(完整版)高维数据可视化
(完整版)可视化方法与技术

可视化方法与技术计算机系统在各领域中的广泛应用导致海量数据的产生,数据处理能力的滞后迫切需要研究和开发新的信息处理技术和方法。
基于此,海量、异构、时变、多维数据的可视化表示和分析在各领域中日益受到重视并得到越来越广泛的应用.一、可视化概述测量的自动化、网络传输过程的数字化和大量的计算机仿真产生了海量数据,超出了人类分析处理的能力.可视化提供了解决这种问题的一种新工具。
一般意义下的可视化定义为:可视化是一种使复杂信息能够容易和快速被人理解的手段,是一种聚焦在信息重要特征的信息压缩语言,是可以放大人类感知的图形化表示方法。
可视化就是把数据、信息和知识转化为可视的表示形式并获得对数据更深层次认识的过程。
可视化作为一种可以放大人类感知的数据、信息、知识的表示方法,日益受到重视并得到越来越广泛的应用。
可视化可以应用到简单问题,也可以应用到复杂系统状态表示,从可视化的表示中人们可以发现新的线索、新的关联、新的结构、新的知识,促进人机系统的结合,促进科学决策。
可视化充分利用计算机图形学、图像处理、用户界面、人机交互等技术,形象、直观地显示科学计算的中间结果和最终结果并进行交互处理。
可视化技术以人们惯于接受的表格、图形、图像等方法并辅以信息处理技术将客观事物及其内在的联系进行表现,可视化结果便于人们记忆和理解。
可视化为人类与计算机这两个信息处理系统之间提供了一个接口。
可视化对于信息的处理和表达方式有其它方式无法取代的优势,其特点可总结为可视性、交互性和多维性。
二、可视化技术目前,可视化技术包括数据可视化、科学计算可视化、信息可视化和知识可视化等,这些概念及应用存在着区别、交叉和联系.(一)数据可视化数据可视化技术指的是运用计算机图形学和图像处理技术,将数据转换为图形或图像在屏幕上显示出来,并进行交互处理的理论、方法和技术。
数据可视化的重点是将多维数据在二维或三维空间内显示,这对初步的数据分类理解是有意义的。
针对于此,产生了许多数据可视化的技术,大体分为散点矩阵法、投影矩阵法、平行坐标法、面向象素的可视化技术、层次技术、动态技术、图标表示技术、直方图法及一些几何学技术等等。
Umatrix高维数据结构可视化工具说明书
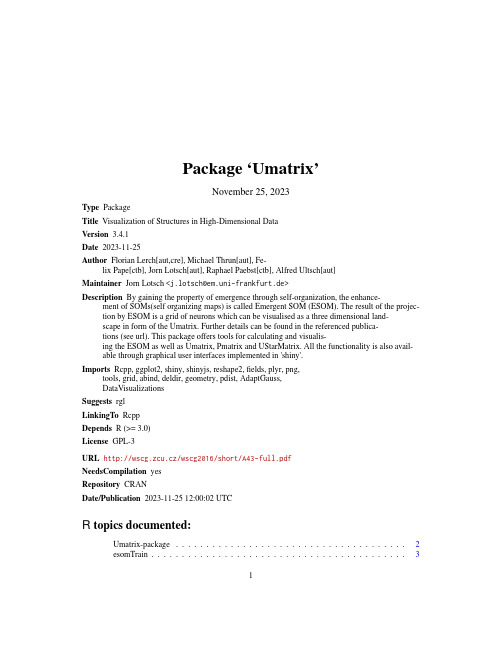
Package‘Umatrix’November25,2023Type PackageTitle Visualization of Structures in High-Dimensional DataVersion3.4.1Date2023-11-25Author Florian Lerch[aut,cre],Michael Thrun[aut],Fe-lix Pape[ctb],Jorn Lotsch[aut],Raphael Paebst[ctb],Alfred Ultsch[aut]Maintainer Jorn Lotsch<*************************.de>Description By gaining the property of emergence through self-organization,the enhance-ment of SOMs(self organizing maps)is called Emergent SOM(ESOM).The result of the projec-tion by ESOM is a grid of neurons which can be visualised as a three dimensional land-scape in form of the Umatrix.Further details can be found in the referenced publica-tions(see url).This package offers tools for calculating and visualis-ing the ESOM as well as Umatrix,Pmatrix and UStarMatrix.All the functionality is also avail-able through graphical user interfaces implemented in'shiny'.Imports Rcpp,ggplot2,shiny,shinyjs,reshape2,fields,plyr,png,tools,grid,abind,deldir,geometry,pdist,AdaptGauss,DataVisualizationsSuggests rglLinkingTo RcppDepends R(>=3.0)License GPL-3URL http://wscg.zcu.cz/wscg2016/short/A43-full.pdfNeedsCompilation yesRepository CRANDate/Publication2023-11-2512:00:02UTCR topics documented:Umatrix-package (2)esomTrain (3)12Umatrix-package Hepta (4)iClassification (5)iEsomTrain (6)iUmapIsland (7)iUstarmatrix (7)plotMatrix (8)pmatrixForEsom (10)showMatrix3D (11)umatrixForEsom (12)ustarmatrixCalc (12)Index14 Umatrix-package Umatrix-packageDescriptionThe ESOM(emergent self organizing map)is an improvement of the regular SOM(self organizing map)which allows for toroid grids of neurons and is intended to be used in combination with the Umatrix.The set of neurons is referred to as weights within this package,as they represent the values within the high dimensional space.The neuron with smallest distance to a datapoint is calleda Bestmatch and can be considered as projection of said datapoint.As the Umatrix is usually toroid,it is drawn four consecutive times to remove border effects.An island,or Imx,is afilter mask,which cuts out a subset of the Umatrix,which shows every point only a single time while avoiding border effects cutting through potential clusters.Finally the Pmatrix shows the density structures within the grid,by a set radius.It can be combined with the Umatrix resulting in the UStarMatrix,which is therefore a combination of density based structures as well as clearly divided ones. ReferencesUltsch,A.:Data mining and knowledge discovery with emergent self-organizing feature maps for multivariate time series,In Oja,E.