DOMpro protein domain prediction using profiles, secondary structure, relative solvent acce
蛋白质-适配体相互作用预测的方法
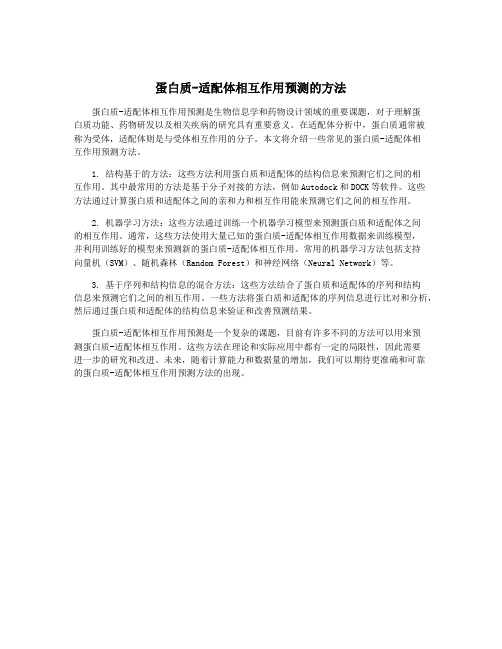
蛋白质-适配体相互作用预测的方法蛋白质-适配体相互作用预测是生物信息学和药物设计领域的重要课题,对于理解蛋白质功能、药物研发以及相关疾病的研究具有重要意义。
在适配体分析中,蛋白质通常被称为受体,适配体则是与受体相互作用的分子。
本文将介绍一些常见的蛋白质-适配体相互作用预测方法。
1. 结构基于的方法:这些方法利用蛋白质和适配体的结构信息来预测它们之间的相互作用。
其中最常用的方法是基于分子对接的方法,例如Autodock和DOCK等软件。
这些方法通过计算蛋白质和适配体之间的亲和力和相互作用能来预测它们之间的相互作用。
2. 机器学习方法:这些方法通过训练一个机器学习模型来预测蛋白质和适配体之间的相互作用。
通常,这些方法使用大量已知的蛋白质-适配体相互作用数据来训练模型,并利用训练好的模型来预测新的蛋白质-适配体相互作用。
常用的机器学习方法包括支持向量机(SVM)、随机森林(Random Forest)和神经网络(Neural Network)等。
3. 基于序列和结构信息的混合方法:这些方法结合了蛋白质和适配体的序列和结构信息来预测它们之间的相互作用。
一些方法将蛋白质和适配体的序列信息进行比对和分析,然后通过蛋白质和适配体的结构信息来验证和改善预测结果。
蛋白质-适配体相互作用预测是一个复杂的课题,目前有许多不同的方法可以用来预测蛋白质-适配体相互作用。
这些方法在理论和实际应用中都有一定的局限性,因此需要进一步的研究和改进。
未来,随着计算能力和数据量的增加,我们可以期待更准确和可靠的蛋白质-适配体相互作用预测方法的出现。
同源域蛋白
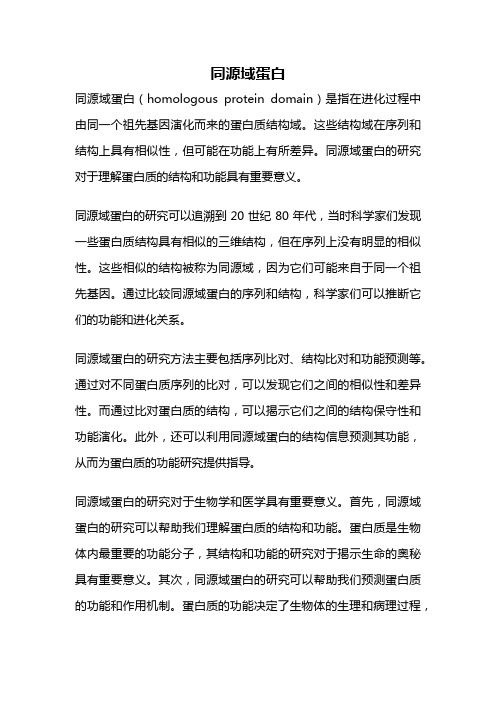
同源域蛋白同源域蛋白(homologous protein domain)是指在进化过程中由同一个祖先基因演化而来的蛋白质结构域。
这些结构域在序列和结构上具有相似性,但可能在功能上有所差异。
同源域蛋白的研究对于理解蛋白质的结构和功能具有重要意义。
同源域蛋白的研究可以追溯到20世纪80年代,当时科学家们发现一些蛋白质结构具有相似的三维结构,但在序列上没有明显的相似性。
这些相似的结构被称为同源域,因为它们可能来自于同一个祖先基因。
通过比较同源域蛋白的序列和结构,科学家们可以推断它们的功能和进化关系。
同源域蛋白的研究方法主要包括序列比对、结构比对和功能预测等。
通过对不同蛋白质序列的比对,可以发现它们之间的相似性和差异性。
而通过比对蛋白质的结构,可以揭示它们之间的结构保守性和功能演化。
此外,还可以利用同源域蛋白的结构信息预测其功能,从而为蛋白质的功能研究提供指导。
同源域蛋白的研究对于生物学和医学具有重要意义。
首先,同源域蛋白的研究可以帮助我们理解蛋白质的结构和功能。
蛋白质是生物体内最重要的功能分子,其结构和功能的研究对于揭示生命的奥秘具有重要意义。
其次,同源域蛋白的研究可以帮助我们预测蛋白质的功能和作用机制。
蛋白质的功能决定了生物体的生理和病理过程,因此了解蛋白质的功能对于疾病的防治具有重要意义。
最后,同源域蛋白的研究还可以帮助我们设计新的蛋白质,从而开发新的药物和治疗方法。
通过对同源域蛋白的研究,可以揭示蛋白质的结构和功能之间的关系,从而为蛋白质工程和药物设计提供指导。
同源域蛋白的研究在生物学和医学领域取得了重要的进展。
科学家们通过对同源域蛋白的研究,发现了许多重要的蛋白质家族和结构域,如酶、抗体、膜蛋白等。
这些发现不仅拓宽了我们对蛋白质的认识,还为新药物的研发提供了重要的靶点。
同时,同源域蛋白的研究还促进了生物信息学和计算生物学的发展。
通过利用大规模的生物信息学数据和计算方法,科学家们可以对成千上万个蛋白质进行同源域分析和功能预测,从而加快了蛋白质的研究进程。
蛋白质结构域预测

蛋白质结构域预测蛋白质结构域预测是蛋白质功能注释中的一个重要任务。
蛋白质结构域是指在蛋白质中具有特定结构和功能的连续序列段。
准确地预测蛋白质结构域可以帮助我们理解蛋白质的功能和作用机制,对药物设计和疾病治疗等领域具有重要意义。
随着高通量测序技术的迅猛发展,大量的蛋白质序列数据被积累,蛋白质结构域预测方法也得到了长足的进步。
基于比对的方法是将待预测序列与已知结构域库中的序列进行比对,根据比对结果来判断待预测序列是否含有特定的结构域。
通过这种方法可以预测到已知结构域的序列,但是对于新发现的结构域或者与已知结构域相似度较低的序列预测效果较差。
基于机器学习的方法是利用已知结构域的序列和非结构域的序列作为训练集,通过机器学习算法构建一个预测模型,然后用该模型对待预测序列进行预测。
这种方法可以预测到新发现的结构域,并且可以预测与已知结构域相似度较低的序列。
目前,基于机器学习的方法在蛋白质结构域预测中占据主导地位。
常见的机器学习算法包括SVM(支持向量机)、DT(决策树)、RF(随机森林)等。
这些算法可以通过学习已知结构域的特征和非结构域的特征,来区分结构域和非结构域的序列。
除了机器学习算法,人工神经网络(ANN)也是常用的预测模型。
人工神经网络模型可以建立一个多层的神经网络,通过自我调整权重和阈值参数来计算输入和输出之间的关系。
通过训练样本,可以优化神经网络的参数,使之能够对待预测序列进行准确的预测。
此外,一些新兴的预测方法也逐渐得到应用。
例如,通过整合不同的预测结果进行综合预测。
这种方法可以利用多个预测方法的优势,提高预测的准确性。
同时,一些基于深度学习的方法也逐渐应用于蛋白质结构域预测中。
深度学习利用多层神经网络模型进行特征学习和表征学习,可以从海量的数据中发现隐藏的规律和模式,进一步提高预测效果。
总的来说,蛋白质结构域的准确预测对于研究生命科学和药物设计具有重要意义。
基于比对和机器学习的方法已经取得了显著的进展,通过不断地创新和技术的进步,预测方法将会更加精确和有效。
蛋白质-适配体相互作用预测的方法
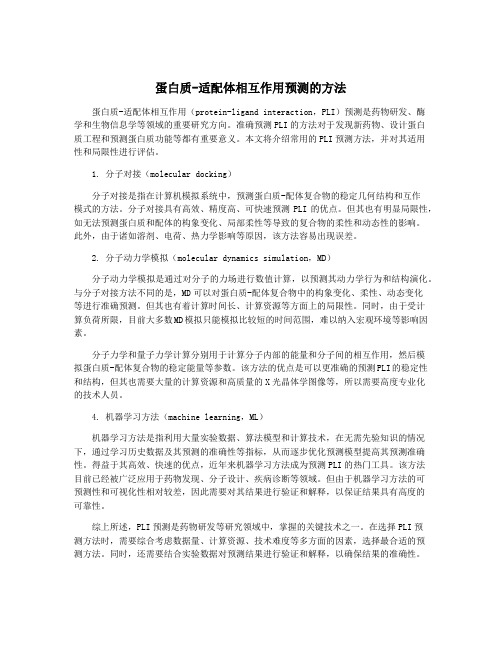
蛋白质-适配体相互作用预测的方法蛋白质-适配体相互作用(protein-ligand interaction,PLI)预测是药物研发、酶学和生物信息学等领域的重要研究方向。
准确预测PLI的方法对于发现新药物、设计蛋白质工程和预测蛋白质功能等都有重要意义。
本文将介绍常用的PLI预测方法,并对其适用性和局限性进行评估。
1. 分子对接(molecular docking)分子对接是指在计算机模拟系统中,预测蛋白质-配体复合物的稳定几何结构和互作模式的方法。
分子对接具有高效、精度高、可快速预测PLI的优点。
但其也有明显局限性,如无法预测蛋白质和配体的构象变化、局部柔性等导致的复合物的柔性和动态性的影响。
此外,由于诸如溶剂、电荷、热力学影响等原因,该方法容易出现误差。
2. 分子动力学模拟(molecular dynamics simulation,MD)分子动力学模拟是通过对分子的力场进行数值计算,以预测其动力学行为和结构演化。
与分子对接方法不同的是,MD可以对蛋白质-配体复合物中的构象变化、柔性、动态变化等进行准确预测。
但其也有着计算时间长、计算资源等方面上的局限性。
同时,由于受计算负荷所限,目前大多数MD模拟只能模拟比较短的时间范围,难以纳入宏观环境等影响因素。
分子力学和量子力学计算分别用于计算分子内部的能量和分子间的相互作用,然后模拟蛋白质-配体复合物的稳定能量等参数。
该方法的优点是可以更准确的预测PLI的稳定性和结构,但其也需要大量的计算资源和高质量的X光晶体学图像等,所以需要高度专业化的技术人员。
4. 机器学习方法(machine learning,ML)机器学习方法是指利用大量实验数据、算法模型和计算技术,在无需先验知识的情况下,通过学习历史数据及其预测的准确性等指标,从而逐步优化预测模型提高其预测准确性。
