A Scalable Framework for Information Visualization
阿里莱德斯管理框架(AMF)说明书
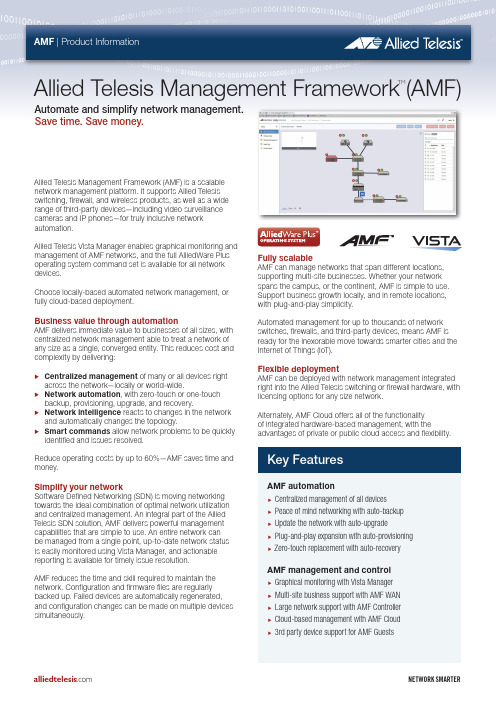
Allied Telesis Management Framework (AMF) is a scalable network management platform. It supports Allied Telesis switching, firewall, and wireless products, as well as a wide range of third-party devices—including video surveillance cameras and IP phones—for truly inclusive network automation.Allied Telesis Vista Manager enables graphical monitoring and management of AMF networks, and the full AlliedWare Plus operating system command set is available for all network devices.Choose locally-based automated network management, or fully cloud-based deployment.Business value through automationAMF delivers immediate value to businesses of all sizes, with centralized network management able to treat a network of any size as a single, converged entity. This reduces cost and complexity by delivering:ۼCentralized management of many or all devices right across the network—locally or world-wide.ۼNetwork automation , with zero-touch or one-touch backup, provisioning, upgrade, and recovery.ۼNetwork intelligence reacts to changes in the network and automatically changes the topology.ۼSmart commands allow network problems to be quickly identified and issues resolved.Reduce operating costs by up to 60%—AMF saves time and money.Simplify your networkSoftware Defined Networking (SDN) is moving networking towards the ideal combination of optimal network utilization and centralized management. An integral part of the Allied Telesis SDN solution, AMF delivers powerful management capabilities that are simple to use. An entire network can be managed from a single point, up-to-date network status is easily monitored using Vista Manager, and actionable reporting is available for timely issue resolution.AMF reduces the time and skill required to maintain the network. Configuration and firmware files are regularly backed up. Failed devices are automatically regenerated, and configuration changes can be made on multiple devices simultaneously.AMF automationۼCentralized management of all devicesۼPeace of mind networking with auto-backup ۼUpdate the network with auto-upgradeۼPlug-and-play expansion with auto-provisioning ۼZero-touch replacement with auto-recovery AMF management and control ۼGraphical monitoring with Vista Manager ۼMulti-site business support with AMF WAN ۼLarge network support with AMF Controller ۼCloud-based management with AMF Cloud ۼ3rd party device support for AMF GuestsFully scalableAMF can manage networks that span different locations, supporting multi-site businesses. Whether your network spans the campus, or the continent, AMF is simple to use. Support business growth locally, and in remote locations, with plug-and-play simplicity.Automated management for up to thousands of network switches, firewalls, and third-party devices, means AMF is ready for the inexorable move towards smarter cities and the Internet of Things (IoT).Flexible deploymentAMF can be deployed with network management integrated right into the Allied Telesis switching or firewall hardware, with licensing options for any size network.Alternately, AMF Cloud offers all of the functionality of integrated hardware-based management, with theadvantages of private or public cloud access and flexibility.alliedtelesis .comNETWORK SMARTERAllied Telesis Management Framework (AMF)Automate and simplify network management. Save time. Save money.AMF | Product InformationKey FeaturesTM2 | AMFVISTA MANAGERۼThe Allied Telesis graphical management tool, Vista Manager, provides visual management of an AMFnetwork. Users can monitor and manage networkswitches and firewalls, as well as wireless AccessPoints (APs). Manage many or all devices at once, while actionable reporting highlights any problems requiringattention. Vista Manager version 2.0* will add intuitiveusability with an overview dashboard, simple navigation, and further network information—all just a click away. AMF WANۼThe benefits of AMF are not limited to the LAN. AMF networks can also tunnel across the internet so thatdevices in remote locations can be easily managed froma central location—perfect for multi-site businesses. Thetunneled links can even reach across external (non AMF) routers for complete deployment flexibility.ۼRemote offices can be connected to a head office with encrypted connections for secure company-wideautomated network management. Any size AMF network can extend across different locations and different time zones.AMF ControllerۼAn AMF network with a single Master can support up to 300 devices, which is ideal for small to medium-sized Enterprise networks. For larger networks, the AMFController extends the benefits of AMF to over 7,000devices. It enables time and money to be saved on amuch larger scale, as the entire network (whether local, city-wide, or global) takes advantage of centralizedmanagement, and automated network back-up,upgrade, provisioning, and recovery.AMF CloudۼAMF Cloud allows the AMF Master and/or Controller to be virtual appliances, rather than integrated into an Allied Telesis switch or firewall. AMF Cloud offers allof the functionality of integrated hardware-based AMF network management, with the advantages ofcloud-based access and flexibility. Powerful benefitsinclude:ۼFlexible deployment with private or public cloud installation—use your own local server, or deploy fully online with Amazon web services.ۼScalable for any size network, with multiple licensing options.ۼLower cost of entry with no dedicated hardware requirements.ۼReduce costs with simple pay-as-you-go.ۼWeb-based interface for remote network monitoring and management—anywhere, anytime.ۼPeace of mind networking with a full back-up stored in the cloud.ۼEco-friendly—cloud deployment requires no rack space, cooling or power.AMF GuestsۼAMF guestnode allows Allied Telesis wireless APs and additional switching products, as well as third-partydevices such as IP phones and security cameras, to be part of an AMF network. AMF guests are recognizedby the network, providing a broader view of connected devices, and certain Allied Telesis wireless productsutilize selected AMF automated features.