优先队列式分支限界法求解0-1背包问题
分支限界法结局0~1背包问题
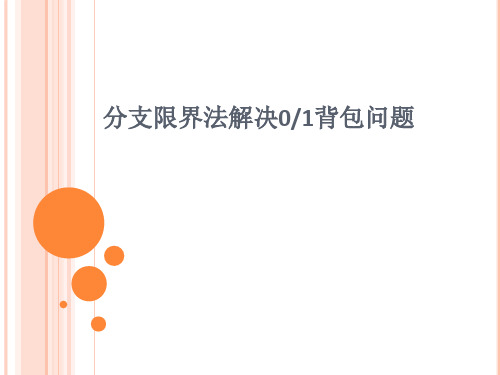
Bound( i ) cleft = c – cw; b = cp; while( i <= n && w[i] <= cleft ){ cleft -= w[i]; b += p[i]; i++; } if( i<=n) b += p[i]/w[i] * cleft; return b; }
此后,从活结点表中取下一结点成为当前扩展结点,并重复上述结 点扩展过程。这个过程一直持续到找到所需的解或活结点表为空时为 止。
与回溯法区别
求解目标不同: 一般而言,回溯法的求解目标是找出解空间树中满 足的约束条件的所有解,而分支限界法的求解目标 则是尽快的找出满足约束条件的一个解。
搜索方法不同 回溯法使用深度优先方法搜索,而分支限界一般用宽 度优先或最佳优先方法来搜索;
按照队列先进先出(FIFO)原则选取下一个节点为扩展节点;
数据结构:队列
(2)优先队列式分支限界法
按照优先队列中规定的优先级选取优先级最高的节点成为当前 扩展节点。 数据结构:堆 最大优先队列:使用最大堆,体现最大效益优先
最小优先队列:使用最小堆,体现最小费用优先
【0-1背包问题】
物品数量n=3,重量w=(20,15,15),价值v=(40,25,25) 背包容量c=30,试装入价值和最大的物品? 解空间:{(0,0,0),(0,0,1),…,(1,1,1)}
分支限界法解决0/1背包问题
分支限界法思想概述 与回溯法区别 求解步骤 常见的两种分支限界法 0-1背包问题
分支限界法的基本思想
分支限界法基本思想
分支限界法常以广度优先或以最小耗费(最大效益)优先的方式搜 索问题的解空间树。
0036算法笔记——【分支限界法】0-1背包问题
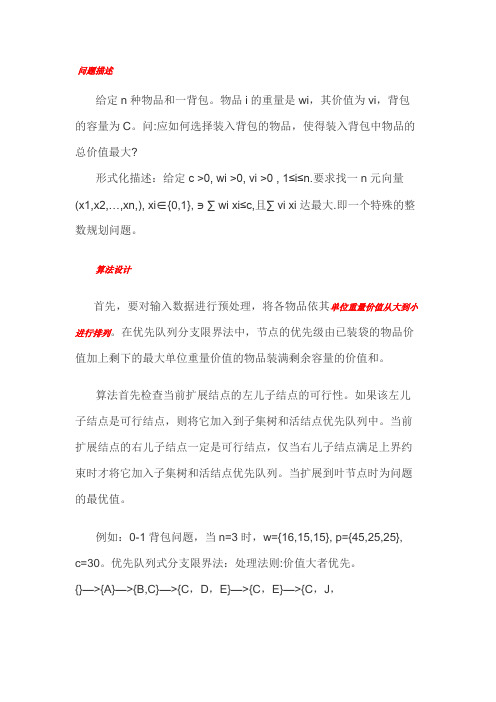
问题描述给定n种物品和一背包。
物品i的重量是wi,其价值为vi,背包的容量为C。
问:应如何选择装入背包的物品,使得装入背包中物品的总价值最大?形式化描述:给定c >0, wi >0, vi >0 , 1≤i≤n.要求找一n元向量(x1,x2,…,xn,), xi∈{0,1}, ∋∑ wi xi≤c,且∑ vi xi达最大.即一个特殊的整数规划问题。
算法设计首先,要对输入数据进行预处理,将各物品依其单位重量价值从大到小进行排列。
在优先队列分支限界法中,节点的优先级由已装袋的物品价值加上剩下的最大单位重量价值的物品装满剩余容量的价值和。
算法首先检查当前扩展结点的左儿子结点的可行性。
如果该左儿子结点是可行结点,则将它加入到子集树和活结点优先队列中。
当前扩展结点的右儿子结点一定是可行结点,仅当右儿子结点满足上界约束时才将它加入子集树和活结点优先队列。
当扩展到叶节点时为问题的最优值。
例如:0-1背包问题,当n=3时,w={16,15,15}, p={45,25,25}, c=30。
优先队列式分支限界法:处理法则:价值大者优先。
{}—>{A}—>{B,C}—>{C,D,E}—>{C,E}—>{C,J,K}—>{C}—>{F,G}—>{G,L,M}—>{G,M}—>{G}—>{N,O}—>{O}—>{}算法代码实现如下:1、MaxHeap.h[cpp]view plain copy1.template<class T>2.class MaxHeap3.{4.public:5. MaxHeap(int MaxHeapSize = 10);6. ~MaxHeap() {delete [] heap;}7.int Size() const {return CurrentSize;}8.9. T Max()10. { //查11.if (CurrentSize == 0)12. {13.throw OutOfBounds();14. }15.return heap[1];16. }17.18. MaxHeap<T>& Insert(const T& x); //增19. MaxHeap<T>& DeleteMax(T& x); //删20.21.void Initialize(T a[], int size, int ArraySize);22.23.private:24.int CurrentSize, MaxSize;25. T *heap; // element array26.};27.28.template<class T>29.MaxHeap<T>::MaxHeap(int MaxHeapSize)30.{// Max heap constructor.31. MaxSize = MaxHeapSize;32. heap = new T[MaxSize+1];33. CurrentSize = 0;34.}35.36.template<class T>37.MaxHeap<T>& MaxHeap<T>::Insert(const T& x)38.{// Insert x into the max heap.39.if (CurrentSize == MaxSize)40. {41. cout<<"no space!"<<endl;42.return *this;43. }44.45.// 寻找新元素x的位置46.// i——初始为新叶节点的位置,逐层向上,寻找最终位置47.int i = ++CurrentSize;48.while (i != 1 && x > heap[i/2])49. {50.// i不是根节点,且其值大于父节点的值,需要继续调整51. heap[i] = heap[i/2]; // 父节点下降52. i /= 2; // 继续向上,搜寻正确位置53. }54.55. heap[i] = x;56.return *this;57.}58.59.template<class T>60.MaxHeap<T>& MaxHeap<T>::DeleteMax(T& x)61.{// Set x to max element and delete max element from heap.62.// check if heap is empty63.if (CurrentSize == 0)64. {65. cout<<"Empty heap!"<<endl;66.return *this;67. }68.69. x = heap[1]; // 删除最大元素70.// 重整堆71. T y = heap[CurrentSize--]; // 取最后一个节点,从根开始重整72.73.// find place for y starting at root74.int i = 1, // current node of heap75. ci = 2; // child of i76.77.while (ci <= CurrentSize)78. {79.// 使ci指向i的两个孩子中较大者80.if (ci < CurrentSize && heap[ci] < heap[ci+1])81. {82. ci++;83. }84.// y的值大于等于孩子节点吗?85.if (y >= heap[ci])86. {87.break; // 是,i就是y的正确位置,退出88. }89.90.// 否,需要继续向下,重整堆91. heap[i] = heap[ci]; // 大于父节点的孩子节点上升92. i = ci; // 向下一层,继续搜索正确位置93. ci *= 2;94. }95.96. heap[i] = y;97.return *this;98.}99.100.template<class T>101.void MaxHeap<T>::Initialize(T a[], int size,int ArraySize) 102.{// Initialize max heap to array a.103.delete [] heap;104. heap = a;105. CurrentSize = size;106. MaxSize = ArraySize;107.108.// 从最后一个内部节点开始,一直到根,对每个子树进行堆重整109.for (int i = CurrentSize/2; i >= 1; i--)110. {111. T y = heap[i]; // 子树根节点元素112.// find place to put y113.int c = 2*i; // parent of c is target114.// location for y115.while (c <= CurrentSize)116. {117.// heap[c] should be larger sibling118.if (c < CurrentSize && heap[c] < heap[c+1])119. {120. c++;121. }122.// can we put y in heap[c/2]?123.if (y >= heap[c])124. {125.break; // yes126. }127.128.// no129. heap[c/2] = heap[c]; // move child up130. c *= 2; // move down a level131. }132. heap[c/2] = y;133. }134.}2、6d5.cpp[cpp]view plain copy1.//0-1背包问题分支限界法求解2.#include "stdafx.h"3.#include "MaxHeap.h"4.#include <iostream>ing namespace std;6.7.class Object8.{9.template<class Typew,class Typep>10.friend Typep Knapsack(Typep p[],Typew w[],Typew c,int n, int bestx[]);11.public:12.int operator <= (Object a) const13. {14.return d>=a.d;15. }16.private:17.int ID;18.float d;//单位重量价值19.};20.21.template<class Typew,class Typep> class Knap;22.23.class bbnode24.