得益于其高效、快速的优点,近年来机器学习方法成为预测PLI的热门工具。
该方法目前已经被广泛应用于药物发现、分子设计、疾病诊断等领域。
保守结构域序列构建进化树
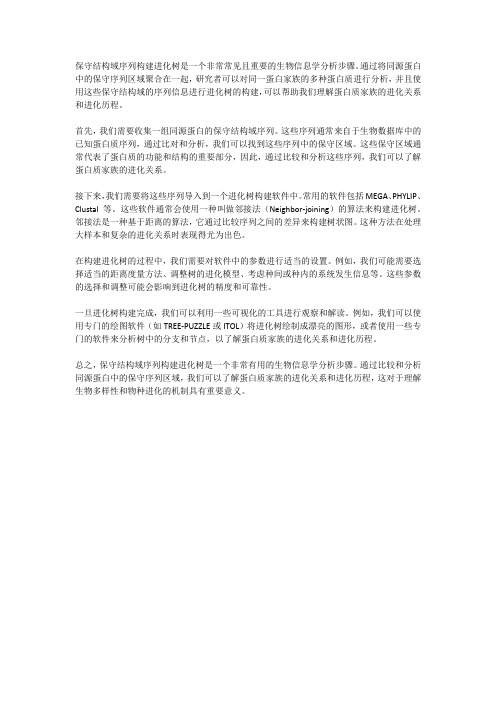
保守结构域序列构建进化树是一个非常常见且重要的生物信息学分析步骤。
通过将同源蛋白中的保守序列区域聚合在一起,研究者可以对同一蛋白家族的多种蛋白质进行分析,并且使用这些保守结构域的序列信息进行进化树的构建,可以帮助我们理解蛋白质家族的进化关系和进化历程。
首先,我们需要收集一组同源蛋白的保守结构域序列。
这些序列通常来自于生物数据库中的已知蛋白质序列,通过比对和分析,我们可以找到这些序列中的保守区域。
这些保守区域通常代表了蛋白质的功能和结构的重要部分,因此,通过比较和分析这些序列,我们可以了解蛋白质家族的进化关系。
接下来,我们需要将这些序列导入到一个进化树构建软件中。
常用的软件包括MEGA、PHYLIP、Clustal等。
这些软件通常会使用一种叫做邻接法(Neighbor-joining)的算法来构建进化树。
邻接法是一种基于距离的算法,它通过比较序列之间的差异来构建树状图。
这种方法在处理大样本和复杂的进化关系时表现得尤为出色。
在构建进化树的过程中,我们需要对软件中的参数进行适当的设置。
例如,我们可能需要选择适当的距离度量方法、调整树的进化模型、考虑种间或种内的系统发生信息等。
这些参数的选择和调整可能会影响到进化树的精度和可靠性。
一旦进化树构建完成,我们可以利用一些可视化的工具进行观察和解读。
例如,我们可以使用专门的绘图软件(如TREE-PUZZLE或ITOL)将进化树绘制成漂亮的图形,或者使用一些专门的软件来分析树中的分支和节点,以了解蛋白质家族的进化关系和进化历程。
总之,保守结构域序列构建进化树是一个非常有用的生物信息学分析步骤。
通过比较和分析同源蛋白中的保守序列区域,我们可以了解蛋白质家族的进化关系和进化历程,这对于理解生物多样性和物种进化的机制具有重要意义。
蛋白相互作用结构域预测
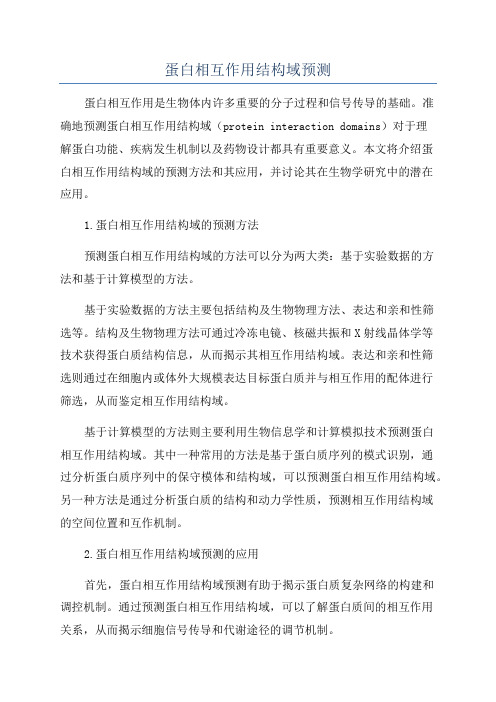
蛋白相互作用结构域预测蛋白相互作用是生物体内许多重要的分子过程和信号传导的基础。
准确地预测蛋白相互作用结构域(protein interaction domains)对于理解蛋白功能、疾病发生机制以及药物设计都具有重要意义。
本文将介绍蛋白相互作用结构域的预测方法和其应用,并讨论其在生物学研究中的潜在应用。
1.蛋白相互作用结构域的预测方法预测蛋白相互作用结构域的方法可以分为两大类:基于实验数据的方法和基于计算模型的方法。
基于实验数据的方法主要包括结构及生物物理方法、表达和亲和性筛选等。
结构及生物物理方法可通过冷冻电镜、核磁共振和X射线晶体学等技术获得蛋白质结构信息,从而揭示其相互作用结构域。
表达和亲和性筛选则通过在细胞内或体外大规模表达目标蛋白质并与相互作用的配体进行筛选,从而鉴定相互作用结构域。
基于计算模型的方法则主要利用生物信息学和计算模拟技术预测蛋白相互作用结构域。
其中一种常用的方法是基于蛋白质序列的模式识别,通过分析蛋白质序列中的保守模体和结构域,可以预测蛋白相互作用结构域。
另一种方法是通过分析蛋白质的结构和动力学性质,预测相互作用结构域的空间位置和互作机制。
2.蛋白相互作用结构域预测的应用首先,蛋白相互作用结构域预测有助于揭示蛋白质复杂网络的构建和调控机制。
通过预测蛋白相互作用结构域,可以了解蛋白质间的相互作用关系,从而揭示细胞信号传导和代谢途径的调节机制。
其次,蛋白相互作用结构域预测可用于研究疾病发生机制。
许多重要的疾病如癌症和神经退行性疾病都与蛋白相互作用的异常有关。
通过预测蛋白相互作用结构域,可以揭示蛋白质突变和异常结构对于疾病发生的影响,为疾病预防和治疗提供新的靶点和策略。
另外,蛋白相互作用结构域预测还可以用于药物设计和优化。
许多药物通过与特定的蛋白相互作用来发挥其药理活性。
通过预测蛋白相互作用结构域,可以设计具有高亲和力和选择性的药物靶点,并优化药物分子的结构,提高疗效和减少副作用。
蛋白抗原表位预测及抗原多肽设计之欧阳育创编

蛋白抗原表位预测及抗原多肽设计利用在线软件BepiPred 1.0 Server(http://www.cbs.dtu.dk/services/BepiPred/)从蛋白序列直接预测抗原表位还有其他在线预测网站/Links.htm/Tools/index.html进A ntigenic Peptide Prediction 用tools/Tools/antigenic.pl把氨基酸序列粘贴进去,就可以直接得出预测结果抗原多肽选择的基本原则1、尽可能是在蛋白表面2、保证该段序列不形成α-helix3、N,C端的肽段比中间的肽段更好4、避免蛋白内部重复或接近重复段的序列5、避免同源性太强的肽段6、交联可以交联在N,C两端,选择依据就是交联在对产生抗体不太重要的一端7、序列中不能有太多的Pro,但有一两个Pro有好处,可以使肽链结构相对稳定一些,对产生特异性抗体有益。
抗原多肽设计的基本原则为了使生产抗体获得最佳效果,仔细地设计抗原多肽是很有必要的,设计应满足一个基本条件:在免疫过程中,该抗原既不会产生过强的免疫反应,同时又能产生出对感兴趣的蛋白有结合能力的抗体。
尽管抗原设计是一个很复杂的课题,有诸多需要注意的细节,已超过了我们所能提供的范围,根据我们所积累的经验,有几点关键的基本设计原则可以提供给大家参考:1、确定抗体的用途(应用)新开展一个研究项目,弄清楚所感兴趣的蛋白的一些基本特性是很有必要的,特别是如果知道蛋白的结构会对选择抗体易于接触和识别的识别区域有很大的帮助。
然而,在没有这样精确的结构信息(多数是这种情况)的情况下,了解研究的用途(应用)会影响多肽设计的策略。
例如:如果研究重点是集中在蛋白的不同区域,如C端或N端,或在一种特定状态下的蛋白,如磷酸化等,那么按照所需序列设计的多肽和产生的相应的抗体在应用上应该没有太大的困难,然而,蛋白的构象将影响抗体与其识别区域之间的相互作用。
这种情况下可能存在的问题是如果在折叠的蛋白中,该识别区域被藏在蛋白的内部,抗体将无法接触到该区域。
寻找上游靶基因的方法
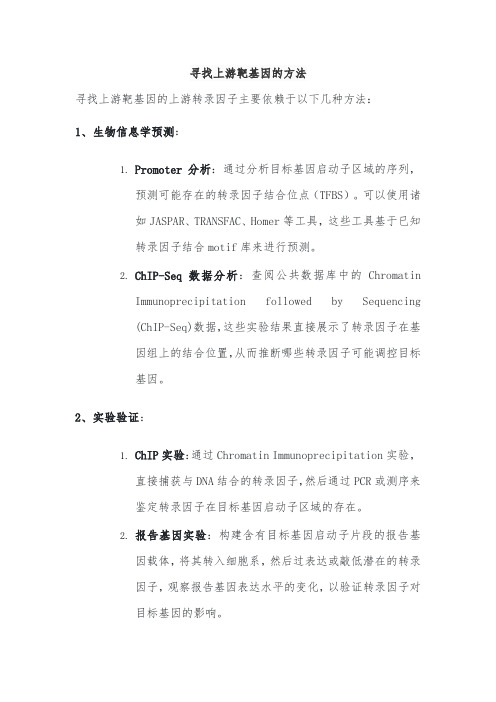
寻找上游靶基因的方法寻找上游靶基因的上游转录因子主要依赖于以下几种方法:1、生物信息学预测:1.Promoter分析:通过分析目标基因启动子区域的序列,预测可能存在的转录因子结合位点(TFBS)。
可以使用诸如JASPAR、TRANSFAC、Homer等工具,这些工具基于已知转录因子结合motif库来进行预测。
2.ChIP-Seq数据分析:查阅公共数据库中的ChromatinImmunoprecipitation followed by Sequencing(ChIP-Seq)数据,这些实验结果直接展示了转录因子在基因组上的结合位置,从而推断哪些转录因子可能调控目标基因。
2、实验验证:1.ChIP实验:通过Chromatin Immunoprecipitation实验,直接捕获与DNA结合的转录因子,然后通过PCR或测序来鉴定转录因子在目标基因启动子区域的存在。
2.报告基因实验:构建含有目标基因启动子片段的报告基因载体,将其转入细胞系,然后过表达或敲低潜在的转录因子,观察报告基因表达水平的变化,以验证转录因子对目标基因的影响。
3、基因表达谱关联分析:1.结合转录组测序(RNA-Seq)或微阵列数据,分析转录因子敲除或过表达时,下游基因表达谱的变化,找出与转录因子表达水平显著相关的基因,进一步筛选可能的靶基因。
4、CRISPR/Cas9基因编辑技术:1.利用CRISPR-Cas9系统在目标基因启动子区域内进行定点编辑,破坏潜在的转录因子结合位点,通过观察靶基因表达的变化,来验证转录因子与靶基因的关系。
综合以上方法,既可以初步通过生物信息学预测缩小范围,也能通过实验手段来验证预测结果,从而确定转录因子对靶基因的调控关系。
