介绍qtl及标记辅助选择等基本的概念Euphytica (2005)
QTL 定位方法---

QTL 定位方法分子标记技术和数量遗传学的发展,使得分子遗传学与数量遗传学相互渗透和融合,从而形成了一个新的研究领域—分子数量遗传学(Molecular Quantitative Genetics)。
分子数量遗传学研究的内容,就是借助分子标记,采用适当的统计分析方法明确QTL 在染色体上的位置及其效应。
而QTL 定位的原理是:利用适当的分离群体,构建较高密度的、分布较均匀的、覆盖全基因组的分子标记连锁图。
根据遗传连锁的基本遗传学原理,对分离群体中单株的标记基因型和性状的表型值进行一定的统计分析,将决定数量性状的QTL 定位在分子标记连锁图中。
目前,QTL 定位的方法主要有单标记分析法(Edwards et al, 1987),区间作图法(Lander and Botstein, 1989)和复合区间作图法(Zeng, 1994)等。
单标记法(Single marker analysis)是最简单的分析标记与性状关联的方法,包括以标记为基础的分析方法(Marker-based analysis, MBA)和以性状为基础的分析方法(Trait-based analysis, TBA,Lebowitz et al., 1987)。
前者利用每个标记位点不同基因型间的性状均值差异,以传统的单因素方差分析法测验被研究的数量性状在标记基因型间的差异显著性。
对于一个作图群体而言,任意标记位点具有三种基因型(F2群体)或两种基因型(回交群体、重组自交系、双单倍体系),分析每一基因型个体的数量性状均值的差异,并进行F 测验,当F 测验显著时,则表明该标记位点可能与一个或多个QTL 连锁。
利用这种方法进行的数量性状分析,既简单又符合QTL 定位的基本统计原理,且不需要完整的分子标记连锁图,是定位QTL 的最为有效方法。
但不足之处是不能准确估计QTL 的位置,且往往会低估其遗传效应。
以性状为基础的分析方法的原理是假定因选择而使数量性状的高表型个体中的QTL 增效等位基因和低表型个体中的QTL 减效等位基因的频率增加,当QTL 的等位基因与某一标记基因连锁时,会因相互关联而导致高、低表型个体间标记基因频率的差异。
QTL的名词解释
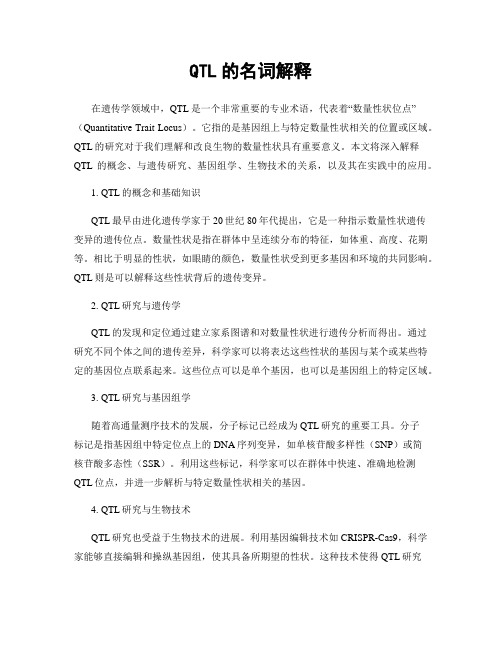
QTL的名词解释在遗传学领域中,QTL是一个非常重要的专业术语,代表着“数量性状位点”(Quantitative Trait Locus)。
它指的是基因组上与特定数量性状相关的位置或区域。
QTL的研究对于我们理解和改良生物的数量性状具有重要意义。
本文将深入解释QTL的概念、与遗传研究、基因组学、生物技术的关系,以及其在实践中的应用。
1. QTL的概念和基础知识QTL最早由进化遗传学家于20世纪80年代提出,它是一种指示数量性状遗传变异的遗传位点。
数量性状是指在群体中呈连续分布的特征,如体重、高度、花期等。
相比于明显的性状,如眼睛的颜色,数量性状受到更多基因和环境的共同影响。
QTL则是可以解释这些性状背后的遗传变异。
2. QTL研究与遗传学QTL的发现和定位通过建立家系图谱和对数量性状进行遗传分析而得出。
通过研究不同个体之间的遗传差异,科学家可以将表达这些性状的基因与某个或某些特定的基因位点联系起来。
这些位点可以是单个基因,也可以是基因组上的特定区域。
3. QTL研究与基因组学随着高通量测序技术的发展,分子标记已经成为QTL研究的重要工具。
分子标记是指基因组中特定位点上的DNA序列变异,如单核苷酸多样性(SNP)或简核苷酸多态性(SSR)。
利用这些标记,科学家可以在群体中快速、准确地检测QTL位点,并进一步解析与特定数量性状相关的基因。
4. QTL研究与生物技术QTL研究也受益于生物技术的进展。
利用基因编辑技术如CRISPR-Cas9,科学家能够直接编辑和操纵基因组,使其具备所期望的性状。
这种技术使得QTL研究不仅限于观察性的位点关联,还包括功能性的基因验证和工程。
这有助于我们更好地理解QTL与数量性状之间的关系,并为生物改良和育种提供新的可能性。
5. QTL的应用QTL的发现对农业、畜牧业和医学等领域具有重要意义。
在农业领域,科学家可以通过QTL的定位将与高产量、抗病性和适应性等重要农艺性状相关的基因或位点引入到重要的农作物中。
(整理)数量性状的分子标记QTL定位的原理和方法讲义

数量性状的分子标记(QTL定位的原理和方法讲义)作物中大多数重要的农艺性状和经济性状如产量、品质、生育期、抗逆性等都是数量性状。
与质量性状不同,数量性状受多基因控制,遗传基础复杂,且易受环境影响,表现为连续变异,表现型与基因型之间没有明确的对应关系。
因此,对数量性状的遗传研究十分困难。
长期以来,只能借助于数理统计的手段,将控制数量性状的多基因系统作为一个整体来研究,用平均值和方差来反映数量性状的遗传特征,无法了解单个基因的位置和效应。
这种状况制约了人们在育种中对数量性状的遗传操纵能力。
分子标记技术的出现,为深入研究数量性状的遗传基础提供了可能。
控制数量性状的基因在基因组中的位置称为数量性状基因座(QTL)。
利用分子标记进行遗传连锁分析,可以检测出QTL,即QTL定位(QTL mapping)。
借助与QTL连锁的分子标记,就能够在育种中对有关的QTL的遗传动态进行跟踪,从而大大增强人们对数量性状的遗传操纵能力,提高育种中对数量性状优良基因型选择的准确性和预见性。
因此,QTL定位是一项十分重要的基础研究工作。
1988年,Paterson等发表了第一篇应用RFLP连锁图在番茄中定位QTL的论文。
之后,随着分子标记技术的不断发展以及许多物种中分子连锁图谱的相继建成,全世界出现了研究QTL的热潮,每年发表有关QTL 研究的论文数量几乎呈指数增长(图5.1),显示了该研究领域的勃勃生机。
目前,QTL定位研究已在许多重要作物中展开,并且进展迅速。
本章主要介绍QTL定位的原理和方法。
图5.11986~1998年期间国际上每年发表有关QTL研究的论文的数量. 数据从英国BIDS信息系统检索得到第一节数量性状基因的初级定位QTL定位就是检测分子标记(下面将简称为标记)与QTL间的连锁关系,同时还可估计QTL的效应。
QTL定位研究常用的群体有F2、BC、RI和DH。
这些群体可称为初级群体(primary population)。
qtl名词解释

qtl名词解释QTL(QuantitativeTraitLoci)是指在染色体上能影响特定量化性状的细胞或组织的一些位点,它们可以被用来推断种群遗传变异的遗传机制,也可以分析多个植物的遗传和生理机制。
QTLs是基因组学研究的一个重要组成部分,在遗传学、分子生物学和生物信息学领域都有重要应用。
QTL实际上是一个抽象概念,指的是存在于基因组中的某一位置,这个位置上的基因会影响一个特定量化性状,比如颜色、抗病性、抗虫性、汁液分泌等。
QTL可以是一个简单的基因座,也可以是多个基因座的组合,由于不一定断定QTL对应哪一个实际的基因,也就不一定能说明它的表型产生方式,所以QTL的研究具有很大的挑战性和技术性。
QTL研究主要分为两大步骤,即定位和认识。
首先,利用数学方法定位QTL,即在遗传谱系中找出与特定性状相关联的染色体位点;第二步,进行QTL认识,即定义QTL的表型影响。
QTL认识可以通过分析已有数据和新技术进行获取,比如对QTL对应基因进行测序、克隆和表达谱分析,从而获取更多的关键信息。
QTL研究可以帮助科学家研究特定性状的遗传机理,由于此类研究的受益者比较多,比如农业、植物科学和医学等,所以QTL研究得到了越来越多的关注。
QTL研究在分子水稻育种领域的应用,尤其是对于基因定位的研究,取得了重要的成果,例如根瘤病抗性、米粒粗糙度、米质等特定性状。
了解QTLs在植物特定性状上所发挥的作用,可以提高植物种质的利用效率,培育优良品种,进而改善作物产量和质量,促进农作物的可持续发展。
QTL的研究也可以应用于其他生物,比如动物、微生物等,诸如特定疾病的发生、性状的发育等,都可以通过QTL研究来探究其遗传机制。
例如,在研究非洲黄热病病毒基因中,科学家们发现了一个重要的QTL,它可以预测昆虫是否会发病,从而开展防控研究,从而降低昆虫对病毒感染的风险。
综上所述,QTL是指染色体细胞上可以影响某一特定量化性状的位点,利用QTL可以获得更多的基因结构信息,这有助于我们深入理解植物的遗传和生理机制,也有助于提高农作物的产量和质量,QTL 的研究也可以应用于其他生物,用于研究特定疾病的发生等。
作物QTL分析的原理与方法
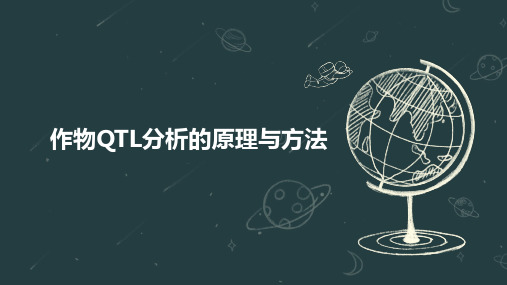
作物QTL定位方法与技术作物QTL定位的方法主要有传统连锁分析、基因芯片 技术和深度学习等。连锁分析通过群体遗传学手段,鉴定两个或多个基因位点 间的连锁关系,进而确定控制性状的QTL。基因芯片技术利用基因组wide的标 记分布,对大量基因位点进行同时检测,高效地定位QTL。深度学习则利用神 经网络等算法,自动化学习和识别数据中的特征,实现对QTL的精准定位。
四、自然群体
自然群体是指在没有人为干预下自然形成的群体,如野生种、地方品种、自然 变异群体等。这些群体通常具有丰富的遗传变异和复杂的遗传结构,对于研究 作物的适应性、抗逆性和产量等性状的遗传基础非常有用。此外,自然群体还 可以用于发现和克隆稀有或特殊的QTL。
五、基于基因组的作图群体
随着基因组学技术的发展,基于基因组的作图群体越来越受到重视。这种群体 可以通过重测序技术获得大量的SNP(单核苷酸多态性)标记,并利用这些标 记构建高密度的遗传图谱。这种图谱可以用于精细定位和克隆QTL,以及研究 基因组中的结构变异和非编码区基因组。
2、QTL分析的具体步骤
(1)数据采集:收集作物的基因型和表型数据。基因型数据可以通过高通量 测序技术获得,而表型数据则可以通过田间试验和室内分析等方法获得。
(2)作图:利用作图软件将基因型和表型数据组装成图,以展示它们之间的 关系。常用的作图软件包括QTL Cartographer、QTL IciMapping等。
原理
1、QTL的概念及定义
QTL是指作物基因组中控制数量性状的基因座位,它们可以通过影响表型变异 来影响作物的农艺性状。QTL通常分为两类:主效QTL和微效QTL。主效QTL是 指对表型变异起主要作用的QTL,而微效QTL则是指对表型变异起较小作用的 QTL。
QTL 定位方法---

QTL 定位方法分子标记技术和数量遗传学的发展,使得分子遗传学与数量遗传学相互渗透和融合,从而形成了一个新的研究领域—分子数量遗传学(Molecular Quantitative Genetics)。
分子数量遗传学研究的内容,就是借助分子标记,采用适当的统计分析方法明确QTL 在染色体上的位置及其效应。