{25.friend Knap<int,int>;26.template<class Typew,class Typep>27.friend Typep Knapsack(Typep p[],Typew w[],Typew c,int n, int bestx[]);28.private:29. bbnode * parent; //指向父节点的指针30.bool LChild; //左儿子节点标识31.};32.33.template<class Typew,class Typep>34.class HeapNode35.{36.friend Knap<Typew,Typep>;37.public:38. operator Typep() const39. {40.return uprofit;41. }42.private:43. Typep uprofit, //节点的价值上界44. profit; //节点所相应的价值45. Typew weight; //节点所相应的重量46.int level; //活节点在子集树中所处的层序号47. bbnode *ptr; //指向活节点在子集中相应节点的指针48.};49.50.template<class Typew,class Typep>51.class Knap52.{53.template<class Typew,class Typep>54.friend Typep Knapsack(Typep p[],Typew w[],Typew c,int n, int bestx[]);55.public:56. Typep MaxKnapsack();57.private:58. MaxHeap<HeapNode<Typep,Typew>> *H;59. Typep Bound(int i);60.void AddLiveNode(Typep up,Typep cp,Typew cw,bool ch,int lev);61.62. bbnode *E; //指向扩展节点的指针63. Typew c; //背包容量64.int n; //物品数65.66. Typew *w; //物品重量数组67. Typep *p; //物品价值数组68. Typew cw; //当前重量69.70. Typep cp; //当前价值71.int *bestx; //最优解72.};73.74.template <class Type>75.inline void Swap(Type &a,Type &b);76.77.template<class Type>78.void BubbleSort(Type a[],int n);79.80.int main()81.{82.int n = 3;//物品数83.int c = 30;//背包容量84.int p[] = {0,45,25,25};//物品价值下标从1开始85.int w[] = {0,16,15,15};//物品重量下标从1开始86.int bestx[4];//最优解87.88. cout<<"背包容量为:"<<c<<endl;89. cout<<"物品重量和价值分别为:"<<endl;90.91.for(int i=1; i<=n; i++)92. {93. cout<<"("<<w[i]<<","<<p[i]<<") ";94. }95. cout<<endl;96.97. cout<<"背包能装下的最大价值为:"<<Knapsack(p,w,c,n,bestx)<<endl;98. cout<<"此背包问题最优解为:"<<endl;99.for(int i=1; i<=n; i++)100. {101. cout<<bestx[i]<<" ";102. }103. cout<<endl;104.return 0;105.}106.107.template<class Typew,class Typep>108.Typep Knap<Typew,Typep>::Bound(int i)//计算节点所相应价值的上界109.{110. Typew cleft = c-cw; //剩余容量高111. Typep b = cp; //价值上界112.//以物品单位重量价值递减序装填剩余容量113.while(i<=n && w[i]<=cleft)114. {115. cleft -= w[i];116. b += p[i];117. i++;118. }119.120.//装填剩余容量装满背包121.if(i<=n)122. {123. b += p[i]/w[i]*cleft;124. }125.126.return b;127.}128.129.//将一个活节点插入到子集树和优先队列中130.template<class Typew,class Typep>131.void Knap<Typew,Typep>::AddLiveNode(Typep up,Typep cp,Typew cw,bool ch,int lev)132.{133. bbnode *b = new bbnode;134. b->parent = E;135. b->LChild = ch;136.137. HeapNode<Typep,Typew> N;138. N.uprofit = up;139. N.profit = cp;140. N.weight = cw;141. N.level = lev;142. N.ptr = b;143.144. H->Insert(N);145.}146.147.//优先队列式分支限界法,返回最大价值,bestx返回最优解148.template<class Typew,class Typep>149.Typep Knap<Typew,Typep>::MaxKnapsack()150.{151. H = new MaxHeap<HeapNode<Typep,Typew>>(1000);152.153.//为bestx分配存储空间154. bestx = new int[n+1];155.156.//初始化157.int i = 1;158. E = 0;159. cw = cp = 0;160. Typep bestp = 0;//当前最优值161. Typep up = Bound(1); //价值上界162.163.//搜索子集空间树164.while(i!=n+1)165. {166.//检查当前扩展节点的左儿子节点167. Typew wt = cw + w[i];168.if(wt <= c)//左儿子节点为可行节点169. {170.if(cp+p[i]>bestp)171. {172. bestp = cp + p[i];173. }174. AddLiveNode(up,cp+p[i],cw+w[i],true,i+1); 175. }176.177. up = Bound(i+1);178.//检查当前扩展节点的右儿子节点179.if(up>=bestp)//右子树可能含有最优解180. {181. AddLiveNode(up,cp,cw,false,i+1);182. }183.184.//取下一扩展节点185. HeapNode<Typep,Typew> N;186. H->DeleteMax(N);187. E = N.ptr;188. cw = N.weight;189. cp = N.profit;190. up = N.uprofit;191. i = N.level;192. }193.194.//构造当前最优解195.for(int j=n; j>0; j--)196. {197. bestx[j] = E->LChild;198. E = E->parent;199. }200.return cp;201.}202.203.//返回最大价值,bestx返回最优解204.template<class Typew,class Typep>205.Typep Knapsack(Typep p[],Typew w[],Typew c,int n, int bestx[]) 206.{207.//初始化208. Typew W = 0; //装包物品重量209. Typep P = 0; //装包物品价值210.211.//定义依单位重量价值排序的物品数组212. Object *Q = new Object[n];213.for(int i=1; i<=n; i++)214. {215.//单位重量价值数组216. Q[i-1].ID = i;217. Q[i-1].d = 1.0*p[i]/w[i];218. P += p[i];219. W += w[i];220. }221.222.if(W<=c)223. {224.return P;//所有物品装包225. }226.227.//依单位价值重量排序228. BubbleSort(Q,n);229.230.//创建类Knap的数据成员231. Knap<Typew,Typep> K;232. K.p = new Typep[n+1];233. K.w = new Typew[n+1];234.235.for(int i=1; i<=n; i++)236. {237. K.p[i] = p[Q[i-1].ID];238. K.w[i] = w[Q[i-1].ID];239. }240.241. K.cp = 0;242. K.cw = 0;243. K.c = c;244. K.n = n;245.246.//调用MaxKnapsack求问题的最优解247. Typep bestp = K.MaxKnapsack();248.for(int j=1; j<=n; j++)249. {250. bestx[Q[j-1].ID] = K.bestx[j]; 251. }252.253.delete Q;254.delete []K.w;255.delete []K.p;256.delete []K.bestx;257.return bestp;258.}259.260.template<class Type>261.void BubbleSort(Type a[],int n)262.{263.//记录一次遍历中是否有元素的交换264.bool exchange;265.for(int i=0; i<n-1;i++)266. {267. exchange = false ;268.for(int j=i+1; j<=n-1; j++) 269. {270.if(a[j]<=a[j-1])271. {272. Swap(a[j],a[j-1]);273. exchange = true;274. }275. }276.//如果这次遍历没有元素的交换,那么排序结束277.if(false == exchange)278. {279.break ;280. }281. }282.}283.284.template <class Type>285.inline void Swap(Type &a,Type &b) 286.{287. Type temp = a;288. a = b;289. b = temp;290.}程序运行结果如图:。