- 1、下载文档前请自行甄别文档内容的完整性,平台不提供额外的编辑、内容补充、找答案等附加服务。
- 2、"仅部分预览"的文档,不可在线预览部分如存在完整性等问题,可反馈申请退款(可完整预览的文档不适用该条件!)。
- 3、如文档侵犯您的权益,请联系客服反馈,我们会尽快为您处理(人工客服工作时间:9:00-18:30)。
Protein Domain Prediction Using Profiles,Secondary Structure,Relative Solvent Accessibility,and Recursive Neural Networks Jianlin Cheng Michael J.Sweredoski Pierre BaldiSchool of Information and Computer ScienceInstitute for Genomics and BioinformaticsUniversity of California,Irvine{jianlinc,msweredo,pfbaldi}@AbstractProtein domains are considered the basic unit of protein tertiary struc-ture.We have developed a1D-Recursive Neural Network(1D-RNN)called DOMpro that predicts protein domains using a combination ofevolutionary information in the form of profiles and predicted secondarystructure and relative solvent accessibility.DOMpro is trained and testedon a curated dataset derived from the CATH database.DOMpro cor-rectly predicts the number of domains for69%of the combined datasetof single and multi-domain chains.79%of the single domain proteinsare correctly predicted as having no domain boundaries.The numberof domains is correctly predicted for43%of the multi-domain proteins.DOMpro is able to correctly predict both the domain number and do-main boundary location for25%of the two domain chains.DOMpro sa member of the SCRATCH suite of predictors available through http:///servers/servers.html.1IntroductionDomains are considered the basic unit of protein tertiary structure.Most defi-nitions of domains rely on different criteria ranging from the ability to fold in-dependently to evolutionary conservation and discrete functionality(Holm and Sander,1994).A domain can span an entire polypeptide chain or a subunit of a polypeptide chain that can fold into a stable tertiary structure independently of any other domain(Levitt and Chothia,1976).Additionally,while many do-mains are comprised of a single continuous polypeptide segment,in some cases domains may be comrpised of several discontinuous polypeptide segments.The identification of protein domains is an important step when classifying or predicting protein structures.The topology of secondary structure elements1in a domain is used by human experts or automated systems in structural clas-sification databases such as FSSP-Dali Domain Dictionary(Holm and Sander, 1998a;Holm and Sander,1998b),SCOP(Murzin et al.,1995),CATH(Orengo et al.,2002).The prediction of protein tertiary structure,especially ab initio prediction,can be improved by using domain boundary information(Chivian et al.,2003)and applying prediction methods separately to each domain.How-ever,the identification of protein domains based on sequence alone remains a challenging problem.A number of methods have been developed to identify protein domains from sequences.Some of these methods use a sequence alignment approach whereby domains are identified by aligning the target sequence against sequences in a domain classification database(Marchler-Bauer et al.,2003).Other methods use alignments of secondary structure(Marsden et al.,2002).In these meth-ods,domains are assigned by aligning the predicted secondary structure of a target sequence against the secondary structure of chains in CATH with known domain boundaries.Tertiary structure folding approaches such as Snap-DRAGON(George and Heringa,2002)average several hundred predictions ob-tained from coarse ab initio simulations of protein folding for a given