支付宝:2016 年中国人支付账单 移动端支付占71%
初一到初三全英语单词表[1]
![初一到初三全英语单词表[1]](https://img.taocdn.com/s3/m/b412393f26fff705cd170a1b.png)
初一到初三全英语单词表注:n 名词v 动词adj形容词adv 副词prep介词conj连词phr.短语num数词第一册1-—--8331 what pron 什么2 is v 是3 what's what is的缩写形式4 your pron 你的,你们的5 name n 名字6 my pron 我的7 I pron 我8 am v 是9 I'm I am的缩写形式10 in prep 在.。
.里(内,上)11 row n (一)排,(一)行12 one num 一13 number n 数字,号码14 two num 二15 too adv 也16 three num 三17 are v 是18 you pron 你,你们19 yes adv 是20 four num 四21 five num 五22 no adv &adj 不,不是23 not adv 不24 hi interj 喂(问候或唤起注意)25 class n (学校里的)班级,年级26 grade n 年级27 six num 六28 seven num 七29 eight num 八30 nine num 九31 ten num 十32 zero num &n 零33 plus prep 加,加上34 it pron 它35 it's it is的缩写形式36 how adv (指程度)多少,怎样37 old adj 。
.岁的,老的38 eleven num 十一39 twelve num 十二40 minus prep 减,减去41 thirteen num 十三42 fourteen num 十四43 fifteen num 十五44 hello interj 喂(问候或唤起注意)45 please interj 请46 can v。
aux 能,可以,会47 spell v 拼写48 that pron 那,那个49 secret n 秘密50 this pron 这,这个51 in prep 用...(表达)52 English n & adj 英语,英国人英国的,英国人的53 in English phr. 用英语(表达)54 a art 一(个,件。
office2016vlookup函数的使用方法
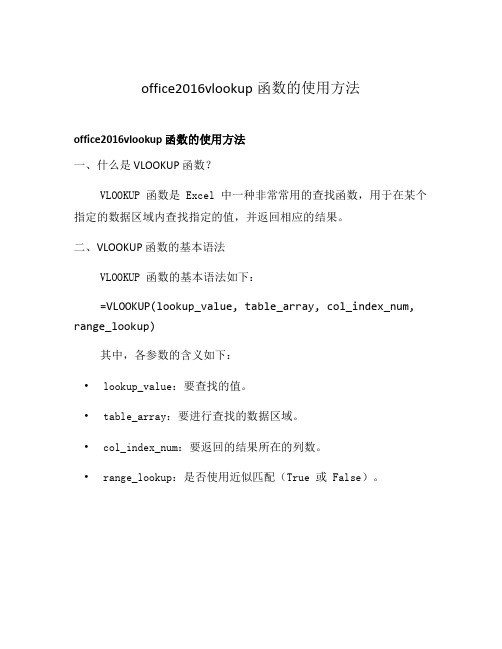
office2016vlookup函数的使用方法office2016vlookup 函数的使用方法一、什么是 VLOOKUP 函数?VLOOKUP 函数是 Excel 中一种非常常用的查找函数,用于在某个指定的数据区域内查找指定的值,并返回相应的结果。
二、VLOOKUP 函数的基本语法VLOOKUP 函数的基本语法如下:=VLOOKUP(lookup_value, table_array, col_index_num, range_lookup)其中,各参数的含义如下:•lookup_value:要查找的值。
•table_array:要进行查找的数据区域。
•col_index_num:要返回的结果所在的列数。
•range_lookup:是否使用近似匹配(True 或 False)。
三、使用示例1. 基本的查找操作假设我们有一个名为成绩表的数据区域,包含学生姓名和对应的成绩。
我们要查找某个学生的成绩。
通过下面的公式即可实现:=VLOOKUP("小明", 成绩表, 2, False)其中,“小明”是要查找的学生姓名,成绩表是数据区域的范围,2是指返回的结果在数据表中的第二列。
2. 使用近似匹配在一些情况下,我们可能希望用近似匹配的方式进行查找。
=VLOOKUP(80, 成绩表, 2, True)以上公式中,80 是要查找的分数,成绩表是数据区域的范围,2 是指返回的结果在数据表中的第二列。
3. 使用变量进行查找除了直接指定值进行查找外,我们还可以使用单元格中的值进行查找。
=VLOOKUP(A1, 成绩表, 2, False)以上公式中,A1 是包含要查找的值的单元格,成绩表是数据区域的范围,2 是指返回的结果在数据表中的第二列。
四、总结通过本文的介绍,我们已经了解了 VLOOKUP 函数的基本用法和常见示例。
通过灵活运用 VLOOKUP 函数,我们可以方便地进行数据查找和计算,提高工作效率。
USB Type-C 规范1.2(中文版)
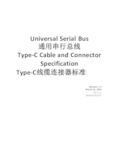
知识产权声明
THIS SPECIFICATION IS PROVIDED TO YOU “AS IS” WITH NO WARRANTIES WHATSOEVER, INCLUDING ANY WARRANTY OF MERCHANTABILITY, NON-INFRINGEMENT, OR FITNESS FOR ANY PARTICULAR PURPOSE. THE AUTHORS OF THIS SPECIFICATION DISCLAIM ALL LIABILITY, INCLUDING LIABILITY FOR INFRINGEMENT OF ANY PROPRIETARY RIGHTS, RELATING TO USE OR IMPLEMENTATION OF INFORMATION IN THIS SPECIFICATION. THE PROVISION OF THIS SPECIFICATION TO YOU DOES NOT PROVIDE YOU WITH ANY LICENSE, EXPRESS OR IMPLIED, BY ESTOPPEL OR OTHERWISE, TO ANY INTELLECTUAL PROPERTY RIGHTS.
