新浪微博用户等级体系分析
新浪微博用户及其微博特征分析-微博用户分析

新浪微博用户及其微博特征分析作者:周世妍来源:《新媒体研究》2017年第21期摘要新浪微博作为国内最大、最有价值的微博平台,其用户特征及需求的分析对其他网络社交平台乃至政府部门决策都有着一定的借鉴意义。
文章从传播学角度对新浪微博用户的构成、特征以及使用需求进行了全面的分析,从中获取所需要的数据信息,以期解密新浪微博发展迅猛的原因,并为进一步促进新浪微博的良性发展提供启示和帮助。
关键词新浪微博;用户特征;网络平台;发展对策中图分类号 G2 文献标识码 A 文章编号 2096-0360(2017)21-0008-022009年8月新浪微博推出后,用户数量呈“爆炸式”增长,短短3年时间就突破了5亿。
直至现在,新浪微博一直在各大在线社交网站中处于“领先者”地位。
从传播学的角度讲,新浪微博碎片式的文本内容、核聚变式的传播方式以及零时差的信息发布极大地满足了用户的需求。
反过来,新浪微博邀请用户参与产品体验,对用户需求进行汇总,有效地抓住了用户对新浪微博的需求点,因而才能牢牢地抓住用户。
探析新浪微博用户及其微博特征对其他企业把握用户的兴趣、爱好及特点,确定企业产品及服务的定位具有很高的指导意义。
1 新浪微博用户的构成和特征新浪微博用户群体十分广泛,涉及影视明星、文化名人、企业高管、网络红人、普通大众等各个社会群体。
总体而言,新浪微博的用户门槛比较低,有着一定的“草根化”特征。
大部分用户都是“草根化”的个人用户。
这些个人用户是新浪微博用户的“主力军”,覆盖面广、影响力大。
具体而言,新浪微博的个人用户呈现以下几点特征。
1)以年轻人为主。
新浪微博用户中,以19~39岁的年轻用户为主体,也就是我们所说的“80后”“90后”。
处于这个年龄阶段的人热衷于追求各种新鲜事物,接受新事物能力比较强,个人自主意识强烈,喜欢赶潮流,对“互联网+”时代背景下的各种新兴事物大力追捧。
2)女性用户倾诉欲强烈。
新浪微博用户中,女性数量以及活跃度明显高于男性。
新浪微博社区公约(试行)

第一章总则第一条为维护新浪微博社区秩序,更好的保障用户合法权益,新浪微博社区管理中心(以下统称“站方”)根据现行法律法规及《新浪微博社区公约(试行)》,制定本规定。
第二条新浪微博用户在本平台的活动不得违反现行法律法规。
本平台将按照相关法律法规及用户注册协议,配合司法机关维护被侵权人合法权益。
第三条站方负责本规定的解释及执行。
第二章基本原则本规定通过以下基本原则,保障执行过程中的公正与透明:第四条本规定适用于新浪微博所有用户。
第五条完成真实身份验证的用户,可通过新浪微博提供的“举报”功能对违规行为进行举报。
完成真实身份验证的用户包括:个人认证用户、机构认证用户、微博达人、绑定手机或其他完成身份验证的用户。
第六条站方对用户举报的受理条件公开透明。
第七条对于可明显识别的违规行为,由站方直接处理;其他违规行为,由社区委员会判定后处理。
站方服从社区委员会的判定结果。
第八条违规的判定、处理公开透明。
用户可查阅除危害信息外任何违规的处理过程(自受理之后到完成处理)。
查询范围包括已完成处理的和正在处理的。
第三章社区委员会第九条社区委员会由公开招募的微博用户构成。
第十条社区委员会分为两类:判定用户纠纷的普通委员会,判定不实信息的专家委员会。
第十一条普通委员会成员总数量为5000名至10000名,专家委员会成员总数量为1000名至1500名。
第十二条在涉嫌违规行为的判定中,社区委员会在时限内以多数决的方式,形成判定结果,站方据此完成处理。
第十三条普通委员会报名及审核条件:(一) 完成真实身份验证的个人用户。
包括:个人认证用户、微博达人、绑定手机或其他完成身份验证的用户;(二) 年满18周岁;(三) 注册时间大于180天,发布微博数量大于100条;(四) 粉丝数大于50;(五) 最近一个自然月里15天有登录行为;(六) 按报名顺序选取。
第十四条专家委员会报名及审核条件:(一) 具有公开身份和一定权威性的个人认证用户和机构认证用户。
社区用户等级—勋章体系分析
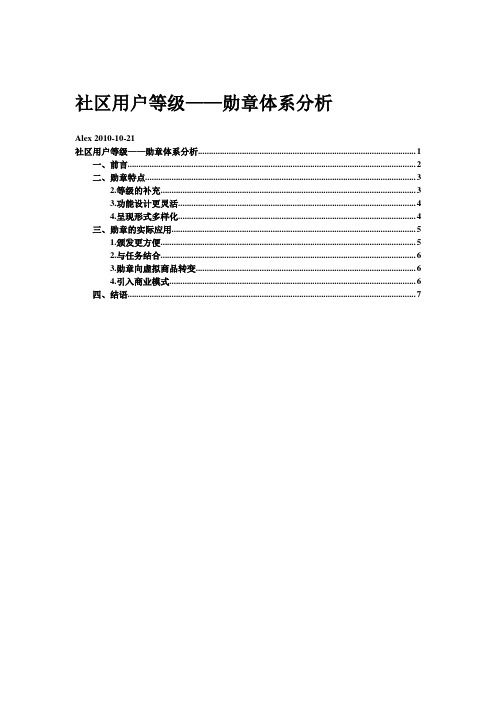
社区用户等级——勋章体系分析Alex 2010-10-21社区用户等级——勋章体系分析 (1)一、前言 (2)二、勋章特点 (3)2.等级的补充 (3)3.功能设计更灵活 (4)4.呈现形式多样化 (4)三、勋章的实际应用 (5)1.颁发更方便 (5)2.与任务结合 (6)3.勋章向虚拟商品转变 (6)4.引入商业模式 (6)四、结语 (7)一、前言随着互联网产品的发展,各种功能不断地整合,当一个产品所覆盖的功能越来越广,拥有的用户数量越来越多,它就逐步演变成了一个社区。
就像天涯社区虽然起初以论坛为主要功能,但随着发展“博客、我的天涯”这种个人产品也同样占据重要分量;又像Discuz同uchome、supesite等产品的整合,使得Discuz系列版本的社区性质更加明显。
作为一个社区,为了激励用户操作,增加用户的归属感,社区中慢慢形成了用户等级的概念,用来描述一个用户的身份属性,告诉大家谁是资深、谁是菜鸟。
同时,等级的提出不但增强了用户的荣誉感,还丰富了用户的想象空间,为社区添加了游戏化的成分,但面对社区内日益增多的功能与服务,以及用户操作行为的细化,等级的功能开始显得相形见绌。
设计者慢慢发现,在等级规则的制定上,即使再完善周密的用户等级体系也无法预见日后新的产品形态,也无法满足新产品中用户对自身等级和身份上的需求。
于是,用户勋章的提出从另一个侧面解决了这种矛盾。
给相应的用户发一枚虚拟的勋章(通常是一个精心设计的小图标,显示在用户个人信息页面中),这种做法正灵活的应用于不同的产品和网站中。
二、勋章特点1.增强用户荣誉感和用户等级的作用一样,用户勋章也是为了增强用户荣誉感、鼓励用户行为、增加用户操作趣味性而存在的,用户勋章同时也作为用户等级衡量的一个维度,但它相对等级更为灵活,其命名方式、样式设计、增加删减、机制设置等都更便捷,针对用户的需求可以及时做出调整,更加个性化。
举个例子,就像在一个poweerd by phpwind的社区里,除了系统原有的用户等级,还应用了用户勋章功能作为等级的补充,使拥有勋章的用户荣誉感更强,也激励了获得勋章用户在社区内部的进一步操作。
腾讯微博用户的特征分析
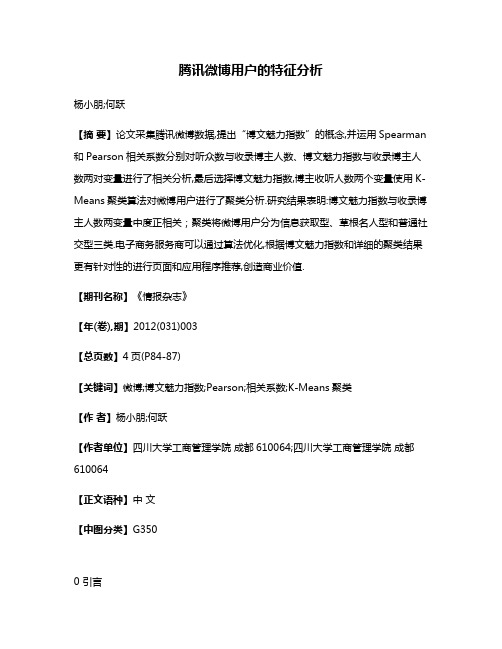
腾讯微博用户的特征分析杨小朋;何跃【摘要】论文采集腾讯微博数据,提出“博文魅力指数”的概念,并运用Spearman 和Pearson相关系数分别对听众数与收录博主人数、博文魅力指数与收录博主人数两对变量进行了相关分析,最后选择博文魅力指数,博主收听人数两个变量使用K-Means聚类算法对微博用户进行了聚类分析.研究结果表明:博文魅力指数与收录博主人数两变量中度正相关;聚类将微博用户分为信息获取型、草根名人型和普通社交型三类.电子商务服务商可以通过算法优化,根据博文魅力指数和详细的聚类结果更有针对性的进行页面和应用程序推荐,创造商业价值.【期刊名称】《情报杂志》【年(卷),期】2012(031)003【总页数】4页(P84-87)【关键词】微博;博文魅力指数;Pearson;相关系数;K-Means聚类【作者】杨小朋;何跃【作者单位】四川大学工商管理学院成都610064;四川大学工商管理学院成都610064【正文语种】中文【中图分类】G3500 引言微博具有实时性、浅易性、选择性三个倾听特质。
