固定效应的分位数回归估计--经典
固定效应模型的估计原理说明

固定效应模型的估计原理说明
固定效应模型是一种用于估计面板数据(panel data)中个体特征不变的情况下,解释变量对于因变量的影响的经济计量模型。
在固定效应模型中,个体固定效应被视为截距,并且通过引入虚拟变量来捕捉个体间的差异。
在固定效应模型中,变量变为:
Yit = αi + βXit + εit
其中,Yit是个体i在时间t上的因变量观测值,αi是个体i的固定效应(个体固定截距),Xit是个体i在时间t上的解释变量观测值,β是解释变量的系数,εit是误差项。
个体固定效应αi代表个体固有的特征,例如个体的个体动态特征、管理水平或其他个体特征,它们在观测期间保持不变。
为了对个体固定效应进行估计,我们需要引入个体虚拟变量。
个体虚拟变量是一个二进制变量,以个体为单位,并且在个体i上为1,否则为0。
这些变量的引入可以控制个体固有的效应,消除个体之间的异质性。
建议至少引入N-1个个体虚拟变量(N是个体的数量),以避免陷入虚拟变量陷阱。
在固定效应模型中,我们做出了一些假设:(1)解释变量是不随时间而变化的;(2)个体固定效应是不随时间而变化的;(3)解释变量和个体固定效应之间不存在相关性。
为了估计固定效应模型,可以使用最小二乘法(OLS)估计。
OLS估计首先对每个个体的回归方程进行估计,然后将结果进行汇总。
由于引入了个体固定效应,固定效应模型具有更多的解释力和统计效率。
总之,固定效应模型的估计原理是通过引入个体虚拟变量来捕捉个体之间的异质性,并控制个体固有的特征,从而解释解释变量对因变量的影响。
固定效应模型可以提供更准确、有效的估计结果,并且可以避免个体异质性带来的偏误。
分位数回归方法及应用PPT18页
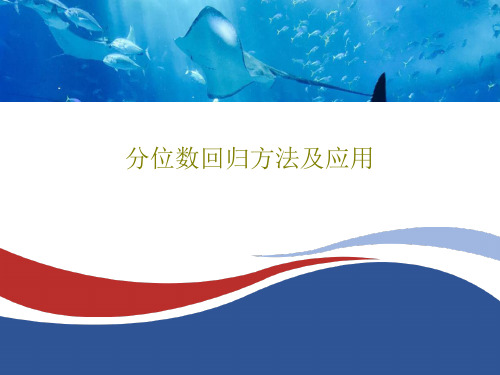
▪
26、要使整个人生都过得舒适、愉快,这是不可能的,因为人类必须具备一种能应付逆境的态度。——卢梭
▪
Байду номын сангаас
27、只有把抱怨环境的心情,化为上进的力量,才是成功的保证。——罗曼·罗兰
▪
28、知之者不如好之者,好之者不如乐之者。——孔子
▪
29、勇猛、大胆和坚定的决心能够抵得上武器的精良。——达·芬奇
▪
30、意志是一个强壮的盲人,倚靠在明眼的跛子肩上。——叔本华
谢谢!
18
分位数回归方法及应用
16、人民应该为法律而战斗,就像为 了城墙 而战斗 一样。 ——赫 拉克利 特 17、人类对于不公正的行为加以指责 ,并非 因为他 们愿意 做出这 种行为 ,而是 惟恐自 己会成 为这种 行为的 牺牲者 。—— 柏拉图 18、制定法律法令,就是为了不让强 者做什 么事都 横行霸 道。— —奥维 德 19、法律是社会的习惯和思想的结晶 。—— 托·伍·威尔逊 20、人们嘴上挂着的法律,其真实含 义是财 富。— —爱献 生
分位数回归模型及其应用研究
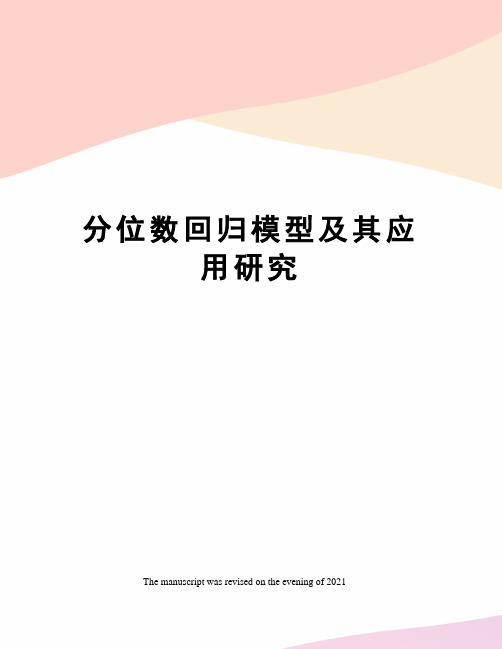
分位数回归模型及其应用研究The manuscript was revised on the evening of 2021第一组计量经济学理论与方法分位数回归模型及其应用研究王桂胜1(首都经济贸易大学,北京,100026)摘要:本文在对分位数回归方法的含义和基本原理进行全面分析说明的基础上,对分位数回归方法在PANEL DATA模型中的应用作了深入分析,并对不同回归估计方法在PANEL DATA模型中的估计效果进行了比较分析。
在此基础上,通过分别采取一般最小平方法和分位数回归法对中国15省区的人均消费和人均收入的回归方程估计的统计结果比较,发现分位数回归方法在进行某些特殊的PANEL DATA模型估计时具有一定的优势。
关键词:分位数回归、面板数据模型、惩罚分位数回归估计一、分位数回归研究介绍自Koenker 和 Bassett (1978)提出线性分位数回归理论以来,分位数回归(QR)即成为近几十年来发展较快、应用广泛的回归模型方法,它不仅深化了对传统回归模型的理解,而且也推广了回归模型的类型和应用,使得回归模型拟合有关统计数据更加准确细致。
分位数回归模型是在稳健估计模型基础上发展形成。
稳健估计(Robust Estimation)理论包括基于一般凸损失函数的M 估计理论、基于样本秩统计量的R估计理论和基于样本次序统计量的L估计理论1王桂胜:男,1970年生,首都经济贸易大学劳动经济学院副教授,清华大学经管学院博士生。
等。
分位数回归强调以解释变量的分位数来估计推断因变量的分位数,通过建立分位数估计方程,并运用线性规划方法或非参数估计等方法来估计相应于不同分位数的解释变量系数或未知参数。
分位数回归是中位数回归和均值回归的推广。
分位数回归模型具体又分为四分位数回归、十分位数回归、百分位数回归、LOGIT分位数回归、审查分位数回归等模型。
关于分位数回归研究的最近发展,主要表现在分位数回归技术方法和方法应用等两方面的研究上。
最新24分位数回归估计

• 例:软件EVIEWS6.0使用手册中实例的斜率对称性检验 结果,其中Y为家庭食物消费支出,X为家庭收入。
Symmetric Quantiles Test
Equation: EQ1
Specification: Y C X
Chi-Sq. d.f. 2
Std. Error 0.025923 0.030529
Prob. 0.0000
Prob. 0.0009 0.0060
Wald统计量 为25.22, 应该拒绝斜
率在 tau=0.25、 0.5和0.75相 等性的假设, 即斜率在不 同分位点上 的值是不同
的。
4、斜率对称性检验
LR()2(V (1 ())V sˆ(()))~2(q)
有约束情况下 最小化θ分位 数回归的目标
函数值
稀疏度
无约束情况下 最小化θ分位 数回归的目标
函数值
约束的数目
3、斜率相等检验
• 斜率相等检验,即检验对于不同的分位点,估计 得到的结构参数(在线性模型中即为斜率)是否 相等。
• 原假设被设定为:
24分位数回归估计
一、分位数回归的提出
1、分位数回归Βιβλιοθήκη 理F(y)=Prob(Yy)
Q ()= in f{ y:F (y) }
Q n()= in f{y:F n(y)}
假定随机变量y的概率分布函数
定义y的θ分位数
给定y的n个观测值,相对应的 分位数
等价地转化为求一个最优化问题
Q n ( ) = a r g m i n { i : Y i |Y i | i : Y i ( 1 ) |Y i | } = a r g m i n { i ( Y i ) }
– 一是与均值回归类似的检验,例如拟合优度检验、约 束回归检验等;
分位数回归估计课件
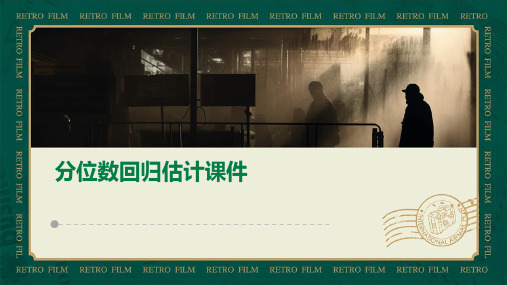
在某些情况下,分位数回归的结果可能对模型假设的违背较为敏感。
分位数回归与其他方法的比较
与普通最小二乘法的比较
普通最小二乘法只关注数据的均值和方差,而 分位数回归可以提供更全面的信息。
与核密度估计的比较
核密度估计主要用于探索性数据分析,而分位 数回归主要用于因果关系推断。
与决策树和随机森林的比较
这些方法主要用于分类问题,而分位数回归主要用于回归问题。
05 分位数回归的未来发展
分位数回归的理论研究
01
深入研究分位数回归的理论基础,包括其假设、性 质和限制条件,以完善其理论体系。
02
探讨分位数回归与其他统计方法的结合,如混合模 型、贝叶斯方法等,以拓展其应用范围。
03
针对分位数回归的统计推断问题,研究更有效的推 断方法和理论。
灵活性
可以估计多个分位数,而不仅 仅是均值。
无分布假设
不需要假定误差项服从特定的 分布,比如正态分布。
刻画异质性
可以更好地捕捉数据的异质性 ,提供更全面的信息。
分位数回归的缺点
计算复杂度
相对于普通最小二乘法,计算成本较高。
解释性
分位数回归的系数较难解释,不如普通最小二乘法直观。
对离群值的敏感性
离群值可能会对分位数回归的结果产生较大影响。
$Y = Xbeta + epsilon$,其中$Y$是因变量,$X$是自变量,$beta$是待估 计的参数,$epsilon$是误差项。
非线性分位数回归模型
通过引入非线性函数或变换,使得模型能够更好地拟合非线性关系。
分位数回归的估计方法
最小二乘法
通过最小化残差平方和来估计参数。
迭代加权最小二乘法
我国固定资产投资的分位数回归解析

龙源期刊网
我国固定资产投资的分位数回归解析
作者:李超
来源:《北方经济》2009年第24期
摘要:基于对中国固定资产投资规模诸多影响因素的定性分析基础上,运用普通回归分析挑选出其中4个主要影响因素.利用分位数回归技术对《中国固定投资统计年鉴》中的全国省市自治区(西藏、台湾除外)的固定资产资投资调查数据考察了其分布特征,然后分别对国家固定资产投资的主要影响因素进行统计建模与分析,揭示了其在不同分位点时的关系变动情况及影响效果。
关键词:固定资产投资分位数回归分布低端分布高端。
分位数回归及其实例
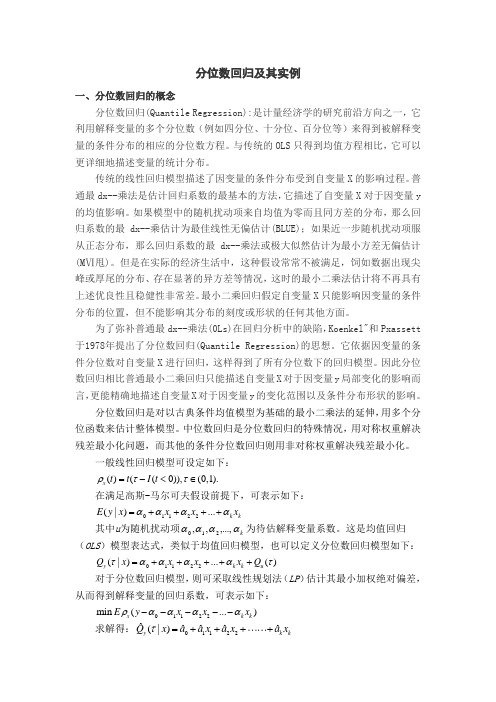
分位数回归及其实例一、分位数回归的概念分位数回归(Quantile Regression):是计量经济学的研究前沿方向之一,它利用解释变量的多个分位数(例如四分位、十分位、百分位等)来得到被解释变量的条件分布的相应的分位数方程。
与传统的OLS 只得到均值方程相比,它可以更详细地描述变量的统计分布。
传统的线性回归模型描述了因变量的条件分布受到自变量X 的影响过程。
普通最dx--乘法是估计回归系数的最基本的方法,它描述了自变量X 对于因变量y 的均值影响。
如果模型中的随机扰动项来自均值为零而且同方差的分布,那么回归系数的最dx--乘估计为最佳线性无偏估计(BLUE);如果近一步随机扰动项服从正态分布,那么回归系数的最dx--乘法或极大似然估计为最小方差无偏估计(M Ⅵ甩)。