sequence to assign its domain content.One drawback to such approaches is that they are computationally intensive and not yet particularly accurate.Statistical meth-ods such as Domain Guess by Size(DGS)(Wheelan et al.,2000)predict the likelihood of domain boundaries within a given sequence based on statistical distributions of chain and domain lengths.The prediction of domains using machine learning techniques is aided by the availability of large,high quality domain classification databases such as CATH,SCOP and DaliDD.Two recently published algorithms attempt to pre-dict domain boundaries using neural networks(Liu and Rost,2004;Nagarajan and Yona,2004).The networks used by Nagarajan and Yona(2004)incorpo-rate the position specific physio-chemical properties of amino acid and predicted secondary structure.Liu and Rost(2004)use neural networks with amino acid composition and predicted secondary structure and solvent accessibility.Here we describe DOMpro,a novel machine learning approach for predict-ing domains which uses position specific scoring matrices(PSSMs)along with predicted secondary structure and solvent accessibility in a1D-recursive neural network(1D-RNN).These networks are also used for prediction of the secondary structure and solvent accessibility(Pollastri et al.,2001a;Pollastri et al.,2001b) in our SCRATCH suite of servers(Baldi and Pollastri,2003).The use of PSSMs in DOMpro is based on the assumption that sequence motifs in the boundary regions are different from those found in the rest of the protein.Thefinal assign-ment of protein domains is the result of post-processing and statistical inference on the output of the neural network.DOMpro was evaluated along with several other protein domain predictors in CASP6.The results of CASP6are available at http://predictioncenter. /casp6/Casp6.html.22Methods2.1DataDOMpro is trained and tested on a dataset derived from annotated domains in the CATH domain database,version2.5.1.Because the CATH database con-tains only the sequences of domain regions,we must incorporate the sequences from the PDB to reconstruct the entire chains.Once the chains are reconstructed,short sequences(<40residues)arefil-tered out of our dataset.We then use uniqueProt(Mika and Rost,2003)to reduce the sequence redundancy in the dataset.We ensure that no pair of se-quences in the dataset have a HSSP value greater than5.The HSSP value between two sequences is a measure of their similarity and considers both se-quence identity and sequence length.A HSSP value of5corresponds roughly to a pair of250residue proteins with20%sequence identity.Figure1:Frequency of single and multi-domain chains in the redundancy-reduced dataset.After redundancy reduction,our dataset contains355multi-domain chains and963single domain chains.The ratio of single to multi-domain chains reflects the skewed distribution of single domain chains in the PDB(Berman et al.,2000). Figure1shows the frequency of multiple and single domain chains in our dataset. Figure2shows the distribution of chain lengths among single and multi-domain chains.Because the neural networks are trained to recognize domain boundaries, only multi-domain proteins are used during the training process.During the training and testing of the neural networks on multi-domain proteins,10fold3Figure2:Distribution of the lengths of single and multi-domain chains in the redundancy-reduced dataset.cross-validation is