而QTL 定位的原理是:利用适当的分离群体,构建较高密度的、分布较均匀的、覆盖全基因组的分子标记连锁图。
根据遗传连锁的基本遗传学原理,对分离群体中单株的标记基因型和性状的表型值进行一定的统计分析,将决定数量性状的QTL 定位在分子标记连锁图中。
目前,QTL 定位的方法主要有单标记分析法(Edwards et al, 1987),区间作图法(Lander and Botstein, 1989)和复合区间作图法(Zeng, 1994)等。
单标记法(Single marker analysis)是最简单的分析标记与性状关联的方法,包括以标记为基础的分析方法(Marker-based analysis, MBA)和以性状为基础的分析方法(Trait-based analysis, TBA,Lebowitz et al., 1987)。
前者利用每个标记位点不同基因型间的性状均值差异,以传统的单因素方差分析法测验被研究的数量性状在标记基因型间的差异显著性。
对于一个作图群体而言,任意标记位点具有三种基因型(F2群体)或两种基因型(回交群体、重组自交系、双单倍体系),分析每一基因型个体的数量性状均值的差异,并进行F 测验,当F 测验显著时,则表明该标记位点可能与一个或多个QTL 连锁。
利用这种方法进行的数量性状分析,既简单又符合QTL 定位的基本统计原理,且不需要完整的分子标记连锁图,是定位QTL 的最为有效方法。
但不足之处是不能准确估计QTL 的位置,且往往会低估其遗传效应。
以性状为基础的分析方法的原理是假定因选择而使数量性状的高表型个体中的QTL 增效等位基因和低表型个体中的QTL 减效等位基因的频率增加,当QTL 的等位基因与某一标记基因连锁时,会因相互关联而导致高、低表型个体间标记基因频率的差异。
精选F遗传连锁图谱和纤维品质性状QTL定位
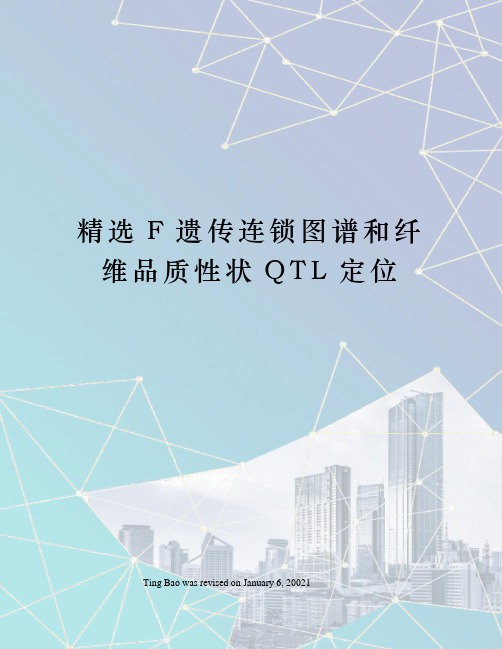
精选F遗传连锁图谱和纤维品质性状Q T L定位 Ting Bao was revised on January 6, 20021编号NO:河北农业大学本科毕业论文论文题目 BC1F2遗传连锁图谱和纤维品质性状QTL定位学生姓名唐冰川学号10213 成绩学院农学院专业班级农学0902 指导教师姓名张艳指导教师职称讲师材料目录:1、任务书(1)份2、开题报告(含文献综述)(1)份3、指导教师评阅书(1)份4、答辩记录表(1)份5、论文正文(1)份6、其它材料河北农业大学本科毕业论文任务书学院:农学院教师姓名:张艳职称:讲师2012 年 5 月 4 日农学院农学专业唐冰川学生:现把 2012-2013 学年,第二学期的毕业论文安排下达给你,你本学期承担的毕业论文任务如下:1、依据本任务书中论文题目、目的意义、可行性分析的内容完成开题报告。
2、按照开题报告的要求按期完成毕业论文各项工作的实施。
3、完成毕业论文的撰写。
4、完成毕业论文的答辩。
请按相关要求完成毕业论文任务。
教师签字:2012 年 5 月 3日河北农业大学本科毕业论文开题报告题目:BC1F2遗传连锁图谱和纤维品质性状QTL定位学院:农学院学生姓名:唐冰川专业:农学班级学号: 10213指导教师姓名:张艳指导教师职称:讲师2012年 6 月5 日河北农业大学本科毕业论文指导教师评阅书学生姓名:唐冰川学号:10213专业班级:农学0902 所在学院:农学院论文题目:BC1F2遗传连锁图谱构建和纤维品质性状QTL定位河北农业大学本科毕业论文答辩评分表河北农业大学2013届本科毕业论文答辩记录表所在学院:农学院专业班级:农学0902班时 2013年6月6日河北农业大学本科毕业论文(设计)题目:BC1F2遗传连锁图谱构建和纤维品质性状QTL定位学院:农学院专业班级:农学0902班学号: 10213学生姓名:唐冰川指导教师姓名:张艳指导教师职称:讲师二O一三年六月六日BC1F2遗传连锁图谱构建和纤维品质性状QTL定位唐冰川农学0902指导教师:张艳摘要:本研究利用海陆杂交BC1F2群体进行遗传连锁图谱构建,190对SSR引物共产生262个多态性位点,其中208个位点被定位到30个遗传连锁群。
分子标记辅助小麦抗赤霉病育种与抗(感)赤霉病QTL的定位

分子标记辅助小麦抗赤霉病育种与抗(感)赤霉病QTL的定位小麦赤霉病是世界温暖暖湿润地区的重要灾害性病害,其抗性是数量性状的遗传。
培育和种植抗病品种是防治小麦赤霉病发生的最经济有效措施。
本研究应用分子标记辅助选择技术和回交育种方法,以苏麦3号和望水白为赤霉病抗性基因Fhbl和Fhb2的供体亲本,对我国种植面积最大的两个弱筋小麦品种扬麦13和扬麦15进行赤霉病抗性改良。
在2007-2010年度采用田间自然发病和单花滴注接种赤霉病鉴定方法,以望水白×安农8455和苏麦3号×安农2号两个重组自交系群体为试验材料,进行抗(感)赤霉病侵染和扩展QTL的定位与作图;研究发现与赤霉病抗性相关的表型性状。
试验结果表明:1.应用Fhbl和Fhb2基因的分子标记辅助选择技术,对扬麦13和扬麦15的赤霉病抗性进行回交改良是有效的。
在同一组合中,同一回交次数的不同世代间抗性选择效率随着自交世代增加而提高,即在F4代选择高于F3代,F3代高于F2代;F3、F4代的病小穗率随着回交次数增加有上升趋势,抗病性比轮回亲本的降幅减小,赤霉病抗性降低。
育成了携带Fhb1和Fhb2基因的扬麦13和扬麦15抗赤霉病品系,与轮回亲本相比较,病小穗率降低了87.51%-91.82%和产量提高了17.24%-26.72%的R扬麦13-2、13-7、13-8,以及病小穗率降低了69.69%-90.36%和产量提高了11.34%-23.08%的R扬麦15-2、15-3、15-5、15-6、15-7,试验认为可以替代大面积生产上不抗赤霉病的扬麦13和扬麦15。
试验证实了3BS染色体上Fhb1是赤霉病抗性主要效应QTL,且Fhb1、Fhb2基因对赤霉病抗性具有加性效应。
在扬麦13和扬麦15的背景中导入Fhb1基因,病小穗率降幅达44.66%以上;导入Fhb1和Fhb2基因,病小穗率比含Fhb1基因的又降低了14.86%-55.06%,抗性基因聚合可进一步提高赤霉病抗性水平。
- 1、下载文档前请自行甄别文档内容的完整性,平台不提供额外的编辑、内容补充、找答案等附加服务。
- 2、"仅部分预览"的文档,不可在线预览部分如存在完整性等问题,可反馈申请退款(可完整预览的文档不适用该条件!)。
- 3、如文档侵犯您的权益,请联系客服反馈,我们会尽快为您处理(人工客服工作时间:9:00-18:30)。
Euphytica(2005)142:169–196DOI:10.1007/s10681-005-1681-5C Springer2005 An introduction to markers,quantitative trait loci(QTL)mapping and marker-assisted selection for crop improvement:The basic conceptsB.C.Y.Collard1,4,∗,M.Z.Z.Jahufer2,J.B.Brouwer3&E.C.K.Pang11Department of Biotechnology and Environmental Biology,RMIT University,P.O.Box71,Bundoora,Victoria3083, Australia;2AgResearch Ltd.,Grasslands Research Centre,Tennent Drive,Private Bag11008,Palmerston North, New Zealand;3P.O.Box910,Horsham,Victoria,Australia3402;4Present address:Plant Breeding,Genetics and Biotechnology Division,International Rice Research Institute(IRRI),DAPO Box7777,Metro Manila,Philippines; (∗author for correspondence:e-mail:bcycollard@)Received11July2004;accepted2February2005Key words:bulked-segregant analysis,DNA markers,linkage map,marker-assisted selection,quantitative trait loci (QTLs),QTL analysis,QTL mappingSummaryRecognizing the enormous potential of DNA markers in plant breeding,many agricultural research centers and plant breeding institutes have adopted the capacity for marker development and marker-assisted selection(MAS). However,due to rapid developments in marker technology,statistical methodology for identifying quantitative trait loci(QTLs)and the jargon used by molecular biologists,the utility of DNA markers in plant breeding may not be clearly understood by non-molecular biologists.This review provides an introduction to DNA markers and the concept of polymorphism,linkage analysis and map construction,the principles of QTL analysis and how markers may be applied in breeding programs using MAS.This review has been specifically written for readers who have only a basic knowledge of molecular biology and/or plant genetics.Its format is therefore ideal for conventional plant breeders,physiologists,pathologists,other plant scientists and