但是在实际的经济生活中,这种假设常常不被满足,饲如数据出现尖峰或厚尾的分布、存在显著的异方差等情况,这时的最小二乘法估计将不再具有上述优良性且稳健性非常差。
最小二乘回归假定自变量X 只能影响因变量的条件分布的位置,但不能影响其分布的刻度或形状的任何其他方面。
为了弥补普通最dx--乘法(0Ls)在回归分析中的缺陷,Koenkel"和Pxassett 于1978年提出了分位数回归(Quantile Regression)的思想。
它依据因变量的条件分位数对自变量X 进行回归,这样得到了所有分位数下的回归模型。
因此分位数回归相比普通最小二乘回归只能描述自变量X 对于因变量y 局部变化的影响而言,更能精确地描述自变量X 对于因变量y 的变化范围以及条件分布形状的影响。
分位数回归是对以古典条件均值模型为基础的最小二乘法的延伸,用多个分位函数来估计整体模型。
中位数回归是分位数回归的特殊情况,用对称权重解决残差最小化问题,而其他的条件分位数回归则用非对称权重解决残差最小化。
一般线性回归模型可设定如下:()((0)),(0,1).x t t I t ρττ=-<∈在满足高斯-马尔可夫假设前提下,可表示如下:01122(|)...k k E y x x x x αααα=++++其中u 为随机扰动项k αααα,...,,,210为待估解释变量系数。
分位数回归
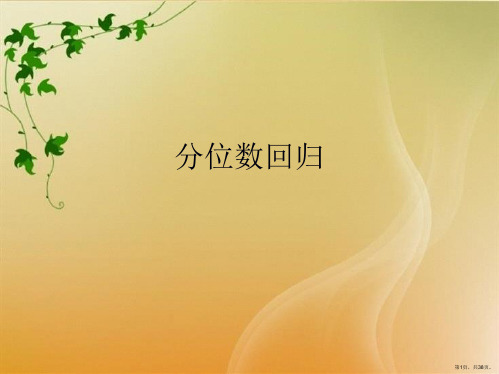
分位数回归原理
假设随机变量的分布函数为:
F(y)=Prob(Yy)
Y的 分位数的定义为:
Q () = in f { y :F ( y ) } ,0 < < 1
回归分析的基本思想就是使样本值与拟合值之间
的距离最短,对于Y的一组随机样本 ,样本均值
回归是使误差平方和最小,即
• 应用软件中给出了一些相应的检验统计量。
第36页,共38页。
5、斜率对称性检验
斜率对称性检验,即检验对于给定的X,Y的分布是否 是对称的。假设我们要检验的分位数回归模型有m个, m是奇数,且中间值τ(m+1)/2是0.5,其他τ都关于0.5对称, 即τj=1−τm-j+1, j=1,…,(m-1)/2。参数估计量按照τk的大小
LT()2(Q ~(1())sQˆ(()))
T()(12Q ˆ()s)()logQ Q ~ˆ((()))
两个统计量都渐近服从自由度为q的卡方分布,其中q
是原假设目标函数中约束条件的个数。
和
~ Q
(分) 别
代表Qˆ 有( )约束的和无约束目标方程的极小值。s(τ)是分位数
密度函数。
第28页,共38页。
似然比检验:
• 似然比
• 命题: H0:gC
• 检验思想:如果约束是无效的,有约束的最大似然函数值 当然不会超过无约束的最大似然函数值,但如果约束条件 “有效”,有约束的最大值应当“接近”无约束的最大值, 这正是似然比检验的基本思路。
•无约似束然模比型:似 然 函LL (( 数ˆ ,,值ˆ 22 )):
因的作为用越强,,所越以远R*远(τQ)ˆ小(的 ) 于值在,0和R*1(之τ)越间Q~(接,) 近解于释1变,量反 之,越接近于0。所以可用来考察解释变量对被解释 变量第τ分位数回归拟和的好坏。
- 1、下载文档前请自行甄别文档内容的完整性,平台不提供额外的编辑、内容补充、找答案等附加服务。
- 2、"仅部分预览"的文档,不可在线预览部分如存在完整性等问题,可反馈申请退款(可完整预览的文档不适用该条件!)。
- 3、如文档侵犯您的权益,请联系客服反馈,我们会尽快为您处理(人工客服工作时间:9:00-18:30)。
Private school vouchers and student achievement:Afixed effects quantile regression evaluation ☆Carlos Lamarche ⁎Department of Economics,University of Oklahoma,321Hester Hall,729Elm Avenue,Norman,OK 73019,United StatesAvailable online 3May 2008AbstractFundamental to the recent debate over school choice is the issue of whether voucher programs actually improve students'academic ing newly developed quantile regression approaches,this paper investigates the distribution of achievement gains in the first school voucher program implemented in the US.We find that while high-performing students selected for the Milwaukee Parental Choice program had a positive,convexly increasing gain in mathematics,low-performing students had a nearly linear loss.However,the program seems to prevent low-performing students from having an even bigger loss experienced by students in the public schools.©2008Elsevier B.V .All rights reserved.JEL classification:I21;I28Keywords:School choice;V ouchers;Milwaukee;Fixed effects;Quantile regression“While we celebrate those [students]doing well,we can't turn a blind eye to those who are not ”Rod Paige,US secretary of Education,to the NY Times.Available online at Labour Economics 15(2008)575–590☆Version:March 31,2008.This paper is based on Chapter 2of my dissertation “Quantile Regression for Panel Data ”at the University of Illinois at Urbana-Champaign.I especially thank my advisor Roger Koenker for advice and detailed comments.I am grateful to Dan Bernhardt,Greg Burge,Todd Elder,Lynn Gottschalk,Kevin Hallock,Kangoh Lee,Darren Lubotsky,as well as labor lunch participants at the University of Illinois at Urbana-Champaign and seminar participants at Texas Tech and the 2007Annual Meetings of the European Association of Labour Economists.I thank Professor Cecilia Rouse for providing the Milwaukee Parental Choice program's data.I would like to thank the Guest Editor as well as two anonymous referees for their very helpful comments.⁎Tel.:+14053255857.E-mail address:lamarche@.0927-5371/$-see front matter ©2008Elsevier B.V .All rights reserved.doi:10.1016/beco.2008.04.007576 marche/Labour Economics15(2008)575–5901.IntroductionFundamental to the recent debate over school choice is the issue of whether voucher programs actually improve students'academic achievement,while decreasing inequalities between the best and worst ton Friedman's proposal to use vouchers as a method of improving the quality of education is based on the idea that private schools are more productive than public schools,which is still a highly controversial issue.If private schools are in fact