&Kaski,S.(Eds.),Kohonen maps,(1ed.,pp.33-46),Elsevier, 1999.Ultsch,A.:Maps for the visualization of high-dimensional data spaces,Proc.Workshop on Self organizing Maps(WSOM),pp.225-230,Kyushu,Japan,2003.Ultsch,A.:U*C:Self-organized Clustering with Emergent Feature Maps,Lernen,Wissensent-deckung und Adaptivitaet(LWA),pp.240-244,Saarbruecken,Germany,2005.Loetsch,J.,Ultsch,A.:Exploiting the Structures of the U-Matrix,in Villmann,T.,Schleif,F.-M., Kaden,M.&Lange,M.(eds.),Proc.Advances in Self-Organizing Maps and Learning Vector Quantization,pp.249-257,Springer International Publishing,Mittweida,Germany,2014.Ultsch,A.,Behnisch,M.,Loetsch,J.:ESOM Visualizations for Quality Assessment in Clustering, In Merenyi,E.,Mendenhall,J.M.&O’Driscoll,P.(Eds.),Advances in Self-Organizing Maps and Learning Vector Quantization:Proceedings of the11th International Workshop WSOM2016,pp.39-48,Houston,Texas,USA,January6-8,2016,(10.1007/978-3-319-28518-4_3),Cham,Springer International Publishing,2016.esomTrain3 Thrun,M.C.,Lerch,F.,Loetsch,J.,Ultsch,A.:Visualization and3D Printing of Multivariate Data of Biomarkers,in Skala,V.(Ed.),International Conference in Central Europe on Computer Graphics,Visualization and Computer Vision,Plzen,2016.esomTrain Train an ESOM(emergent self organizing map)and project dataDescriptionThe ESOM(emergent self organizing map)algorithm as defined by[Ultsch1999].A set of weigths(neurons)on a two-dimensional grid get trained to adapt the given datastructure.The weights will be used to project data on a two-dimensional space,by seeking the BestMatches for every datapoint.ArgumentsData Data that will be used for training and projectionLines Height of gridColumns Width of gridEpochs Number of Epochs the ESOM will runToroid If TRUE,the grid will be toroidNeighbourhoodFunctionType of Neighbourhood;Possible values are:"cone","mexicanhat"and"gauss"StartLearningRateInitial value for LearningRateEndLearningRateFinal value for LearningRateStartRadius Start value for the Radius in which will be searched for neighboursEndRadius End value for the Radius in which will be searched for neighboursNeighbourhoodCoolingCooling method for radius;"linear"is the only available option at the moment LearningRateCoolingCooling method for LearningRate;"linear"is the only available option at themomentshinyProgress Generate progress output for shiny if Progress Object is givenShiftToHighestDensityIf True,the Umatrix will be shifted so that the point with highest density will beat the centerInitMethod name of the method that will be used to choose initializations Valid Inputs: "uni_min_max":uniform distribution with minimum and maximum from sam-pleData"norm_mean_2std":normal distribuation based on mean and standarddeviation of sampleDataKey Vector of numeric keys matching the datapoints.Will be added to Bestmatches UmatrixForEsom If TRUE,Umatrix based on resulting ESOM is calculated and returned4HeptaDetailsOn a toroid grid,opposing borders are connected.ValueList withBestMatches BestMatches of