算法 0-1背包问题教学教材
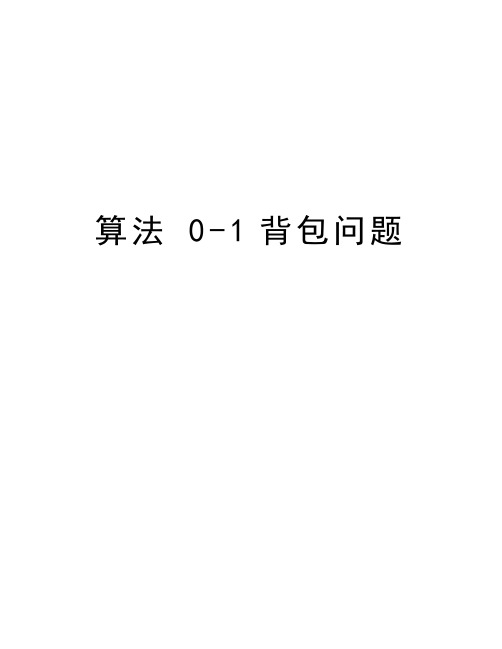
算法0-1背包问题一、实验目的与要求掌握回溯法、分支限界法的原理,并能够按其原理编程实现解决0-1背包问题,以加深对回溯法、分支限界法的理解。
1.要求分别用回溯法和分支限界法求解0-1背包问题;2.要求交互输入背包容量,物品重量数组,物品价值数组;3.要求显示结果。
二、实验方案在选择装入背包的物品时,对每种物品i只有2种选择,即装入背包或不装入背包。
不能将物品i装入背包多次,也不能只装入部分的物品i。
三、实验结果和数据处理1.用回溯法解决0-1背包问题:代码:import java.util.*;public class Knapsack{private double[] p,w;//分别代表价值和重量private int n;private double c,bestp,cp,cw;private int x[]; //记录可选的物品private int[] cx;public Knapsack (double pp[],double ww[],double cc){this.p=pp;this.w=ww;this.n=pp.length-1;this.c=cc;this.cp=0;this.cw=0;this.bestp=0;x=new int[ww.length];cx=new int[pp.length];}void Knapsack(){backtrack(0);}void backtrack(int i){if(i>n){ //判断是否到达了叶子节点if(cp>bestp){for(int j=0;j<x.length;j++)x[j]=cx[j];bestp=cp;}return;}if(cw+w[i]<=c){//搜索右子树cx[i]=1;cw+=w[i];cp+=p[i];backtrack(i+1);cw-=w[i];cp-=p[i];}cx[i]=0;backtrack(i+1); //检查左子树}void printResult(){System.out.println("回溯法");System.out.println("物品个数:n=4");System.out.println("背包容量:c=7");System.out.println("物品重量数组:w= {3,5,2,1}"); System.out.println("物品价值数组:p= {9,10,7,4}"); System.out.println("最优值:="+bestp);System.out.println("选中的物品是:");for(int i=0;i<x.length;i++){System.out.print(x[i]+" ");}}public static void main(String[] args){double p[]={9,10,7,4};double w[]={3,5,2,1};int maxweight=7;Knapsack ks=new Knapsack(p,w,maxweight);ks.Knapsack(); //回溯搜索ks.printResult();}}运行结果:2.用优先队列式分支限界法解决0-1背包问题:代码:public class Knapsack{static double c;static int n;static double w[];static double p[];static double cw;static double cp;static int bestX[];static MaxHeap heap;//上界函数bound计算结点所相应价值的上界private static double bound(int i){double cleft=c-cw;double b=cp;while(i<=n&&w[i]<=cleft){cleft=cleft-w[i];b=b+p[i];i++;}//装填剩余容量装满背包if(i<=n)b=b+p[i]/w[i]*cleft;return b;}//addLiveNode将一个新的活结点插入到子集树和优先队列中private static void addLiveNode(double up,double pp,double ww,int lev,BBnode par,boolean ch){//将一个新的活结点插入到子集树和最大堆中BBnode b=new BBnode(par,ch);HeapNode node =new HeapNode(b,up,pp,ww,lev);heap.put(node);}private static double MaxKnapsack(){//优先队列式分支限界法,返回最大价值,bestx返回最优解BBnode enode=null;int i=1;double bestp=0;//当前最优值double up=bound(1);//当前上界while(i!=n+1){//非叶子结点//检查当前扩展结点的左儿子子结点double wt=cw+w[i];if(wt<=c){if(cp+p[i]>bestp)bestp=cp+p[i];addLiveNode(up,cp+p[i],cw+w[i],i+1,enode,true);}up=bound(i+1);if(up>=bestp)addLiveNode(up,cp,cw,i+1,enode,false);HeapNode node =(HeapNode)heap.removeMax();enode=node.liveNode;cw=node.weight;cp=node.profit;up=node.upperProfit;i=node.level;}for(int j=n;j>0;j--){bestX[j]=(enode.leftChild)?1:0;enode=enode.parent;}return cp;}public static double Knapsack(double pp[],double ww[],double cc,int xx[]) {//返回最大值,bestX返回最优解c=cc;n=pp.length-1;//定义以单位重量价值排序的物品数组Element q[]=new Element[n];double ws=0.0;double ps=0.0;for(int i=0;i<n;i++){q[i]=new Element(i+1,pp[i+1]/ww[i+1]);ps=ps+pp[i+1];ws=ws+ww[i+1];}if(ws<=c)return ps;}p=new double[n+1];w=new double[n+1];for(int i=0;i<n;i++){p[i+1]=pp[q[i].id];w[i+1]=ww[q[i].id];}cw=0.0;cp=0.0;bestX = new int[n+1];heap = new MaxHeap(n);double bestp = MaxKnapsack();for(int j=0;j<n;j++)xx[q[j].id]=bestX[j+1];return bestp;}public static void main(String [] args){double w[]=new double[5];w[1]=3;w[2]=5;w[3]=2;w[4]=1;double p[]=new double[5];p[1]=9;p[2]=10;p[3]=7;p[4]=4;double c=7;int x[] = new int[5];double m = Knapsack(p,w,c,x);System.out.println("优先队列式分支限界法:");System.out.println("物品个数:n=4");System.out.println("背包容量:c=7");System.out.println("物品重量数组:w= {3,5,2,1}"); System.out.println("物品价值数组:p= {9,10,7,4}"); System.out.println("最优值:="+m);System.out.println("选中的物品是:");for(int i=1;i<=4;i++)System.out.print(x[i]+" ");}//子空间中节点类型class BBnode{BBnode parent;//父节点boolean leftChild;//左儿子节点标志BBnode(BBnode par,boolean ch){parent=par;leftChild=ch;}}class HeapNode implements Comparable{BBnode liveNode; // 活结点double upperProfit; //结点的价值上界double profit; //结点所相应的价值double weight; //结点所相应的重量int level; // 活结点在子集树中所处的层次号//构造方法public HeapNode(BBnode node, double up, double pp , double ww,int lev) {liveNode = node;upperProfit = up;profit = pp;weight = ww;level = lev;}public int compareTo(Object o){double xup = ((HeapNode)o).upperProfit;if(upperProfit < xup)return -1;if(upperProfit == xup)return 0;elsereturn 1;}}class Element implements Comparable{int id;double d;public Element(int idd,double dd){id=idd;d=dd;}public int compareTo(Object x){double xd=((Element)x).d;if(d<xd)return -1;if(d==xd)return 0;return 1;}public boolean equals(Object x){return d==((Element)x).d;}}class MaxHeap{static HeapNode [] nodes;static int nextPlace;static int maxNumber;public MaxHeap(int n){maxNumber = (int)Math.pow((double)2,(double)n); nextPlace = 1;//下一个存放位置nodes = new HeapNode[maxNumber];}public static void put(HeapNode node){nodes[nextPlace] = node;nextPlace++;heapSort(nodes);}public static HeapNode removeMax(){HeapNode tempNode = nodes[1];nextPlace--;nodes[1] = nodes[nextPlace];heapSort(nodes);return tempNode;}private static void heapAdjust(HeapNode [] nodes,int s,int m) {HeapNode rc = nodes[s];for(int j=2*s;j<=m;j*=2){if(j<m&&nodes[j].upperProfit<nodes[j+1].upperProfit) ++j;if(!(rc.upperProfit<nodes[j].upperProfit))break;nodes[s] = nodes[j];s = j;}nodes[s] = rc;}private static void heapSort(HeapNode [] nodes){for(int i=(nextPlace-1)/2;i>0;--i){heapAdjust(nodes,i,nextPlace-1);}}}运行结果:3.用队列式分支限界法解决0-1背包问题:代码:#include<stdio.h>#include<stdlib.h>#define MAXNUM 100struct node{int step;double price;double weight;double max, min;unsigned long po;};typedef struct node DataType;struct SeqQueue{ /* 顺序队列类型定义 */int f, r;DataType q[MAXNUM];};typedef struct SeqQueue *PSeqQueue;PSeqQueue createEmptyQueue_seq( void ){PSeqQueue paqu;paqu = (PSeqQueue)malloc(sizeof(struct SeqQueue));if (paqu == NULL)printf("Out of space!! \n");elsepaqu->f = paqu->r = 0;return paqu;}int isEmptyQueue_seq( PSeqQueue paqu ){return paqu->f == paqu->r;}/* 在队列中插入一元素x */void enQueue_seq( PSeqQueue paqu, DataType x ) {if((paqu->r + 1) % MAXNUM == paqu->f)printf( "Full queue.\n" );else{paqu->q[paqu->r] = x;paqu->r = (paqu->r + 1) % MAXNUM;}}/* 删除队列头元素 */void deQueue_seq( PSeqQueue paqu ){if( paqu->f == paqu->r )printf( "Empty Queue.\n" );elsepaqu->f = (paqu->f + 1) % MAXNUM; }/* 对非空队列,求队列头部元素 */DataType frontQueue_seq( PSeqQueue paqu ){return (paqu->q[paqu->f]);}/* 物品按性价比从新排序*/void sort(int n, double p[], double w[]){int i, j;for (i = 0; i < n-1; i++)for (j = i; j < n-1; j++){double a = p[j]/w[j];double b = p[j+1]/w[j+1];if (a < b){double temp = p[j];p[j] = p[j+1];p[j+1] = temp;temp = w[j];w[j] = w[j+1];w[j+1] = temp;}}}/* 求最大可能值*/double up(int k, double m, int n, double p[], double w[]) {int i = k;double s = 0;while (i < n && w[i] < m){m -= w[i];s += p[i];i++;}if (i < n && m > 0){s += p[i] * m / w[i];i++;}return s;}/* 求最小可能值*/double down(int k, double m, int n, double p[], double w[]) {int i = k;double s = 0;while (i < n && w[i] <= m){m -= w[i];s += p[i];i++;}return s;}/* 用队列实现分支定界算法*/double solve(double m, int n, double p[], double w[], unsigned long* po) {double min;PSeqQueue q = createEmptyQueue_seq();DataType x = {0,0,0,0,0,0};sort(n, p, w);x.max = up(0, m, n, p, w);x.min = min = down(0, m, n, p, w);if (min == 0) return -1;enQueue_seq(q, x);while (!isEmptyQueue_seq(q)){int step;DataType y;x = frontQueue_seq(q);deQueue_seq(q);if (x.max < min) continue;step = x.step + 1;if (step == n+1) continue;y.max = x.price + up(step, m - x.weight, n, p, w);if (y.max >= min){y.min = x.price + down(step, m-x.weight, n, p, w); y.price = x.price;y.weight = x.weight;y.step = step;y.po = x.po << 1;if (y.min >= min){min = y.min;if (step == n) *po = y.po;}enQueue_seq(q, y);}if (x.weight+w[step-1]<= m){y.max = x.price + p[step-1]+up(step, m-x.weight-w[step-1], n, p, w);if (y.max >= min) {y.min = x.price + p[step-1] +down(step, m-x.weight-w[step-1], n, p, w); y.price = x.price + p[step-1];y.weight = x.weight + w[step-1];y.step = step;y.po = (x.po << 1) + 1;if (y.min >= min){min = y.min;if (step == n) *po = y.po;}enQueue_seq(q, y);}}}return min;}#define n 4double m = 7;double p[n] = {9, 10, 7, 4};double w[n] = {3, 5, 1, 2};int main(){int i;double d;unsigned long po;d = solve(m, n, p, w, &po);if (d == -1)printf("No solution!\n");else{for (i = 0; i < n; i++)printf("x%d 为 %d\n", i + 1, ((po & (1<<(n-i-1))) != 0)); printf("最优值是:%f\n", d);}getchar();return 0;}运行结果:。
优先队列式分支限界法求解0-1背包问题
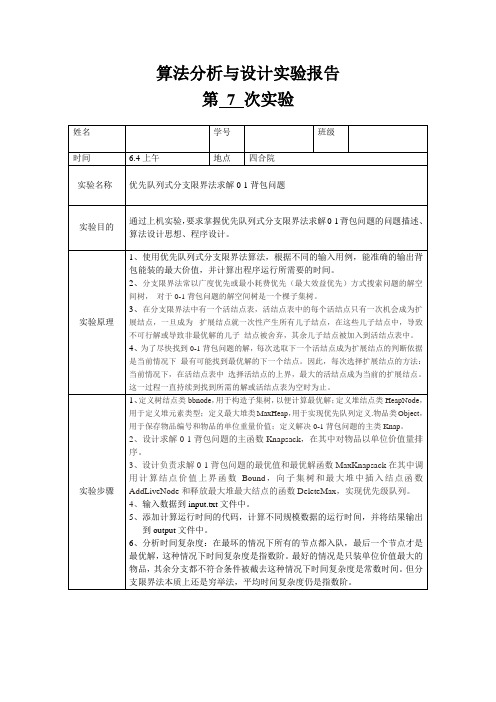