more efficient than public schools,governments can improve the quality of education by offering tuition vouchers to families that want to send their children to private schools.The Milwaukee Parental Choice program,the first program implemented in the US,has been providing vouchers to low-income students to attend private school since1990.The simplicity of the program's idea contrasts sharply with the complexities that plague the program's evaluation.The voucher programs'effect,for example from time t to t',is the difference between what would have happened at time t'if the student was selected and remained in a choice school during the time interval,and what would have happened at time t'if the student was not selected,and remained in the Milwaukee public school.This counterfactual exercise is impossible to obtain using observational data(Rubin,1974).It may be possible,however,to construct groups using a randomized experiment(e.g.,children randomly assigned to attend choice schools and to attend public schools).At time t',the difference between students' academic performances could be attributed to the type of school since the initial assignment was random.However,the Milwaukee Parental Choice program was not implemented under idealized conditions(Witte,2000),and therefore the selection of the control group plays a major role.Given the lack of a valid control group,it is not surprising that previous empirical studies have delivered mixed findings.Rouse's(1998)seminal study used a sample of students in the Milwaukee public schools as a comparison group and individual fixed effects to control for latent characteristics,such as more motivated parents or student abilities that may differ between treatment and comparison groups.The presented approach builds upon Rouse by employing a newly developed fixed effects form of quantile regression that not only controls for unobserved individual heterogeneity,but also allows an examination of the program effects at different points of the educational attainment distribution.The empirical literature(e.g.,Witte,1997;Green et al.,1997;Rouse,1998)has focused upon estimating how the selection to attend the Milwaukee choice schools affects mean test scores. This approach to evaluation may be incomplete for policy analysis of programs serving heterogeneous students.To illustrate,if a stated policy goal is to raise students'achievement to a predetermined minimum standard,it may not be optimal to pursue a program that benefits strong students while causing weaker students to fall further behind.Because education is expected to play an important role in mitigating inequality,“the distribution of achievement gains... constitutes an appropriate criterion for evaluating a school choice intervention”(Howell and Peterson,2002).This paper focuses on the estimation of the selection to attend choice schools on the entire distribution of test scores,considering patterns of achievement in terms of quantiles.There are important reasons why economists,educators,and policy makers are interested in how voucher programs affect students'achievement beyond the mean effect,which is typically estimated with Ordinary Least Squares(OLS)or Instrumental Variables(IV).First,the standard methodology may miss how a policy affects achievement differently at different points of the conditional test score distribution,as illustrated in Eide and Showalter(1998).Second,the possibility that vouchers may increase the differences between high-and low-performing students in the private schools,concern notably reflected in Ascher,Fruchter,and Berne(1996)question“What mechanisms ensure those students [in private schools]who need extra time and attention...receive this more costly instruction?”