datapointsWeights Trained weightsLines Height of gridColumns Width of gridToroid TRUE if grid is a toroidReferencesKohonen,T.,Self-organized formation of topologically correct feature maps.Biological cybernet-ics,1982.43(1):p.59-69.Ultsch,A.,Data mining and knowledge discovery with emergent self-organizing feature maps for multivariate time series.Kohonen maps,1999.46:p.33-46.Examplesdata( Hepta )res=esomTrain(Hepta$Data,Key=1:nrow(Hepta$Data))Hepta Hepta from FCPS(Fundamental Clustering Problem Suite)DescriptionDataset with7easily seperable classes.Usagedata("Hepta")DetailsSize212,Dimensions3,stored in Hepta$DataClasses7,stored in Hepta$ClsReferencesUltsch,A.:U*C:Self-organized Clustering with Emergent Feature Maps,Lernen,Wissensent-deckung und Adaptivitaet(LW A),pp.240-244,Saarbruecken,Germany,2005.iClassification5 Examplesdata(Hepta)str(Hepta)iClassification GUI for manual classificationDescriptionThis tool is a’shiny’GUI that visualizes a given Umatrix and allows the user to select areas and mark them as clusters.ArgumentsUmatrix Matrix of Umatrix HeightsBestMatches Array with positions of BestmatchesCls Classification of the BestmatchesImx Matrix of an island that will be cut out of the UmatrixToroid Are BestMatches placed on a toroid grid?TRUE by defaultValueA vector containing the selected class ids.The order is corresponding to the given BestmatchesReferencesThrun,M.C.,Lerch,F.,Loetsch,J.,Ultsch,A.:Visualization and3D Printing of Multivariate Data of Biomarkers,in Skala,V.(Ed.),International Conference in Central Europe on Computer Graphics,Visualization and Computer Vision,Plzen,2016.Examples##Not run:data("Hepta")e=esomTrain(Hepta$Data,Key=1:nrow(Hepta$Data))cls=iClassification(e$Umatrix,e$BestMatches)##End(Not run)6iEsomTrain iEsomTrain iEsomTrainDescriptionTrains the ESOM and shows the Umatrix.ArgumentsData Matrix of Data that will be used to learn.One DataPoint per rowBestMatches Array with positions of BestmatchesCls Classification of the Bestmatches as a vectorKey Numeric vector of keys matching the BestmatchesToroid Are BestMatches placed on a toroid grid?TRUE by defaultValueList withUmatrix matrix with height values of the umatrixBestMatches matrix containing the bestmatchesLines number of lines of the chosen ESOMColumns number of columns of the chosen ESOMEpochs number of epochs of the chosen ESOMWeights List of weightsToroid True if a toroid grid was usedEsomDetails Further details describing the chosen ESOM parametersReferencesThrun,M.C.,Lerch,F.,Loetsch,J.,Ultsch,A.:Visualization and3D Printing of Multivariate Data of Biomarkers,in Skala,V.(Ed.),International Conference in Central Europe on Computer Graphics,Visualization and Computer Vision,Plzen,2016.iUmapIsland7 iUmapIsland iUmapIslandDescriptionThe toroid Umatrix is usually drawn4times,so that connected areas on borders can be seen asa whole.An island is a manual cutout of such a tiled visualization,that is selected such that allconnected areas stay intact.This’shiny’tool allows the user to do this manually.ArgumentsUmatrix Matrix of Umatrix HeightsBestMatches Array with positions of BestMatchesCls Classification of the BestMatchesValueBoolean Matrix that represents the island within the tiled UmatrixReferencesThrun,M.C.,Lerch,F.,Loetsch,J.,Ultsch,A.:Visualization and3D Printing of Multivariate Data of Biomarkers,in Skala,V.