预发行行业审查公司提供反馈
Revision History.......................................................................................................................14
LIMITED COPYRIGHT LICENSE: The USB 3.0 Promoters grant a conditional copyright license under the copyrights embodied in the USB Type-C Cable and Connector Specification to use and reproduce the Specification for the sole purpose of, and solely to the extent necessary for, evaluating whether to implement the Specification in products that would comply with the specification.
sqlserver cve-2004-2761

SQL Server是微软公司的一款关系数据库管理系统,广泛用于企业级应用程序和全球信息站开发中。
2004年,SQL Server出现了一个安全漏洞,CVE编号为CVE-2004-2761。
该漏洞允许攻击者通过精心构造的SQL查询来执行未经授权的数据库操作,可能导致数据泄露、数据库瘫痪,甚至服务器被入侵。
以下是对该漏洞的详细解读:一、漏洞描述CVE-2004-2761是SQL Server的一个认证绕过漏洞。
在受影响的SQL Server版本中,当用户尝试使用FTP或HTTP上传文件时,攻击者可以通过夹带特定的SQL查询,绕过认证机制实现未经授权的数据库操作。
这种漏洞的存在可能会严重影响服务器的安全性和稳定性。
二、受影响的版本CVE-2004-2761影响的SQL Server版本包括但不限于:- Microsoft SQL Server 7.0- Microsoft SQL Server 2000- Microsoft SQL Server 2005- Microsoft SQL Server 2008三、攻击方式攻击者可以利用CVE-2004-2761漏洞,构造恶意的SQL查询,并将其夹带在FTP或HTTP上传的文件中。
当服务器接收到这些文件并解析时,恶意的SQL查询将被执行,从而导致数据库操作的未经授权执行。
四、潜在威胁CVE-2004-2761漏洞的潜在威胁包括但不限于:- 数据泄露:攻击者可以利用漏洞来获取数据库中的敏感信息,如用户账户、密码等。
- 数据库瘫痪:攻击者可能执行恶意操作导致数据库瘫痪,影响正常的业务操作。
- 服务器入侵:利用漏洞进行未经授权的数据库操作,最终可能导致服务器被入侵控制。
五、解决方案针对CVE-2004-2761漏洞,建议采取以下解决方案:1. 及时安装补丁:微软公司已发布针对该漏洞的安全补丁,建议及时对受影响的SQL Server版本进行升级和更新补丁。
2. 进行安全配置:对SQL Server进行安全配置,限制用户权限,避免恶意SQL查询的执行。
编译原理期末试题8套含答案大题集

《编译原理》期末试题(一)一、是非题(请在括号内,正确的划√,错误的划×)(每个2分,共20分)1.编译程序是对高级语言程序的解释执行。
(× )2.一个有限状态自动机中,有且仅有一个唯一的终态。
(×)3.一个算符优先文法可能不存在算符优先函数与之对应。
(√ )4.语法分析时必须先消除文法中的左递归。
(×)5.LR分析法在自左至右扫描输入串时就能发现错误,但不能准确地指出出错地点。
(√)6.逆波兰表示法表示表达式时无须使用括号。
(√ )7.静态数组的存储空间可以在编译时确定。
(×)8.进行代码优化时应着重考虑循环的代码优化,这对提高目标代码的效率将起更大作用。
(×) 9.两个正规集相等的必要条件是他们对应的正规式等价。
(× )10.一个语义子程序描述了一个文法所对应的翻译工作。
(×)二、选择题(请在前括号内选择最确切的一项作为答案划一个勾,多划按错论)(每个4分,共40分) 1.词法分析器的输出结果是_____。
A.( ) 单词的种别编码B.( ) 单词在符号表中的位置C.( ) 单词的种别编码和自身值D.( ) 单词自身值2.正规式M 1 和M 2 等价是指_____。
A.( ) M1和M2的状态数相等B.( ) M1和M2的有向边条数相等C.( ) M1和M2所识别的语言集相等D.( ) M1和M2状态数和有向边条数相等3.文法G:S→xSx|y所识别的语言是_____。
A.( ) xyx B.( ) (xyx)* C.( ) xnyxn(n≥0) D.( ) x*yx*4.如果文法G是无二义的,则它的任何句子α_____。
A.( )最左推导和最右推导对应的语法树必定相同B.( ) 最左推导和最右推导对应的语法树可能不同C.( ) 最左推导和最右推导必定相同D.( )可能存在两个不同的最左推导,但它们对应的语法树相同5.构造编译程序应掌握______。
Techniques and Tools for Analyzing Intrusion Alerts
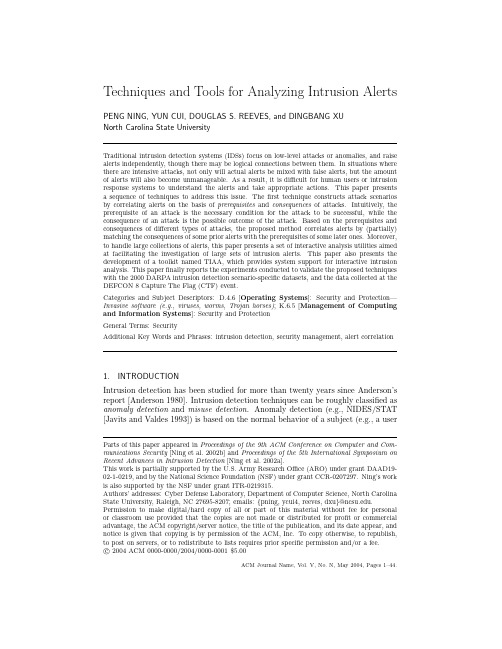
Techniques and Tools for Analyzing Intrusion AlertsPENG NING,YUN CUI,DOUGLAS S.REEVES,and DINGBANG XUNorth Carolina State University1.INTRODUCTIONIntrusion detection has been studied for more than twenty years since Anderson’s report[Anderson1980].Intrusion detection techniques can be roughly classified as anomaly detection and misuse detection.Anomaly detection(e.g.,NIDES/STAT [Javits and Valdes1993])is based on the normal behavior of a subject(e.g.,a user2·Ning,Cui,Reeves,and Xuor a system);any action that significantly deviates from the normal behavior is con-sidered intrusive.Misuse detection(e.g.,NetSTAT[Vigna and Kemmerer1999]) detects attacks based on the characteristics of known attacks or system vulnerabil-ities;any action that conforms to the pattern of a known attack or vulnerability is considered intrusive.Traditional intrusion detection systems(IDSs)focus on low-level attacks or anoma-lies,and raise alerts independently,though there may be logical connections be-tween them.In situations where there are intensive attacks,not only will actual alerts be mixed with false alerts,but the amount of alerts will also become unman-ageable.As a result,it is difficult for human users or intrusion response systems to understand the alerts and take appropriate actions.Therefore,it is necessary to develop techniques to construct attack scenarios(i.e.,steps that attackers use in their attacks)from alerts to facilitate intrusion analysis.In this paper,we develop a sequence of techniques to address the aforemen-tioned problem.Our techniques start with a practical alert correlation method for constructing attack scenarios from IDS alerts.This technique can be explained through the following observation:most attacks are not isolated,but related as dif-ferent stages of attack sequences,with the early stages preparing for the later ones. For example,in Distributed Denial of Service(DDoS)attacks,the attacker has to install the DDoS daemon programs in vulnerable hosts before he can instruct the daemons to launch an attack.In other words,an attacker has to(or usually does) reach a certain state before he can carry out certain attacks,and usually reaches the state by launching some other attacks.Based on this observation,we correlate alerts using prerequisites and consequences of attacks.Intuitively,the prerequisite of an attack is the necessary condition for the attack to be successful,while the consequence of an attack is the possible outcome of the attack.For example,the existence of a vulnerable service is the prerequisite of a remote buffer overflow attack against the service,and as the consequence of the attack,the