微博带来的是一种新的信息传播和交流方式,每个人都可以形成一个自己的听众群落,通过微博,将个人的见解和观点发布给自己的听众,以最精炼的词汇来表达最原创的观点。
因为可以随意又简单地发布与反馈信息,尤其是支持用手机随时发送信息,用户能够及时发现世界正在关注什么,或随时将发生在自己身边的事件迅速地传递给世界。
微博是继视频网站、社交网站之后新兴的开放互联网社交服务。
最早也是最著名的微博是美国的Twitter,于2006年3月由Evan Williams推出。
根据网络监测机构RJMetrics最近发布的报告显示,Twitter注册用户数目前已达7500万,信息总量突破100亿条。
腾讯微博是一个由腾讯公司推出,提供微博服务的网站。
腾讯微博注册用户突破了一亿大关。
国外对微博的研究较多,采用了很多定量的分析方法,研究的内容涉及到了广泛的领域,如商业应用模式及微博用户中社区特性的深层联系等。
超话等级规则

超话等级规则1. 背景介绍超话是新浪微博平台上的一个功能,它为用户提供了一个创建和管理自己的兴趣主题社区的机会。
用户可以创建自己感兴趣的超话,并邀请其他用户加入讨论。
为了保持超话社区的秩序和品质,新浪微博制定了一套超话等级规则。
2. 超话等级规则概述超话等级规则是指根据超话在平台上的活跃度、关注度和内容质量等因素,给予超话不同的等级称号。
根据不同等级,超话将享有不同的特权和权益。
3. 超话等级划分标准3.1 活跃度指标活跃度是衡量超话在平台上活跃程度的指标之一。
新浪微博通过以下因素来评估超话的活跃度:•发帖频率:每日、每周或每月发布帖子数量。
•成员互动:成员之间互相评论、转发和点赞帖子的频率。
•帖子热度:帖子被阅读和转发次数。
3.2 关注度指标关注度是衡量超话受欢迎程度的指标之一。
新浪微博通过以下因素来评估超话的关注度:•成员数量:超话中的成员数量。
•新成员增长率:每天或每周新成员加入的数量。
•超话标签热度:超话标签在平台上的搜索次数。
3.3 内容质量指标内容质量是衡量超话质量和影响力的重要指标。
新浪微博通过以下因素来评估超话的内容质量:•原创内容比例:超话中发布的原创内容占总帖子数量的比例。
•内容相关性:超话发布的内容与主题相关性。
•用户反馈:用户对超话发布内容进行评论、点赞和转发等行为。
4. 超话等级称号和权益根据上述指标,新浪微博将超话分为以下等级,每个等级都对应着不同的称号和权益:4.1 初级超话初级超话是活跃度、关注度和内容质量较低的超话。
它们通常是新创建不久或者没有积累足够活跃用户群体的社区。
初级超话享有以下特权:•可以自定义头像、封面和主题色。
•可以发布帖子和评论,但发帖数量有限制。
•可以邀请朋友加入超话。
4.2 中级超话中级超话是活跃度、关注度和内容质量适中的超话。
它们拥有一定的用户基础,但还需要进一步提升社区活跃度和质量。
中级超话享有以下特权:•可以自定义头像、封面和主题色。
•发帖数量限制相对较高。
微博用户特征分析与分类 - 蒋欣1,李文民2说明书

3rd International Conference on Machinery, Materials and Information Technology Applications (ICMMITA 2015) Research on Characteristics Analysis and Classification of MicroblogUsersJiang Xin1, a, Li WenMin2,b1,2State Key Laboratory of Networking and Switching TechnologyBeijing University of Posts and Telecommunications, Beijing 100876, Chinaa***************.cn,b************.cnKeywords: Microblog Users; Feature Detection; Classification Algorithm; C4.5 Decision Tree Abstract. Microblog has become an important part of social media, a large number of users send and thus spread information on this platform. Nowadays, the network environment of microblog is impacted by the presence of anomalies in users seriously. So the research on identifying the types of microblog users is of great significance. Based on the example of microblog, this paper selects some microblog users as research objects and thus analyzes and extracts the features of the selected users. Meanwhile, it uses statistical methods and classification methods in data mining to analyze user data. With the breakthrough point, the classification method C4.5 Decision Tree, this paper has trained the history data to form a classifier to make prognostic classification of new sample, which has realized high accuracy.IntroductionIn recent years, microblog has become an important part of social network with the rapid development and popularity of microblog in netizens. However, there are a lot of abnormal users in the network created by microblog. This phenomenon has been seriously affecting the normal user experience. Therefore, designing a kind of effective method to identify abnormal users effectively is of practical significance