ۼAllied Telesis will innovate and deliver further AMF functionality to third-party devices.The power of AMF extends beyond simplifying and automating network administration. With features such asgraphical monitoring and management through Vista Manager, large global network support, cloud-based networkcontrol, and the addition of 3rd party devices, AMF is a powerful and comprehensive network management solution.* Vista Manager version 2.0 available end of 2016AMF | 3 NETWORK SMARTER4 | AMFAMF | 5NETWORK SMARTERAMF product compatibility matrix for AlliedWare PlusAMF is available for the following Allied Telesis products, running the advancedAlliedWare Plus™ operating system:1 CentreCOM products support AMF edge. AMF edge is for products used at the edge of the network, and only support a single AMF link. They cannot use cross links or virtual links.6 | AMF617-000610 Rev ENETWORK SMARTERalliedtelesis .com© 2016 Allied Telesis, Inc. All rights reserved. Information in this document is subject to change without notice. All company names, logos, and product designs that are trademarks or registered trademarks are the property of their respective owners.North America Headquarters | 19800 North Creek Parkway | Suite 100 | Bothell | WA 98011 | USA | T: +1 800 424 4284 | F: +1 425 481 3895Asia-Pacific Headquarters | 11 Tai Seng Link | Singapore | 534182 | T: +65 6383 3832 | F: +65 6383 3830EMEA & CSA Operations | Incheonweg 7 | 1437 EK Rozenburg | The Netherlands | T: +31 20 7950020 |F: +31 20 7950021AMF Cloud Master and Controller LicensesTo find out more about AMF and how it can improve your network, please contact your local Allied Telesis sales office or visit us online at /amfAMF Cloud server specificationsThe following Virtual Machine (VM) environment is required to install and run AMF Cloud.。
m3e向量检索方案

m3e向量检索方案English Answer:M3E Vector Retrieval Architecture.The M3E vector retrieval architecture is a powerful framework for efficient and accurate retrieval of semantically similar documents from a large document corpus. This architecture combines three key components:1. Transformer Encoder:The transformer encoder is a deep learning model that converts the input documents into dense vectors. These vectors capture the semantic meaning of the documents, allowing for efficient and effective similarity comparisons.2. Memory Bank:The memory bank stores a collection of vectorsrepresenting documents from a pre-defined corpus. This memory bank provides a rich source of candidate documentsfor retrieval.3. Retrieval Function:The retrieval function computes the similarity between the query vector and the vectors in the memory bank. The resulting similarity scores are used to rank and retrievethe most relevant documents.Key Advantages of M3E:Semantic Similarity: The transformer encoder captures the semantic meaning of documents, enabling retrieval based on conceptual equivalence rather than exact keyword matches.Efficiency: The dense vector representations allow for fast similarity computations, making M3E suitable forlarge-scale retrieval scenarios.Scalability: The architecture is highly scalable,allowing for the incorporation of new documents into the memory bank and the efficient handling of growing document collections.Applications of M3E:M3E has a wide range of applications, including:Search engines.Recommender systems.Chatbots.Question answering systems.中文回答:M3E向量检索架构。
UTS-702电话手柄使用说明书

ANSI/IEEE C95.3-1992, IEEE Recommended
Key Lock .............................................................................................. 33 Security ID .......................................................................................... 34 Dial Lock .............................................................................................. 35 To activate dial lock ........................................................................ 35 To deactivate dial lock .................................................................... 36 Phonebook Lock ................................................................................. 37 Other Function Settings ...................................................................... 38 Zone-in tone ..................................................................................... 38 Echo suppression ............................................................................. 39 Charge check tone .......................................................................... 40 Key tone ............................................................................................ 41 Calling line Identification ................................................................ 42 Auto call ........................................................................................... 43 Any key answer ............................................................................... 44 Power saving ................................................................................... 45 TAM (Telephone Answering Machine) ........................................... 46 Recording your outgoing message ............................................... 46 Activating TAM ............................................................................... 47 Erasing the outgoing message ...................................................... 47 Setting the delay time .................................................................... 48 Setting the ring tone type .............................................................. 49 Playing back and erasing the (ICM) incoming message ........... 50 Voice Memo ..................................................................................... 51 Recording a Voice Memo ............................................................. 51 Playing back or erasing a Voice Memo ...................................... 52 ISDN Sub-address Selling ............................................................... 53 Activating sub-address setting ....................................................... 53 Storing the phone number of ISDN station .................................. 54 Making a call by specifying sub-address ..................................... 55 Setting Check .................................................................................... 56 Home Antenna Mode ...................................................................... 57 Registering with home antenna ..................................................... 57 Clearing registration ....................................................................... 58 Selecting Home Antenna mode ..................................................... 59