.Our analysis shows that being selected to participate in the program had an heterogeneous effect on educational attainment,which had not been uncovered by the previous work focusing on mean effects.First,we find that the selected students had an increase in math achievements that ranges from 3.1to 1.2percentile points per year across quantiles.The program seems to dramatically improve the academic achievements of the weak students,having a relatively modest effect on achievements among strong students.Second,although the mean effect suggests that the program has no effect on reading,we find some evidence suggesting that the program improved and reduced the achievements of the low-and high-performing students,ing a new instrumental variable estimator for quantile regression (Chernozhukov and Hansen,2005),we find that the effect of being enrolled in choice schools ranges from 2.3percentile points per year at the 0.1quantile of the conditional distribution of reading scores,to −2.4at the 0.9quantile.This result is important for policy analysis since the Milwaukee vouchers seem to have no effect at the mean,but indeed matter at the tails of the educational attainment distribution.Lastly,our results also reveal that students'gains,measured as the differences between test scores conditional on years since application to the program,are subtle in nature.While the “average ”student in the program had a linear gain in mathematics,high-performing students had a positive,convexly increasing gain,and low-performing students had a nearly linear loss.The offer of vouchers seems to increase the inequalities between low-and high-performing students.However,as we mentioned above,weak students'scores increased dramatically,in levels,compared to public school students'scores.Therefore,the evidence suggests that being selected for the choice program prevented low-performing students from having an even bigger loss experienced by low-performing students in the public schools.The next section briefly introduces a simple behavioral framework,and Section 3presents models and estimators.Section 4describes the data and Section 5the empirical results.Section 6offers conclusions.2.A simple behavioral frameworkWe develop a simple variation of a model of mothers'decisions to enroll children in preschool (Behrman et al.,2004).1The mother of student i maximizes a time separable utility function that depends on consumption C and the child's test score T .There is a technology that produces test scores depending on socioeconomic characteristics x ,attendance to a choice school P ,and stochastic factors εj ,T =T (x ,P ,εj ).We assume that εj is realized after the student attends school.For example,dissatisfaction with teachers,quality of education,school transportation problems associated either with choice school j =1or public school j =0attendance.The variable actual attendance to a choice school P is equal to aS −1,taking value one if the student actually attends a choice school,0otherwise.The variable attendance to a choice school a takes the value one if the mother decides that the student should attend a choice school,and the variable selected to attend choice school in period t −1,S −1,take the value one if the student was stly,each period the mother must spend assets A on consumption and,if she decides to send her child to a choice school,on a fixed cost K .1The framework models heterogeneity,which is unobserved by the econometrician,that can be recovered using quantile regression techniques (Koenker,2005).Alternative strategies are considered in Bitler et al.(2006),Hastings et al.(2006),and Cullen et al.(2005).577marche /Labour Economics 15(2008)575–590It is possible to express the mother's dynamic problem simply as max a {υ0,υ1},where υ0represents the value of not attending a choice school,and υ1denotes the value of attending a choice school.If at time t the student was not selected,her mother trivially chooses a =0because she does not want to give up consumption to pay K .On the other hand,if at time t the student was selected to attend a choice school,the decision is simply,a =1{υ1≥υ0|S −1=1}.This decision depends,among other factors,on the cost of attending a choice school,dissatisfaction with the choice schools,and mother's weight to her child educational achievements.These factors may be different among families,and consequently the probability of attendance may be different as well.3.Evaluation of the Milwaukee voucher programThe previous behavioral framework impose two restrictions on the evaluation of the voucher program,T it ¼T x it ;P it ;e it ðÞ¼x V it A þb P it Àe itð1ÞP it ¼1υ1it z υ0it ÞS it À1¼d þq it S it À1:Èð2ÞThe assumption of linearity in Eqs.