for purifying the Internet environment and improving the user experience.To some extent, the research on identifying microblog’s user type is also the research on the case of user classification. Based on users’ socialization and their choice of microblog’s text properties, Fabricio et al. held YouTube as the object to study how to identify spam and normal users in video network[1]. Gianluca Stringhini et al. studied how to forecast spam in Facebook, MySpace, Twitter through the establishment of user behavior model[2]. Liu Kan et al. used Random Forest to do a research aimed at the identification of machine-operated users, which finally achieved good results[3].The research on microblog users of domestic studies just has got started over the past two years[4], so there are some deficiencies. As for the research on the classification of user type, there are two features: qualitative research methods are widely used while quantitative research methods are seldom used; Most researches concentrate on the identification of certain kind of abnormal users and normal users[3,5], while less researches emphasize on the identification of abnormal users and normal users as a whole.The latter part of this paper is divided into three parts. The second part is mainly about the theoretical foundation and construction algorithm that the C4.5 Decision Tree involves; The third part is mainly about the extraction of the features of users and data analysis; The fourth part is mainly about using C4.5 Decision Tree to classify user data.Related KnowledgeThis section describes the relevant concepts of the main classification algorithm, C4.5 Decision Tree. C4.5[6] is the algorithm used in the classification of data mining algorithm. It can deal with attributes of discrete type and continuous type with higher accuracy. Info Gain Ratio is the main reason for node split in the process of building C4.5 Decision Tree.Info Gain.The concept of Info Gain [6] comes from information entropy. Entropy means the degree of a system in disorder. The more disordered a system becomes, the higher the entropy gets. Set S as a set having positive and negative sample. If the target attribute has n different values, the entropy of S after classification under n different conditions is defined as:21()*log ni i i Entropy S p p ==-∑Of which, i p is the proportion of S which belongs to category i .Info Gain aims to each attribute. For an attribute A , the difference between the status under which there is or not A in the computing system is the amount of information that attribute A brings about, i.e., Info Gain. The Info Gain of the relative sample set of the attribute A , S , is defined as:()||()()()|v v v Values A S Gain S,A Entropy S Entropy S S |∈=-∑ Of which, Values(A) is all possible values for the attribute A , v S is the subset of attribute A of S equalto v .Info Gain Ratio.When C4.5 Decision Tree selects features, it will use Info Gain Ratio to overcome the shortcomings when Info Gain prefers to select attributes with more values, and achieves a better result. Info Gain