算法分析与设计实验报告第7 次实验}1、测试自己输入的小规模数据2、测试随机生成1003、随机生成1000数据4、随机生成1000数据附录:完整代码#include <iostream>#include<time.h>#include<algorithm>#include<fstream>using namespace std;ifstream in("input.txt");ofstream out("output.txt");typedef int Typew;typedef int Typep;//物品类class Object{friend Typep Knapsack(Typew *, Typep *, Typew, int, int *); public:int operator <= (Object a) const{return (d >= a.d);}private:int ID; //物品编号float d; //单位重量价值};//树结点类class bbnode{friend class Knap;friend Typep Knapsack(Typew *, Typep *, Typew, int, int *); private:bbnode *parent; //指向父节点的指针int LChild;};//堆结点类class HeapNode{friend class Knap;friend class MaxHeap;public:operator Typep()const{return uprofit;};private:Typep uprofit, //结点的价值上界profit; //结点所相应的价值Typew weight; //结点所相应的重量int level; //活结点在子集树中所处的层序号bbnode *elemPtr; //指向该活结点在子集树中相应结点的指针};//最大堆类class MaxHeap{public:MaxHeap(int maxElem){HeapElem = new HeapNode* [maxElem+1]; //下标为0的保留capacity = maxElem;size = 0;}void InsertMax(HeapNode *newNode);HeapNode DeleteMax(HeapNode* &N);private:int capacity;int size;HeapNode **HeapElem;};//0-1背包问题的主类class Knap{friend Typep Knapsack(Typew *, Typep *, Typew, int, int *); public:Typep MaxKnapsack();private:MaxHeap *H;Typep Bound(int i);void AddLiveNode(Typep up, Typep cp, Typew cw, int ch, int level);bbnode *E; //指向扩展结点的指针Typew c; //背包容量int n; //物品总数Typew *w; //物品重量数组(以单位重量价值降序)Typep *p; //物品价值数组(以单位重量价值降序)Typew cw; //当前装包重量Typep cp; //当前装包价值int *bestx; //最优解};void MaxHeap::InsertMax(HeapNode *newNode){int i = 1;for (i = ++size; i/2 > 0 && HeapElem[i/2]->uprofit < newNode->uprofit; i /= 2){HeapElem[i] = HeapElem[i/2];}HeapElem[i] = newNode;}HeapNode MaxHeap::DeleteMax(HeapNode *&N){if(size >0 ){N = HeapElem[1];int i = 1;while(i < size){if(((i*2 +1) <= size) && HeapElem[i*2]->uprofit > HeapElem[i*2 +1]->uprofit){HeapElem[i] = HeapElem[i*2];i = i*2;}else{if(i*2 <= size){HeapElem[i] = HeapElem[i*2];i = i*2;}elsebreak;}}if(i < size)HeapElem[i] = HeapElem[size];}size--;return *N;}Typep Knap::MaxKnapsack(){H = new MaxHeap(10000);bestx = new int [n+1];int i = 1;E = 0;cw = 0;cp = 0;Typep bestp = 0;Typep up = Bound(1);while (i != n+1){Typew wt = cw + w[i];if(wt <= c) {if(cp + p[i] > bestp)bestp = cp + p[i];AddLiveNode(up, cp + p[i], cw + w[i], 1, i);}up = Bound(i + 1);if(up >= bestp)AddLiveNode(up, cp, cw, 0, i);HeapNode* N;H->DeleteMax(N);E = N->elemPtr;cw = N->weight;cp = N->profit;up = N->uprofit;i = N->level + 1;}for (int i = n; i > 0; i--){bestx[i] = E->LChild;E = E->parent;}return cp;}Typep Knap::Bound(int i){Typew cleft = c - cw;Typep b = cp;while (i<=n && w[i] <= cleft){cleft -= w[i];b += p[i];i++;}if(i<=n) b += p[i]/w[i] * cleft;return b;}void Knap::AddLiveNode(Typep up, Typep cp, Typew cw, int ch, int level) {bbnode *b=new bbnode;b->parent=E;b->LChild=ch;HeapNode *N = new HeapNode;N->uprofit=up;N->profit=cp;N->weight=cw;N->level=level;N->elemPtr=b;H->InsertMax(N);}//Knapsack返回最大价值,最优值保存在bestxTypep Knapsack(Typew *w, Typep *p, Typew c, int n, int *bestx){Typew W = 0;Typep P = 0;Object *Q = new Object[n];for(int i =1; i<=n; i++){Q[i-1].ID = i;Q[i-1].d = 1.0*p[i]/w[i];P += p[i];W += w[i];}if (W <= c){for(int i =1; i<=n; i++){bestx[i] = p[i];}return P;}for(int i = 1; i<n; i++)for(int j = 1; j<= n-i; j++){if(Q[j-1].d < Q[j].d){Object temp = Q[j-1];Q[j-1] = Q[j];Q[j] = temp;}}Knap K;K.p = new Typep [n+1];K.w = new Typew [n+1];for(int i = 1; i<=n; i++){K.p[i] = p[Q[i-1].ID];K.w[i] = w[Q[i-1].ID];}K.cp = 0;K.cw = 0;K.c = c;K.n = n;Typep bestp = K.MaxKnapsack();for(int i = 1; i<=n; i++){bestx[Q[i-1].ID] = K.bestx[i];}delete [] Q;delete [] K.w;delete [] K.p;delete [] K.bestx;delete [] K.H;return bestp;}int main(){cout<<"请在input.txt文件中输入物品数量、背包容量"<<endl;int N ;in>>N;Typew c; //背包容量in>>c;int bestx[N+1]; //最优解int bestp; //最优值Typep p[N+1];//物品价值Typew w[N+1];//物品重量cout<<"在input.txt文件中读取的物品总数N = "<< N<<",背包容量C = "<< c<<endl; cout<<"请选择生成数据的规模大小:200请输入1,2000请输入2,20000请输入3"<<endl; int x;cin>>x;if(x==1){ofstream in1("input1.txt");srand(time(NULL));int n=200;int *a=new int[n];for(int i=0;i<n;i++){a[i]=rand()%91;in1<<a[i]<<" ";}cout<<"随机数已请生成到input1文件中,请将数据添加到input.txt文件中"<<endl; }else if(x==2){ofstream in1("input1.txt");srand(time(NULL));int n=2000;int *a=new int[n];for(int i=0;i<n;i++){a[i]=rand()%91;in1<<a[i]<<" ";}cout<<"随机数已请生成到input1文件中,请将数据添加到input.txt文件中"<<endl; }else if(x==3){ofstream in1("input1.txt");srand(time(NULL));int n=20000;int *a=new int[n];for(int i=0;i<n;i++){a[i]=rand()%91;in1<<a[i]<<" ";}cout<<"随机数已请生成到input1文件中,请将数据添加到input.txt文件中"<<endl;}cout<<"添加完毕后请输入1"<<endl;int m;cin>>m;clock_t start,finish;start=clock();for (int i = 1; i <= N; i++){in>>w[i];}for (int i = 1; i <= N; i++){in>>p[i];}cout<<"已在input文件中读取物品重量和价值。
分支限界法解决01背包问题
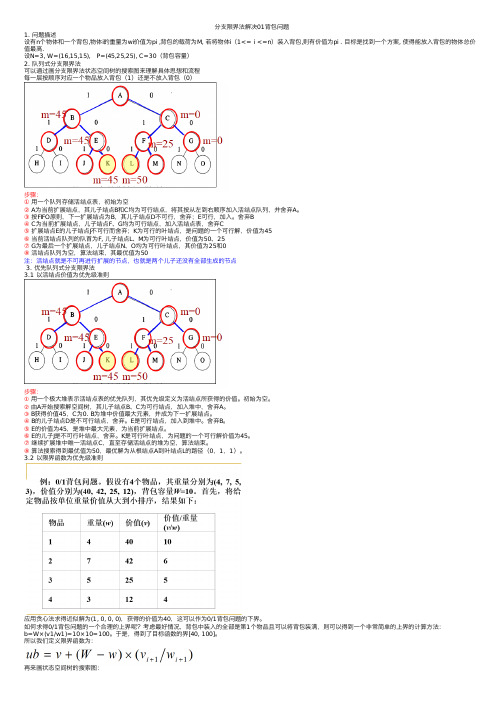