used.Additional testing is performed on single domain pro-teins using models trained with multi-domain proteins.2.21D-Recursive Neural Networks(1D-RNN)The problem of predicting domain boundary regions can be viewed as a bi-nary classification problem with numerical and nominal inputs.The target output class for each residue is defined as follows.Residues within20amino acids of a domain boundary are considered domain boundary residues and all other residues are considered non-boundary residues.For the prediction of domain boundary regions,each residue has a corresponding input vector of length25.Twenty of the values are real numbers which correspond to the profile of the PSSM.This profile is generated from the NR database using PSI-BLAST(Altschul et al.,1997).The otherfive values are binary.Three of the values correspond to the predicted secondary structure class of the residue and the other two correspond to the predicted relative solvent accessibility of the residue(i.e.,under or over25%exposed).These predictions are obtained from the SSpro and ACCpro(Pollastri et al.,2001a;Pollastri et al.,2001b;Baldi and Pollastri,2003)servers in the SCRATCH suite.Once the problem is formalized in this way,a variety of machine learning techniques can be applied to it.DOMpro employs a1D recursive neural net-work(1D-RNN)which can handle variable length inputs.The architecture of the1D-RNN is described in Figures3and4and is associated with a set of input variables I i,a forward H F i and backward H B i chain of hidden variables,4and a set O i of output variables.In terms of probabilistic graphical models (Bayesian networks)this is essentially the connectivity pattern of an input-output HMM(Bengio and Frasconi,1996),augmented with a backward chain of hidden states.The backward chain is of course optional and used here to capture the spatial,rather than temporal,properties of biologicalsequences.The relationship between the variables can be modeled using three types of feed-forward neural networks to compute the output,forward,and backward variables respectively.One fairly general form of weight sharing is to assume stationarity for the output,forward,and backward networks,whichfinally leads to a1D-RNN architectures,previously named bidirectional RNN architecture (BRNN),implemented using three neural networks N O,N F,and N B in the formO i=N O(I i,H F i,H B i)H F i=N F(I i,H F i−1)(1)H B i=N B(I i,H B i+1)as depicted in Figure4.In this form,the output depends on the local input I i at position i,the forward(upstream)hidden context H F i∈I R n and the backward (downstream)hidden context H B i∈I R m,with usually m=n.The boundary conditions for H F i and H B i can be set to0,i.e.H F0=H B N+1=0where N is the length of the sequence being processed.Alternatively these boundaries can also be treated as a learnable parameter.Intuitively,we can think of N F and N B in terms of two“wheels”that can be rolled along the sequence.For the prediction at position i,we roll the wheels in opposite directions starting from the N-and C-terminus and up to position i.Then we combine the wheel outputs at position i together with the input I i to compute the output prediction O i using N O.In domain boundary prediction,the output O i is computed by two normalized-exponential units and correspond to the membership probability of the residue5Figure4:A1D-RNN architecture with a left(forward)and right(backward) context