Ratio [6] is defined as follows: ()()()Gain S,A GainRatio S,A SplitInformation S,A =Of which, ()Gain S,A is the Info Gain of attribute A to set S . ()SplitInformation S,A is information separation measure, presenting n sample subsets from 1S to n S . Use attribute A to partition S and then we’ll get it. It’s defined as: 21||||()log ||||ni i i S S SplitInformation S,A S S ==-∑ C4.5 Algorithm.The process of C4.5 algorithm [6] constructing a decision tree is the key step to construct the decision tree classifier. Down from the root node, choose the attribute of maximum Information Gain Ratio as the node to split. Then build the tree one layer after another until all attributes run out at the leaf node. The algorithm is described as follow:C4.5 Algorithm (R : the no category attribute set ,C : class set ,S : training set)beginIf S is null, return a single node equal to failure ;If S comprises records of the same categorical attribute value, then return a singlenode with such value;If R is null, then return a single node. Its value is the categorical attribute value withthe highest frequency in the records of S ;for all attributes R (Ri ) doif attribute Ri is continuous attribute ,thenbeginassign the minimum of Ri to A1:assign the maximum of Rm to Am ;for j from 2 to m -1 do Aj =A1+j*(A1Am)/m ;assign the attribute (Ri,S ) with the maximal Info Gain based on{<=Aj ,>Aj } to A at Ri ;end ;assign the attribute (D ,S ) with the maximal Info Gain among the attributes of Rto D ;assign the value of attribute D to {dj/j=1,2...m };assign the subset of S made up of records of the value dj corresponding to Drespectively to {sj/j=1,2...m };return a tree and mark its root as D ; mark its brunch as d1,d2...dm ;then construct follow trees respectively:C4.5(R-{D},C ,S1),C4.5(R-{D},C ,S2)...C4.5(R-{D},C ,Sm ); end C4.5The Extraction of User CharacteristicsOne important step of the research on the classification of microblog users is the extraction of user characteristics. This section combines the selection of user characteristics [2-4] and introduction of every field in sina API in previous research. Then it selects user characteristics and analyze the extracted features by the means of statistics.The Extraction of User Characteristics.User characteristics extracted from sina API can present the types users belong to roundly. However, not all field information is meaningful. Considering the three important factors, microblog users, the relationship between users and microblog itself, and three concepts, social relations, behavior patterns and the content, we extract 11 user characteristics.1) Attributes Based on Social RelationsFriends Count. There are many differences in the average number of the friends count of normal users and abnormal users. Abnormal users follow lots of other users to achieve the purpose of spreading information.Followers Count. Normal users maintain normal social relations. Their microblogs are so colorful that they attract many people to follow. So abnormal users can’t have such many followers.Bi Followers Count. In the process of