students.Abbreviations:AFLP:amplified fragment length polymorphism;BC:backcross;BSA:bulked-segregant analysis; CIM:composite interval mapping;cM:centiMorgan;DH:doubled haploid;EST:expressed sequence tag;SIM:sim-ple interval mapping;LOD:logarithm of odds;LRS:likelihood ratio statistic;MAS:marker-assisted selection;NIL: near isogenic lines;PCR:polymerase chain reaction;QTL:quantitative trait loci;RAPD:random amplified poly-morphic DNA;RI:recombinant inbred;RFLP:restriction fragment length polymorphism;SSR:simple sequence repeats(microsatellites);SCAR:sequence characterized amplified region;SNP:single nucleotide polymorphism; STS:sequence tagged siteIntroductionMany agriculturally important traits such as yield,qual-ity and some forms of disease resistance are controlled by many genes and are known as quantitative traits(also ‘polygenic,’‘multifactorial’or‘complex’traits).The regions within genomes that contain genes associated with a particular quantitative trait are known as quan-titative trait loci(QTLs).The identification of QTLs based only on conventional phenotypic evaluation is not possible.A major breakthrough in the characteri-zation of quantitative traits that created opportunities to select for QTLs was initiated by the development of DNA(or molecular)markers in the1980s.One of the main uses of DNA markers in agricul-tural research has been in the construction of linkage maps for diverse crop species.Linkage maps have been utilised for identifying chromosomal regions that contain genes controlling simple traits(controlled by a single gene)and quantitative traits using QTL170analysis(reviewed by Mohan et al.,1997).The process of constructing linkage maps and conducting QTL analysis–to identify genomic regions associated with traits–is known as QTL mapping(also‘genetic,’‘gene’or‘genome’mapping)(McCouch&Doerge,1995; Mohan et al.,1997;Paterson,1996a,b).DNA markers that are tightly linked to agronomically important genes (called gene‘tagging’)may be used as molecular tools for marker-assisted selection(MAS)in plant breeding (Ribaut&Hoisington,1998).MAS involves using the presence/absence of a marker as a substitute for or to as-sist in phenotypic selection,in a way which may make it more efficient,effective,reliable and cost-effective compared to the more conventional plant breeding methodology.The use of DNA markers in plant(and animal)breeding has opened a new realm in agriculture called‘molecular breeding’(Rafalski&Tingey,1993).DNA markers are widely accepted as potentially valuable tools for crop improvement in rice(Mackill et al.,1999;McCouch&Doerge,1995),wheat(Eagles et al.,2001;Koebner&Summers,2003;Van Sanford et al.,2001),maize(Stuber et al.,1999;Tuberosa et al., 2003),barley(Thomas,2003;Williams,2003),tuber crops(Barone,2004;Fregene et al.,2001;Gebhardt &Valkonen,2001),pulses(Kelly et al.,2003; Muehlbauer et al.,1994;Svetleva et al.,2003;Weeden et al.,1994),oilseeds(Snowdon&Friedt,2004), horticultural crop species(Baird et al.,1996,1997; Mehlenbacher,1995)and pasture species(Jahufer et al.,2002).Some studies suggest that DNA markers will play a vital role in enhancing global food produc-tion by improving the efficiency of conventional plant breeding programs(Kasha,1999;Ortiz,1998).Al-though there has been some concern that the outcomes of DNA marker technology as proposed by initial stud-ies may not be as effective asfirst thought,many plant breeding institutions have adopted the capacity for marker development and/or MAS(Eagles et al.,2001; Kelly&Miklas,1998;Lee,1995).An understanding of the basic concepts and methodology of DNA marker development and MAS,including some of the termi-nology used by molecular biologists,will enable plant breeders and researchers working in other relevant disciplines to work together towards a common goal –increasing the efficiency of global food production.A number of excellent reviews have been written about the construction of linkage maps,QTL analy-sis and the application of markers in marker-assisted selection(for example:Haley&Andersson,1997; Jones et al.,1997;Paterson et al.,1991a;Paterson, 1996a,b;Staub et al.,1996;Tanksley,1993;Young,1994).However,the authors of these reviews assumed that the reader had an advanced level of knowledge in molecular biology and plant genetics,with the possi-ble exceptions of the reviews by Paterson(1996a,b) and Jones et al.