attacker may gain access to the host.Accordingly,we correlate the alerts together when the attackers launch some early attacks to prepare for the prerequisites of some later ones.For example,if they use a UDP port scan to discover the vulnerable services,followed by an attack against one of the services, we can correlate the corresponding alerts together.It is well-known that current IDSs often miss unknown attacks,or variations of known attacks.To tolerate missing detections,our method allows partial satisfaction of prerequisites of an attack.In addition,our method allowsflexible alert aggregation,and provides intuitive representations of correlated alerts.We observe that it is quite challenging to analyze intrusion alerts even with the help of alert correlation,particularly due to the large amount of alerts produced by IDSs.As indicated in[Manganaris et al.2000],“encountering10–20,000alarms per sensor per day is common.”In this paper,we also present six utilities(called aggregation/disaggregation,focused analysis,clustering analysis,frequency analy-sis,link analysis,and association analysis)to facilitate the analysis of large sets of correlated alerts.The proposed alert correlation method and these utilities are then integrated into one system,providing human users a platform to analyze and understand the correlated alerts as well as the strategies behind them.ACM Journal Name,Vol.V,No.N,May2004.Techniques and Tools for Analyzing Intrusion Alerts·3 The contribution of this paper is as follows.First,we develop a formal framework for alert correlation.Our method can deal with attack attempts and correlate alerts as long as there are signs of connections between them,even if some related attacks fail or bypass the IDSs.In addition,our method provides an intuitive mechanism (called hyper-alert correlation graph)to represent the attack scenarios constructed through alert correlation.Second,we develop a set of interactive utilities to fa-cilitate the analysis of very large attack scenarios.These utilities either reduce the size of large attack scenarios(while keeping the structure of the attacks),or extract selected features from large attack scenarios.Third,we develop a toolkit named TIAA(A Toolkit for Intrusion Alert Analysis),which integrates the alert correlation method and the analysis utilities into one system to provide support for interactive intrusion analysis.Finally,we perform a series of experiments to validate the alert correlation method and the analysis utilities using2000DARPA intrusion detection scenario specific datasets[MIT Lincoln Lab2000]and the net-work traffic captured at the DEFCON8Capture the Flag(CTF)event[DEFCON 2000].Our results show that our correlation method not only correlates related alerts and uncovers the attack strategies,but also provides a way to differentiate between alerts,and that the interactive analysis utilities can effectively simplify the analysis of large amounts of alerts.The remainder of this paper is organized as follows.The next section discusses related work.Section3presents our formal framework for correlating alerts using prerequisites and consequences of attacks.Section4describes the analysis utilities for analyzing attack scenarios constructed from large collections of alerts.Section 5presents the development of the toolkit named TIAA for intrusion alert analysis. Section6reports our experiments with the2000DARPA intrusion detection sce-nario specific datasets and the DEFCON8CTF dataset.Section7discusses the advantages and limitations of our approach,and Section8concludes this paper and points out some future research directions.2.RELATED WORKIntrusion detection has been studied for more than twenty years since Anderson’s report[Anderson1980].A survey of the early work on intrusion detection is given in[Mukherjee et al.1994],and an excellent overview of current intrusion detection techniques and related issues can be found in a recent book[Bace2000]. Research on intrusion alert correlation has been rather active recently.Thefirst class of approaches(e.g.,Spice[Staniford et al.2002],probabilistic alert correlation [Valdes and Skinner2001],and the alert clustering methods in[Cuppens2001]and [Julisch2001])correlates alerts based on the similarities between alert attributes. Though they are effective for clustering similar alerts(e.g.,alerts with the same source and destination IP addresses),they cannot fully discover the causal relation-ships between related alerts.Another class of methods(e.g.,correlation based on STATL[Eckmann et al.2002] or LAMBDA[Cuppens and Ortalo2000],and the data mining approach[Dain and Cunningham2001])performs alert correlation based on attack scenarios specified by human users,or learned from training datasets.A limitation of these methods is that they are restricted to known attack scenarios,or those that can be generalizedACM Journal Name,Vol.V,No.N,May2004.4·Ning,Cui,Reeves,and Xufrom known scenarios.A variation in this class