高三英语信息技术单选题50题
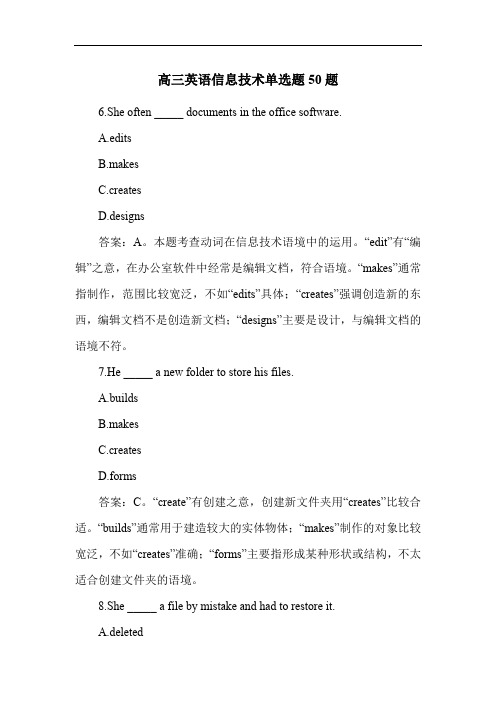
高三英语信息技术单选题50题6.She often _____ documents in the office software.A.editsB.makesC.createsD.designs答案:A。
本题考查动词在信息技术语境中的运用。
“edit”有“编辑”之意,在办公室软件中经常是编辑文档,符合语境。
“makes”通常指制作,范围比较宽泛,不如“edits”具体;“creates”强调创造新的东西,编辑文档不是创造新文档;“designs”主要是设计,与编辑文档的语境不符。
7.He _____ a new folder to store his files.A.buildsB.makesC.createsD.forms答案:C。
“create”有创建之意,创建新文件夹用“creates”比较合适。
“builds”通常用于建造较大的实体物体;“makes”制作的对象比较宽泛,不如“creates”准确;“forms”主要指形成某种形状或结构,不太适合创建文件夹的语境。
8.She _____ a file by mistake and had to restore it.A.deletedB.removedC.lostD.discarded答案:A。
“delete”表示删除,不小心删除了文件符合语境。
“removed”通常指移除某个物体,不一定是删除文件;“lost”是丢失,不一定是主动删除导致的;“discarded”侧重于丢弃不要的东西,不如“deleted”准确。
9.He _____ the file to another location.A.movedB.shiftedC.transferredD.carried答案:C。
“transfer”有转移、传送之意,把文件转移到另一个位置用“transferred”比较恰当。
“moved”和“shifted”比较笼统,没有“transfer”在信息技术语境中那么准确;“carried”通常指携带,不太适合文件转移的语境。
OSHA现场作业手册说明书
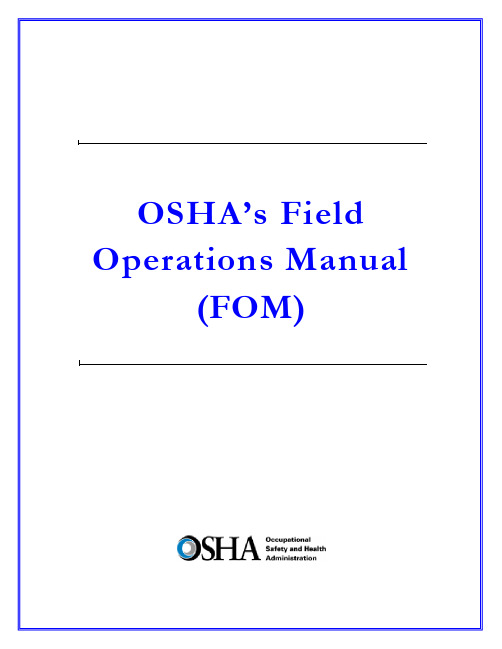
DIRECTIVE NUMBER: CPL 02-00-150 EFFECTIVE DATE: April 22, 2011 SUBJECT: Field Operations Manual (FOM)ABSTRACTPurpose: This instruction cancels and replaces OSHA Instruction CPL 02-00-148,Field Operations Manual (FOM), issued November 9, 2009, whichreplaced the September 26, 1994 Instruction that implemented the FieldInspection Reference Manual (FIRM). The FOM is a revision of OSHA’senforcement policies and procedures manual that provides the field officesa reference document for identifying the responsibilities associated withthe majority of their inspection duties. This Instruction also cancels OSHAInstruction FAP 01-00-003 Federal Agency Safety and Health Programs,May 17, 1996 and Chapter 13 of OSHA Instruction CPL 02-00-045,Revised Field Operations Manual, June 15, 1989.Scope: OSHA-wide.References: Title 29 Code of Federal Regulations §1903.6, Advance Notice ofInspections; 29 Code of Federal Regulations §1903.14, Policy RegardingEmployee Rescue Activities; 29 Code of Federal Regulations §1903.19,Abatement Verification; 29 Code of Federal Regulations §1904.39,Reporting Fatalities and Multiple Hospitalizations to OSHA; and Housingfor Agricultural Workers: Final Rule, Federal Register, March 4, 1980 (45FR 14180).Cancellations: OSHA Instruction CPL 02-00-148, Field Operations Manual, November9, 2009.OSHA Instruction FAP 01-00-003, Federal Agency Safety and HealthPrograms, May 17, 1996.Chapter 13 of OSHA Instruction CPL 02-00-045, Revised FieldOperations Manual, June 15, 1989.State Impact: Notice of Intent and Adoption required. See paragraph VI.Action Offices: National, Regional, and Area OfficesOriginating Office: Directorate of Enforcement Programs Contact: Directorate of Enforcement ProgramsOffice of General Industry Enforcement200 Constitution Avenue, NW, N3 119Washington, DC 20210202-693-1850By and Under the Authority ofDavid Michaels, PhD, MPHAssistant SecretaryExecutive SummaryThis instruction cancels and replaces OSHA Instruction CPL 02-00-148, Field Operations Manual (FOM), issued November 9, 2009. The one remaining part of the prior Field Operations Manual, the chapter on Disclosure, will be added at a later date. This Instruction also cancels OSHA Instruction FAP 01-00-003 Federal Agency Safety and Health Programs, May 17, 1996 and Chapter 13 of OSHA Instruction CPL 02-00-045, Revised Field Operations Manual, June 15, 1989. This Instruction constitutes OSHA’s general enforcement policies and procedures manual for use by the field offices in conducting inspections, issuing citations and proposing penalties.Significant Changes∙A new Table of Contents for the entire FOM is added.∙ A new References section for the entire FOM is added∙ A new Cancellations section for the entire FOM is added.∙Adds a Maritime Industry Sector to Section III of Chapter 10, Industry Sectors.∙Revises sections referring to the Enhanced Enforcement Program (EEP) replacing the information with the Severe Violator Enforcement Program (SVEP).∙Adds Chapter 13, Federal Agency Field Activities.∙Cancels OSHA Instruction FAP 01-00-003, Federal Agency Safety and Health Programs, May 17, 1996.DisclaimerThis manual is intended to provide instruction regarding some of the internal operations of the Occupational Safety and Health Administration (OSHA), and is solely for the benefit of the Government. No duties, rights, or benefits, substantive or procedural, are created or implied by this manual. The contents of this manual are not enforceable by any person or entity against the Department of Labor or the United States. Statements which reflect current Occupational Safety and Health Review Commission or court precedents do not necessarily indicate acquiescence with those precedents.Table of ContentsCHAPTER 1INTRODUCTIONI.PURPOSE. ........................................................................................................... 1-1 II.SCOPE. ................................................................................................................ 1-1 III.REFERENCES .................................................................................................... 1-1 IV.CANCELLATIONS............................................................................................. 