(1997).Our review has been specifi-cally written for readers with only a basic knowledge of molecular biology and/or plant genetics.It will be a useful reference for conventional plant breeders,physi-ologists,pathologists and other plant scientists,as well as students who are not necessarily engaged in applied molecular biology research but need an understanding of the exciting opportunities offered by this new tech-nology.This review consists offive sections:genetic markers,construction of linkage maps,QTL analysis, towards marker-assisted selection and marker-assisted selection.Section I:Genetic markersWhat are genetic markers?Genetic markers represent genetic differences between individual organisms or species.Generally,they do not represent the target genes themselves but act as‘signs’or‘flags’.Genetic markers that are located in close proximity to genes(i.e.tightly linked)may be referred to as gene‘tags’.Such markers themselves do not affect the phenotype of the trait of interest because they are located only near or‘linked’to genes controlling the trait.All genetic markers occupy specific genomic po-sitions within chromosomes(like genes)called‘loci’(singular‘locus’).There are three major types of genetic markers: (1)morphological(also‘classical’or‘visible’)mark-ers which themselves are phenotypic traits or charac-ters;(2)biochemical markers,which include allelic variants of enzymes called isozymes;and(3)DNA (or molecular)markers,which reveal sites of varia-tion in DNA(Jones et al.,1997;Winter&Kahl,1995). Morphological markers are usually visually character-ized phenotypic characters such asflower colour,seed shape,growth habits or pigmentation.Isozyme mark-ers are differences in enzymes that are detected by electrophoresis and specific staining.The major dis-advantages of morphological and biochemical mark-ers are that they may be limited in number and are influenced by environmental factors or the develop-mental stage of the plant(Winter&Kahl,1995). However,despite these limitations,morphological and biochemical markers have been extremely useful to171plant breeders(Eagles et al.,2001;Weeden et al., 1994).DNA markers are the most widely used type of marker predominantly due to their abundance.They arise from different classes of DNA mutations such as substitution mutations(point mutations),rearrange-ments(insertions or deletions)or errors in replication of tandemly repeated DNA(Paterson,1996a).These markers are selectively neutral because they are usu-ally located in non-coding regions of DNA.Unlike morphological and biochemical markers,DNA mark-ers are practically unlimited in number and are not af-fected by environmental factors and/or the develop-mental stage of the plant(Winter&Kahl,1995).Apart from the use of DNA markers in the construction of linkage maps,they have numerous applications in plant breeding such as assessing the level of genetic diver-sity within germplasm and cultivar identity(Baird et al., 1997;Henry,1997;Jahufer et al.,2003;Weising et al., 1995;Winter&Kahl,1995).DNA markers may be broadly divided into three classes based on the method of their detection:(1) hybridization-based;(2)polymerase chain reaction (PCR)-based and(3)DNA sequence-based(Gupta et al.,1999;Jones et al.,1997;Joshi et al.,1999;Win-ter&Kahl,1995).Essentially,DNA markers may re-veal genetic differences that can be visualised by us-ing a technique called gel electrophoresis and stain-ing with chemicals(ethidium bromide or silver)or detection with radioactive or colourimetric probes. DNA markers are particularly useful if they reveal differences between individuals of the same or dif-ferent species.These markers are called polymorphic markers,whereas markers that do not discriminate between genotypes are called monomorphic markers (Figure1).Polymorphic markers may also be de-scribed as codominant or dominant.This description is based on whether markers can discriminate between homozygotes and heterozygotes(Figure2).Codomi-nant markers indicate differences in size whereas dom-inant markers are either present or absent.Strictly speaking,the different forms of a DNA marker(e.g. different sized bands on gels)are called marker‘al-leles’.Codominant markers may have many differ-ent alleles whereas a dominant marker only has two alleles.It is beyond the scope of this review to discuss the technical method of how DNA markers are gen-erated.However the advantages and disadvantages of the most commonly used markers are presented in Table1.Figure1.Diagram representing hypothetical DNA markers between genotypes A,B,C and D.Polymorphic markers are indicated by ar-rows.Markers that do not discriminate between genotypes are called monomorphic markers.(a)Example of SSR markers.The polymor-phic marker reveals size differences for the marker alleles of the four genotypes,and represent a single genetic locus.