uses a consequence mechanism to specify what types of attacks may follow a given attack,partially addressing this problem[Debar and Wespi2001].A third class of methods,including JIGSAW[Templeton and Levitt2000],the MIRADOR correlation method[Cuppens and Miege2002],and our approach,tar-gets recognition of multi-stage attacks;it correlates alerts if the prerequisites of some later alerts are satisfied by the consequences of some earlier alerts.Such methods can potentially uncover the causal relationship between alerts,and are not restricted to known attack scenarios.Our method can be considered as a variation of JIGSAW[Templeton and Levitt 2000].Both methods try to uncover attack scenarios based on specifications of indi-vidual attacks.However,our method also differs from JIGSAW.First,our method allows partial satisfaction of prerequisites(i.e.,required capabilities in JIGSAW [Templeton and Levitt2000]),recognizing the possibility of undetected attacks and that of attackers gaining information through non-intrusive ways(e.g.,talking to a friend working in the victim organization),while JIGSAW requires all required capabilities be satisfied.Second,our method allows aggregation of alerts,and thus can reduce the complexity involved in alert analysis,while JIGSAW currently does not have any similar mechanisms.Third,we develop a set of utilities for alert correlation and interactive analysis of correlated alerts,which is not provided by JIGSAW.The work closest to ours is the MIRADOR correlation method proposed in[Cup-pens and Miege2002],which was developed independently and in parallel to ours. These two methods share substantial similarity.The MIRADOR approach also correlates alerts using partial match of prerequisites(pre-conditions)and conse-quences(post-conditions)of attacks.However,the MIRADOR approach uses a different formalism than ours.In particular,the MIRADOR approach treats alert aggregation as an individual stage before alert correlation,while our method allows alert aggregation during and after correlation.As we will see in Section4,our treatment of alert aggregation leads to the utilities for interactive alert analysis.A formal model named M2D2was proposed in[Morin et al.2002]to correlate alerts by using multiple information sources,including the characteristics of the monitored systems,the vulnerability information,the information about the mon-itoring tools,and information of the observed events.Due to the multiple infor-mation sources used in alert correlation,this method can potentially lead to better results than those simply looking at intrusion alerts.A mission-impact-based ap-proach was proposed in[Porras et al.2002]to correlate alerts raised by INFOSEC devices such as IDSs andfirewalls.A distinguishing feature of this approach is that it correlates the alerts with the importance of system assets so that attention can be focused on critical resources.These methods are complementary to ours. Several techniques have used the prerequisites and consequences of attacks for vulnerability analysis purposes.In[Ritchey and Ammann2000],a model checking technique was applied to analyze network vulnerabilities on the basis of prerequisites and results(i.e.,consequences)of exploits(i.e.,attacks),along with hosts and network connectivity information.In[Sheyner et al.2002]and[Jha et al.2002], the technique in[Ritchey and Ammann2000]was further extended to generate ACM Journal Name,Vol.V,No.N,May2004.Techniques and Tools for Analyzing Intrusion Alerts·5 and analyze all possible attacks against a vulnerable networked system.These techniques are focused on analyzing what attacks may happen to violate a given security property.In contrast,our purpose is to reconstruct what has happened according to the alerts reported by IDSs,and our technique has to deal with the inaccuracy of IDSs(i.e.,false alerts and undetected attacks).We consider our method as complementary to these vulnerability analysis techniques.Several languages have been proposed to represent attacks,including STAT[Il-gun et al.1995;Vigna and Kemmerer1999;Eckmann et al.2002],Colored-Petri Automata(CPA)[Kumar and Spafford1994;Kumar1995],LAMBDA[Cuppens and Ortalo2000],and MuSig[Lin et al.1998]and its successor[Ning et al.2001]. In particular,LAMBDA uses a logic-based method to specify the pre-condition and post-condition of attacks,which is similar to our method.However,all these languages specify entire attack scenarios,which are limited to known scenarios.In contrast,our method(as well as JIGSAW and the MIRADOR correlation method) describes prerequisites and consequences of individual attacks,and correlates de-tected attacks(i.e.,alerts)based on the relationship between these prerequisites and consequences.Thus,our method can potentially correlate alerts in unknown attack scenarios.GrIDS uses activity graphs to represent the causal structure of network activi-ties and detect propagation of large-scale attacks[Staniford-Chen et al.1996].Our method