证据推理理论(Introduction to Evidential Reasoning)
证据理论方法详解分析
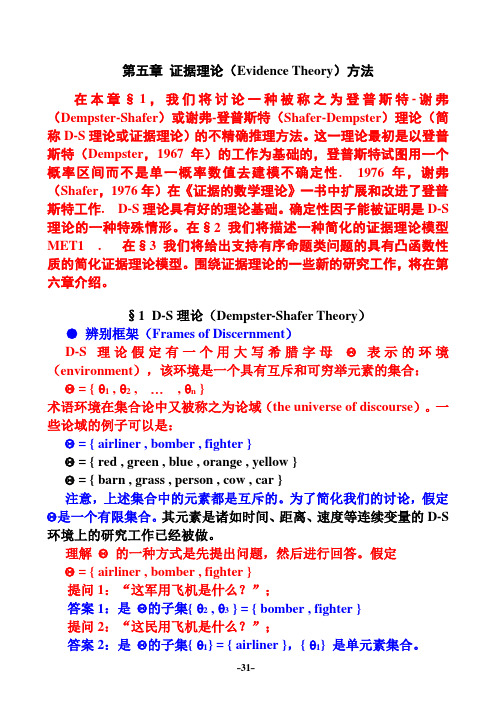
第五章证据理论(Evidence Theory)方法在本章§1,我们将讨论一种被称之为登普斯特-谢弗(Dempster-Shafer)或谢弗-登普斯特(Shafer-Dempster)理论(简称D-S理论或证据理论)的不精确推理方法。
这一理论最初是以登普斯特(Dempster,1967年)的工作为基础的,登普斯特试图用一个概率区间而不是单一概率数值去建模不确定性. 1976年,谢弗(Shafer,1976年)在《证据的数学理论》一书中扩展和改进了登普斯特工作. D-S理论具有好的理论基础。
确定性因子能被证明是D-S 理论的一种特殊情形。
在§2我们将描述一种简化的证据理论模型MET1 . 在§3我们将给出支持有序命题类问题的具有凸函数性质的简化证据理论模型。
围绕证据理论的一些新的研究工作,将在第六章介绍。
§1D-S理论(Dempster-Shafer Theory)●辨别框架(Frames of Discernment)D-S理论假定有一个用大写希腊字母Θ表示的环境(environment),该环境是一个具有互斥和可穷举元素的集合:Θ = { θ1 , θ2 , ⋯, θn }术语环境在集合论中又被称之为论域(the universe of discourse)。
一些论域的例子可以是:Θ = { airliner , bomber , fighter }Θ = { red , green , blue , orange , yellow }Θ = { barn , grass , person , cow , car }注意,上述集合中的元素都是互斥的。
为了简化我们的讨论,假定Θ是一个有限集合。
其元素是诸如时间、距离、速度等连续变量的D-S 环境上的研究工作已经被做。
理解Θ的一种方式是先提出问题,然后进行回答。
假定Θ = { airliner , bomber , fighter }提问1:“这军用飞机是什么?”;答案1:是Θ的子集{ θ2 , θ3 } = { bomber , fighter }提问2:“这民用飞机是什么?”;答案2:是Θ的子集{ θ1} = { airliner },{ θ1} 是单元素集合。
证据理论ppt课件
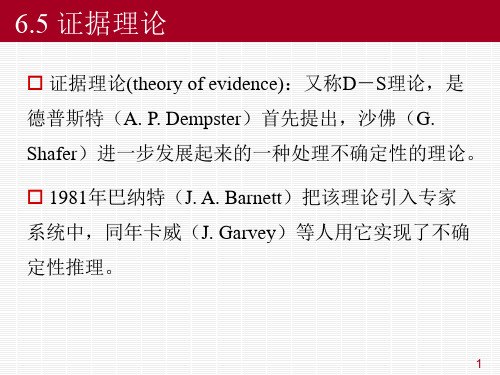
6.5 证据理论
M({黑}) = K M1(x) M2(y)
xy={黑}
=(1/0.61) [M1({黑}) M2({黑}) + M1({黑}) M2({黑,白}) + M1({黑,白}) M2({黑})]
=(1/0.61)[0.30.6+0.30.1+0.20.6=0.54
同理可得 M({白})=0.43, M({黑,白})=0.03 组合后的概率分配函数为: M1({黑},{白},{黑,白}, )=(0.54,0.43,0.03,0)
16
6.5 证据理论
A(0.25,1):由于Bel(A)=0.25,说明对A为真有一定 程度的信任,信任度为0.25;另外,由于Bel(¬A)=1-Pl(A) =1-1=2,说明对¬A不信任,所以A(0.25,0)表示对A为 真有0.25的信任度。
A(0.25 ,0.85 ): Bel(A)=0.25,说明对A为真信 有0.25的信任度;另外,由于Bel(¬A)=1-Pl(A)=1- 0.85 =0.15,说明对A为假有0.15的信任度,所以, A (0.25 ,0.85 )表示对A为真的信任度比对A为假的信任 度稍高一些。
2Ω [0,1] 且 Be(A l)=∑ M(B)
B⊆ A
Bel(A) :对命题A为真的总的信任程度。
Bel :
∀A⊆D
▪ 由信▪ 任设函Ω数={及红概,黄率,分蓝配} 函数的定义推出:
M({红})=0.3, M({黄})=0,M({红,黄})=0.2,
B( e) lM ( )0
B ( 红 , { 黄 e } l M ) ( 红 } { M ( ) 黄 } { M ( ) 红 , { 黄 })
6.5 证据理论
故障选线问题集
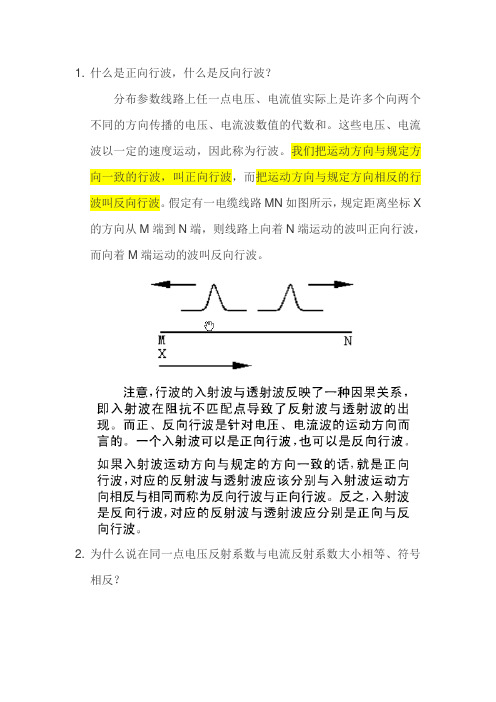
1.什么是正向行波,什么是反向行波?分布参数线路上任一点电压、电流值实际上是许多个向两个不同的方向传播的电压、电流波数值的代数和。
这些电压、电流波以一定的速度运动,因此称为行波。
我们把运动方向与规定方向一致的行波,叫正向行波,而把运动方向与规定方向相反的行波叫反向行波。
假定有一电缆线路MN如图所示,规定距离坐标X 的方向从M端到N端,则线路上向着N端运动的波叫正向行波,而向着M端运动的波叫反向行波。