(b)Examples of markers generated by the RAPD technique.Note that these mark-ers are either present or absent.Often,the sizes of these markers in nucleotide base pairs(bp)are also provided;these sizes are estimated from a molecular weight(MW)DNA ladder.For both polymorphic markers,there are only two different marker alleles.Section II:Construction of linkage mapsWhat are linkage maps?A linkage map may be thought of as a‘road map’of the chromosomes derived from two different parents(Pa-terson,1996a).Linkage maps indicate the position and relative genetic distances between markers along chro-mosomes,which is analogous to signs or landmarks along a highway.The most important use for linkage maps is to identify chromosomal locations contain-ing genes and QTLs associated with traits of interest; such maps may then be referred to as‘QTL’(or‘ge-netic’)maps.‘QTL mapping’is based on the principle that genes and markers segregate via chromosome re-combination(called crossing-over)during meiosis(i.e. sexual reproduction),thus allowing their analysis in the progeny(Paterson,1996a).Genes or markers that are close together or tightly-linked will be transmitted172Table 1.Advantages and disadvantages of most commonly-used DNA markers for QTL analysis Molecular Codominant (C)markeror Dominant (D)Advantages DisadvantagesReferencesRestrictionC•Robust •Time-consuming,laborious Beckmann &Soller (1986),fragment length •Reliableand expensiveKochert (1994),Tanksley polymorphism •Transferable across •Large amounts of DNA required et al.(1989)(RFLP)populations•Limited polymorphism (especially in related lines)Random D•Quick and simple •Problems with reproducibility Penner (1996),Welsh &lified •Inexpensive•Generally not transferableMcClelland (1990),polymorphic •Multiple loci from a single Williams et al.(1990)DNA (RAPD)primer possible •Small amounts of DNA required Simple sequence C•Technically simple •Large amounts of time and McCouch et al.(1997),repeats (SSRs)∗•Robust and reliable labour required for production Powell et al.(1996),or ‘microsatellites’•Transferable between of primers Taramino &Tingey (1996)populations •Usually require polyacrylamideelectrophoresis AmplifiedD•Multiple loci •Large amounts of DNA required V os et al.(1995)fragment Length•High levels of•Complicated methodologyPolymorphism (AFLP)polymorphism generated∗SSRsare also known as sequence tagged microsatellite site (STMS)markers (Davierwala et al.,2000;Huettel et al.,1999;Mohapatra et al.,2003;Winter et al.,1999).Figure parison between (a)codominant and (b)dominant markers.Codominant markers can clearly discriminate between homozygotes and heterozygotes whereas dominant markers do not.Genotypes at two marker loci (A and B)are indicated below the gel diagrams.together from parent to progeny more frequently than genes or markers that are located further apart (Fig-ure 3).In a segregating population,there is a mixture of parental and recombinant genotypes.The frequency of recombinant genotypes can be used to calculate re-combination fractions,which may by used to infer the genetic distance between markers.By analysing the segregation of markers,the relative order and dis-tances between markers can be determined–the lower the frequency of recombination between two markers,the closer they are situated on a chromosome (con-versely,the higher the frequency of recombination be-tween two markers,the further away they are situated on a chromosome).Markers that have a recombination frequency of 50%are described as ‘unlinked’and as-sumed to be located far apart on the same chromosome or on different chromosomes.For a more detailed ex-planation of genetic linkage,the reader is encouraged to consult basic textbooks on genetics or quantitative genetics (for example,Hartl &Jones,2001;Kearsey &Pooni,1996).Mapping functions are used to convert recombination fractions into map units called centi-Morgans (cM)(discussed later).Linkage maps are con-structed from the analysis of many segregating mark-ers.The three main steps of linkage map construction are:(1)production of a mapping population;(2)iden-tification of polymorphism and (3)linkage analysis of markers.Mapping populationsThe construction of a linkage map requires a segregat-ing plant population (i.e.a population derived from sex-ual reproduction).The parents selected for the mapping population will differ for one or more traits of interest.Population sizes used in preliminary genetic mapping studies generally range from 50to 250individuals173 Figure3.Diagram indicating cross-over or recombination events between homologous chromosomes that occur during meiosis.Gametes that are produced after meiosis are either parental(P)or recombinant(R).The smaller the distance between two markers,the smaller the chance of recombination occurring between the two markers.Therefore,recombination between markers G and H should occur more frequently than recombination between markers E and F.This can be observed in a segregating mapping population.By analysing the number of recombinants in a population,it could be determined that markers E and F are closer together compared to G and H.