spam detection, foreign scholars put forward the feature as Ffratio in their paper [2] to describe the features of users on social relations. The calculation formula is as follow:___friends count ffratio bi followers count2) Attributes Based on User Behavior PatternsStatuses Count. Abnormal user always release some advertising or useless articles, they can't continue to publish microblog like normal users. So the total number of their microblogs is different. Average message sent. This attribute combines the field statuses_count and the field created_at of user interface. We divide the time (month) from the registration date and the data collection date by the total number of microblogs, and then we will get the average messages sent of users.Interval Time. This attribute gets the time of the latest microblog through the field status in user interface. Then it calculates the time difference of data collection. Abnormal users are quite different from normal users in this attribute because of the existence of the Zombies. Zombies refer to the fake fans in microblogs. These fake fans can be bought by money.Forward Rate. Divide the field statuses_count of user interface by the number of forwarded microblogs and we’ll get this attribute. Whether microblogs can be forwarded is decided by the interface field reposts_count of microblog. Among abnormal users, some advertisers produce lots of forwarded information through the forwarding of sales information of online shops on purpose.3)The Attributes Based on The Content of MicroblogsUrl ratio. Divide the field statuses_count of user interface by the number of all microblogs with links and we’ll get this attribute. The interface field text of microblogs will decide whether there is a link in a microblog.Average comments. This attribute presents the mean value of the number of comments. The number can be got through the interface field comments_count of microblogs. There are interactions between normal users and their friends. On the contrary, abnormal users don’t have real friends.Average length. This attribute presents the mean value of the length of microblogs of user. The length of microblogs can be got through the interface field text of microblog. Since abnormal users put so much ad information in their microblogs, the length of their microblogs is much longer. Normal users won’t involve so much information in their microblogs.The Analysis of User Characteristics.The selected user characteristics should have differentiation in terms of statistical properties. We adopt three commonly used statistical parameters: arithmetic mean, 10% trimmed mean, coefficient of variation[7] to distinguish normal users and abnormal users.This paper get 4137 user samples as data source for research. With the method of manual marking, artificial classification is made for the total sample, including 3613 positive samples and 524 negative samples. Calculating its statistical properties, this paper makes a list for such as shown in table 1.As is shown, according to different user attributes, normal users and