分⽀限界法解决01背包问题1. 问题描述设有n个物体和⼀个背包,物体i的重量为wi价值为pi ,背包的载荷为M, 若将物体i(1<= i <=n)装⼊背包,则有价值为pi . ⽬标是找到⼀个⽅案, 使得能放⼊背包的物体总价值最⾼.设N=3, W=(16,15,15), P=(45,25,25), C=30(背包容量)2. 队列式分⽀限界法可以通过画分⽀限界法状态空间树的搜索图来理解具体思想和流程每⼀层按顺序对应⼀个物品放⼊背包(1)还是不放⼊背包(0)步骤:①⽤⼀个队列存储活结点表,初始为空② A为当前扩展结点,其⼉⼦结点B和C均为可⾏结点,将其按从左到右顺序加⼊活结点队列,并舍弃A。
③按FIFO原则,下⼀扩展结点为B,其⼉⼦结点D不可⾏,舍弃;E可⾏,加⼊。
舍弃B④ C为当前扩展结点,⼉⼦结点F、G均为可⾏结点,加⼊活结点表,舍弃C⑤扩展结点E的⼉⼦结点J不可⾏⽽舍弃;K为可⾏的叶结点,是问题的⼀个可⾏解,价值为45⑥当前活结点队列的队⾸为F, ⼉⼦结点L、M为可⾏叶结点,价值为50、25⑦ G为最后⼀个扩展结点,⼉⼦结点N、O均为可⾏叶结点,其价值为25和0⑧活结点队列为空,算法结束,其最优值为50注:活结点就是不可再进⾏扩展的节点,也就是两个⼉⼦还没有全部⽣成的节点3. 优先队列式分⽀限界法3.1 以活结点价值为优先级准则步骤:①⽤⼀个极⼤堆表⽰活结点表的优先队列,其优先级定义为活结点所获得的价值。
初始为空。
②由A开始搜索解空间树,其⼉⼦结点B、C为可⾏结点,加⼊堆中,舍弃A。
③B获得价值45,C为0. B为堆中价值最⼤元素,并成为下⼀扩展结点。
④ B的⼉⼦结点D是不可⾏结点,舍弃。
E是可⾏结点,加⼊到堆中。
舍弃B。
⑤ E的价值为45,是堆中最⼤元素,为当前扩展结点。
⑥ E的⼉⼦J是不可⾏叶结点,舍弃。
K是可⾏叶结点,为问题的⼀个可⾏解价值为45。
⑦继续扩展堆中唯⼀活结点C,直⾄存储活结点的堆为空,算法结束。
回溯法、分支限界法解0-1背包问题(计算机算法设计与分析实验报告)
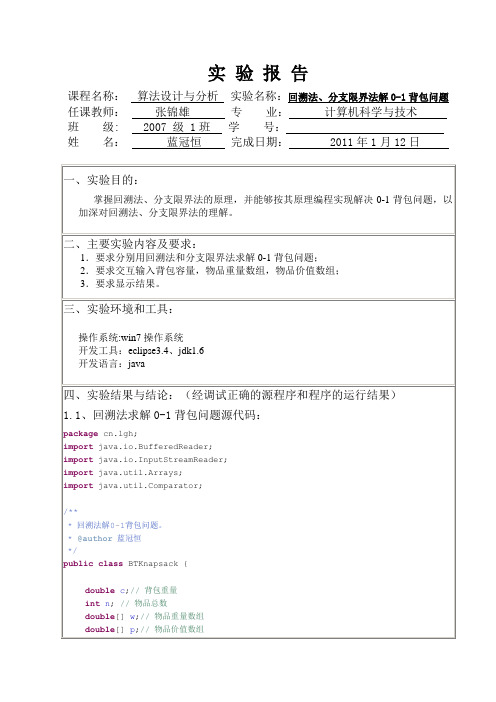
inti = 1;
doublebestp = 0.0;
doubleup = bound(1);
while(i !=n+ 1) {
doublewt =cw+w[i];
//检查当前扩展节点的左儿子节点
if(wt <=c) {
if(cp+p[i] > bestp) {
}
do{
System.out.println("请输入背包的容量:");
input = in.readLine().trim();
input = in.readLine().replaceAll(" ","");
}while(input.equals(""));
if(input.equals("2")){
w=newdouble[n+ 1];
for(inti = 1; i <=n; i++) {
p[i] = pp[q[i - 1].id- 1];
w[i] = ww[q[i - 1].id- 1];
}
backtrack(1);
returnbestp;
}
//回溯过程
privatevoidbacktrack(inti) {
c= cc;
n= pp.length;
Element[] q =newElement[n];
doublews = 0.0;
doubleps = 0.0;
for(inti = 0; i <n; i++) {
q[i] =newElement(i + 1, pp[i] / ww[i]);
最新实验 4 用分支限界法实现0-1背包问题
实验四用分支限界法实现0-1背包问题一.实验目的1.熟悉分支限界法的基本原理。
2.通过本次实验加深对分支限界法的理解。
二.实验内容及要求内容:.给定n种物品和一个背包。
物品i的重量是w,其价值为v,背包容量为c。
问应该如何选择装入背包的物品,使得装入背包中物品的总价值最大?要求:使用优先队列式分支限界法算法编程,求解0-1背包问题三.程序列表#include<iostream>#include<stack>using namespace std;#define N 100class HeapNode//定义HeapNode结点类{public:double upper, price, weight; //upper为结点的价值上界,price是结点所对应的价值,weight 为结点所相应的重量int level, x[N]; //活节点在子集树中所处的层序号};double MaxBound(int i);double Knap();void AddLiveNode(double up, double cp, double cw, bool ch, int level);//up是价值上界,cp是相应的价值,cw是该结点所相应的重量,ch是ture or falsestack<HeapNode> High; //最大队Highdouble w[N], p[N]; //把物品重量和价值定义为双精度浮点数double cw, cp, c; //cw为当前重量,cp为当前价值,定义背包容量为cint n; //货物数量为int main(){cout <<"请输入背包容量:"<< endl;cin >> c;cout <<"请输入物品的个数:"<< endl;cin >> n;cout <<"请按顺序分别输入物品的重量:"<< endl;int i;for (i = 1; i <= n; i++)cin >> w[i]; //输入物品的重量cout <<"请按顺序分别输入物品的价值:"<< endl;for (i = 1; i <= n; i++)cin >> p[i]; //输入物品的价值cout <<"最优值为:";cout << Knap() << endl; //调用knap函数输出最大价值return 0;}double MaxBound(int k) //MaxBound函数求最大上界{double cleft = c - cw; //剩余容量double b = cp; //价值上界while (k <= n&&w[k] <= cleft) //以物品单位重量价值递减装填剩余容量{cleft -= w[k];b += p[k];k++;}if (k <= n)b += p[k] / w[k] * cleft; //装填剩余容量装满背包return b;}void AddLiveNode(double up, double cp, double cw, bool ch, int lev) //将一个新的活结点插入到子集数和最大堆High中{HeapNode be;be.upper = up;be.price = cp;be.weight = cw;be.level = lev;if (lev <= n)High.push(be);}//调用stack头文件的push函数 }double Knap() //优先队列分支限界法,返回最大价值,bestx返回最优解{int i = 1;cw = cp = 0;double bestp = 0; //best为当前最优值double up = MaxBound(1);//价值上界//搜索子集空间树while (1) //非叶子结点{double wt = cw + w[i];if (wt <= c) //左儿子结点为可行结点{if (cp + p[i]>bestp)bestp = cp + p[i];AddLiveNode(up, cp + p[i], cw + w[i], true, i + 1);}up = MaxBound(i + 1);if (up >= bestp) //右子数可能含最优解AddLiveNode(up, cp, cw, false, i + 1);if (High.empty())return bestp;HeapNode node = High.top(); //取下一扩展结点High.pop();cw = node.weight;cp = node.price;up = node.upper;i = node.level;}}四.实验结果酒店服务员年度工作汇报20xx年是自我挑战的一年,我将努力改正过去一年工作中的不足,把新一年的工作做好,过去的一年在领导的关心和同事的热情帮助,通过自身的不懈努力,在工作上取得了一定的成果,现将工作总结如下。
分支限界法解决01背包问题
分⽀限界法解决01背包问题 分⽀限界法和之前讲的回溯法有⼀点相似,两者都是在问题的解的空间上搜索问题的解。
但是两者还是有⼀些区别的,回溯法是求解在解的空间中的满⾜的所有解,分⽀限界法则是求解⼀个最⼤解或最⼩解。
这样,两者在解这⼀⽅⾯还是有⼀些不同的。
之前回溯法讲了N后问题,这个问题也是对于这有多个解,但是今天讲的01背包问题是只有⼀个解的。
下⾯就讲讲分⽀限界法的基本思想。
分⽀限界法常以⼴度优先或以最⼩消耗(最⼤效益)优先的⽅式搜索问题的解空间树。
问题的解空间树是表⽰问题解空间的⼀颗有序树,常见的有⼦集树和排列树。
分⽀限界法和回溯法的区别还有⼀点,它们对于当前扩展结点所采⽤的扩展⽅式也是不相同的。
分⽀限界法中,对于每⼀个活结点只有⼀次机会成为扩展结点。
活结点⼀旦成为了扩展结点,就⼀次性产⽣其所有的⼦结点,⼦结点中,不符合要求的和⾮最优解的⼦结点将会被舍弃,剩下的⼦结点将加⼊到活结点表中。
再重复上⾯的过程,直到没有活结点表中没有结点,⾄此完成解决问题的⽬的。
分⽀限界法⼤致的思想就是上⾯的叙述,现在就可以发现,对于结点的扩展将会成为分⽀限界法的主要核⼼。
所以,分⽀限界法常见的有两种扩展结点的⽅式,1.队列式(FIFO)分⽀限界法,2.优先队列式分⽀限界法。
两种⽅法的区别就是对于活结点表中的取出结点的⽅式不同,第⼀种⽅法是先进先出的⽅式,第⼆种是按优先级取出结点的⽅式。
两中⽅法的区别下⾯也会提到。
在背包问题中还会提到⼀个⼦树上界的概念,其实就是回溯法中的剪枝函数,只不过,分⽀限界法⾥的剪枝函数改进了⼀些,剪枝函数同样也是分⽀限界法⾥⽐较重要的东西。
下⾯就讲⼀讲01背包问题的实现。
01背包问题和前⾯讲的背包问题的区别不⼤,就是01背包问题的物品不可以只放⼊部分,01背包问题的物品只能放⼊和不放⼊两个选择,这也是名字中01的原因。
其他的和背包问题相差不⼤,这⾥也不再累述。
算法的主体是⽐较容易想的,⾸先,将数据进⾏处理,这也是上⾯讲到的第⼆种取结点的⽅式(优先队列式)。