(Mohan et al.,1997),however larger populations arerequired for high-resolution mapping.If the map willbe used for QTL studies(which is usually the case),then an important point to note is that the mapping pop-ulation must be phenotypically evaluated(i.e.trait datamust be collected)before subsequent QTL mapping.Generally in self-pollinating species,mapping pop-ulations originate from parents that are both highly ho-mozygous(inbred).In cross pollinating species,the sit-uation is more complicated since most of these speciesdo not tolerate inbreeding.Many cross pollinating plantspecies are also polyploid(contain several sets of chro-mosome pairs).Mapping populations used for mappingcross pollinating species may be derived from a crossbetween a heterozygous parent and a haploid or ho-mozygous parent(Wu et al.,1992).For example,inboth the cross pollinating species white clover(Tri-folium repens L.)and ryegrass(Lolium perenne L.),F1generation mapping populations were successfully de-veloped by pair crossing heterozygous parental plantsthat were distinctly different for important traits asso-ciated with plant persistence and seed yield(Barrettet al.,2004;Forster et al.,2000).Several different populations may be utilizedfor mapping within a given plant species,with eachpopulation type possessing advantages and disadvan-tages(McCouch&Doerge,1995;Paterson,1996a)(Figure4).F2populations,derived from F1hybrids,and backcross(BC)populations,derived by crossingthe F1hybrid to one of the parents,are the simplesttypes of mapping populations developed for selfpollinating species.Their main advantages are thatthey are easy to construct and require only a shorttime to produce.Inbreeding from individual F2plantsallows the construction of recombinant inbred(RI)lines,which consist of a series of homozygous lines,each containing a unique combination of chromosomalsegments from the original parents.The length oftime needed for producing RI populations is the majordisadvantage,because usually six to eight generationsare required.Doubled haploid(DH)populations maybe produced by regenerating plants by the inductionof chromosome doubling from pollen grains,however,the production of DH populations is only possible inspecies that are amenable to tissue culture(e.g.cerealspecies such as rice,barley and wheat).The majoradvantages of RI and DH populations are that theyproduce homozygous or‘true-breeding’lines that canbe multiplied and reproduced without genetic changeoccurring.This allows for the conduct of replicated174Figure4.Diagram of main types of mapping populations for self-pollinating species.trials across different locations and years.Thus both RI and DH populations represent‘eternal’resources for QTL mapping.Furthermore,seed from individual RI or DH lines may be transferred between different laboratories for further linkage analysis and the addition of markers to existing maps,ensuring that all collaborators examine identical material(Paterson, 1996a;Young,1994).Identification of polymorphismThe second step in the construction of a linkage map is to identify DNA markers that reveal differences be-tween parents(i.e.polymorphic markers).It is critical that sufficient polymorphism exists between parents in order to construct a linkage map(Young,1994).In gen-eral,cross pollinating species possess higher levels of DNA polymorphism compared to inbreeding species; mapping in inbreeding species generally requires the selection of parents that are distantly related.In many cases,parents that provide adequate polymorphism are selected on the basis of the level of genetic diversity between parents(Anderson et al.,1993;Collard et al., 2003;Joshi&Nguyen,1993;Yu&Nguyen,1994).The choice of DNA markers used for mapping may de-pend on the availability of characterised markers or the appropriateness of particular markers for a particular species.Once polymorphic markers have been identified, they must be screened across the entire mapping pop-ulation,including the parents(and F1hybrid,if pos-sible).This is known as marker‘genotyping’of the population.Therefore,DNA must be extracted from each individual of the mapping