in the sense that it is goal-oriented. The MSA uses available information to learn customer behavior and the relation between customer actions and effects of sales tactics. To satisfy the e-store's goal, MSA creates and updates the set of adjusted tactics. PSAs are considered reactive agents. They use the adjusted sales tactics and the knowledge of customer behavior produced by the MSA to assign a tactic to a customer based on his/her online actions.In an e-store, the number of concurrent visiting customers can be very large, while computing and communication resources are limited. Moreover, not all customers have long history of visit, or spend enough time in a single visit to provide adequate learning opportunity to terminal agents (here PSAs). Our two-level structure incorporates the learning element of an Intelligent Agent approach with no side-effects on the effectiveness of terminal agents: First, it reduces the processing burden of PSAs to improve their response time. Second, it allows sharing observations for similar visitors to strengthen the learning process. Finally, by considering the option that PSAs can update clickstream directly, we can eliminate processing step that extracts clickstream data from the log-files. PSAs can filter non-informative, short sessions to increase system's performance by saving recording and retrieving times.Figure 2 shows the interaction of our sales agents with a hypothetical e-store's database systems. Information used by agents is supported by sales, customer relationship management (CRM), inventory, and product information databases, and server log-files. Sales, CRM, and inventory databases are part of traditional information systems for both e-stores and brick-and-mortar stores. However, server log-files are an explicit part of the e-store information system. Product characteristics may be available to both types of stores in a database format; nonetheless, some of the subtle relationships between products can only be documented in e-stores using information provided by server log-files. Such product relationships, called non-transparent relations, can be extracted from visitors’ viewing actions. Recommender agents exploit these relationships to assist/persuade online visitors. Tactics and Strategies and Website Database are other external information required for implementing our sales agents. Other required information, such as customer behavior and adjusted sales tactics, can be generated and shared internally between different sales agents.Figure 2. Sales Agents and their interaction with a hypothetical e-store.Order-Fulfillment Agent (OFA)Today’s e-commerce offers e-stores the use of a large number of providers and supports the high speed of transactions. This, in return, requires e-stores to face more frequent purchase/replenishment operations. Therefore, e-stores can only fully utilize their advantages (operating globally and holding zero-inventory) if they support their sales operation with a reliable and fast order-fulfillment operation. Such an operation relies on access to a set of vendors as well as a selection process that accounts for vendor reliability and shipping alternatives. Let us consider the simple case that vendors are negotiated and selected in advance. A reactive OFA can handle the shipping process of all active orders (updated by PSAs). As soon as an order is activated, the OFA will select the appropriate vendor and shipping method based on the information provided by order itself, product specifications, and available shipping methods (given as tactics). Information of fulfilled orders finally is added to the sales history that is used for future analysis.The above simple approach can be extended in two directions. First, OFA can cooperate closely with the supply chain management system of the e-store. In some cases, e-stores can benefit from the vendors' competition by postponing their negotiations until an order is made. The OFA can initiate the negotiation process and support the process by providing the shipping costs and constraints to the negotiating agent(s).Another improvement can be made by assigning one OFA to each outstanding order. Then allowing OFAs to distribute orders (by creating new OFAs) and/or mix orders (by merging some OFAs together) in different stages of shipping. In the latter case, OFAs are more valuable when they coordinate shipping processes of more than one store.From implementation point of view, a two-level approach (similar to our sales agents) can combine both suggested extensions. The master agent will manage the distributing/mixing operations for the other OFA agents. It also will cooperate with negotiating agents (or perform their tasks if no such agent is implemented). Figure 3 shows the relationship of the OFA(s) with e-store database systems and other agents in the system.Figure 3. Interaction of the OFA with e-store database systems and PSAs.Demand Forecasting Agent (DFA)Forecasting demand is an essential part of planning operations for all stores. Demands are usually forecasted periodically based on patterns of sales made in the past. These forecasts are then used for long-term planning such as vendor acquisitions and forward contracting. In e-stores, long-term planning may not be a necessity; nonetheless, frequent and accurate demand forecasts can provide useful insights for both acquisition and sales operations. Negotiator agents (in e-commerce) can use forecasts of demand to strengthen their bonds with to-be-needed vendors or reduce the acquisition time by initiating negotiations for future purchases. Furthermore, the MSA can use demand forecasts for adjusting sales tactics (e.g. offering seasonal sales when demand in diminishing).In general, demands are forecasted based on the sales history. To improve the accuracy of forecasts, we allow DFA to benefit from the knowledge created by MSA (i.e. MSA's perception of customer intention) for a better interpretation of sales history, and consequently, a better demand forecast. In this approach, DFA accounts for customers' intention through weighting salespatterns by the sales tactics used prior to each purchase. Figure 4 shows the interconnection of the DFA with existing databases and agents in the system.Figure 4. Interaction of DFA with e-store database systems and other agents.SALES AGENTS AT E-STORES: MODELING ISSUESWe considered the areas that IAs can provide support to e-stores’ operations. In our framework, we introduced three different IAs and discussed the way that they perceive environment and the actions that they can take. Regarding implementation,available sources of information and those to be learned were discussed. From other implementation issues, including modeling agents’ decision engines and software development, we only consider the modeling issue. Some of required models for our application of IAs are: 1) a customer identification model for the MSA that creates knowledge of customer online behavior from available information such as clickstream and sales history; 2) a tactic-effect model that allows MSA to learn the effects of tactics used on customer purchasing behavior; 3) a tactics adjustment/selection models that give the MSA the ability to set store-wide sales tactics and guidelines for PSAs on personal tactic selection; 4) a demand model that provides bases for forecasting demands, and discovers