1-8 V. ACTION INFORMATION ................................................................................. 1-8A.R ESPONSIBLE O FFICE.......................................................................................................................................... 1-8B.A CTION O FFICES. .................................................................................................................... 1-8C. I NFORMATION O FFICES............................................................................................................ 1-8 VI. STATE IMPACT. ................................................................................................ 1-8 VII.SIGNIFICANT CHANGES. ............................................................................... 1-9 VIII.BACKGROUND. ................................................................................................. 1-9 IX. DEFINITIONS AND TERMINOLOGY. ........................................................ 1-10A.T HE A CT................................................................................................................................................................. 1-10B. C OMPLIANCE S AFETY AND H EALTH O FFICER (CSHO). ...........................................................1-10B.H E/S HE AND H IS/H ERS ..................................................................................................................................... 1-10C.P ROFESSIONAL J UDGMENT............................................................................................................................... 1-10E. W ORKPLACE AND W ORKSITE ......................................................................................................................... 1-10CHAPTER 2PROGRAM PLANNINGI.INTRODUCTION ............................................................................................... 2-1 II.AREA OFFICE RESPONSIBILITIES. .............................................................. 2-1A.P ROVIDING A SSISTANCE TO S MALL E MPLOYERS. ...................................................................................... 2-1B.A REA O FFICE O UTREACH P ROGRAM. ............................................................................................................. 2-1C. R ESPONDING TO R EQUESTS FOR A SSISTANCE. ............................................................................................ 2-2 III. OSHA COOPERATIVE PROGRAMS OVERVIEW. ...................................... 2-2A.V OLUNTARY P ROTECTION P ROGRAM (VPP). ........................................................................... 2-2B.O NSITE C ONSULTATION P ROGRAM. ................................................................................................................ 2-2C.S TRATEGIC P ARTNERSHIPS................................................................................................................................. 2-3D.A LLIANCE P ROGRAM ........................................................................................................................................... 2-3 IV. ENFORCEMENT PROGRAM SCHEDULING. ................................................ 2-4A.G ENERAL ................................................................................................................................................................. 2-4B.I NSPECTION P RIORITY C RITERIA. ..................................................................................................................... 2-4C.E FFECT OF C ONTEST ............................................................................................................................................ 2-5D.E NFORCEMENT E XEMPTIONS AND L IMITATIONS. ....................................................................................... 2-6E.P REEMPTION BY A NOTHER F EDERAL A GENCY ........................................................................................... 2-6F.U NITED S TATES P OSTAL S ERVICE. .................................................................................................................. 2-7G.H OME-B ASED W ORKSITES. ................................................................................................................................ 2-8H.I NSPECTION/I NVESTIGATION T YPES. ............................................................................................................... 2-8 V.UNPROGRAMMED ACTIVITY – HAZARD EVALUATION AND INSPECTION SCHEDULING ............................................................................ 2-9 VI.PROGRAMMED INSPECTIONS. ................................................................... 2-10A.S ITE-S PECIFIC T ARGETING (SST) P ROGRAM. ............................................................................................. 2-10B.S CHEDULING FOR C ONSTRUCTION I NSPECTIONS. ..................................................................................... 2-10C.S CHEDULING FOR M ARITIME I NSPECTIONS. ............................................................................. 2-11D.S PECIAL E MPHASIS P ROGRAMS (SEP S). ................................................................................... 2-12E.N ATIONAL E MPHASIS P ROGRAMS (NEP S) ............................................................................... 2-13F.L OCAL E MPHASIS P ROGRAMS (LEP S) AND R EGIONAL E MPHASIS P ROGRAMS (REP S) ............ 2-13G.O THER S PECIAL P ROGRAMS. ............................................................................................................................ 2-13H.I NSPECTION S CHEDULING AND I NTERFACE WITH C OOPERATIVE P ROGRAM P ARTICIPANTS ....... 2-13CHAPTER 3INSPECTION PROCEDURESI.INSPECTION PREPARATION. .......................................................................... 3-1 II.INSPECTION PLANNING. .................................................................................. 3-1A.R EVIEW OF I NSPECTION H ISTORY .................................................................................................................... 