2.为什么说在同一点电压反射系数与电流反射系数大小相等、符号相反?3.行波中的零模分量和线模分量是什么?有什么区别?4.小波变换?特别是二进制小波变换?5.何为对端母线?对端负载?6.暂态电流形成的反向行波浪涌与其对应的正向行波浪涌的极性?7.利用直流高压或脉冲高压信号的作用把电缆故障点击穿?把故障点击穿是什么意思?8.冲闪测试是什么?以“冲闪法”为原理的电缆故障测试仪,实际应当叫“高压电缆的高阻故障测试仪”,它对高压电缆的高阻闪络及高阻泄露故障解决起来非常适合,而且目前也只有此种方式可以解决,这是因为此类故障一般在电缆的外皮看不出有什么痕迹,只有用“冲闪法”迫使故障点放电,才能测出故障点距离,击穿电缆外皮使故障点表露出来,才能确定故障点的准确位置。
9.线性电流耦合器?10.高速采集技术?11.高阻故障是什么?12.什么是奇异信号?在信号系统分析中,经常要遇到函数本身有不连续点或其导数与积分有不连续点的情况,这类函数称为奇异函数或奇异信号。
奇异性实际上是衡量信号在各尺度上某一点或某一区间在各个尺度的衰减程度。
可以用如下公式求解:abs(wf(u,s))<=A*s*exp (a+1/2)来求解。
u是时移,s是尺度,u在区间[a,b]上,公式中的a就是lipschitz指数,这是区间上的一致性,当然还有点态lipschtiz 指数,仅供参考。
奇异信号可分为斜变信号、阶跃信号(最重要)、冲激信号(最重要)、冲激偶信号。
一种新的证据距离表示方法
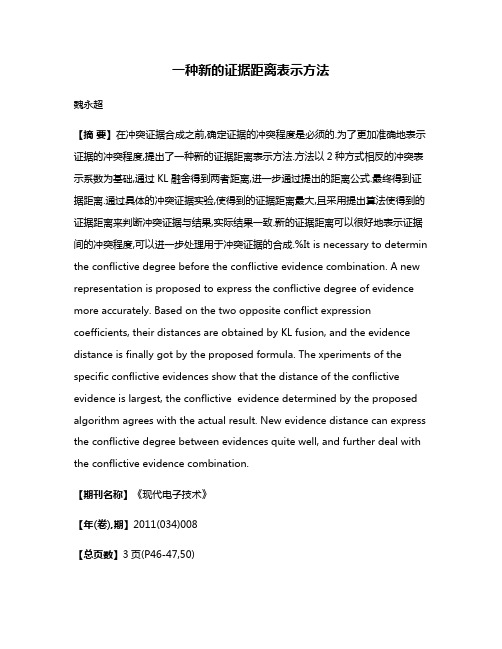
一种新的证据距离表示方法魏永超【摘要】在冲突证据合成之前,确定证据的冲突程度是必须的.为了更加准确地表示证据的冲突程度,提出了一种新的证据距离表示方法.方法以2种方式相反的冲突表示系数为基础,通过KL融舍得到两者距离,进一步通过提出的距离公式.最终得到证据距离.通过具体的冲突证据实验,使得到的证据距离最大,且采用提出算法使得到的证据距离来判断冲突证据与结果,实际结果一致.新的证据距离可以很好地表示证据间的冲突程度,可以进一步处理用于冲突证据的合成.%It is necessary to determin the conflictive degree before the conflictive evidence combination. A new representation is proposed to express the conflictive degree of evidence more accurately. Based on the two opposite conflict expression coefficients, their distances are obtained by KL fusion, and the evidence distance is finally got by the proposed formula. The xperiments of the specific conflictive evidences show that the distance of the conflictive evidence is largest, the conflictive evidence determined by the proposed algorithm agrees with the actual result. New evidence distance can express the conflictive degree between evidences quite well, and further deal with the conflictive evidence combination.【期刊名称】《现代电子技术》【年(卷),期】2011(034)008【总页数】3页(P46-47,50)【关键词】信息融合;证据合成;冲突;证据距离【作者】魏永超【作者单位】中国民用航空飞行学院,飞行技术与飞行安全科研基地,四川,广汉,618307【正文语种】中文【中图分类】TN91-340 引言D-S理论是Dempster和Shafer共同创立的一种不确定性推理理论,由于它对不确定信息的表达和合成提供了自然而强有力的方法,因此在信息融合中获得了广泛的应用[1-3]。
证据理论方法详解
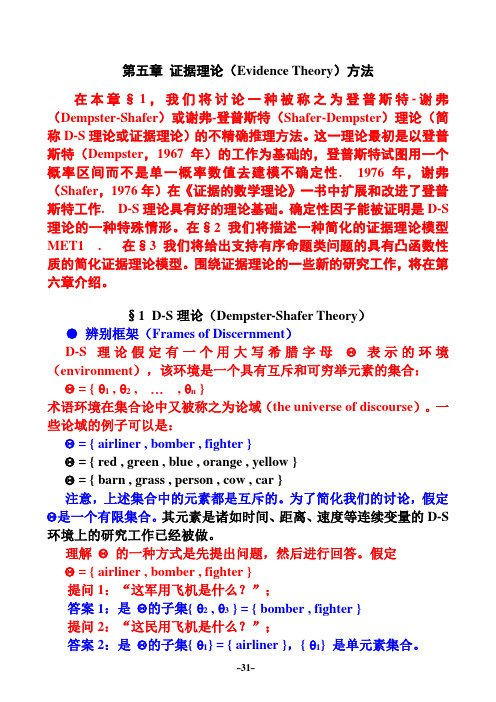
第五章证据理论(Evidence Theory)方法在本章§1,我们将讨论一种被称之为登普斯特-谢弗(Dempster-Shafer)或谢弗-登普斯特(Shafer-Dempster)理论(简称D-S理论或证据理论)的不精确推理方法。
这一理论最初是以登普斯特(Dempster,1967年)的工作为基础的,登普斯特试图用一个概率区间而不是单一概率数值去建模不确定性. 1976年,谢弗(Shafer,1976年)在《证据的数学理论》一书中扩展和改进了登普斯特工作. D-S理论具有好的理论基础。
确定性因子能被证明是D-S 理论的一种特殊情形。