associated with two recurrent networks(wheels).at position i in either the boundary or non-boundary class.The error function is the relative entropy between the true distribution and the predicted distribu-tion.All the weights of the1D-RNN architecture,including the weights in the recurrent wheels,are trained in a supervised fashion using a generalized form of gradient descent derived by unfolding the wheels in space.2.3Post-Processing Of1D-RNN OutputThe raw output from our1D-RNN is quite noisy(See Figure5).DOMpro uses smoothing to help correct for the random noise that is the result of false positive hits.The smoothing is accomplished by averaging over a window of length3 arouind each position.Figure5shows how this smoothing technique helps to reduce the noise found in the raw output of the1D-RNN.After smoothing,a do-main state(boundary/not boundary)is assigned to each residue by thresholding our networks output at.5.While smoothing the neural network output helps correct for random spikes, it does not necessarily create the long,continuous segments of boundary residues that are required for domain assignment.Therefore,further inference on the output is required.DOMpro infers domain boundary regions from residues predicted as domain boundaries by pattern matching on the discretized output.Any section of the output which matches the pattern((B+N{0,m})*B+)is considered a domain boundary regions,where B is a predicted boundary residue,N is a predicted6Raw output from1D-RNN Smoothed output from1D-RNNwith window Width3Figure5:Smoothing of raw output from1D-RNNFalse positive boundaries True boundaries Figure6:Length distributions of true and false positive boundary regions non-boundary residue and m is the maximum separation between two boundary residues which should be merged into one region.Once DOMpro has inferred all possible domain boundary regions,we need to identify false positive domain boundary regions.DOMpro considers the bound-ary region’s length a measure of its signal strength.Figure6shows that there is a clear difference between the length distributions of true domain boundary regions and false domain boundary regions.Based on these statistics,domain boundary regions shorter than3residues are considered false positive hits and are ignored.The target sequence is then cut into domain segments at the middle residue of each boundary region.A target sequence with no predicted domain boundaries is classified as a single domain chain.Thefinal step of DOMpro is to assign domain numbers to each predicted domain segment.One naive method is to assign each domain segment to a separate domain.However,this method fails to identify discontinuous domains.7One possible strategy to overcome this problem is to combine predicted domain segment information with predicted contact map information in order to assign domain numbers.To handle discontinuous domains comprising two ore more disjoint segments,the predicted contact map from CMAPpro(Baldi and Pol-lastri,2003)is used to decide whether non-adjacent segments have a sufficient number of residue-residue contacts to be considered a single domain.3ResultsFigure7:Frequency of under and over prediction of the number of domains by DOMpro and a naive predictorThefirst step in evaluating a domain predictor is to compare the predicted number of domains to the true number of domains.DOMpro correctly predicted the number of domains for69%of the