(Ed.),International Conference in Central Europe on Computer Graphics,Visualization and Computer Vision,Plzen,2016.Examples##Not run:data("Hepta")e=esomTrain(Hepta$Data,Key=1:nrow(Hepta$Data))Imx=iUmapIsland(e$Umatrix,e$BestMatches)plotMatrix(e$Umatrix,e$BestMatches,Imx=Imx$Imx)##End(Not run)iUstarmatrix iUstarmatrixDescriptionCalculates the Ustarmatrix by combining a Umatrix with a Pmatrix.ArgumentsWeights Weights that were trained by the ESOM algorithmLines Height of the used gridColumns Width of the used gridData Matrix of Data that was used to train the ESOM.One datapoint per rowImx Island mask that will be cut out from displayed UmatrixCls Classification of the BestmatchesToroid Are weights placed on a toroid grid?ValueUstarmatrix matrix with height values of the UstarmatrixReferencesThrun,M.C.,Lerch,F.,Loetsch,J.,Ultsch,A.:Visualization and3D Printing of Multivariate Data of Biomarkers,in Skala,V.(Ed.),International Conference in Central Europe on Computer Graphics,Visualization and Computer Vision,Plzen,2016.plotMatrix plotMatrixDescriptionDraws a plot based of given Umatrix or Pmatrix.ArgumentsMatrix Umatrix or Pmatrix to be plottedBestMatches Positions of BestmMtches to be plotted onto the UmatrixCls Class identifier for the BestMatchesClsColors Vector of colors that will be used to colorize the different classesColorStyle If"Umatrix"the colors of a Umatrix(Blue->Green->Brown->White)will be used;If"Pmatrix"the colors of a Pmatrix(White->Yellow->Red)will beusedToroid Should the Umatrix be drawn4times?BmSize Integer between0.1and5,magnification factor of the drawn BestMatch circles DrawLegend If TRUE,a color legend will be drawn next to the plotFixedRatio If TRUE,the plot will be drawn with afixed ratio of x and y axisCutoutPol Only draws the area within given polygonNrlevels Number of height levels that will be used within the UmatrixTransparentContoursUse half transparent contours.Looks better but is slowImx Mask to cut out an island.Every value should be either1(stays in)or0(gets cut out)Clean If TRUE axis,margins,...surrounding the Umatrix image will be removed RemoveOcean If TRUE,the surrounding blue area around an island will be reduced as much as possible(while still maintaining a rectangular form)TransparentOceanIf TRUE,the surrounding blue area around an island will be transparent Title A title that will be drawn above the plotBestMatchesLabelsVector of strings corresponding to the order of BestMatches which will be drawnon the plot as labelsBestMatchesShapeNumeric value of Shape that will be used.Responds to the usual shapes ofggplotMarkDuplicatedBestMatchesIf TRUE,BestMatches that are shown more than once within an island,will bemarkedYellowCircle If TRUE,a yellow circle is drawn around Bestmatches to distinct them better from backgroundDetailsThe heightScale(nrlevels)is set at the proportion of the1percent quantile against the99percent quantile of the matrix values.ValueA’ggplot’of a MatrixReferencesThrun,M.C.,Lerch,F.,Loetsch,J.,Ultsch,A.:Visualization and3D Printing of Multivariate Data of Biomarkers,in Skala,V.