also uses graphs to represent correlated alerts.However,unlike GrIDS,in which nodes represent hosts or departments and edges represent network traffic be-tween them,our method uses nodes to represent alerts,and edges the relationships between the alerts.Alert correlation has been studied in the context of network management(e.g., [Gruschke1998],[Ricciulli and Shacham1997],and[Gardner and Harle1998]).In theory,alert correlation methods for network management are applicable to intru-sion alert correlation.However,intrusion alert correlation faces more challenges than its counter part in network management:While alert correlation for network management deals with alerts about natural faults,which usually exhibits regular patterns,intrusion alert correlation has to cope with less predictable,malicious intruders.3.A FRAMEWORK FOR ALERT CORRELATIONAs discussed in the introduction,our method is based on the observation that in a series of attacks,the attacks are usually not isolated,but related as different stages of the attack sequence,with the early ones preparing for the later ones.To take advantage of this observation,we propose to correlate the alerts generated by IDSs using prerequisites and consequences of the corresponding attacks.Intu-itively,the prerequisite of an attack is the necessary condition for the attack to be successful.For example,the existence of a vulnerable service is a prerequisite for a remote buffer overflow attack against the service.Moreover,the attacker may make progress in gaining access to the victim system(e.g.,discover the vulnerable services,install a Trojan horse program)as a result of an rmally,we call the possible outcome of an attack the(possible)consequence of the attack.In a series of attacks where the attackers launch earlier attacks to prepare for later ones,ACM Journal Name,Vol.V,No.N,May2004.6·Ning,Cui,Reeves,and Xuthere are usually strong connections between the consequences of the earlier attacks and the prerequisites of the later ones.Indeed,if an earlier attack is to prepare for a later attack,the consequence of the earlier attack should at least partly satisfy the prerequisite of the later attack.Accordingly,we propose to identify the prerequisites(e.g.,existence of vulnerable services)and the consequences(e.g.,discovery of vulnerable services)of each type of attack.These are then used to correlate alerts,which are attacks detected by IDSs,by matching the consequences of(the attacks corresponding to)some previous alerts and the prerequisites of(the attacks corresponding to)some later ones.For example,if wefind a Sadmind Ping followed by a buffer overflow attack against the corresponding Sadmind service,we can correlate them to be parts of the same series of attacks.In other words,we model the knowledge(or state)of attackers in terms of individual attacks,and correlate alerts if they indicate the progress of attacks.Note that an attacker does not have to perform early attacks to prepare for a later attack,even though the later attack has certain prerequisites.For example,an attacker may launch an individual buffer overflow attack against a service blindly, without knowing if the service exists.In other words,the prerequisite of an attack should not be mistaken for the necessary existence of an earlier attack.However, if the attacker does launch attacks with earlier ones preparing for later ones,our method can correlate them,provided that the attacks are detected by IDSs.In the following subsections,we adopt a formal approach to develop our alert correlation method.3.1Prerequisite and Consequence of AttacksWe propose to use predicates as basic constructs to represent the prerequisites and (possible)consequences of attacks.For example,a scanning attack may discover UDP services vulnerable to a certain buffer overflow attack.We can use the pred-icate UDPVulnerableToBOF(VictimIP,VictimPort)to represent the attacker’s discovery(i.e.,the consequence of the attack)that the host having the IP address VictimIP runs a service(e.g.,sadmind)at UDP port VictimPort and that the ser-vice is vulnerable to the buffer overflow attack.Similarly,if an attack requires a UDP service vulnerable to the buffer overflow attack,we can use the same predicate to represent the prerequisite.Some attacks may require several conditions be satisfied at the same time in order to be successful.To represent such complex conditions,we use a logical combination of predicates to describe the prerequisite of an attack.For example, a certain network launched buffer overflow attack may require that the target host have a vulnerable UDP service accessible to the attacker through thefirewall.This prerequisite can be represented by UDPVulnerableToBOF(VictimIP,VictimPort)∧UDPAccessibleViaFirewall(VictimIP,VictimPort).To simplify the following discussion,we restrict the logical operators in predicates to∧(conjunction)and∨(disjunction).We also use a set of predicates to represent the(possible)consequence of an