在§2我们将描述一种简化的证据理论模型MET1 . 在§3我们将给出支持有序命题类问题的具有凸函数性质的简化证据理论模型。
围绕证据理论的一些新的研究工作,将在第六章介绍。
§1D-S理论(Dempster-Shafer Theory)●辨别框架(Frames of Discernment)D-S理论假定有一个用大写希腊字母Θ表示的环境(environment),该环境是一个具有互斥和可穷举元素的集合:Θ = { θ1 , θ2 , ⋯, θn }术语环境在集合论中又被称之为论域(the universe of discourse)。
一些论域的例子可以是:Θ = { airliner , bomber , fighter }Θ = { red , green , blue , orange , yellow }Θ = { barn , grass , person , cow , car }注意,上述集合中的元素都是互斥的。
为了简化我们的讨论,假定Θ是一个有限集合。
其元素是诸如时间、距离、速度等连续变量的D-S 环境上的研究工作已经被做。
理解Θ的一种方式是先提出问题,然后进行回答。
假定Θ = { airliner , bomber , fighter }提问1:“这军用飞机是什么?”;答案1:是Θ的子集{ θ2 , θ3 } = { bomber , fighter }提问2:“这民用飞机是什么?”;答案2:是Θ的子集{ θ1} = { airliner },{ θ1} 是单元素集合。
证据英文_精品文档
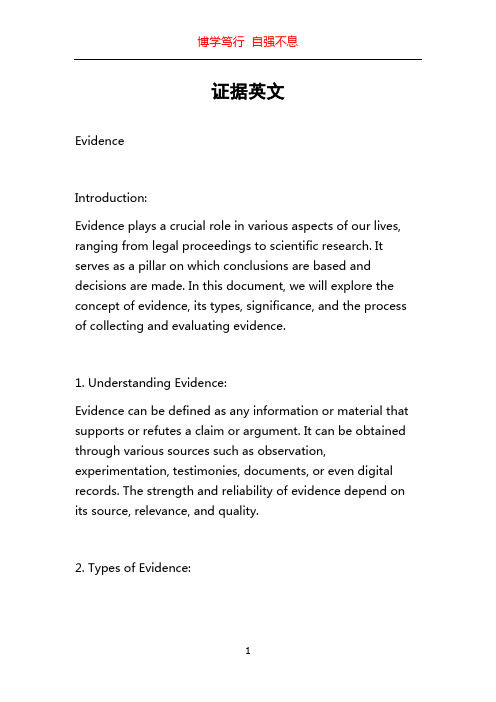
证据英文EvidenceIntroduction:Evidence plays a crucial role in various aspects of our lives, ranging from legal proceedings to scientific research. It serves as a pillar on which conclusions are based and decisions are made. In this document, we will explore the concept of evidence, its types, significance, and the process of collecting and evaluating evidence.1. Understanding Evidence:Evidence can be defined as any information or material that supports or refutes a claim or argument. It can be obtained through various sources such as observation, experimentation, testimonies, documents, or even digital records. The strength and reliability of evidence depend on its source, relevance, and quality.2. Types of Evidence:a) Direct Evidence: This type of evidence directly proves or disproves a fact or claim. For example, eyewitness testimonies, surveillance footage, or DNA analysis.b) Circumstantial Evidence: Unlike direct evidence, circumstantial evidence does not directly prove a claim but can be inferred to support it. It relies on the logical connection between the evidence and the conclusion. For instance, finding a suspect's fingerprints at a crime scene.c) Documentary Evidence: This category includes written or recorded evidence, such as contracts, emails, diaries, photographs, or official documents. It serves as a primary source of evidence in legal cases or historical