分支限界法0-1背包问题-队列式
分⽀限界法0-1背包问题-队列式⼀.分⽀限界法概述(1)分⽀限界法就是采⽤⼴度优先的策略,依次搜索活结点所有的分枝,也就额是所有的相邻结点。
在求最优解时采⽤⼀个限界函数,计算限界函数值,选择⼀个最有利的⼦节点作为扩展结点,使搜索树朝着解空间树上有最优解的分⽀推进,以便尽快找出⼀个最优解。
(2)常见的两种分⽀限界法 先进先出(FIFO)队列式:在先进先出的分⽀限界法中,⽤队列作为组织活结点表的数据结构,并按照队列先进先出的原则选择结点作为扩展结点。
优先队列(PQ):⽤优先队列作为组织活结点表的数据结构。
⼆.0-1背包问题问题:给定n种物品和⼀背包。
物品i的重量是wi,其价值为pi,背包的容量为C。
问应如何选择装⼊背包的物品,使得装⼊背包中物品的总价值最⼤?#include<iostream>#include<queue>using namespace std;const int maxn=99;int n,c;int w[maxn];int v[maxn];int bestv=0;int bestx[maxn];int total=1; //解空间中的节点数累计,全局变量struct nodetype //队列中的结点类型{int no; //结点编号,从1开始int i; //当前结点在搜索空间中的层次int w; //当前结点的总重量int v; //当前结点的总价值int x[maxn]; //当前结点包含的解向量double ub; //上界};void input(){cout<<"请输⼊物品的个数:"<<endl;cin>>n;cout<<"请输⼊每个物品的重量及价值(如5 4):"<<endl;for(int i = 1; i <= n; i++){cin>>w[i]>>v[i];}cout<<"请输⼊背包的容量:"<<endl;cin>>c;}void bound(nodetype &e) //计算分⽀结点e的上界{int i=e.i+1; //考虑结点e的余下物品int sumw=e.w;double sumv=e.v;while((sumw+w[i]<=c)&&i<=n){sumw+=w[i];sumv+=v[i];i++;}if(i<=n) //余下物品只能部分装⼊e.ub=sumv+(c-sumw)*v[i]/w[i];else e.ub=sumv;}void enqueue(nodetype e,queue<nodetype> &qu)//结点e进队qu{if(e.i==n) //到达叶⼦节点,不在扩展对应⼀个解{if(e.v>bestv) //找到更⼤价值的解{bestv=e.v;for(int j=1;j<=n;j++)bestx[j]=e.x[j];}}else qu.push(e); //⾮叶⼦结点进队}void bfs(){int j;nodetype e,e1,e2;queue<nodetype> qu;e.i=0;e.w=0;e.v=0;e.no=total++;for(j=1;j<=n;j++)e.x[j]=0;bound(e);qu.push(e);while(!qu.empty()){e=qu.front();qu.pop(); //出队结点eif(e.w+w[e.i+1]<=c) //剪枝,检查左孩⼦结点{e1.no=total++; //建⽴左孩⼦结点e1.i=e.i+1;e1.w=e.w+w[e1.i];e1.v=e.v+v[e1.i];for(j=1;j<=n;j++)e1.x[j]=e.x[j];e1.x[e1.i]=1;bound(e1); //求左孩⼦的上界enqueue(e1,qu); //左孩⼦结点进队}e2.no=total++;e2.i=e.i+1;e2.w=e.w;e2.v=e.v;for(j=1;j<=n;j++)e2.x[j]=e.x[j];e2.x[e2.i]=0;bound(e2);if(e2.ub>bestv) //若右孩⼦结点可⾏,则进队,否则被剪枝 enqueue(e2,qu);}}void output(){cout<<"最优值是:"<<bestv<<endl;cout<<"(";for(int i=1;i<=n;i++)cout<<bestx[i]<<"";cout<<")";}int main(){input();bfs();output();return0;}。
用分支限界求解0-1背包问题
实验题目:用分支限界求解0-1背包问题物品个数n=4,背包容量c=7,价值向量p={9,10,7,4},重量向量w={3,5,2,1}请求出最优的解及其目标函数值。
#include <stdio.h>#include<malloc.h>#defineMaxSize 100//最多结点数typedefstructQNode{floatweight;floatvalue;intceng;structQNode *parent;bool leftChild;}QNode,*qnode;//存放每个结点typedef struct{qnodeQ[MaxSize];int front,rear;}SqQueue;//存放结点的队列SqQueuesq;float bestv=0; //最优解int n=0;//实际物品数float w[MaxSize];//物品的重量float v[MaxSize];//物品的价值int bestx[MaxSize];//存放最优解qnode bestE;void InitQueue(SqQueue &sq ) //队列初始化{}sq.front=1;sq.rear=1;bool QueueEmpty(SqQueue sq) //队列是否为空{}void EnQueue(SqQueue &sq,qnode b)//入队{}qnode DeQueue(SqQueue &sq)//出队{qnode e;if(sq.front==sq.rear){}e=sq.Q[sq.front];printf("队列已空!");return 0;if(sq.front==(sq.rear+1)%MaxSize){}sq.Q[sq.rear]=b;sq.rear=(sq.rear+1)%MaxSize;printf("队列已满!");return ;if(sq.front==sq.rear)return true;elsereturn false;}sq.front=(sq.front+1)%MaxSize;return e;voidEnQueue1(float wt,float vt, int i ,QNode *parent, bool leftchild) {}voidmaxLoading(float w[],float v[],int c){float wt=0;float vt=0;int i=1;//当前的扩展结点所在的层qnode b;if (i==n)//可行叶子结点{}b=(qnode)malloc(sizeof(QNode));//非叶子结点b->weight=wt;b->value=vt;b->ceng=i;b->parent=parent;b->leftChild=leftchild;EnQueue(sq,b);if (vt==bestv){}return;bestE=parent;bestx[n]=(leftchild)?1:0;float ew=0;//扩展节点所相应的当前载重量float ev=0;//扩展结点所相应的价值qnode e=NULL;qnode t=NULL;InitQueue(sq);EnQueue(sq,t);//空标志进队列while (!QueueEmpty(sq)){}printf("最优取法为:\n");wt=ew+w[i];vt=ev+v[i];if (wt <= c){if(vt>bestv)bestv=vt;EnQueue1(wt,vt,i,e,true);//左儿子结点进队列}EnQueue1(ew,ev,i,e,false);//右儿子总是可行;e=DeQueue(sq);//取下一扩展结点if (e == NULL){}ew=e->weight;//更新当前扩展结点的值ev=e->value;if (QueueEmpty(sq))break; EnQueue(sq,NULL);//同层结点尾部标志e=DeQueue(sq);//取下一扩展结点i++;for( int j=n-1;j>0;j--)//构造最优解{}for(int k=1;k<=n;k++){}printf("\n");printf("最优价值为:%.1f\n\n",bestv);if(bestx[k]==1)printf("\n物品%d:重量:%.1f,价值:%.1f\n",k,w[k],v[k]);bestx[j]=(bestE->leftChild?1:0);bestE=bestE->parent;}void main(){int c;float ewv[MaxSize];printf("510专区\n");printf("请输入物品的数量:\n");scanf("%d",&n);printf("请输入背包容量:\n");scanf("%d",&c);printf("\n请输入物品价值和重量:\n\n"); for(int i=1;i<=n;i++){printf("物品%d:",i);scanf("%f%f",&ewv[i],&w[i]);v[i]=w[i]*ewv[i];printf("\n");}}实验结果:maxLoading(w, v, c);。
- 1、下载文档前请自行甄别文档内容的完整性,平台不提供额外的编辑、内容补充、找答案等附加服务。
- 2、"仅部分预览"的文档,不可在线预览部分如存在完整性等问题,可反馈申请退款(可完整预览的文档不适用该条件!)。
- 3、如文档侵犯您的权益,请联系客服反馈,我们会尽快为您处理(人工客服工作时间:9:00-18:30)。