combined dataset of single and multi-domain proteins.79%of the single domain proteins were correctly predicted as having no domain boundaries.The number of domains is correctly predicted for43%of the multi-domain proteins.Figure7shows the relative frequency of under and over prediction of the number of domains by DOMpro in addition to a predictor based solely on the chain lengths.This simple predictor classifies a chain as having one domain if its length is less than220residues,two domains if its length is between220and400residues,three domains if its length is between 400and600residues and four domains if its length is greater than600residues. These thresholds come from statistics on the number of residues per domain.DOMpro is able to correctly predict both the domain number and domain boundary location for20%of the multi-domain chains.For the evaluation of8multi-domain chains,we consider that a domain boundary has been correctly identified if the predicted domain boundary is within20residues of the true domain boundary,as annotated in the CATH database.The comparison of domain predictors is complicated by the existence of mul-tiple domain datasets which sometimes conflict with each other.Thus,the per-formance of a predictor on a dataset other than its training dataset is bounded by the percentage of agreement between the training and testing datasets.With this in mind,we will only make specific comparisons between domain predic-tors.DOMpro is able to correctly predict25%of the two domain proteins in our dataset derived from CATH.This is in comparison to Liu and Rost(2004)who achieves19%accuracy on a different dataset derived from CATH and SCOP. 4ConclusionsWe have created DOMpro,an ab initio predictor of protein domains using neural networks with PSSMs and predicted secondary structure and relative solvent accessibility.DOMpro raw output isfiltered in order to produce thefinal domain segmentation and assignment.Our analysis shows that DOMpro achieves a level of performances that is better or comparable to the level of current ab initio domain boundary predictors.Domain prediction,however,remains a challenging problem and clearly there is room for considerable improvement.We are currently adding a module to DOMpro to use homology for domain assignment for proteins that are homol-ogous to known structures in the PDB and CATH databases.In addition,we are focusing on the prediction/classification of discontinuous domains.To over-come the limitations of our naive assignment,we are experimenting with the use of predicted contact maps,as well as domain length statistics,in deciding whether or not two non-adjacent domain segments should be joined.The con-tact maps are predicted using2D-RNNs(Pollastri and Baldi,2002;Baldi and Pollastri,2003).The basic idea is that two discontinuous segments witht he proper length statistics and with a sufficient number of inter-segment residue-residue contacts might be considered as belonging to the same domain. AcknowledgmentWork supported by the Institute for Genomics and Bioinformatics at UCI,a Laurel Wilkening Faculty Innovation award,an NIH Biomedical Informatics Training grant(LM-07443-01),an NSF MRI grant(EIA-0321390),a Sun Mi-crosystems award,a grant from the University of California Systemwide Biotech-nology Research and Education Program(UC BREP)to PB.References9Altschul,S.,Madden,T.,Schaffer,A.,Zhang,J.,Zhang,Z.,Miller,W.and Lip-man,D.