(1)and (2)gives a direct link to Rouse's (1998)structural equation model.Rouse estimates a reduced form equation assuming that the effect of selection into the voucher program on the probability of attendance is constant among families (ρit =ρ),yielding an “intention-to-treat ”effect π1that is equal to ρβ.An enormous difficulty in the evaluation of the Milwaukee Parental Choice program is the lack of a valid control group.Initial randomized treatment is needed to obtain an unbiased estimator of the voucher offer,but the program's randomization was based on the applicant group.2Green et al.(1997)used the students not selected to attend choice schools as a comparison group,but this group may lead to selection bias (Rouse,1998;Witte,2000).Witte (1997)used a random sample of students from public schools as a comparison group,but as Rouse (1998)pointed out,there may be differences between the students that were selected to attend choice schools and the students that remained in the public schools.3Only eligible parents who are more interested in their child's achievements apply to the program,which suggests that students who remained in the public system may have parents with a less strong preference for private schools.To get around these problems,Rouse (1998)used both the unsuccessful applicants and a random sample of students from the public schools as comparison groups,and individual fixed effects to control for unobservables such us more motivated parents or student ability.Our approach builds upon Rouse,estimating a fixed effects version of the quantile regression model,Q T it s j j S it À1;x it ;a i ÀÁ¼p 0s j ÀÁþp 1s j ÀÁS it À1þx V it p 2s j ÀÁþa i ;ð3Þwhere Q (·|·)is the τj -th conditional quantile function,and αi is an individual fixed effect.The non-selected students that are omitted for simplicity in Eq.(3)will be introduced in the regression 2The Wisconsin legislature required choice schools to select students at random when the grade was oversubscribed.Although the best schools received more applications,the total number of applications exceeded the number of seats (Witte,2000).3For instance,consider a family making a decision as to whether or not to apply to the program based on υi 0j =x i 0′μ+αi j ,where the binary variable j takes the value 1if the student applies to the program,and the value 0otherwise.From Eq.(2),the participation in the program may depend entirely on parent's weight to her child educational achievements,P i 0=1{υi 01−υi 00≥0}=1{αi 1−αi 0≥0}.578 marche /Labour Economics 15(2008)575–590models estimated in Section 5.If we consider the possibility that the effect of selection to attend choice schools on the probability of attendance ρit is a linear function of ωi (e.g.,individual or family reasons to leave the choice schools)and εit (e.g.,choice program or private school factors),it is straightforward to show that the intention-to-treat effect,p 1s j ÀÁ¼b q ÀF À1e s j ÀÁÀÁ¼Q T it s j j S it À1¼1;x it ;a i ÀÁÀQ T it s j j S it À1¼0;x it ;a i ÀÁ:The model,in its simplest version,assumes that the effect of the voucher offer π1(τj )is decreasing on the quantile τ,and depends on the effect of the selection on the probability of attendance and the differential school effect on achievement.We briefly consider two competing explanations.First,we may consider that the effect is a combination of a treatment effect βand a location-scale shift effect ρ(τj )=ρ−F ε−1(τj ).4We conjecture that while only high-motivated students among low-performing students stay in the choice schools,most students among the high-performing students stay.Since those lower-performing students in the private schools are relatively more motivated than the lower-performing students in the public schools,the ‘intention-to-treat effect ’should be decreasing in terms of quantiles.Alternatively,we can hypothesize that it is a combination of a scale treatment effect β(τj )=−βF ε−1(τj )and a location shift effect β×ρ.It has been argued that private schools may work on all students equally,while