abnormal users have obvious differences in statistical properties. This shows the differentiation of selected users.C4.5 Decision Tree Classification ExperimentBefore making a C4.5 Decision Tree train,we should do the attribute reduction firstly. Attribute reduction, i.e. feature selection. It refers to removing redundant or unrelated condition attributes from the whole to leave some important condition attributes behind. The reasonable dimension reducing methods mentioned in the literature [8] will make it appear on most classifiers that the result can be improved and near stationary with the increase in the number of features. However, if the number is too large, performance will decrease.C4.5 Decision Tree classifies on the basis of Info Gain Ratio as a node splitting, this paper adopts the method of Info Gain to deal with attribute reduction. Making use of the Info gain formula mentioned in section 2.1to calculate the value for Info Gain of 11 user properties, we rank the importance of the attributes.Based on the ranking, this paper uses different classification algorithm to evaluate and analyze the results of ranking. Then it get the trend based on the ranking that the number of attributes changeswith every evaluation index. This paper compares BayesNet, C4.5 Decision Tree, Logic, SMO, Adaboost, Random Forest, and other classical classification algorithm in data mining[9].In data mining, Precision, Recall, F-Measure[10]are often used to evaluating the result of classification. The figure 1-3 under different algorithms show that based on the ranking of Info Gain, the number of attributes changes with weighted Precision, Recall and F-Measure.Figure 1 Precision/Attributes Figure 2 Recall/AttributesFigure 3 F-Measure/AttributesAs is shown in the figures above, evaluation indexes don’t increase with the increasing of the number of attributes. When the number of attributes is greater than a certain value, the curve flattens. This illustrate that, the attribute set after reduction can achieve the result of total attribute set, the 11 attribute can be cut to 5 to replace all attributes.Combining the figures, C4.5 Decision Tree achieves the best result. So C4.5 Decision Tree algorithm is used to build classification rules for the classification training of user data. The process of constructing a decision tree is just like C4.5 Decision Tree algorithm in 2.3. Select the attribute with the maximal Info Gain Ratio from the continuous values as the split node. Firstly, select the attribute with maximal Info Gain Ratio as the split node in first layer. Then find out the attribute from other properties with the maximal Info Gain Ratio to be the node of next layer. After traversing all attributes, the decision tree forms. Each leaf node in the tree has the function of decision.This paper use Java to write classification program invoking weka.jar. It works in the form of 10-fold cross-validation. The decision tree contains 9 leaf nodes to decide and the decision-making system can reach the accuracy of 92.68% when predicting user type. It has reached