population when DNA markers are used.Examples of DNA markers screened across different populations are shown in Figure5.The expected segregation ratios for codominant and domi-nant markers are presented in Table2.Significant de-viations from expected ratios can be analysed using Table2.Expected segregation ratios for markers in different popu-lation typesPopulation type Codominant markers Dominant markersF21:2:1(AA:Aa:aa)3:1(B:bb) Backcross1:1(Cc:cc)1:1(Dd:dd) Recombinant inbred or1:1(EE:ee)1:1(FF:ff)doubled haploid175 Figure5.Hypothetical gel photos representing segregating codominant markers(left-hand side)and dominant markers(right-hand side)for typical mapping populations.Codominant markers indicate the complete genotype of a plant.Note that dominant markers cannot discriminate between heterozygotes and one homozygote genotype in F2populations.The segregation ratios of markers can be easily understood by using Punnett squares to derive population genotypes.chi-square tests.Generally,markers will segregate in a Mendelian fashion although distorted segregation ra-tios may be encountered(Sayed et al.,2002;Xu et al., 1997).In some polyploid species such as sugarcane, identifying polymorphic markers is more complicated (Ripol et al.,1999).The mapping of diploid relatives of polyploid species can be of great benefit in developing maps for polyploid species.However,diploid relatives do not exist for all polyploid species(Ripol et al.,1999; Wu et al.,1992).A general method for the mapping of polyploid species is based on the use of single-dose restriction fragments(Wu et al.,1992).Linkage analysis of markersThefinal step of the construction of a linkage map in-volves coding data for each DNA marker on each indi-vidual of a population and conducting linkage anal-ysis using computer programs(Figure6).Missing marker data can also be accepted by mapping programs.Although linkage analysis can be performed manu-ally for a few markers,it is not feasible to manually analyze and determine linkages between large numbers of markers that are used to construct maps;computer programs are required for this purpose.Linkage be-tween markers is usually calculated using odds ratios (i.e.the ratio of linkage versus no linkage).This ratio is more conveniently expressed as the logarithm of the ratio,and is called a logarithm of odds(LOD)value or LOD score(Risch,1992).LOD values of>3are typi-cally used to construct linkage maps.A LOD value of 3between two markers indicates that linkage is1000 times more likely(i.e.1000:1)than no linkage(null hypothesis).LOD values may be lowered in order to detect a greater level of linkage or to place additional markers within maps constructed at higher LOD monly used software programs include Map-maker/EXP(Lander et al.,1987;Lincoln et al.,1993a) and MapManager QTX(Manly et al.,2001),which are freely available from the internet.JoinMap is an-other commonly-used program for constructing link-age maps(Stam,1993).176Figure6.Construction of a linkage map based on a small recombinant inbred population(20individuals).Thefirst parent(P1)is scored as an ‘A’whereas the second parent(P2)is scored as a‘B’.Coding of marker data varies depending on the type of population used.This linkage map was constructed using Map Manager QTX(Manly et al.,2001)using the Haldane mapping function.A typical output of a linkage map is shown in Figure7.Linked markers are grouped together into‘linkage groups,’which represent chromosomal segments or entire chromosomes.Referring to the road map analogy,linkage groups represent roads and markers represent signs or landmarks.A difficulty associated with obtaining an equal number of linkage groups and chromosomes is that the polymorphic markers detected are not necessarily evenly distributed over the chromosome,but clustered in some regions and absent in others(Paterson,1996a).In addition to the non-random distribution of markers,the frequency of recombination is not equal along chromosomes (Hartl&Jones,2001;Young,1994).The accuracy of measuring the genetic distance and determining marker order is directly related to the number of individuals studied in the mapping popu-lation.Ideally,mapping populations should consist of177 Figure7.Hypothetical‘framework’linkage map offive chromosomes(represented by linkage groups)and26markers.Ideally,a framework map should consist of evenly spaced markers for subsequent QTL analysis.If possible,the framework map should also consist of anchor markers that are present in several maps,so that they can be used to compare regions between maps.a minimum of50individuals for constructing linkage