public schools that are interested in raising mean achievement may give less amount of attention to lower-performing students rather than higher-performing students (Howell and Peterson,2002,p.156).Although the model now suggests that the voucher program has a different mechanism to affect achievement,we again expect to see a decreasing π1over the quantiles.The empirical evidence will offer below (see,e.g.,Figs.1and 2)provides some support for the latter explanation.3.1.EstimationWe estimate the ‘intention-to-treat ’effect π1(τj )using a quantile regression version of the classical fixed effects estimator introduced by Koenker (2004),b p s j ÀÁÈÉJ j ¼1;b a i f g N i ¼1n o u arg min p ;a X J j ¼1X T t ¼1X N i ¼1x j q s j T it Àp 0s j ÀÁÀp 1s j ÀÁS it À1Àa i ÀÁwhere ρτj (u )=u (τj −I (u ≤0))is the quantile loss function,and ωj is the weight (e.g.,1/J )given to the j th quantile.For simplicity,the vector of independent variables x it ,defined below,is omitted.Parents'and students'unobserved characteristics that may differ between students'selected to attend choice schools and students in the public schools are captured by individual fixed effects αi 's,independent of the quantiles.We advance the method in two directions.First,we propose to use panel-bootstrap to estimate the precision of the estimates of the parameters of the model.Our strategy accommodates to forms of heteroscedasticity replacing pairs {(T i ,S i )}:i =1,…,N }over cross-sectional units i .For small and fixed number of time series observations,the standard errors are consistent provided that the 4It seems natural then to explore the differences between the treatment effect βand the intention-to-treat effect βρat different quantiles of the educational attainment distribution.We first estimated a reduced form model based on Eqs.(1)and (2)to obtain b p 1¼b b q .We then estimated Eq.(1)to obtain βb considering the instrumental variable approach described in Section 3.1.We found that the percentage change between the treatment effect and the intention-to-treat effect has a tendency to increase suggesting that the effect of selection on the probability of attending choice schools is decreasing in τ.579marche /Labour Economics 15(2008)575–590Fig.2.Estimates of the treatment effect.The panels present instrumental variable quantile regression estimates (solid line with dots),classical instrumental variable estimates (dashed line),and .95%confidenceintervals.Fig.1.Estimated effects of being selected for the choice program times years since application on educational attainment.The panels present quantile regression estimates (solid line with dots),classical fixed effects (dashed line),and .95%confidence intervals for the point estimates.580 marche /Labour Economics 15(2008)575–590number of cross-sectional units passes to infinity.Second,we develop a simple framework for testing,described in Appendix A.We also implement an instrumental variable form of quantile regression to estimate the causal effect of the program.By accommodating the function T (·)and the error term,Eqs.(1)and (2)are the system of equations considered by Chernozhukov and Hansen (2005,3.1),who propose an instrumental variable (IV)method for estimating quantile regression treatment effects.We estimate the effect of choice schools on test scores considering,Q T it s j P it ;x it ðÞ¼g s ðÞþb s ðÞP it þx V it A s ðÞð4Þwhere β(τ)is the parameter of interest.The vector x it includes “applicant pool ”dummy variables,a dummy variable for gender,family income,an indicator if family income is missing,and the grade level of the student when the student took the test.Since the selection to attend choice school is exogenous conditional on the school and grade to which the student applied,we use whether the child was randomly selected to attend choice schools S it −1as an instrument for actual enrollment P it .4.DataWe analyze data from the Milwaukee Parental Choice program.5We consider a sample that contains information on applicants to the program including the students that were not selected for the choice schools and students from the Milwaukee public schools as in Rouse's paper (Table 1).There is data on reading and math test scores,based on the normal curve equivalent measure (NCE)from the Iowa Tests Basic Skills (ITBS).The empirical analysis is based on a sample of African-American and Hispanic students who applied to the choice program between 1990and 1993,and a sample of students from the Milwaukee public schools.6Test scores:The Normal Curve Equivalent (NCE)measure is a transformation of the ITBS that produces an integer-level measure,ranging from 1to 99,with a national mean of 50and a standard deviation of 21.We rely on math and reading NCE test scores because (a)the results can be compared with previous paper's findings (e.g.,Witte,1997;Rouse,1998),and (b)the NCE measure has the advantage that it can be averaged.According to test makers,the NCE test score is the same if all the students make one year of progress after one academic year.If some students make more (less)progress in one year than the students in the population of interest,the NCE test score will be higher (lower).75The Milwaukee Parental Choice program was targeted and limited,aimed to provide better educational opportunities to low income families.The program target was poor families living in the city of Milwaukee whose children were not attending private school that year.Families with income,at most,1.75times the national poverty line were eligible to apply (e.g.,the threshold for a family of three was $21,000).6We use Rouse's sample of public school students assuring the comparability of results.As a robustness check,we estimated the effect of the voucher offer considering public school students with income less or equal to 1.75times the national poverty line in 1990.The main empirical findings of this paper are robust to restricting the control group to include only low income students.7One-point increase at the quantile τmay not be translated to the same amount of knowledge in different grades,but addressing this possibility is beyond the scope of this paper.(Note that this same possibility is present when analyzing mean effects).Rather,the present exercise is focused on an investigation of the changes in the educational attainment conditional distribution for the selected students and public school students.Furthermore,we analyze trends over several years to avoid drawing conclusions based on one-year changes that are likely to be noisy measures of performance (Kane and Staiger,2002).581marche /Labour Economics 15(2008)575–590Year since application:This variable is defined as the first year in which a student applied if he either was selected the first time he applied or he was never selected to attend choice school.In case a student applied two or more times,the first year she was accepted is considered the year of application.In the case of the students in the Milwaukee public schools,the year of application was imputed consid-ering year t the “year of application ”for students who have a valid year t +1test score (Rouse,1998).Application lotteries:The probability of being selected to attend choice school is random conditional on the school and grade to which the student applied,because the students were randomly selected when the school was oversubscribed for a particular grade.Consequently,we need controls in model (4)for the school and grade to which the student applied (see,e.g.,Rouse,1998).The application lotteries are time-invariant dummy variables,therefore they will not be included in model (3)because the variables are subsumed in the individual effects.5.ResultsWe shall present the empirical results.Instead of the focus on the mean achievement,we will report the effect of being selected to attend choice schools on the entire distribution of educational attainment.5.1.The heterogeneous impactWe will estimate a somewhat more complicated model than Eq.