attack.For example,an attack may result in compromise of the root privilege as well as modification of the.rhostfile.Thus,we may use the following to repre-sent the corresponding consequence:{GainRootAccess(VictimIP),rhostModified ACM Journal Name,Vol.V,No.N,May2004.Techniques and Tools for Analyzing Intrusion Alerts·7 (VictimIP)}.Note that the set of predicates used to represent the consequence is essentially the logical combination of these predicates and can be represented by a single logical formula.However,representing the consequence as a set of predicates rather than a long formula is more convenient and will be used here.The consequence of an attack is indeed the possible result of the attack.In other words,the attack may or may not generate the stated consequence.For example, after a buffer overflow attack against a service,an attacker may or may not gain the root access,depending on if the service is vulnerable to the attack.We use possible consequences instead of actual consequences due to the following two reasons.First,an IDS may not have enough information to decide if an attack is effective or not.For example,a network based IDS can detect certain buffer overflow attacks by matching the patterns of the attacks;however,it cannot decide whether the attempts succeed or not without more information from the related hosts.Thus,it may not be practical to correlate alerts using the actual consequences of attacks.In contrast,the possible consequence of a type of attack can be analyzed and made available for the IDS.Second,even if an attack fails to prepare for the follow-up attacks,the follow-up attacks may still occur simply because,for example, the attacker wants to test the success of the previous attack,or the attacker uses a script to launch a series of ing possible consequences of attacks will lead to better opportunity to correlate such attacks.For the sake of brevity,we refer to a possible consequence simply as a consequence throughout this paper.3.2Hyper-alert Type and Hyper-alertUsing predicates as the basic construct,we introduce the notion of a hyper-alert type to represent the prerequisite and the consequence of each type of alert.Definition3.1.A hyper-alert type T is a triple(fact,prerequisite,consequence), where(1)fact is a set of attribute names,each with an associated domain of values, (2)prerequisite is a logical combination of predicates whose free variables are all in fact,and(3)consequence is a set of predicates such that all the free variables in consequence are in fact.Each hyper-alert type encodes the knowledge about a type of attack.The compo-nent fact of a hyper-alert type tells what kind of information is reported along with the alert(i.e.,detected attack),prerequisite specifies what must be true in order for the attack to be successful,and consequence describes what could be true if the attack indeed succeeds.For the sake of brevity,we omit the domains associated with the attribute names when they are clear from the context.Example3.2.Consider the buffer overflow attack against the sadmind remote administration tool.We may have a hyper-alert type SadmindBufferOverflow= ({VictimIP,VictimPort},ExistHost(VictimIP)∧VulnerableSadmind(VictimIP), {GainRootAccess(VictimIP)})for such attacks.Intuitively,this hyper-alert type says that such an attack is against the host at IP address VictimIP.(We expect the actual values of VictimIP are reported by an IDS.)For the attack to be successful, there must exist a host at IP address VictimIP,and the corresponding sadmind service must be vulnerable to buffer overflow attacks.The attacker may gain root privilege as a result of the attack.ACM Journal Name,Vol.V,No.N,May2004.8·Ning,Cui,Reeves,and XuGiven a hyper-alert type,a hyper-alert instance can be generated if the corre-sponding attack is detected and reported by an IDS.For example,we can generate a hyper-alert instance of type SadmindBufferOverflow from a corresponding alert. The notion of hyper-alert instance is formally defined as follows:Definition3.3.Given a hyper-alert type T=(fact,prerequisite,consequence), a hyper-alert(instance)h of type T is afinite set of tuples on fact,where each tuple is associated with an interval-based timestamp[begin time].The hyper-alert h implies that prerequisite must evaluate to True and all the predicates in consequence might evaluate to True for each of the tuples.