(Ed.),International Conference in Central Europe on Computer Graphics,Visualization and Computer Vision,Plzen,2016.Ultsch,A.:Maps for the visualization of high-dimensional data spaces,Proc.Workshop on Self organizing Maps(WSOM),pp.225-230,Kyushu,Japan,2003.Siemon,H.P.,Ultsch,A.:Kohonen Networks on Transputers:Implementation and Animation,in: Proceedings Intern.Neural Networks,Kluwer Academic Press,Paris,pp.643-646,1990.Examplesdata("Hepta")e=esomTrain(Hepta$Data,Key=1:nrow(Hepta$Data))plotMatrix(e$Umatrix,e$BestMatches)10pmatrixForEsom pmatrixForEsom pmatrixForEsomDescriptionGenerates a Pmatrix based on the weights of an ESOM.ArgumentsData A[n,k]matrix containing the dataWeights Weights stored as a list in a2D matrixLines Number of lines of the SOM that is described by weightsColumns Number of columns of the SOM that is described by weightsRadius The radius for measuring the density within the hyperspherePlotIt If set the Pmatrix will also be plottedToroid Are BestMatches placed on a toroid grid?TRUE by defaultValueUstarMatrixReferencesUltsch,A.:Maps for the visualization of high-dimensional data spaces,Proc.Workshop on Self organizing Maps(WSOM),pp.225-230,Kyushu,Japan,2003.Ultsch,A.,Loetsch,J.:Computed ABC Analysis for Rational Selection of Most Informative Vari-ables in Multivariate Data,PloS one,V ol.10(6),pp.e0129767.doi10.1371/journal.pone.0129767, 2015.Thrun,M.C.,Lerch,F.,Loetsch,J.,Ultsch,A.:Visualization and3D Printing of Multivariate Data of Biomarkers,in Skala,V.(Ed.),International Conference in Central Europe on Computer Graphics,Visualization and Computer Vision,Plzen,2016.Examplesdata("Hepta")e=esomTrain(Hepta$Data,Key=1:nrow(Hepta$Data))Pmatrix=pmatrixForEsom(Hepta$Data,e$Weights,e$Lines,e$Columns,e$Toroid)plotMatrix(Pmatrix,ColorStyle="Pmatrix")showMatrix3D11 showMatrix3D showMatrix3DDescriptionVisualizes the matrix(Umatrix/Pmatrix)in an interactive window in3D.ArgumentsMatrix Matrix to be plottedBestMatches Positions of BestMatches to be plotted onto the matrixCls Class identifier for the BestMatch at the given pointImx a mask(island)that will be used to cut out the UmatrixToroid Should the Matrix be drawn4times(in a toroid view)HeightScale Optional.Scaling Factor for Mountain HeightBmSize Size of drawn BestMatchesRemoveOcean Remove as much area sourrounding an island as possibleColorStyle Either"Umatrix"or"Pmatrix"respectevily for their colorsShowAxis Draw an axis arround the drawn matrixSmoothSlope Try to increase the island size,to get smooth slopes around the islandClsColors Vector of colors that will be used for classesFileName Name for a stlfile to write the Matrix toDetailsThe heightScale is set at the proportion of the1percent quantile against the99percent quantile of the Matrix values.ReferencesThrun,M.C.,Lerch,F.,Loetsch,J.,Ultsch,A.:Visualization and3D Printing of Multivariate Data of Biomarkers,in Skala,V.(Ed.),International Conference in Central Europe on Computer Graphics,Visualization and Computer Vision,Plzen,2016.Examples##Not run:data("Hepta")e=esomTrain(Hepta$Data,Key=1:nrow(Hepta$Data))showMatrix3D(e$Umatrix)##End(Not run)umatrixForEsom umatrixForEsomDescriptionCalculate the Umatrix for given ESOM projectionArgumentsWeights Weights from which the Umatrix will be calculatedLines Number of lines of the SOM that is described by weightsColumns Number of columns of the SOM that is described by weightsToroid Boolean describing