(3)containing the selected students,the non-selected students,and the sample of students from the public schools.We also introduce interactions between the indicator for whether or not the student was selected to attend a choice school and the years since application because previous researchers have argued that child's educational achievement may not improve immediately.We consider first the simplest version,assuming that gains are equal from year-to-year,T it ¼p 0þp 1d it ÂS it ðÞþp 2d it ÂN it ðÞþX4k ¼À3p 3k d it Àk þp 4S it þp 5N it þa i þe it ð5ÞTable 1Descriptive statistics for the main variables in Rouse's sample analysisVariables StudentsSelectedNot selected MPS Proportion currently enrolled in a choice school0.558(0.497)0.010(0.102)–Math (NCE)scores39.236(18.706)38.108(18.777)40.411(18.483)Reading (NCE)scores37.647(16.265)37.886(17.090)38.700(16.461)Proportion female0.536(0.499)0.470(0.499)0.523(0.500)Family income (in thousands of 1994dollars)12.052(5.915)12.510(5.901)21.750(8.658)Proportion missing income family0.412(0.492)0.542(0.498)0.747(0.435)Proportion African-American0.795(0.404)0.864(0.343)0.868(0.338)Proportion Hispanic0.205(0.404)0.136(0.343)0.132(0.338)Grade level test3.767(2.250) 3.857(2.088)4.337(2.122)Proportion with imputed math test(Rouse,1998methodology)0.074(0.262)0.194(0.396)0.164(0.371)Number of students9863582014Number of observations24628595408MPS stands for Milwaukee public schools.582 marche /Labour Economics 15(2008)575–590where d it −k indicates the number of years pre-or post-application to the program,(d it ×S it )is an interaction between the number of years since application and whether the student was selected to attend choice schools,and Nit is a dummy variable indicating whether the student applied to the program and was not accepted.5.1.1.Fixed effects estimatesWe report estimates for the full sample in Table 2.First,the table shows negative estimates for the effect of being selected into a choice school,suggesting that the selected students had lower math test scores than the students in the public schools when they entered the program.8Although we cannot provide a definitive explanation,it may be possible that program's applicants were not doing well in the public schools.Witte (2000)presents evidence on parental dissatisfaction with their kids'prior school within the Milwaukee public schools finding that (a)choice students seem to have less satisfied parents,and (b)the level of parents'dissatisfaction is statistically different between the choice students and the students in the public schools.He also argues that attitudes of parents toward their kids'prior school may be associated to their children's academic 8Research had indicated that choice students have low test scores relative to low-income public school students (see,e.g.,Witte,2000),but the literature is relatively silent on explaining why the academic achievement of these students was low when they entered the program.As shown in Table 2,Rouse's (1998)estimated mean effect of being selected into the program on math test scores is negative and significant.Table 2Estimates of the selection to participate in the choice program on Math and Reading scores estimates for the full sampleQuantiles0.10.250.50.750.9MeanFixed effects methods Dependent variable=math (NCE)test scoreSelected to attendchoice school−3.649(1.866)−4.270(1.429)−3.321(1.167)−2.288(1.192)−1.173(1.633)−3.391(1.114)Not selected to attendchoice school−2.758(3.192)−5.026(2.109)−3.621(2.170)−2.769(2.150)−1.326(2.707)−3.052(1.741)Selected×numberof years2.323(0.794)3.143(0.608) 2.321(0.454) 1.685(0.477) 1.178(0.669) 2.294(0.399)Not selected×numberof years 0.919(1.643) 1.681(1.122) 1.526(1.138)0.844(0.960)−0.675(1.026)0.672(0.783)Fixed effects methods Dependent variable=reading (NCE)test scoreSelected to attendchoice school−0.320(1.625)−1.390(1.372)0.180(1.254) 1.400(1.169) 2.780(1.412)0.750(1.027)Not selected to attendchoice school−2.140(3.479)−3.200(2.070)−0.555(2.161)1.350(2.008) 2.605(2.410)−0.644(1.584)Selected×numberof years0.320(0.687)0.690(0.630)−0.120(0.541)−0.700(0.472)−1.160(0.635)−0.249(0.368)Not selected×numberof years 0.440(1.555) 1.500(1.069)0.555(0.898)−0.350(0.813)−0.985(1.015)0.247(0.706)Math regressions include a dummy variable for whether the test score was imputed.The total number of observations are 8729(Math)and 8751(Reading).The standard errors (in parenthesis)are obtained after 1000panel-bootstrap replications.583marche /Labour Economics 15(2008)575–590。