a quite high level in the supervised classification systems.ConclusionsThis paper takes Sina Microblog as an example to do a research on its users according to the features of microblog users. After obtaining the actual user data, this paper extracts user characteristics. Then it selects the differentiated features to analyze. Last, using method of C4.5Decision Tree classification helps achieve a good result.This paper emphasizes on the attribute of samples and the research on the number of attributes and the results of classification. It doesn’t consider too much about the influence of the ratio of positive samples to negative samples on the classification results. It will cause the decision-making system tending to identify normal users if positive samples take up too much proportion. Wish researches on these topics can go much further.AcknowledgementsThis work is supported by NSFC (Grant Nos. 61300181, 61502044), the Fundamental Research Funds for the Central Universities (Grant No. 2015RC23).References[1] Fabrício Benevenuto, Tiago Rodrigues, Virgílio Almeida, Jussara Almeida and Marcos Goncalves. Detecting Spammers and Content Promoters in Online Video Social Networks [J]. SIGIR’09,July 19–23, 2009.[2] Gianluca Stringhini, Christopher Kruegel, Giovanni Vigna. Detecting Spammers on Social Networks[J]. ACSAC ’10 Dec. 6-10, 2010.[3] Liu Kan, Yuan Yunying, LIU Ping. A Weibo Bot-users Indentification Model Based on Random Forest [J],Acta Scientiarum Naturalium Universitatis Pekinensis, 2015, 51(2).[4] Peng Xi-xian, Zhu Qing-hua, Liu Xuan. Research on Behavior Characteristics and Classification of Micro-blog Users——Taking“Sina Micro-blog”as an Example [J], Information Science, 2015,33(1).[5] Guo Hao,Lu Yuliang,Wang Yu,Yang Bin. Detection of spam mutual concerns in micro-blogs based on multi-features [J], CHINA SCIENCEPAPER, 2012, 7(7).[6] Tom M. Mitchell. [M]. China Machine PRESS, 2003.[7] John A. Rice. Mathematical Statistics and Data Analysis[M]. China Machine PRESS, 2007.[8] Su JS, Zhang BF, Xu X. Advances in Machine Learning Based Text Categorization [J], Journal of Software September 2006.[9] Xindong Wu,Vipin Kumar. The Top the Algorithms in Data Mining [M]. Tsinghua University Press, 2013.[10] Y iming Yang. An Evaluation of Statistical Approaches to Text Categorization[J]. Kluwer Academic Publishers, 2000.。
新浪微博的应用详解
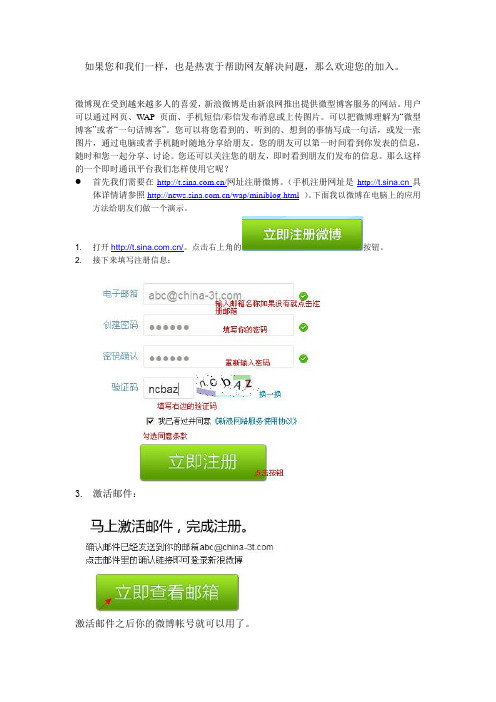
如果您和我们一样,也是热衷于帮助网友解决问题,那么欢迎您的加入。
微博现在受到越来越多人的喜爱,新浪微博是由新浪网推出提供微型博客服务的网站。
用户可以通过网页、W AP页面、手机短信/彩信发布消息或上传图片。
可以把微博理解为“微型博客”或者“一句话博客”。
您可以将您看到的、听到的、想到的事情写成一句话,或发一张图片,通过电脑或者手机随时随地分享给朋友。
您的朋友可以第一时间看到你发表的信息,随时和您一起分享、讨论。
您还可以关注您的朋友,即时看到朋友们发布的信息。
那么这样的一个即时通讯平台我们怎样使用它呢?首先我们需要在/网址注册微博。
(手机注册网址是具体详情请参照/wap/miniblog.html)。
下面我以微博在电脑上的应用方法给朋友们做一个演示。
1. 打开/。
点击右上角的按钮。
2. 接下来填写注册信息:3.激活邮件:激活邮件之后你的微博帐号就可以用了。
如果你有新浪博客帐号也可以直接登录,也可以用msn账号登录微博点击接下来填写你的msn信息连接就可以。
微博注册成功,我们为了找到更多志同道合的朋友,可以为自己加一些个人标签:用途:标签是自定义描述自己职业、兴趣爱好的关键词。
可以让更多人找到你,让你找到更多同类。
已经添加的标签将显示在“我的微博”页面右侧栏中,方便大家了解你。
添加方法:登陆后点击账号设置——个人标签。
限制:每个账户最多可添加10个标签,单个标签最多可输入7个汉字。
添加标签后,点“将我的标签推荐给朋友”,点击后,所有标签会形成一条微博。
微博注册成功了,我们怎样发微博呢?简单的微博应用如图:(一)添加图片时点击图片出现一个下拉框,提示你有两种方法上传图片。