research.d) Digital Evidence: With technology becoming increasingly prevalent, digital evidence has gained importance. It includes digital records, computer files, social media posts, or electronic communications.3. The Significance of Evidence:a) Establishing Truth: Evidence is crucial for establishing the truth or accuracy of a claim. It provides the necessary support to validate or reject a hypothesis or statement.b) Influencing Decision-Making: Evidence plays a vital role in influencing decisions made by individuals, organizations, or even governing bodies. It helps in determining guilt or innocence in legal cases or forming policies based on scientific research.c) Ensuring Fairness: Evidence ensures fairness in legal proceedings by providing an objective basis for judgments. It helps in avoiding bias or subjective decision-making.4. Collecting and Evaluating Evidence:a) Identification: The first step in the process is identifying relevant evidence. This involves determining which type of evidence is necessary and where it can be found.b) Collection: Once identified, evidence needs to be collected using appropriate techniques. This may involve maintaining a proper chain of custody, ensuring the integrity and admissibility of the evidence.c) Preservation: Proper preservation of evidence is crucial to avoid tampering or contamination. This includes storingevidence in controlled environments and adopting best practices to prevent degradation.d) Evaluation: After collecting the evidence, it needs to be carefully evaluated for its relevance, reliability, and credibility. Various methods such as expert analysis or scientific testing can be employed to assess the evidentiary value.e) Presentation: Finally, evidence is presented in a coherent and organized manner to effectively communicate its significance. In legal proceedings, this may include witness testimonies, exhibits, or expert reports.Conclusion:In conclusion, evidence plays a vital role in various aspects of our lives, be it law, science, or everyday decision-making. Understanding the types, significance, and the process of collecting and evaluating evidence is essential for making informed judgments and reaching accurate conclusions. It serves as a foundation for truth-seeking, fairness, and the pursuit of justice in society.。