maps(Young,1994).Genetic distance and mapping functionsThe importance of the distance between genes and markers has been discussed earlier.The greater the distance between markers,the greater the chance of re-combination occurring during meiosis.Distance along a linkage map is measured in terms of the frequency of recombination between genetic markers(Paterson, 1996a).Mapping functions are required to convert recombination fractions into centiMorgans(cM)be-cause recombination frequency and the frequency of crossing-over are not linearly related(Hartl&Jones, 2001;Kearsey&Pooni,1996).When map distances are small(<10cM),the map distance equals the recombi-nation frequency.However,this relationship does not apply for map distances that are greater than10cM (Hartl&Jones,2001).Two commonly used mapping functions are the Kosambi mapping function,which assumes that recombination events influence the oc-currence of adjacent recombination events,and the Haldane mapping function,which assumes no interfer-ence between crossover events(Hartl&Jones,2001; Kearsey&Pooni,1996).It should be noted that distance on a linkage map is not directly related to the physical distance of DNA be-tween genetic markers,but depends on the genome size of the plant species(Paterson,1996a).Furthermore, the relationship between genetic and physical dis-tance varies along a chromosome(Kunzel et al.,2000; Tanksley et al.,1992;Young,1994).For example,there are recombination‘hot spots’and‘cold spots,’which are chromosomal regions in which recombination oc-curs more frequently or less frequently,respectively (Faris et al.,2000;Ma et al.,2001;Yao et al.,2002). Section III:QTL analysisPrinciple of QTL analysisIdentifying a gene or QTL within a plant genome is likefinding the proverbial needle in a haystack.How-ever,QTL analysis can be used to divide the haystack178in manageable piles and systematically search them.In simple terms,QTL analysis is based on the principle of detecting an association between phenotype and the genotype of markers.Markers are used to parti-tion the mapping population into different genotypic groups based on the presence or absence of a particu-lar marker locus and to determine whether significant differences exist between groups with respect to the trait being measured (Tanksley,1993;Young,1996).A significant difference between phenotypic means of the groups (either 2or 3),depending on the marker sys-tem and type of population,indicates that the marker locus being used to partition the mapping population is linked to a QTL controlling the trait (Figure 8).A logical question that may be asked at this point is ‘why does a significant P value obtained for differences between mean trait values indicate linkage between marker and QTL?’The answer is due to recombination.The closer a marker is from a QTL,the lower the chance of recombination occurring between marker and QTL.Therefore,the QTL and marker will be usually be inher-ited together in the progeny,and the mean of the group with the tightly-linked marker will be significantlydif-Figure 8.Principle of QTL mapping (adapted from Young,1996).Markers that are linked to a gene or QTL controlling a particular trait (e.g.plant height)will indicate significant differences when the mapping population is partitioned according to the genotype of the marker.Based on the results in this diagram,Marker E is linked to a QTL because there is a significant difference between means.Marker H is unlinked to a QTL because there is no significant difference between means.The closer the marker is to the QTL of interest,the lower the chance for recombination between marker and QTL.ferent (P <0.05)to the mean of the group without the marker (Figure 9).When a marker is loosely-linked or unlinked to a QTL,there is independent segregation of the marker and QTL.In this situation,there will be no significant difference between means of the genotype groups based on the presence or absence of the loosely-linked marker (Figure 9).Unlinked markers located far apart or on different chromosomes to the QTL are ran-domly inherited with the QTL;therefore,no significant differences between means of the genotype groups will be detected.Methods to detect QTLsThree widely-used methods for detecting QTLs are single-marker analysis,simple interval mapping and composite interval mapping (Liu,1998;Tanksley,1993).Single-marker analysis (also ‘single-point anal-ysis’)is the simplest method for detecting QTLs as-sociated with single markers.The statistical methods used for single-marker analysis include t -tests,analysis of variance (ANOV A)and linear regression.Linear。