算法分析与设计实验报告第7 次实验}1、测试自己输入的小规模数据2、测试随机生成1003、随机生成1000数据4、随机生成1000数据附录:完整代码#include <iostream>#include<time.h>#include<algorithm>#include<fstream>using namespace std;ifstream in("input.txt");ofstream out("output.txt");typedef int Typew;typedef int Typep;//物品类class Object{friend Typep Knapsack(Typew *, Typep *, Typew, int, int *); public:int operator <= (Object a) const{return (d >= a.d);}private:int ID; //物品编号float d; //单位重量价值};//树结点类class bbnode{friend class Knap;friend Typep Knapsack(Typew *, Typep *, Typew, int, int *); private:bbnode *parent; //指向父节点的指针int LChild;};//堆结点类class HeapNode{friend class Knap;friend class MaxHeap;public:operator Typep()const{return uprofit;};private:Typep uprofit, //结点的价值上界profit; //结点所相应的价值Typew weight; //结点所相应的重量int level; //活结点在子集树中所处的层序号bbnode *elemPtr; //指向该活结点在子集树中相应结点的指针};//最大堆类class MaxHeap{public:MaxHeap(int maxElem){HeapElem = new HeapNode* [maxElem+1]; //下标为0的保留capacity = maxElem;size = 0;}void InsertMax(HeapNode *newNode);HeapNode DeleteMax(HeapNode* &N);private:int capacity;int size;HeapNode **HeapElem;};//0-1背包问题的主类class Knap{friend Typep Knapsack(Typew *, Typep *, Typew, int, int *); public:Typep MaxKnapsack();private:MaxHeap *H;Typep Bound(int i);void AddLiveNode(Typep up, Typep cp, Typew cw, int ch, int level);bbnode *E; //指向扩展结点的指针Typew c; //背包容量int n; //物品总数Typew *w; //物品重量数组(以单位重量价值降序)Typep *p; //物品价值数组(以单位重量价值降序)Typew cw; //当前装包重量Typep cp; //当前装包价值int *bestx; //最优解};void MaxHeap::InsertMax(HeapNode *newNode){int i = 1;for (i = ++size; i/2 > 0 && HeapElem[i/2]->uprofit < newNode->uprofit; i /= 2){HeapElem[i] = HeapElem[i/2];}HeapElem[i] = newNode;}HeapNode MaxHeap::DeleteMax(HeapNode *&N){if(size >0 ){N = HeapElem[1];int i = 1;while(i < size){if(((i*2 +1) <= size) && HeapElem[i*2]->uprofit > HeapElem[i*2 +1]->uprofit){HeapElem[i] = HeapElem[i*2];i = i*2;}else{if(i*2 <= size){HeapElem[i] = HeapElem[i*2];i = i*2;}elsebreak;}}if(i < size)HeapElem[i] = HeapElem[size];}size--;return *N;}Typep Knap::MaxKnapsack(){H = new MaxHeap(10000);bestx = new int [n+1];int i = 1;E = 0;cw = 0;cp = 0;Typep bestp = 0;Typep up = Bound(1);while (i != n+1){Typew wt = cw + w[i];if(wt <= c) {if(cp + p[i] > bestp)bestp = cp + p[i];AddLiveNode(up, cp + p[i], cw + w[i], 1, i);}up = Bound(i + 1);if(up >= bestp)AddLiveNode(up, cp, cw, 0, i);HeapNode* N;H->DeleteMax(N);E = N->elemPtr;cw = N->weight;cp = N->profit;up = N->uprofit;i = N->level + 1;}for (int i = n; i > 0; i--){bestx[i] = E->LChild;E = E->parent;}return cp;}Typep Knap::Bound(int i){Typew cleft = c - cw;Typep b = cp;while (i<=n && w[i] <= cleft){cleft -= w[i];b += p[i];i++;}if(i<=n) b += p[i]/w[i] * cleft;return b;}void Knap::AddLiveNode(Typep up, Typep cp, Typew cw, int ch, int level) {bbnode *b=new bbnode;b->parent=E;b->LChild=ch;HeapNode *N = new HeapNode;N->uprofit=up;N->profit=cp;N->weight=cw;N->level=level;N->elemPtr=b;H->InsertMax(N);}//Knapsack返回最大价值,最优值保存在bestxTypep Knapsack(Typew *w, Typep *p, Typew c, int n, int *bestx){Typew W = 0;Typep P = 0;Object *Q = new Object[n];for(int i =1; i<=n; i++){Q[i-1].ID = i;Q[i-1].d = 1.0*p[i]/w[i];P += p[i];W += w[i];}if (W <= c){for(int i =1; i<=n; i++){bestx[i] = p[i];}return P;}for(int i = 1; i<n; i++)for(int j = 1; j<= n-i; j++){if(Q[j-1].d < Q[j].d){Object temp = Q[j-1];Q[j-1] = Q[j];Q[j] = temp;}}Knap K;K.p = new Typep [n+1];K.w = new Typew [n+1];for(int i = 1; i<=n; i++){K.p[i] = p[Q[i-1].ID];K.w[i] = w[Q[i-1].ID];}K.cp = 0;K.cw = 0;K.c = c;K.n = n;Typep bestp = K.MaxKnapsack();for(int i = 1; i<=n; i++){bestx[Q[i-1].ID] = K.bestx[i];}delete [] Q;delete [] K.w;delete [] K.p;delete [] K.bestx;delete [] K.H;return bestp;}int main(){cout<<"请在input.txt文件中输入物品数量、背包容量"<<endl;int N ;in>>N;Typew c; //背包容量in>>c;int bestx[N+1]; //最优解int bestp; //最优值Typep p[N+1];//物品价值Typew w[N+1];//物品重量cout<<"在input.txt文件中读取的物品总数N = "<< N<<",背包容量C = "<< c<<endl; cout<<"请选择生成数据的规模大小:200请输入1,2000请输入2,20000请输入3"<<endl; int x;cin>>x;if(x==1){ofstream in1("input1.txt");srand(time(NULL));int n=200;int *a=new int[n];for(int i=0;i<n;i++){a[i]=rand()%91;in1<<a[i]<<" ";}cout<<"随机数已请生成到input1文件中,请将数据添加到input.txt文件中"<<endl; }else if(x==2){ofstream in1("input1.txt");srand(time(NULL));int n=2000;int *a=new int[n];for(int i=0;i<n;i++){a[i]=rand()%91;in1<<a[i]<<" ";}cout<<"随机数已请生成到input1文件中,请将数据添加到input.txt文件中"<<endl; }else if(x==3){ofstream in1("input1.txt");srand(time(NULL));int n=20000;int *a=new int[n];for(int i=0;i<n;i++){a[i]=rand()%91;in1<<a[i]<<" ";}cout<<"随机数已请生成到input1文件中,请将数据添加到input.txt文件中"<<endl;}cout<<"添加完毕后请输入1"<<endl;int m;cin>>m;clock_t start,finish;start=clock();for (int i = 1; i <= N; i++){in>>w[i];}for (int i = 1; i <= N; i++){in>>p[i];}cout<<"已在input文件中读取物品重量和价值。