DS证据理论课件
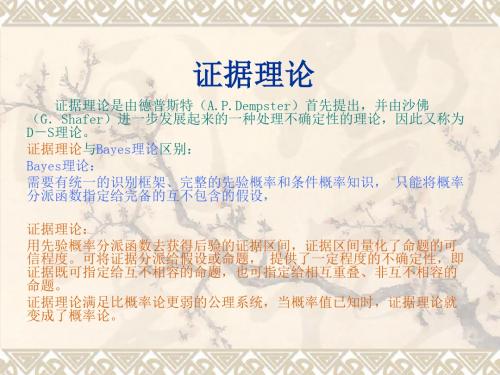
3. 似然函数
定义3: 似然函数Pl:2D→[0,1],且 Pl(A)=1一Bel(¬A) 其中A⊆D 似然函数的含义:由于Bel(A)表示对A为
真的信任程度,所以Bel(¬A)就表示对非A为 真,即A为假的信任程度,由此可推出Pl(A) 表示对A为非假的信任程度。 似然函数又称为不可驳斥函数或上限函数。
0≤CER(E)≤1
4. 组合证据不确定性的算法
当组合证据是多个证据的合取时,即
E=E1 AND E2 AND … AND En
则E的确定性CER(E)为:
CER(E)=min{CER(El),CER(E2), …,CER(En)}
当组合证据是多个证据的析取时,即
E=El OR E2 OR … OR En
则E的确定性CER(E)为
CER(E)=max{CER(El),CER(E2), …,CER(En)}
5. 不确定性的传递算法
对于知识: IF E THEN H={h1,h2,…,hn} CF={c1,c2,…,cn} 结论H的确定性可通过下述步骤求出: (1)求出H的概率分配函数。对上述知识,H的概
定义:若A⊆D则M(A)≠0,称A为M的一个焦元。
3. 概率分配函数不是概率。
2. 信任函数
定义2 :命题的信任函数Bel:2D→[0,1],且 Bel(A)=ΣM(B)对所有的A⊆D
B⊆A
其中2D表示D的所有子集。 Bel函数又称为下限函数,Bel(A)表示对命题A
为真的信任程度。 由信任函数及概率分配函数的定义推出: Bel(Φ)=M(Φ)=0 Bel(D)=ΣM(B)=1
率分配函数为: M({hl},{h2},…,{hn})={CER(E)×c1,
证据理论概述

证据理论概述一、理论的提出1967年,Dempster提出上概率和下概率。
1976年,Shafer进一步完善,建立了命题和集合之间的一一对应关系,把命题的不确定问题转化为集合的不确定问题,满足比概率论弱的情况,形成了一套关于证据推理的数学理论。
证据理论是一种不确定的推理方法,与之类似的还有云模型、模糊理论、Bayes方法。
证据理论可以看作是根据证据做出决策的理论。
一个证据会在对应问题的决策解集合(决策框架)上产生一个基本信任分配(信任函数),该信任分配就是要决策的结果。
多个证据产生多个基本信任分配,再求出多个信任分配的正交和,即证据合成,最终得到一个决策结果。
该决策结果综合了多个专家的经验和知识。
然而,证据理论中,要求参与合成的证据相互独立,这在实际应用时很难做到。
一旦证据发生冲突,往往会得出与事实相悖的结果。
所以,证据理论中,冲突证据的合成仍是一个亟待解决的问题。
二、基本理论证据理论中,决策框架、信任函数、证据合成是关键。
决策框架Θ,是指命题的所有可能的答案组成的完备集合,集合中的所有元素都是两两互斥的,任一时刻,答案只能取集合中的某一元素。
基本信任分配函数m,是一个从Θ的幂集2Θ到[0,1]的映射,其中Θ的任一子集A满足m(Ф)=0, Σm(A)=1,表示证据对A的信任程度。
A=θi,m(A)表示对相应命题A的精确信任度;A=Θ,m(A)是对Θ的各个子集进行信任分配后剩下的部分,表示不知道该如何对它进行分配。
A≠Θ,A≠θi,m(A)是对相应命题A的精确信任度,但却不知道这部分信任具体该分给A中的哪些元素。
注:A∈B,m(A)与m(B)没有任何关系。
信任函数Bel,是一个从Θ的幂集2Θ到[0,1]的映射,其中Θ的任一子集A满足Bel(A)= Σm(B) (B ∈A),Bel(A)称为A的信任函数,表示证据对A为真的信任程度。
信任函数Bel满足1()ABel AA=Θ⎧=⎨≠Θ⎩(空信任函数),才能用D-S证据合成规则。
- 1、下载文档前请自行甄别文档内容的完整性,平台不提供额外的编辑、内容补充、找答案等附加服务。
- 2、"仅部分预览"的文档,不可在线预览部分如存在完整性等问题,可反馈申请退款(可完整预览的文档不适用该条件!)。
- 3、如文档侵犯您的权益,请联系客服反馈,我们会尽快为您处理(人工客服工作时间:9:00-18:30)。
2. plausibility function pl : 2 → [0, 1] is defined as: Shafer : bel is ‘normalized’ => closed world assumption=> bel(Ω)=1, pl(Ω)=1,m(∅)