(Notation-wise,for each tuple t in h,we use t.begin time to refer to the timestamp associated with t.)The fact component of a hyper-alert type is essentially a relation schema(as in relational databases),and a hyper-alert is a relation instance of this schema.One may point out that an alternative way is to represent a hyper-alert as a record, which is equivalent to a single tuple on fact.However,such an alternative cannot accommodate certain alerts possibly reported by an IDS.For example,an IDS may report an IPSweep attack along with multiple swept IP addresses,which cannot be represented as a single record.In addition,our current formalism allows aggregation of alerts of the same type,and isflexible in reasoning about alerts.Therefore,we believe the current notion of a hyper-alert is an appropriate choice.A hyper-alert instantiates its prerequisite and consequence by replacing the free variables in prerequisite and consequence with its specific values.Since all free vari-ables in prerequisite and consequence must appear in fact in a hyper-alert type,the instantiated prerequisite and consequence will have no free variables.Note that prerequisite and consequence can be instantiated multiple times if fact consists of multiple tuples.For example,if an IPSweep attack involves several IP addresses, the prerequisite and consequence of the corresponding hyper-alert type will be in-stantiated for each of these addresses.In the following,we treat timestamps implicitly and omit them if they are not necessary for our discussion.Example3.4.Consider the hyper-alert type SadmindBufferOverflow in example 3.2.There may be a hyper-alert h SadmindBOF as follows:{(VictimIP=152.1.19.5, VictimPort=1235),(VictimIP=152.1.19.7,VictimPort=1235)}.This implies that if the attack is successful,the following two logical formulas must be True as the prerequisites of the attack:ExistHost(152.1.19.5)∧VulnerableSadmind (152.1.19.5),ExistHost(152.1.19.7)∧VulnerableSadmind(152.1.19.7).Moreover, as possible consequences of the attack,the following might be True:GainRootAccess (152.1.19.5),GainRootAccess(152.1.19.7).This hyper-alert says that there are buffer overflow attacks against sadmind at IP addresses152.1.19.5and152.1.19.7, and the attacker may gain root access as a result of the attacks.A hyper-alert may correspond to one or several related alerts.If an IDS reports one alert for a certain attack and the alert has all the information needed to instan-tiate a hyper-alert,a hyper-alert can be generated from the alert.However,some IDSs may report a series of alerts for a single attack.For example,EMERALD may report several alerts(within the same thread)related to an attack that spreads over ACM Journal Name,Vol.V,No.N,May2004.Techniques and Tools for Analyzing Intrusion Alerts·9 a period of time.In this case,a hyper-alert may correspond to the aggregation of all the related alerts.Moreover,several alerts may be reported for the same type of attack in a short period of time.Our definition of hyper-alert allows them to be treated as one hyper-alert,and thus providesflexibility in the reasoning about alerts.Certain constraints are necessary to make sure the hyper-alerts are reason-able.However,since our hyper-alert correlation method does not depend on them directly,we will discuss them after introducing our method.Ideally,we may correlate a set of hyper-alerts with a later hyper-alert if the consequences of the former ones imply the prerequisite of the latter one.However, such an approach may not work in reality due to several reasons.First,the attacker may not always prepare for certain attacks by launching some other attacks.For example,the attacker may learn a vulnerable sadmind service by talking to people who work in the organization where the system is running.Second,the current IDSs may miss some attacks,and thus affect the alert correlation if the above approach is used.Third,due to the combinatorial nature of the aforementioned approach, it is computationally expensive to examine sets of alerts tofind out whether their consequences imply the prerequisite of an alert.Having considered these issues,we adopt an alternative approach.Instead of examining if several hyper-alerts imply the prerequisite of a later one,we check if an earlier hyper-alert contributes to the prerequisite of a later one.Specifically, we decompose the prerequisite of a hyper-alert into individual predicates and test whether the consequence of an earlier hyper-alert makes some of the prerequisites True(i.e.,make the prerequisite easier to satisfy).If the result is yes,then we correlate the hyper-alerts together.This idea is specified formally through the following Definitions.Definition3.5.Consider a hyper-alert type T=(fact,prerequisite,consequence). The prerequisite set(or consequence set,resp.)of T,denoted P(T)(or C(T),resp.), is the set of all predicates that appear in prerequisite(or consequence,resp.).Given a hyper-alert instance h of type T,the prerequisite set(or consequence set,resp.) of h,denoted P(h)(or C(h),resp.),is the set of predicates in P(T)(or C(T),resp.) whose arguments are replaced with the corresponding attribute values of each tuple in h.Each element in P(h)(or C(h),resp.)is associated with the timestamp of the corresponding tuple in h.(Notation-wise,for each p∈P(h)(or C(h),resp.), we use p.begin time to refer to the timestamp associated with p.) Example3.6.Consider the Sadmind Ping attack through which an attacker dis-covers possibly vulnerable sadmind services.The corresponding alerts can be repre-sented by a hyper-alert type SadmindPing=({VictimIP,VictimPort},{ExistHost (VictimIP)},{VulnerableSadmind(VictimIP)}).Suppose a hyper-alert instance h SadmindP ing of type SadmindPing has the follow-ing tuples:{(VictimIP=152.1.19.5,VictimPort=1235),(VictimIP=152.1.19.7, VictimPort=1235),(VictimIP=152.1.19.9,VictimPort=1235)}.Then we have the prerequisite set P(h SadmindP ing)={ExistHost(152.1.19.5),ExistHost (152.1.19.7),ExistHost(152.1.19.9)},and the consequence set C(h SadmindP ing)= {VulnerableSadmind(152.1.19.5),VulnerableSadmind(152.1.19.7),VulnerableSad-mind(152.1.19.9)}.ACM Journal Name,Vol.V,No.N,May2004.。