1)从电脑上传:还可以为你的图片添加水印:当您在微博中发布照片后,如果该图片宽(或高)大于300像素时,系统会自动在图片右下角添加您微博地址的水印信息。
若图片尺寸不足300像素,为了避免影响图片效果,系统不添加水印信息。
图片水印2)还有一种选择就是添加推荐配图里的图片,点击推荐配图会出现各种形式的图片(二)添加视频有两种方法:1)输入视频网站播放页链接地址发布把视频链接输入到编辑器内(必须是以.html结尾的链接),点击“发送”即可。
解读新浪微博会员成长体系
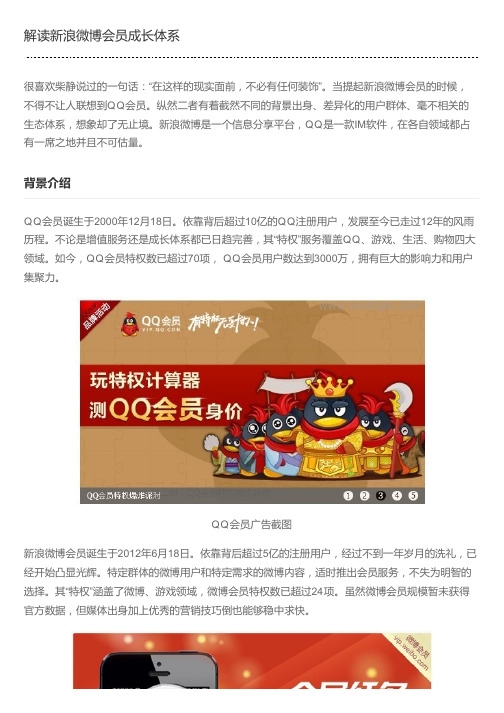
新浪微博会员广告截图不看内核看表象从“微博会员,精彩你的微博生活”的广告语中不难看出,新浪微博会员服务于“你”,是为了更好地服务于“你”的微博。
微博可以是一个名词,也可以是一个动词。
当用户决定花钱为此买单的时候,ta 就仅仅只是“你”的一个消费品而已。
无关风月,只关乎需求。
既然如此,又是何种力量促使“你”萌动掏钱的欲望?1) 特殊标识,凸显独特个性;2) 增加曝光量,增加粉丝以及评论(微博广场等的推荐);3) LV级别能在图标上有显示,升级优先;4) 建立以相同性格或乐趣为基础的人脉关系圈;……你有你的态度,我有我的风格。
要想在这些细微的出发点里面提炼合理的需求,不光是微博会员面临的最现实问题,也是新浪微博今后发展的责任所在。
但要想做好微博会员,试水、猜测、盲目都显得苍白无力,唯有真正了解用户才是王道。
取悦用户是本质需求的提炼在精不在多,还要贯穿于“你”的整个体系。
在QQ会员特权中,有大量暗藏精明的设计。
比如让Q币更便宜、信用卡提供的多倍积分、买电影票折扣更高等等。
最聪明的网络生存高手恐怕正是QQ会员群体,他们的生活方式代表了网络应用的风向标。
从QQ会员的年龄分布来看,17-24岁是最大的区间。
这部分群体对新生事物接受能力强,然而年轻人的生活总会面临各种选择和困惑。
当他们迷茫无助时,内心滋长的是不服输的意志。
而微博会员的年龄分布与QQ会员的有小小的差异,18-26岁是主体成员。
他们推崇实用主义至上,以满足个人需求为准。
对新鲜刺激的事物偏好性降低,以追求稳定顺其自然为主。
他们用自己的视角看世间万物,内心祈求更多的是对生活的美好向往。
就像一个普通用户。
如果他上微博是为了更方便的向朋友和其他人展示自己的摄影成果,那么上传照片就是他的基本需求,而批量上传就是一个很实用的功能。
在微博会员体系中,虽然有部分特权靠近用户需求,但不贴近用户生活。
专属标识、专属模板、专属勋章、语音微博、短信特别关注……这些都难以抵达用户内心所需,貌似只是做了表面功夫。
- 1、下载文档前请自行甄别文档内容的完整性,平台不提供额外的编辑、内容补充、找答案等附加服务。
- 2、"仅部分预览"的文档,不可在线预览部分如存在完整性等问题,可反馈申请退款(可完整预览的文档不适用该条件!)。
- 3、如文档侵犯您的权益,请联系客服反馈,我们会尽快为您处理(人工客服工作时间:9:00-18:30)。
可以看到活跃天数的获得有三种方式:
1. 登录后在线时长
2. 发表微博奖励
3. 连续登录奖励
好吧,这里暂且不谈是否参考了其他公司或产品的用户等级激励成长体系,但是从这里的计算规则来看,这里还是费了一番心思的——在提高用户活跃度上有很多思考和动作。
那么现在新浪微博上是否只有这一种用户激励成长体系呢?下面就已知的几种激励,做一个简单的梳理。
1. V认证用户:新浪微博在最早推出的时候,就拥有了V 认证用户,一度,那个黄色的小V 让无数
人竟折腰。
在某种场合,加V 用户俨然是一种炫耀的资本,尤其是一些小有名气又不太知名的社会人士,一度将微博作为提升自身形象和知名度宣传的一个重要途径。
因为加V代表着身份,代表着话语权,代表着是少数的人,代表着“精英”身份……但最近和朋友聊天,已经有很多朋友陆续说加V已经不值钱了,甚至有的想去V。
记得最早听说加V的条件是——500人以上公司总监级别人士才可以凭借身份证明信息加V认证。
但是目前经常看到以下某某公司销售、某某公司运维字样的认证描述,此时笔者更愿意相信加V 认证已经变成一种很贴近草根的用户激励方式。
2. 微博达人:这应该是最早面向草根的用户激励体系吧,根据官方显示的帮助资料,达人只需满足一定条件如:绑定手机、真实头像、粉丝数达到100等即可申请。
而且具有完整的积分和升级规则——但貌似这一规则没有在网站上公示,我们只找到以下一段文字:“达人积分是根据作为微博达人在微博上的活跃度(登陆、发原创微博、评论)和社区产品活跃度(微群、活动)的使用情况和积分规则,系统自动统计计算出来的,具体积分规则和计算方法请见:/intro,可以在个人微博首页(我的微博)页面查询,也可以在微博达人首页查询“。
而在指定页面也没有关于达人积分的详细描述。
相比V认证用户,达人用户目前在web版微博有展示,但是在任何客户端软件等场合均没有展示。
3. 勋章激励,微博勋章很多时候扮演的是与其他激励相搭配的角色,如:与”微博达人“ 体系搭配的初级达人勋章、中级达人勋章、高级达人勋章等;与”微号“ 和 ”NBA活动“ 等搭配的活动勋章等。
众多勋章当前都有收回机制,而且勋章激励的展示入口貌似也只有web界面,所以,严格来说,勋章系统应该不算一种很独立完整的激励吧。
4. 微博等级,也就是本文开头提到的用户等级概念。
当前没有展示入口,只是
在/ 页面中,用户可以看到自己和相关好友的微博等级。
但这里有一个相对冲突的问题。
就是,微博等级也是按照活跃天数和发微博量等指标来升级的,而”微博达人“激励的部分指标与此冲突。
后期,新浪微博是否会在”微博等级“ 和 ”微博达人“ 两个体系中做出取舍?有待观察。
5. 企业和机构等认证,也就是我们常说的蓝V,由于笔者没有蓝V 的运营帐号,所以无法探知具体的用户成长规则。
但可以了解的是,蓝V 和黄V (名人认证)在终端展示和用户认知度上有较高的
重合。
下面是就以上几种激励体系做出的一个对比表格对比:
可以很明显的看到,几种激励体系已经有了很多交叉,就像前文提到的”微博达人“ 和”微博等级“ 甚至已经造成了交叉冲突。
不知道新浪微博是否也会在分析用户激励体系的时候产生和笔者同样的感觉或想法?
用户激励的目标在于提高用户的活跃度和参与度,尤其作为UGC类型的内容社区来说,提高用户活跃度也就可能意味着更加丰富和高质量的内容。
我们可以看到在人人网(原校内网)、Qzone、腾讯微博、新浪微博等社交网络都存在用户激励体系,而且在在有些平台上确实能有效的提高用户参与的积极性。
但是笔者认为,新浪微博的用户激励体系目前可能过于复杂甚至出现重叠交叉,而且众多的激励体系相关展现方式、以及积分消费等都没有做得很完善——甚至关注过多以后,用户容易产生”迷惑“。
相信在后续的开发和产品迭代中,新浪微博会理清相关激励体系并提供更简洁的版本。
同时,也希望能将适当的激励体系的展现进行拓展,增加展现场景,真正提高用户对激励的认可度,以便用户更好的参与进去。
由于时间和篇幅所限,这里就不对各大社交网站和平台的用户激励体系相关做对比研究。
不足之处,也望读者多加批评指教。
作者:刘三德
人人都是产品经理()中国最大最活跃的产品经理学习、交流、分享平台。