Observations in belief functions
Belief functions
Ω the frame of discernment(elements of the set Ω are called ‘worlds’) One “actual world” ω0. But which? An agent can only express the strength of his/her opinion (called belief) degree of belief that the actual world belongs to this or that subset of Ω. [0, Shafer belief function bel : 2 → [0 1] bel(A) denotes the strength of Agent’s belief that ω0∈A. bel satisfies the following inequalities:
Not supporting any strictly more specific propositions A basic belief mass given to a set A supports also that the actual world is in every subsets that contains A. The degree of belief bel(A) for A quantifies the total amount of justified specific support given to A. We say justified because we include in bel(A) only the basic belief masses given to subsets of A. m({x,y}) given to {x,y} could support x if further information indicates this.However given the available information the basic belief mass can only be given to {x,y}. We say specific because the basic belief mass m(Ø) is not included in bel(A) as it is given to the subset Ø.
Dempster–Shafer approach
Answering the question ‘‘what is the belief in A, as expressed by the probability that the proposition A is provable given the evidence?’’ An alternative to traditional probabilistic theory for the mathematical representation of uncertainty. Whereas a Bayesian approach assesses probabilities directly for the answer, the Dempster–Shafer approach assesses evidence for related questions. -combination of evidence obtained from multiple sources and the modeling of conflict between them. -Allocation of a probability mass to sets or intervals Pros -Ability to model various types of partial ignorance, limited or conflicting evidence -more flexible model than Bayes’ theorem. -computationally simpler than Bayes’ theorem. -No assumption regarding the probability of the individual constituents of the set or interval. -evaluation of risk and reliability in engineering applications when it is not possible to obtain a precise measurement from experiments, or when knowledge is obtained from expert elicitation. Cons -Can produce conclusions that are counter-intuitive. Dempster–Shafer is most suited to situations where beliefs are numerically expressed and where there is some degree of ignorance, i.e. there is an incomplete model.