if the neural grid should be borderlessValueUmatrixReferencesUltsch,A.and H.P.Siemon,Kohonen’s Self Organizing Feature Maps for Exploratory Data Anal-ysis.1990.Examplesdata("Hepta")e=esomTrain(Hepta$Data,Key=1:nrow(Hepta$Data))umatrix=umatrixForEsom(e$Weights,Lines=e$Lines,Columns=e$Columns,Toroid=e$Toroid)plotMatrix(umatrix,e$BestMatches)ustarmatrixCalc ustarmatrixCalcDescriptionThe UStarMatrix is a combination of the Umatrix(average distance to neighbours)and Pmatrix (density in a point).It can be used to improve the Umatrix,if the dataset contains density based structures.ArgumentsUmatrix A given UmatrixPmatrix A density matrixValueUStarMatrixReferencesUltsch,A.U*C:Self-organized Clustering with Emergent Feature Maps.in Lernen,Wissensent-deckung und Adaptivitaet(LW A).2005.Saarbruecken,Germany.Examplesdata("Hepta")e=esomTrain(Hepta$Data,Key=1:nrow(Hepta$Data))Pmatrix=pmatrixForEsom(Hepta$Data,e$Weights,e$Lines,e$Columns,e$Toroid)Ustarmatrix=ustarmatrixCalc(e$Umatrix,Pmatrix)plotMatrix(Ustarmatrix,e$BestMatches)Index∗ESOMesomTrain,3Umatrix-package,2∗High-Dimensional Data Umatrix-package,2∗High-dimensionalUmatrix-package,2∗HighdimensionalUmatrix-package,2∗P-MatrixpmatrixForEsom,10∗P-matrixpmatrixForEsom,10∗PmatrixpmatrixForEsom,10∗ProjectionUmatrix-package,2∗SOMesomTrain,3Umatrix-package,2∗U*-MatrixustarmatrixCalc,12∗U*-matrixustarmatrixCalc,12∗U*matrixustarmatrixCalc,12∗U-MatrixUmatrix-package,2umatrixForEsom,12∗U-matrixumatrixForEsom,12∗UmatrixUmatrix-package,2umatrixForEsom,12∗Ustar-MatrixustarmatrixCalc,12∗Ustar-matrixustarmatrixCalc,12∗UstarmatrixustarmatrixCalc,12∗VisualizationUmatrix-package,2∗datasets Hepta FCPSHepta,4∗esomesomTrain,3∗self organizing mapesomTrain,3∗somesomTrain,3esomTrain,3Hepta,4iClassification,5iEsomTrain,6iUmapIsland,7iUstarmatrix,7plotMatrix,8pmatrixForEsom,10showMatrix3D,11Umatrix-package,2umatrixForEsom,12ustarmatrixCalc,1214。
机器学习的高维数据分析和可视化
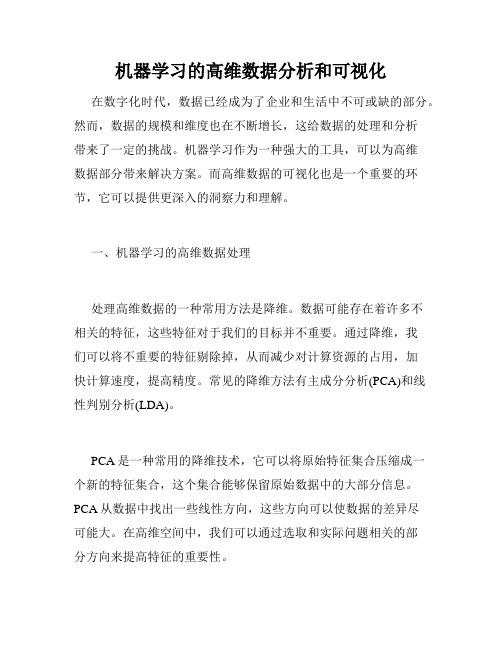
机器学习的高维数据分析和可视化在数字化时代,数据已经成为了企业和生活中不可或缺的部分。
然而,数据的规模和维度也在不断增长,这给数据的处理和分析带来了一定的挑战。
机器学习作为一种强大的工具,可以为高维数据部分带来解决方案。
而高维数据的可视化也是一个重要的环节,它可以提供更深入的洞察力和理解。
一、机器学习的高维数据处理处理高维数据的一种常用方法是降维。
数据可能存在着许多不相关的特征,这些特征对于我们的目标并不重要。
通过降维,我们可以将不重要的特征剔除掉,从而减少对计算资源的占用,加快计算速度,提高精度。
常见的降维方法有主成分分析(PCA)和线性判别分析(LDA)。
PCA是一种常用的降维技术,它可以将原始特征集合压缩成一个新的特征集合,这个集合能够保留原始数据中的大部分信息。
PCA从数据中找出一些线性方向,这些方向可以使数据的差异尽可能大。
在高维空间中,我们可以通过选取和实际问题相关的部分方向来提高特征的重要性。
LDA是另一种常用的降维技术。
它的目标是降低维度并尽量提高类间差异。
LDA通过寻找一组线性方向,使得类内方差最小,类间方差最大。
通过这种方式,LDA可以选择最好的特征来表示数据。
二、机器学习的高维数据可视化化繁为简是数据可视化的一个基本原则。
高维数据在不同维度上的属性分布可能非常复杂,为了更好地理解数据,我们需要将数据可视化为更加简单和易于理解的形式。
一种常见的高维数据可视化技术是热力图。
热力图可以将数据点按照属性值的大小进行颜色编码。
这种方式可以让我们在上百个维度中选择数个属性展示出来,并且能够和其他图表结合起来使用。
用不同的颜色来表示不同的属性值,可以快速地帮助人们理解数据。
另一种高维数据可视化技术是散点图矩阵。
散点图矩阵可以将高维数据转化为二维内部关系。
通过在每个维度上进行散点图分析,我们可以将这些维度中的不同属性直观地展示出来。
如果这些属性关联性非常强,那么就可以展示出属性之间的相关性。
高维数据可视化方法综述

高维数据可视化方法综述高维数据在现代科学和工程领域中得到了广泛应用。
这些数据集往往包含大量的变量,使得对数据的可视化和分析变得非常具有挑战性。
在传统的二维和三维可视化方法难以应对的情况下,高维数据可视化方法应运而生。
本文将综述一些常见的高维数据可视化方法,包括降维方法、聚类方法和可视化技术等,并讨论它们的优缺点以及适用场景。
降维方法旨在将高维数据映射到低维空间,从而方便后续分析和可视化。
其中最常用的方法是主成分分析(PCA)。
PCA通过线性变换将原始数据映射到新的坐标系上,使得映射后的数据在新的坐标系中具有最大的方差。
这样可以大大减少数据的维度,并且保留了大部分的信息量。
然而,PCA只能处理线性相关的数据,对于非线性的数据表现不佳。
为了解决这个问题,许多非线性降维方法被提出,如等距映射(Isomap)和局部线性嵌入(LLE)。
这些方法能够在保持数据的局部结构的同时,将其映射到低维空间中。
尽管这些方法在处理非线性数据时表现较好,但是它们的计算复杂度较高,对大规模数据集的处理存在一定困难。
聚类方法是将高维数据划分为不同的类别或簇。