the non-transparent associations between products, and 5) optimization models for quantifying action (tactic) selection problem needed by (reactive) agents such as PSAs. We focus on customer identification and tactic selection models, and provide some guidelines for developing such models.Customer IdentificationCustomers’ o nline behavior have been of researchers interest for different reasons, including to understand surfing goals,common paths taken, and factors affecting outcomes of customers’ visits. In studies that the visitors’ goal and the associated paths are of interest, the sequence of visiting actions is exclusively modeled. Markov models are used as a common tool for studying clickstream. For example, Markov models are used for representing webpages visited to forecast the next action of online visitors (Pirolli and Pitkow, 1999, and Sen and Hansen, 2003). They also are used for modeling the sequence of documents requested over the time to predict and pre-fetch to-be-requested documents.Other related works in this area are the studies that have marketing incentives and are interested in modeling purchasing behavior of customers. These studies commonly estimate the probability of purchase and/or classify customers based on their purchasing habits. The common sources of information for such studies are surveys (Pedersen and Nysveen, 2005) or summary of viewing activities in a session (Moe, 2003).In our research, we are interested in learning customers' searching behavior from sequence of online actions, as well as purchasing behavior from purchasing outcomes of visiting sessions. Markov models that are usually used for modeling search behavior can be adapted to present purchasing outcomes as follows. A Markov model provides a set of probability of visiting webpages w based on recent webpages visited h , denoted by Pr (w |h ). The probability of purchase can be supported by considering a subset of webpages PURCHASE consists of all confirmation pages in the e-store’s website, and then calculating expected probability of visiting such a subset in a given session, i.e.[])|Pr(h PURCHASE w E ∈.In a similar approach, a dynamic multinomial probit model is used for predicting the purchase conversion rate (Montgomery,Li, Srinivasan and Liechty, 2004). In this study, webpages are aggregated based on their functionality into eight categoriesincluding order; then, the probability of visiting order category is used as the probability of purchase. Nonetheless, this model does not provide a detailed representation of the customers’ s earch behavior. Our approach represents the details of customers’ s earch behavior and offers the ability to predict the product of interest. The measure of customer interests on products can complement the probability of purchase in providing a better assessment of the customers’ value. Consequently, we can improve the performance of MSA and PSAs using a better estimate of customers' value.Tactic SelectionIn brick-and-mortar stores, salespersons have a little (if no) authority in using many sales tactics. In fact, most sales tactics are usually employed and updated by the sales manager after processing recent sales data. Moreover, implementing most tactics is costly (e.g. changing price tags; advertising seasonal sales). Therefore, tactic updates are infrequent. In e-stores, on the other hand, employing sales tactics are not as costly and tactic adjustments can be frequent. In our framework, PSAs can theoretically process available information and select sales tactics frequently on their own (a decentralized decision system). Nonetheless, we suggest using a centralized decision system (similar to brick-and-mortar stores) to increase the response time of PSAs and improve the MSA's learning process by sharing (aggregating) information of similar customers. In our suggested approach, PSAs can use different tactics frequently, but the MSA does not adjust those tactics frequently.Implementing a tactic selection process has following two challenges: 1) the effect of tactics on customer behavior is unknown a priori, and 2) the diversity of tactics types makes comparing them challenging. These challenges are addressed below.Estimating Tactics’ Effects:Statistical methods can be used in conjunction with the customer identification model (discussed earlier) to estimate the effect of each tactic on customers. For example, the average increase in the probability of purchase before and after employing a tactic can be used as a measure of tactic effects. Markov decision models (Choi and Liu, 2002) are another alternative for learning tactics effects. The application of IAs is a natural approach to learning such relations over the time. Tactics Unification:To make tactics comparable, we consider the tactic selection problem as a resource allocation problem. In this approach, a nominal price and demand is set which defines the nominal revenue/benefit of the e-store. Then an amount is allocated as the Maximum Allowed Discount(MAD) that sets a lower bound to the store’s revenue/profit. In this setting, tactics can be represented uniformly by their associated cost. This turns the tactic selection into a resource allocation problem in which MAD is allocated to sales tactics such that the e-store performance is maximized.CONCLUSIONWe considered the application of intelligent agents (IA's) in sales and sales support operations for e-stores. For sales, we proposed a two-level agent structure. The MSA proactively learns the customers’ behavior, the effect of tactics on customers, and adjusts store-wide tactics based on the current market trend. PSAs reactively apply available adjusted tactics to customers based on the knowledge of customer behavior created by the MSA. We also considered using agents for forecasting demands and fulfilling orders. These agents can also provide support for the existing supply chain management operations.We also provided some insight on how existing database systems of e-stores can be used for implementing operations support agents. Utilizing existing databases has some advantages and disadvantages. Ease of development and independence of implementation phases are two advantages of this approach. Agents' software programs do not need to build and maintain their data internally. Moreover, most agents can be implemented and operate regardless of the status of other agents. Nonetheless, using legacy databases imposes some restrictions which might reduce the flexibility of agents in both implementation and operation phases. This is less likely if a well-designed database system is in place.We proposed some modeling approaches for dealing with challenges of learning customers' behavior and selecting tactics. For tactics selection, we suggested adapting a resource allocation approach in which different types of tactics are transformed to comparable monetary resources. For modeling customer behavior, we proposed combining two groups of studies in this context. These studies are marketing related studies, concerned with the customers' shopping intentions, and search behavior related studies, focused on customers' surfing interests. Our motivation for combining these two approaches is to assign a monetary value to the probability of purchase and use the resulting measure as the customer value. The customer value can be used by MSA for adjusting sales tactics.。