Entertained beliefs and beliefs in a decision context
Uncertainty induces beliefs=“graded dispositions that guide our behavior” ‘rational’agent behavior described within decision contexts “It has been argued that decisions are ‘rational’ only if we use a probability
Introduction to Evidential Reasoning
Belief Functions
Ignorance Types
Incompleteness: Combinatory Combinatory Imprecision: Combinatory Combinatory Interval theory Fuzzy sets Possibility Theory (physical form) Uncertainty: Probability Theory Upper-Lower Probabilities the chance of it being "heads" when tossing a coin. John's wife is Jill or Joan. Jill is not John's wife. Paul's height is between 170 and 180. Paul is tall. the possibility for Paul's height to be about 175 cm. John is married, but his wife's name is not given All computer scientists like pizza, but their names are not available.
Other useful functions (‘1-1’ with bel) 1.basic belief assignment (bba) m : 2 → [0, 1] defined as:
m(A) for A ⊆ Ω is called the basic belief mass (bbm) given to A. It may happen that m(∅) > 0. The relation from m to bel is given by:
Answering ‘‘what is the belief in A? as expressed by the unconditional probability that A is true given evidence, e ?’’ Assumption: precise probabilities can be assessed for all events. Too rare… Why believe one hypothesis other than that provided by the evidence?? The evidence have to be re-organised so that probabilities sum to unity. Pros -rules of probability calculus : uncontroversial, constant conclusions with the probability assessments. -Bayesian theory is easy to understand. Cons It is least suited to problems where there is -partial or complete ignorance -limited or conflicting information due to assumptions made(e.g. equi-probability) Cannot deal with imprecise, qualitative or natural language judgements such as ‘‘if A then probably B’’.