其中最常用的方法是k-means算法。
k-means算法根据每个数据点和其周围数据点的距离,将数据划分为k个簇。
每个簇的中心点被称为聚类中心,用来表示该簇的特征。
k-means算法简单且易于理解,但在对非球形簇进行划分时表现不佳。
为了解决这个问题,一些改进的聚类方法被提出,如谱聚类和DBSCAN算法。
这些方法通过引入图论和密度的概念,可以更好地处理复杂的数据形状和噪声点。
除了降维和聚类方法,还有一些特定的可视化技术可以用于高维数据的可视化。
其中最常用的方法是平行坐标图。
平行坐标图通过将每个变量绘制在竖直的平行线上,然后将数据点连接起来,形成一条折线。
通过观察折线的形状和趋势,可以快速地了解数据的特征和模式。
然而,当数据的维度较高时,平行坐标图会变得非常密集和复杂,使得数据的分析和理解变得困难。
高维时空房地产数据的可视分析
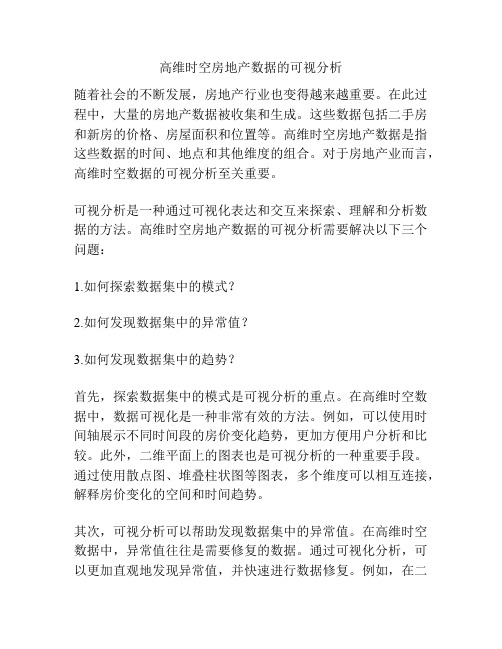
高维时空房地产数据的可视分析随着社会的不断发展,房地产行业也变得越来越重要。
在此过程中,大量的房地产数据被收集和生成。
这些数据包括二手房和新房的价格、房屋面积和位置等。
高维时空房地产数据是指这些数据的时间、地点和其他维度的组合。
对于房地产业而言,高维时空数据的可视分析至关重要。
可视分析是一种通过可视化表达和交互来探索、理解和分析数据的方法。
高维时空房地产数据的可视分析需要解决以下三个问题:1.如何探索数据集中的模式?2.如何发现数据集中的异常值?3.如何发现数据集中的趋势?首先,探索数据集中的模式是可视分析的重点。
在高维时空数据中,数据可视化是一种非常有效的方法。
例如,可以使用时间轴展示不同时间段的房价变化趋势,更加方便用户分析和比较。
此外,二维平面上的图表也是可视分析的一种重要手段。
通过使用散点图、堆叠柱状图等图表,多个维度可以相互连接,解释房价变化的空间和时间趋势。
其次,可视分析可以帮助发现数据集中的异常值。
在高维时空数据中,异常值往往是需要修复的数据。
通过可视化分析,可以更加直观地发现异常值,并快速进行数据修复。
例如,在二手房价格的可视化分析中,通过对价格的分布进行可视化呈现,可以快速发现价格的异常值并进行修复。
最后,可视分析可以帮助发现数据集中的趋势。
在高维时空数据中,趋势是指变量随着时间或空间变化而发生的变化。
通过可视化分析,可以更加清晰地看到趋势的变化。
例如,在房价变动中,通过可视化呈现价格的变化趋势,可以帮助用户更好地预测价格的未来趋势。
在高维时空房地产数据的可视分析中,需要考虑多种方法。
传统的可视化工具如Excel、Tableau和PowerBI等都可以使用,但是在数据量大、维度高的情况下需要考虑更加专业的可视化工具。
例如,Python和R语言是可视分析中非常实用的工具,可以通过matplotlib、seaborn和ggplot等库来创建高质量的图表。
同时,D3.js也是一个非常流行的JavaScript库,提供了大量的可交互和动态效果。
高维数据的聚类分析技术与可视化方法在图像处理中的应用
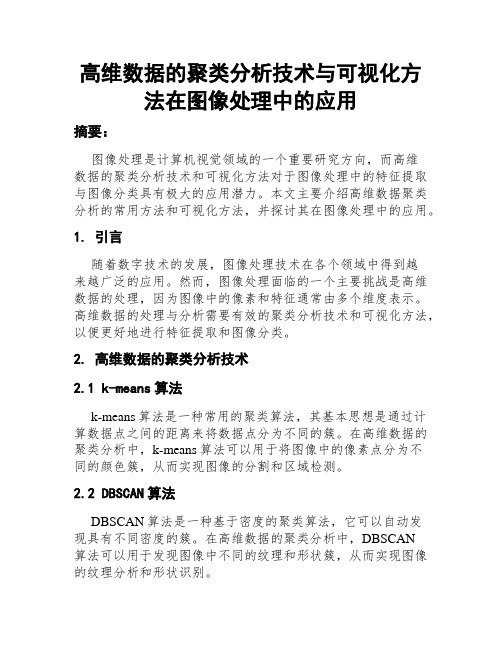
高维数据的聚类分析技术与可视化方法在图像处理中的应用摘要:图像处理是计算机视觉领域的一个重要研究方向,而高维数据的聚类分析技术和可视化方法对于图像处理中的特征提取与图像分类具有极大的应用潜力。
本文主要介绍高维数据聚类分析的常用方法和可视化方法,并探讨其在图像处理中的应用。
1. 引言随着数字技术的发展,图像处理技术在各个领域中得到越来越广泛的应用。
然而,图像处理面临的一个主要挑战是高维数据的处理,因为图像中的像素和特征通常由多个维度表示。
高维数据的处理与分析需要有效的聚类分析技术和可视化方法,以便更好地进行特征提取和图像分类。
2. 高维数据的聚类分析技术2.1 k-means算法k-means算法是一种常用的聚类算法,其基本思想是通过计算数据点之间的距离来将数据点分为不同的簇。
在高维数据的聚类分析中,k-means算法可以用于将图像中的像素点分为不同的颜色簇,从而实现图像的分割和区域检测。
2.2 DBSCAN算法DBSCAN算法是一种基于密度的聚类算法,它可以自动发现具有不同密度的簇。
在高维数据的聚类分析中,DBSCAN算法可以用于发现图像中不同的纹理和形状簇,从而实现图像的纹理分析和形状识别。
2.3 层次聚类算法层次聚类算法是一种基于树状结构的聚类算法,它能够将数据点组织成一棵层次树。
在高维数据的聚类分析中,层次聚类算法可以用于发现图像中不同的物体目标,并对它们进行分类和识别。
3. 高维数据的可视化方法3.1 t-SNE方法t-SNE方法是一种常用的降维和可视化方法,可以将高维数据映射到低维空间中,并保持数据之间的相对距离关系。
在图像处理中,t-SNE方法可以用于将高维图像特征映射到二维平面上,并通过可视化的方式展示不同类别的图像特征。
3.2 PCA方法PCA方法是一种经典的降维和可视化方法,它通过主成分分析将高维数据映射到低维空间中。
在图像处理中,PCA方法可以用于提取图像特征并将其可视化,从而帮助理解和分析图像数据的含义。
高维数据降维分析及可视化方法研究
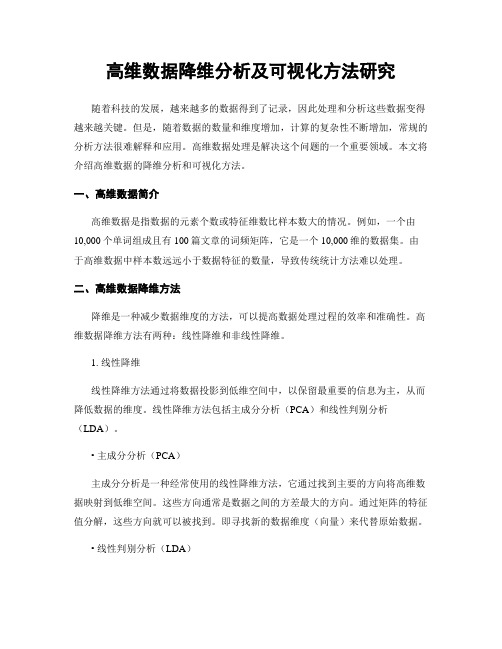
高维数据降维分析及可视化方法研究随着科技的发展,越来越多的数据得到了记录,因此处理和分析这些数据变得越来越关键。
但是,随着数据的数量和维度增加,计算的复杂性不断增加,常规的分析方法很难解释和应用。
高维数据处理是解决这个问题的一个重要领域。
本文将介绍高维数据的降维分析和可视化方法。
一、高维数据简介高维数据是指数据的元素个数或特征维数比样本数大的情况。
例如,一个由10,000个单词组成且有100篇文章的词频矩阵,它是一个10,000维的数据集。
由于高维数据中样本数远远小于数据特征的数量,导致传统统计方法难以处理。
二、高维数据降维方法降维是一种减少数据维度的方法,可以提高数据处理过程的效率和准确性。
高维数据降维方法有两种:线性降维和非线性降维。
1. 线性降维线性降维方法通过将数据投影到低维空间中,以保留最重要的信息为主,从而降低数据的维度。
线性降维方法包括主成分分析(PCA)和线性判别分析(LDA)。
• 主成分分析(PCA)主成分分析是一种经常使用的线性降维方法,它通过找到主要的方向将高维数据映射到低维空间。
这些方向通常是数据之间的方差最大的方向。
通过矩阵的特征值分解,这些方向就可以被找到。
即寻找新的数据维度(向量)来代替原始数据。
• 线性判别分析(LDA)线性判别分析是一种有监督的技术,它试图找到一个投影方式,以便在保留数据最大信息的情况下区分不同类别的数据。
2. 非线性降维非线性降维可以通过将数据投影到一个非线性低维空间中,从而更好地保留数据的结构和特征,尤其是异构数据。
常见的非线性降维方法包括多维缩放(MDS)、非线性主成分分析(NLPCA)和流形学习。
• 多维缩放(MDS)多维缩放主要是在非线性情况下对数据进行降维。
它通过计算与原始数据之间的距离矩阵来表示数据之间的相似度,然后通过优化目标函数将它们投影到低维空间中。
• 非线性主成分分析(NLPCA)非线性主成分分析将主成分分析的思想扩展到非线性情况。
高维数据集聚类结果的可视化分析

第 5期
华丹 阳 : 高维数 据 集聚 类 结果 的可视 化 分析
. 5. 2
括 这 个 非 叶 子 节 点 内 数 据 的 个数 以及 在 每 个 维 度
上 的最 大 值和 最 小 值 。体 部 分 本 身就 是 一 个 子树 ,
缺损 的或者严重 脱离实 际的数据 条 目略 去 , 选取 了其
中的 1 0 0 0 0 0 0 0条数据 条 目输入 系统 , 为了方便操 作 ,