3-1B.R EVIEW OF C OOPERATIVE P ROGRAM P ARTICIPATION .............................................................................. 3-1C.OSHA D ATA I NITIATIVE (ODI) D ATA R EVIEW .......................................................................................... 3-2D.S AFETY AND H EALTH I SSUES R ELATING TO CSHO S.................................................................. 3-2E.A DVANCE N OTICE. ................................................................................................................................................ 3-3F.P RE-I NSPECTION C OMPULSORY P ROCESS ...................................................................................................... 3-5G.P ERSONAL S ECURITY C LEARANCE. ................................................................................................................. 3-5H.E XPERT A SSISTANCE. ........................................................................................................................................... 3-5 III. INSPECTION SCOPE. ......................................................................................... 3-6A.C OMPREHENSIVE ................................................................................................................................................... 3-6B.P ARTIAL. ................................................................................................................................................................... 3-6 IV. CONDUCT OF INSPECTION .............................................................................. 3-6A.T IME OF I NSPECTION............................................................................................................................................. 3-6B.P RESENTING C REDENTIALS. ............................................................................................................................... 3-6C.R EFUSAL TO P ERMIT I NSPECTION AND I NTERFERENCE ............................................................................. 3-7D.E MPLOYEE P ARTICIPATION. ............................................................................................................................... 3-9E.R ELEASE FOR E NTRY ............................................................................................................................................ 3-9F.B ANKRUPT OR O UT OF B USINESS. .................................................................................................................... 3-9G.E MPLOYEE R ESPONSIBILITIES. ................................................................................................. 3-10H.S TRIKE OR L ABOR D ISPUTE ............................................................................................................................. 3-10I. V ARIANCES. .......................................................................................................................................................... 3-11 V. OPENING CONFERENCE. ................................................................................ 3-11A.G ENERAL ................................................................................................................................................................ 3-11B.R EVIEW OF A PPROPRIATION A CT E XEMPTIONS AND L IMITATION. ..................................................... 3-13C.R EVIEW S CREENING FOR P ROCESS S AFETY M ANAGEMENT (PSM) C OVERAGE............................. 3-13D.R EVIEW OF V OLUNTARY C OMPLIANCE P ROGRAMS. ................................................................................ 3-14E.D ISRUPTIVE C ONDUCT. ...................................................................................................................................... 3-15F.C LASSIFIED A REAS ............................................................................................................................................. 3-16VI. REVIEW OF RECORDS. ................................................................................... 3-16A.I NJURY AND I LLNESS R ECORDS...................................................................................................................... 3-16B.R ECORDING C RITERIA. ...................................................................................................................................... 3-18C. R ECORDKEEPING D EFICIENCIES. .................................................................................................................. 3-18 VII. WALKAROUND INSPECTION. ....................................................................... 3-19A.W ALKAROUND R EPRESENTATIVES ............................................................................................................... 3-19B.E VALUATION OF S AFETY AND H EALTH M ANAGEMENT S YSTEM. ....................................................... 3-20C.R ECORD A LL F ACTS P ERTINENT TO A V IOLATION. ................................................................................. 3-20D.T ESTIFYING IN H EARINGS ................................................................................................................................ 3-21E.T RADE S ECRETS. ................................................................................................................................................. 3-21F.C OLLECTING S AMPLES. ..................................................................................................................................... 3-22G.P HOTOGRAPHS AND V IDEOTAPES.................................................................................................................. 3-22H.V IOLATIONS OF O THER L AWS. ....................................................................................................................... 3-23I.I NTERVIEWS OF N ON-M ANAGERIAL E MPLOYEES .................................................................................... 3-23J.M ULTI-E MPLOYER W ORKSITES ..................................................................................................................... 