2-3-习题(含解答)

2-3 习题(含解答)目录第1章编译原理概述 (1)第2章PL/O编译程序的实现 (4)第3章文法和语言 (4)第4章词法分析 (13)第5章自顶向下语法分析方法 (28)第6章自底向上优先分析 (39)第7章LR分析 (42)第8章语法制导翻译和中间代码生成 (60)第9章符号表 (67)第10章目标程序运行时的存储组织 (70)第11章代码优化 (73)第12章代码生成 (76)综合练习一 (79)综合练习二 (84)综合练习三 (90)综合练习四 (95)综合练习五 (101)综合练习六 (107)第1章编译原理概述一、选择题1.一个编译程序中,不仅包含词法分析,语法分析,中间代码生成,代码优化,目标代码生成等五个部分,还应包括 (1) 。
其中, (2) 和代码优化部分不是每个编译程序都必需的。
词法分析器用于识别 (3) ,语法分析器则可以发现源程序中的 (4) 。
(1) A.模拟执行器 B.解释器 C.表格处理和出错处理 D.符号执行器(2) A.语法分析 B.中间代码生成 C.词法分析 D.目标代码生成(3) A.字符串 B.语句 C.单词 D.标识符(4) A.语义错误 B.语法和语义错误 C.错误并校正 D.语法错误2.程序语言的语言处理程序是一种 (1) 。
(2) 是两类程序语言处理程序,他们的主要区别在于 (3) 。
(1) A.系统软件 B.应用软件 C.实时系统 D.分布式系统(2) A.高级语言程序和低级语言程序 B.解释程序和编译程序C.编译程序和操作系统D.系统程序和应用程序(3) A.单用户与多用户的差别 B.对用户程序的查错能力C.机器执行效率D.是否生成目标代码3.汇编程序是将翻译成,编译程序是将翻译成。
A.汇编语言程序B.机器语言程序C.高级语言程序D. A 或者BE. A 或者CF. B或者C4.下面关于解释程序的描述正确的是。
(1) 解释程序的特点是处理程序时不产生目标代码(2) 解释程序适用于COBOL 和 FORTRAN 语言(3) 解释程序是为打开编译程序技术的僵局而开发的A. (1)(2)B. (1)C. (1)(2)(3)D.(2)(3)5.高级语言的语言处理程序分为解释程序和编译程序两种。
信捷伺服手册

b
目录
目录
前言 ......................................................................................................................................................... I 本手册的内容构成........................................................................................................................... I 本手册的适用读者........................................................................................................................... I 手册的获取途径............................................................................................................................... I
2.请勿直接触摸产品的导电部位。 有可能引起误动作、故障。
z 产品的接线
危险
1.在对驱动器进行接线操作前,请务必断开所有外部电源。 有触电的危险。
2.请将 AC 电源正确连接到驱动器的专用电源端子上。 接错电源,可能引起火灾。
注意
1.请勿将驱动器的输出端子 U、V、W 与三相电源连接。 a
- 1、下载文档前请自行甄别文档内容的完整性,平台不提供额外的编辑、内容补充、找答案等附加服务。
- 2、"仅部分预览"的文档,不可在线预览部分如存在完整性等问题,可反馈申请退款(可完整预览的文档不适用该条件!)。
- 3、如文档侵犯您的权益,请联系客服反馈,我们会尽快为您处理(人工客服工作时间:9:00-18:30)。
支付宝:2016 年中国人支付账单移动端支付占71%
1 月 4 日,蚂蚁金服旗下的支付宝发布了 2016 年的中国人全民账单。
这份从多方面诠释国民经济数据的账单,展示了过去的一年里中国消费全面升级、消费主力年龄下降、消费方式多样化等变化趋势。
账单显示,在刚刚过去的 2016 年,4.5 亿实名用户使用了支付宝,71% 的支付笔数发生在移动端,超 10 亿人次使用线上公共服务;中国“千禧一代”80后、90后已经成为中国经济的主流力量,并将影响未来十年的经济格局,比如 80 后人均支付金额已超过 12 万元,90 后使用移动支付更是高达 91%;同时,4.5 亿中国消费者开始呈现出与十年前完全不一样的生活形态。
今年 G20 期间,中国的移动支付普及程度成为了到访中国的各国惊叹的事情。
国际市场研究机构凯度 TNS 日前发布研报称,中国内地、中国香港和韩国已经成为当前全球移动支付市场的三甲。
支付宝 2016 全民账单显示,目前移动支付笔数占整体比例高达 71%,成为生活中像“水电煤”一样的基础设施。
去年这一数据是 65%,今年移动支付渗透率的攀升很大程度上是因为线下手机支付的习惯养成。
从省级地区的全年支付总金额来看,沿海的广东排名第一,占到全国的 16%,随后是浙江、江苏。
从人均支付金额来看,位列第一的上海人均支付金额达到14.8 万元,是去年的近 1.5 倍。
随后是浙江、北京、福建、江苏,这五个沿海省份和直辖市,人均支付金额均超过 10 万元,而 2015 年达到该水平的只有上海市。
(图自:Telegraph)
从移动支付渗透率来看,内陆的西藏以 90% 的移动支付占比排名第一,随后是青海、甘肃,远远超过沿海省份。
从 2012 年开始,西藏移动支付比例蝉联冠军。
目前西藏所有行政村实现移动通讯信号覆盖,因此偏僻村落、广袤牧区的农牧民更依赖移动支付来进行生活必需品的消费。
支付宝年度账单数据显示,2016 年西藏人均手机充值 16.7 次,远高于其他地区。
如果说 2016 年前是移动支付的普及期,那么 2016 年可以称作是这钟支付方式全面深入中国人民日常生活的一年。
随着社会总财富增加,衡量社会价值的维度更多元,同时也体现在人的诉求也不只是单一的,相应的诉求让支付宝也不再只是一个资金账户,更加是信用账户,同时也正逐渐成为碳账户、爱账户。
首先是伴随着行为数据的多元,越来越多的用户开始在网络上积累起信用,并且移动互联网+消费信贷的出现,让用户切身感受到信用的价值。
2016 年,使用花呗支付的笔数超过 32 亿笔,比上一年增长了 344%。
目前,借呗累计服务用户超过 1200 万人,累计放款超过 3000 亿元。
(图自:蚂蚁花呗)
“敢花未来钱”的新生代中国人进入全面的消费升级期,2016 年这个趋势不只是局限于发达地区,比如去年西藏地区使用花呗的人数增长率达到 220%,使用花呗金额增长率达 380%。
“敢花未来钱”伴随的,也是全民对社会信用体系的重视。
在支付宝公布的2016 年借呗“有借有还排行榜”上,最准时还钱的第一名是河南省,随后是安徽省、江苏省。
关注世界的同时,中国人也越来越开始走向世界。
2016 年,中国人出境游同比增长了 160%。
越来越多人带着家人一起旅行,在 350 万通过 airbnb 订房的中国游客中,58% 的家庭游会选择整套公寓,24% 的家庭游会选择别墅,只有1% 的家庭游会选择合住。
在支付宝统计的跨境消费数据中,中国人最爱的前十大扫货地分别是:韩国、香港、泰国、澳门、台湾、日本、澳大利亚、新加坡、新西兰、德国。
2016年,支付宝跨境扫码付单笔最高消费 268645 元,用户来自北京市。
(图自:Pixabay)
消费升级也越来越多中国人对财富管理更加积极,大量消费者感受到自己理财的必要性。
以蚂蚁金服旗下的蚂蚁聚宝为例,截止 2016 年底,三四五线城市及农村的理财用户占 6 成以上,支付宝上购买互联网保险服务的用户在 2016 年超过 3.3 亿。
在追求更好生活品质的同时,中国人也越来越追求效率。
支付宝全民账单显示, 2016 年有超过 10 亿人次使用线上公共服务,比去年增长 218%。
这些服务来自全国 357 个城市,占中国城市总数的 54%,服务内容包括缴纳水电燃气费、缴纳交通违法罚款、地铁票公交票手机购买、在线办理港澳通行证续签、预约挂号、登记结婚、离婚、登记器官捐献、在线预约无人机喷洒农药等。
支付宝账单显示,2016 年中国主力消费者已经完全转移到 80 后、90 后年龄层。
与他们的父辈相比,80 后、90 后这一代人的受教育水平更高,更不容易定居,更崇尚自由、平等,不受拘束,对速度有高要求,他们喜欢消费甚至超前消费,喜欢体验更好的生活享受,是视觉动物、网络原住民、也更重视健康和环保。
2016 年支付宝全民账单显示,2016 年,全国的 80 后人均网上支付金额超过 12 万元,而 90 后移动支付占比近 92 %。
旅行方式上,这个群体更偏爱自由行,本次账单中 2016年境外随身 wifi 租赁增长了 171%,自驾租车增长了 110% 似乎印证了这一点。
而在饮食方式上,到店吃饭、线上叫外卖已经深入人心:90 后用户到店吃饭人数占比高达 46%,外卖更是高达 61%。
加上 80 后之后,比例高达 85%。
年轻人对身体的管理和生活品质的追求也在升级。
2016 年,80 后用户买保健品的消费量下降了 13%,但户外运动则增长了 9%,美妆笔数增长 16%。
而 90 后则更加明显:户外运动增幅 34%,美妆类则高达 63%;在话剧、演出、电影方面的票务支出占全国这类票务购买总量的 84%;
在消费升级潮下,中国 80 后、90 后也是“敢花未来钱”理念的忠实信徒。
目前 1 亿花呗用户中,中国 80 后、90 后占了 86%,也就是中国每 4 个 90 后,就有 1 个使用花呗。
在这份 2016 年“剁手账单”中,一个高度依赖移动支付、消费欲望强劲、愿意接受新奇消费方式的中国消费市场被刻画了出来。
自爱范儿。