(1997)Gapped blast and psi-blast:a new generation of protein database search programs.Nucleic Acids Res,25(17),3389–3402.Baldi,P.and Pollastri,G.(2003)The principled design of large-scale recursive neural network architectures–DAG-RNNs and the protein structure predic-tion problem.Journal of Machine Learning Research,4,575–602.Bengio,Y.and Frasconi,P.(1996)Input-output HMM’s for sequence processing.IEEE Transactions on Neural Networks,7(5),1231–1249.Berman,H.M.,Westbrook,J.,Feng,Z.,Gilliland,G.,Bhat,T.N.,Weissig,H., Shindyalov,I.N.and Bourne,P.(2000)The protein data bank.Nucleic Acids Research,28,235–242.Chivian,D.,Kim,D.,Malmstro,L.,Bradley,P.,Robertson,R.,Murphy,P., Strauss,C.,Bonneau,R.,Rohl,C.and Baker,D.(2003)Automated predic-tion of casp-5structures using the robetta server.Proteins,53(S6), 524–533.George,R.and Heringa,J.(2002)Snapdragon:a method to delineate protein structural domains from sequence data.J.Mol.Biol.,316,839–851. Holm,L.and Sander,C.(1994)Parser for protein folding units.Proteins Struct.Funct.Genet.,19,256–268.Holm,L.and Sander,C.(1998a)Dictionary of recurrent domains in protein struc-tures.Proteins,33,88–96.Holm,L.and Sander,C.(1998b)Touring protein fold space with dali/fssp.Nucl.Acids Res.,26,316–319.Levitt,M.and Chothia,C.(1976)Structural patterns in globular proteins.Na-ture,261(5561),552–558.Liu,J.and Rost,B.(2004)Sequence-based prediction of protein domains.Nucl.Acids Res.,32(12),3522–3530.Marchler-Bauer,A.,Anderson,J.B.,DeWeese-Scott,C.,Fedorova,N.D., Geer,L.Y.,He,S.,Hurwitz,D.I.,Jackson,J.D.,Jacobs,A.R.,Lanczycki,C.J., Liebert,C.A.,Liu,C.,Madej,T.,Marchler,G.H.,Mazumder,R.,Nikol-skaya,A.N.,Panchenko,A.R.,Rao,B.S.,Shoemaker,B.A.,Simonyan,V., Song,J.S.,Thiessen,P.A.,Vasudevan,S.,Wang,Y.,Yamashita,R.A., Yin,J.J.and Bryant,S.H.(2003)CDD:a curated Entrez database of conserved domain alignments.Nucl.Acids Res.,31(1),383–387. Marsden,R.,McGuffin,L.and Jones,D.(2002)Rapid protein domain assignment from amino acid sequence using predicted secondary structure.Protein Science,11,2814–2824.10Mika,S.and Rost,B.(2003)Uniqueprot:creating representative protein-sequence sets.Nucleic Acids Res,31(13),3789–3791.Murzin,A.,Brenner,S.,Hubbard,T.and Chothia,C.(1995)Scop:a structural classification of proteins database for the investigation of sequences and structures.J.Mol.Biol.,247,536–540.Nagarajan,N.and Yona,G.(2004)Automatic prediction of protein domains from sequence information using a hybrid learning system.Bioinformatics,20(9),1335–1360.Orengo,C.,Bray,J.,Buchan,D.,Harrison,A.,Lee,D.,Pearl,F.,Sillitoe,I., Todd,A.and Thornton,J.(2002)The cath protein family database:a re-source for structural and functional annotation of genomes.Proteomics,2, 11–21.Pollastri,G.and Baldi,P.(2002)Predition of contact maps by GIOHMMs and recurrent neural networks using lateral propagation from all four cardinal corners.Bioinformatics,18Supplement1,S62–S70.Proceedings of the ISMB2002Conference.Pollastri,G.,Baldi,P.,Fariselli,P.and Casadio,R.(2001a)Prediction of coordi-nation number and relative solvent accessibility in proteins.Proteins,47, 142–153.Pollastri,G.,Przybylski,D.,Rost,B.and Baldi,P.(2001b)Improving the predic-tion of protein secondary strucure in three and eight classes using recurrent neural networks and profiles.Proteins,47,228–235.Wheelan,S.J.,Marchler-Bauer,A.and Bryant,S.H.(2000)Domain size distribu-tions can predict domain boundaries.Bioinformatics,16(7),613–618.11。