3-27 K.A DMINISTRATIVE S UBPOENA.......................................................................................................................... 3-27 L.E MPLOYER A BATEMENT A SSISTANCE. ........................................................................................................ 3-27 VIII. CLOSING CONFERENCE. .............................................................................. 3-28A.P ARTICIPANTS. ..................................................................................................................................................... 3-28B.D ISCUSSION I TEMS. ............................................................................................................................................ 3-28C.A DVICE TO A TTENDEES .................................................................................................................................... 3-29D.P ENALTIES............................................................................................................................................................. 3-30E.F EASIBLE A DMINISTRATIVE, W ORK P RACTICE AND E NGINEERING C ONTROLS. ............................ 3-30F.R EDUCING E MPLOYEE E XPOSURE. ................................................................................................................ 3-32G.A BATEMENT V ERIFICATION. ........................................................................................................................... 3-32H.E MPLOYEE D ISCRIMINATION .......................................................................................................................... 3-33 IX. SPECIAL INSPECTION PROCEDURES. ...................................................... 3-33A.F OLLOW-UP AND M ONITORING I NSPECTIONS............................................................................................ 3-33B.C ONSTRUCTION I NSPECTIONS ......................................................................................................................... 3-34C. F EDERAL A GENCY I NSPECTIONS. ................................................................................................................. 3-35CHAPTER 4VIOLATIONSI. BASIS OF VIOLATIONS ..................................................................................... 4-1A.S TANDARDS AND R EGULATIONS. .................................................................................................................... 4-1B.E MPLOYEE E XPOSURE. ........................................................................................................................................ 4-3C.R EGULATORY R EQUIREMENTS. ........................................................................................................................ 4-6D.H AZARD C OMMUNICATION. .............................................................................................................................. 4-6E. E MPLOYER/E MPLOYEE R ESPONSIBILITIES ................................................................................................... 4-6 II. SERIOUS VIOLATIONS. .................................................................................... 4-8A.S ECTION 17(K). ......................................................................................................................... 4-8B.E STABLISHING S ERIOUS V IOLATIONS ............................................................................................................ 4-8C. F OUR S TEPS TO BE D OCUMENTED. ................................................................................................................... 4-8 III. GENERAL DUTY REQUIREMENTS ............................................................. 4-14A.E VALUATION OF G ENERAL D UTY R EQUIREMENTS ................................................................................. 4-14B.E LEMENTS OF A G ENERAL D UTY R EQUIREMENT V IOLATION.............................................................. 4-14C. U SE OF THE G ENERAL D UTY C LAUSE ........................................................................................................ 4-23D.L IMITATIONS OF U SE OF THE G ENERAL D UTY C LAUSE. ..............................................................E.C LASSIFICATION OF V IOLATIONS C ITED U NDER THE G ENERAL D UTY C LAUSE. ..................F. P ROCEDURES FOR I MPLEMENTATION OF S ECTION 5(A)(1) E NFORCEMENT ............................ 4-25 4-27 4-27IV.OTHER-THAN-SERIOUS VIOLATIONS ............................................... 4-28 V.WILLFUL VIOLATIONS. ......................................................................... 4-28A.I NTENTIONAL D ISREGARD V IOLATIONS. ..........................................................................................4-28B.P LAIN I NDIFFERENCE V IOLATIONS. ...................................................................................................4-29 VI. CRIMINAL/WILLFUL VIOLATIONS. ................................................... 4-30A.A REA D IRECTOR C OORDINATION ....................................................................................................... 4-31B.C RITERIA FOR I NVESTIGATING P OSSIBLE C RIMINAL/W ILLFUL V IOLATIONS ........................ 4-31C. W ILLFUL V IOLATIONS R ELATED TO A F ATALITY .......................................................................... 4-32 VII. REPEATED VIOLATIONS. ...................................................................... 