每 1 0个条 目编成 一个组 (u l 。 0 T pe )
而 具体 的数据 值 都 存储 在叶 子节 点 上 。数 据按 照 自
底 向上 的顺 序 , 构建 起 D 树 。 A DA 树 与现 有 的数 据 结 构最 大 的区别 在 它 是一 个 专 门 为聚 类 算法 设 计 的 树形 结 构 , 它 的 每 个 非 在
・
2 6・
黄 山 学 院 学 报
参考文献 :
2 l 年 01
【Ja a a t o n ai s o l t m ninla d n tc l n n Sme.F ud t n fnL j e s a n lr H o l l o ei dt su trs【 ] E io .U A a t cue M .1 dt n S :M G a — l2 0 : a r i c rw Hi,0 6 l
数 , 一 维度 的最 大 值 , 小 值 , , 图 1 每 最 等 如 。
图 1 D A树 的数 据 聚 集 部 分
这 样做 的 目的在 于 ,在进 行 聚 类 分析 运 算 时 ,
我们 能 够 明确 聚 类 分析 的 目标对 象 , 便 聚 类 信 息 方 的读 取与 处理 。
- 1、下载文档前请自行甄别文档内容的完整性,平台不提供额外的编辑、内容补充、找答案等附加服务。
- 2、"仅部分预览"的文档,不可在线预览部分如存在完整性等问题,可反馈申请退款(可完整预览的文档不适用该条件!)。
- 3、如文档侵犯您的权益,请联系客服反馈,我们会尽快为您处理(人工客服工作时间:9:00-18:30)。
2013-浙江大学-数据可视化-暑期研讨会
浙江大学CAD&CG国家重点实验室
Visual Analytics Group
平行坐标
2013-浙江大学-数据可视化-暑期研讨会
浙江大学CAD&CG国家重点实验室
Visual Analytics Group
• 1985年由Inselberg提出,用于研究高维几何 • 坐标轴相互平行(二/三维坐标系中坐标轴相互垂
Chad Jones, et al. An Integrated Exploration Approach to Visualizing Multivariate Particle Data. Computing in Science & Engineering, Volume 10, Number 4, July/August, 2008, pp. 20-29
2013-浙江大学-数据可视化-暑期研讨会
浙江大学CAD&CG国家重点实验室
Visual Analytics Group
Protovis中的效果
2013-浙江大学-数据可视化-暑期研讨会
浙江大学CAD&CG国家重点实验室
Visual Analytics Group
D3.js中的效果
2013-浙江大学-数据可视化-暑期研讨会
2013-浙江大学-数据可视化-暑期研讨会
浙江大学CAD&CG国家重点实验室
Visual Analytics Group
径向轴技术
• 平行坐标的径向排列版本 • 以圆周作为坐标轴,沿圆周绘制线图 • 变种有雷达图,星状图等
2013-浙江大学-数据可视化-暑期研讨会
浙江大学CAD&CG国家重点实验室
Visual Analytics Group
2013-浙江大学-数据可视化-暑期研讨会
浙江大学CAD&CG国家重点实验室
Visual Analytics Group
2013-浙江大学-数据可视化-暑期研讨会
浙江大学CAD&CG国家重点实验室
Visual Analytics Group
Advizor软件中的效果
浙江大学CAD&CG国家重点实验室
Visual Analytics Group
2013-浙江大学-数据可视化-暑期研讨会
浙江大学CAD&CG国家重点实验室
Visual Analytics Group
世界500强企业排名
2013-浙江大学-数据可视化-暑期研讨会
浙江大学CAD&CG国家重点实验室
Visual Analytics Group
Visual Analytics Group
2013-浙江大学-数据可视化-暑期研讨会
浙江大学CAD&CG国家重点实验室
Visual Analytics Group
2013-浙江大学-数据可视化-暑期研讨会
浙江大学CAD&CG国家重点实验室
Visual Analytics Group
基于区域的方法
2013-浙江大学-数据可视化-暑期研讨会
浙江大学CAD&CG国家重点实验室
Visual Analytics Group
2013-浙江大学-数据可视化-暑期研讨会
浙江大学CAD&CG国家重点实验室
Visual Analytics Group
2013-浙江大学-数据可视化-暑期研讨会
浙江大学CAD&CG国家重点实验室
2013-浙江大学-数据可视化-暑期研讨会
浙江大学CAD&CG国家重点实验室
Visual Analytics Group
重排坐标轴
2013-浙江大学-数据可视化-暑期研讨会
浙江大学CAD&CG国家重点实验室
Visual Analytics Group
结合散点图与平行坐标
X Yuan, Guo P, H Xiao et al. Scattering points in parallel coordinates. IEEE Transactions on Visualization and Computer Graphics, 2009, 15(6): 1001-1008.
Visual Analytics Group
2013-浙江大学-数据可视化-暑期研讨会
浙江大学CAD&CG国家重点实验室
Visual Analytics Group
维度相关性
ρ=1 ρ = 0.8
ρ=0
ρ = -0.8 ρ = -1
2013-浙江大学-数据可视化-暑期研讨会
浙江大学CAD&CG国家重点实验室
2013-浙江大学-数据可视化-暑期研讨会
2013-浙江大学-数据可视化-暑期研讨会
浙江大学CAD&CG国家重点实验室
Visual Analytics Group
连续式平行坐标
热力图形式展示主要趋势
J. Heinrich and D. Weiskopf, “Continuous Parallel Coordinates,” IEEE Transactions on Visualization and Computer Graphics, vol. 15, no. 6.
Hale Waihona Puke “选中”操作2013-浙江大学-数据可视化-暑期研讨会
浙江大学CAD&CG国家重点实验室
Visual Analytics Group
“刷取”及“过滤”操作
2013-浙江大学-数据可视化-暑期研讨会
浙江大学CAD&CG国家重点实验室
Visual Analytics Group
半透明平行坐标
展示主要趋势
Visual Analytics Group
1880年的平行坐标作品
2013-浙江大学-数据可视化-暑期研讨会
浙江大学CAD&CG国家重点实验室
Visual Analytics Group
至少使用两个轴
“Hyperdimensional Data Analysis Using Parallel Coordinates”, Wegman, 1990 Based on slide from Munzner
直) • 每个数据点对应一条穿过所有坐标轴的折线
Inselberg A. "The Plane with Parallel Coordinates". Visual Computer, 1 (4): 69–91, 1985.
2013-浙江大学-数据可视化-暑期研讨会
浙江大学CAD&CG国家重点实验室