4-32A.F EDERAL AND S TATE P LAN V IOLATIONS. ........................................................................................4-32B.I DENTICAL S TANDARDS. .......................................................................................................................4-32C.D IFFERENT S TANDARDS. .......................................................................................................................4-33D.O BTAINING I NSPECTION H ISTORY. .....................................................................................................4-33E.T IME L IMITATIONS..................................................................................................................................4-34F.R EPEATED V. F AILURE TO A BATE....................................................................................................... 4-34G. A REA D IRECTOR R ESPONSIBILITIES. .............................................................................. 4-35 VIII. DE MINIMIS CONDITIONS. ................................................................... 4-36A.C RITERIA ................................................................................................................................................... 4-36B.P ROFESSIONAL J UDGMENT. ..................................................................................................................4-37C. A REA D IRECTOR R ESPONSIBILITIES. .............................................................................. 4-37 IX. CITING IN THE ALTERNATIVE ............................................................ 4-37 X. COMBINING AND GROUPING VIOLATIONS. ................................... 4-37A.C OMBINING. ..............................................................................................................................................4-37B.G ROUPING. ................................................................................................................................................4-38C. W HEN N OT TO G ROUP OR C OMBINE. ................................................................................................4-38 XI. HEALTH STANDARD VIOLATIONS ....................................................... 4-39A.C ITATION OF V ENTILATION S TANDARDS ......................................................................................... 4-39B.V IOLATIONS OF THE N OISE S TANDARD. ...........................................................................................4-40 XII. VIOLATIONS OF THE RESPIRATORY PROTECTION STANDARD(§1910.134). ....................................................................................................... XIII. VIOLATIONS OF AIR CONTAMINANT STANDARDS (§1910.1000) ... 4-43 4-43A.R EQUIREMENTS UNDER THE STANDARD: .................................................................................................. 4-43B.C LASSIFICATION OF V IOLATIONS OF A IR C ONTAMINANT S TANDARDS. ......................................... 4-43 XIV. CITING IMPROPER PERSONAL HYGIENE PRACTICES. ................... 4-45A.I NGESTION H AZARDS. .................................................................................................................................... 4-45B.A BSORPTION H AZARDS. ................................................................................................................................ 4-46C.W IPE S AMPLING. ............................................................................................................................................. 4-46D.C ITATION P OLICY ............................................................................................................................................ 4-46 XV. BIOLOGICAL MONITORING. ...................................................................... 4-47CHAPTER 5CASE FILE PREPARATION AND DOCUMENTATIONI.INTRODUCTION ............................................................................................... 5-1 II.INSPECTION CONDUCTED, CITATIONS BEING ISSUED. .................... 5-1A.OSHA-1 ................................................................................................................................... 5-1B.OSHA-1A. ............................................................................................................................... 5-1C. OSHA-1B. ................................................................................................................................ 5-2 III.INSPECTION CONDUCTED BUT NO CITATIONS ISSUED .................... 5-5 IV.NO INSPECTION ............................................................................................... 5-5 V. HEALTH INSPECTIONS. ................................................................................. 5-6A.D OCUMENT P OTENTIAL E XPOSURE. ............................................................................................................... 5-6B.E MPLOYER’S O CCUPATIONAL S AFETY AND H EALTH S YSTEM. ............................................................. 5-6 VI. AFFIRMATIVE DEFENSES............................................................................. 5-8A.B URDEN OF P ROOF. .............................................................................................................................................. 5-8B.E XPLANATIONS. ..................................................................................................................................................... 5-8 VII. INTERVIEW STATEMENTS. ........................................................................ 5-10A.G ENERALLY. ......................................................................................................................................................... 5-10B.CSHO S SHALL OBTAIN WRITTEN STATEMENTS WHEN: .......................................................................... 5-10C.L ANGUAGE AND W ORDING OF S TATEMENT. ............................................................................................. 5-11D.R EFUSAL TO S IGN S TATEMENT ...................................................................................................................... 5-11E.V IDEO AND A UDIOTAPED S TATEMENTS. ..................................................................................................... 5-11F.A DMINISTRATIVE D EPOSITIONS. .............................................................................................5-11 VIII. PAPERWORK AND WRITTEN PROGRAM REQUIREMENTS. .......... 5-12 IX.GUIDELINES FOR CASE FILE DOCUMENTATION FOR USE WITH VIDEOTAPES AND AUDIOTAPES .............................................................. 5-12 X.CASE FILE ACTIVITY DIARY SHEET. ..................................................... 5-12 XI. CITATIONS. ..................................................................................................... 5-12A.S TATUTE OF L IMITATIONS. .............................................................................................................................. 5-13B.I SSUING C ITATIONS. ........................................................................................................................................... 5-13C.A MENDING/W ITHDRAWING C ITATIONS AND N OTIFICATION OF P ENALTIES. .................................. 5-13D.P ROCEDURES FOR A MENDING OR W ITHDRAWING C ITATIONS ............................................................ 5-14 XII. INSPECTION RECORDS. ............................................................................... 5-15A.G ENERALLY. ......................................................................................................................................................... 5-15B.R ELEASE OF I NSPECTION I NFORMATION ..................................................................................................... 5-15C. C LASSIFIED AND T RADE S ECRET I NFORMATION ...................................................................................... 5-16。
四大安全会议论文题目
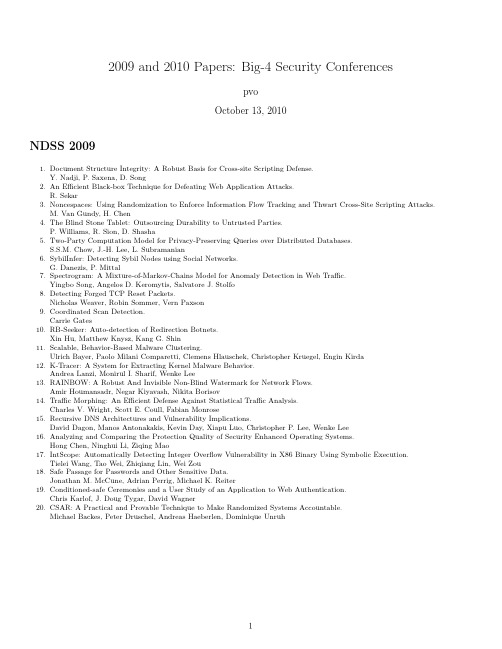
2009and2010Papers:Big-4Security ConferencespvoOctober13,2010NDSS20091.Document Structure Integrity:A Robust Basis for Cross-site Scripting Defense.Y.Nadji,P.Saxena,D.Song2.An Efficient Black-box Technique for Defeating Web Application Attacks.R.Sekar3.Noncespaces:Using Randomization to Enforce Information Flow Tracking and Thwart Cross-Site Scripting Attacks.M.Van Gundy,H.Chen4.The Blind Stone Tablet:Outsourcing Durability to Untrusted Parties.P.Williams,R.Sion,D.Shasha5.Two-Party Computation Model for Privacy-Preserving Queries over Distributed Databases.S.S.M.Chow,J.-H.Lee,L.Subramanian6.SybilInfer:Detecting Sybil Nodes using Social Networks.G.Danezis,P.Mittal7.Spectrogram:A Mixture-of-Markov-Chains Model for Anomaly Detection in Web Traffic.Yingbo Song,Angelos D.Keromytis,Salvatore J.Stolfo8.Detecting Forged TCP Reset Packets.Nicholas Weaver,Robin Sommer,Vern Paxson9.Coordinated Scan Detection.Carrie Gates10.RB-Seeker:Auto-detection of Redirection Botnets.Xin Hu,Matthew Knysz,Kang G.Shin11.Scalable,Behavior-Based Malware Clustering.Ulrich Bayer,Paolo Milani Comparetti,Clemens Hlauschek,Christopher Kruegel,Engin Kirda12.K-Tracer:A System for Extracting Kernel Malware Behavior.Andrea Lanzi,Monirul I.Sharif,Wenke Lee13.RAINBOW:A Robust And Invisible Non-Blind Watermark for Network Flows.Amir Houmansadr,Negar Kiyavash,Nikita Borisov14.Traffic Morphing:An Efficient Defense Against Statistical Traffic Analysis.Charles V.Wright,Scott E.Coull,Fabian Monrose15.Recursive DNS Architectures and Vulnerability Implications.David Dagon,Manos Antonakakis,Kevin Day,Xiapu Luo,Christopher P.Lee,Wenke Lee16.Analyzing and Comparing the Protection Quality of Security Enhanced Operating Systems.Hong Chen,Ninghui Li,Ziqing Mao17.IntScope:Automatically Detecting Integer Overflow Vulnerability in X86Binary Using Symbolic Execution.Tielei Wang,Tao Wei,Zhiqiang Lin,Wei Zou18.Safe Passage for Passwords and Other Sensitive Data.Jonathan M.McCune,Adrian Perrig,Michael K.Reiter19.Conditioned-safe Ceremonies and a User Study of an Application to Web Authentication.Chris Karlof,J.Doug Tygar,David Wagner20.CSAR:A Practical and Provable Technique to Make Randomized Systems Accountable.Michael Backes,Peter Druschel,Andreas Haeberlen,Dominique UnruhOakland20091.Wirelessly Pickpocketing a Mifare Classic Card.(Best Practical Paper Award)Flavio D.Garcia,Peter van Rossum,Roel Verdult,Ronny Wichers Schreur2.Plaintext Recovery Attacks Against SSH.Martin R.Albrecht,Kenneth G.Paterson,Gaven J.Watson3.Exploiting Unix File-System Races via Algorithmic Complexity Attacks.Xiang Cai,Yuwei Gui,Rob Johnson4.Practical Mitigations for Timing-Based Side-Channel Attacks on Modern x86Processors.Bart Coppens,Ingrid Verbauwhede,Bjorn De Sutter,Koen De Bosschere5.Non-Interference for a Practical DIFC-Based Operating System.Maxwell Krohn,Eran Tromer6.Native Client:A Sandbox for Portable,Untrusted x86Native Code.(Best Paper Award)B.Yee,D.Sehr,G.Dardyk,B.Chen,R.Muth,T.Ormandy,S.Okasaka,N.Narula,N.Fullagar7.Automatic Reverse Engineering of Malware Emulators.(Best Student Paper Award)Monirul Sharif,Andrea Lanzi,Jonathon Giffin,Wenke Lee8.Prospex:Protocol Specification Extraction.Paolo Milani Comparetti,Gilbert Wondracek,Christopher Kruegel,Engin Kirda9.Quantifying Information Leaks in Outbound Web Traffic.Kevin Borders,Atul Prakash10.Automatic Discovery and Quantification of Information Leaks.Michael Backes,Boris Kopf,Andrey Rybalchenko11.CLAMP:Practical Prevention of Large-Scale Data Leaks.Bryan Parno,Jonathan M.McCune,Dan Wendlandt,David G.Andersen,Adrian Perrig12.De-anonymizing Social Networks.Arvind Narayanan,Vitaly Shmatikov13.Privacy Weaknesses in Biometric Sketches.Koen Simoens,Pim Tuyls,Bart Preneel14.The Mastermind Attack on Genomic Data.Michael T.Goodrich15.A Logic of Secure Systems and its Application to Trusted Computing.Anupam Datta,Jason Franklin,Deepak Garg,Dilsun Kaynar16.Formally Certifying the Security of Digital Signature Schemes.Santiago Zanella-Beguelin,Gilles Barthe,Benjamin Gregoire,Federico Olmedo17.An Epistemic Approach to Coercion-Resistance for Electronic Voting Protocols.Ralf Kuesters,Tomasz Truderung18.Sphinx:A Compact and Provably Secure Mix Format.George Danezis,Ian Goldberg19.DSybil:Optimal Sybil-Resistance for Recommendation Systems.Haifeng Yu,Chenwei Shi,Michael Kaminsky,Phillip B.Gibbons,Feng Xiao20.Fingerprinting Blank Paper Using Commodity Scanners.William Clarkson,Tim Weyrich,Adam Finkelstein,Nadia Heninger,Alex Halderman,Ed Felten 21.Tempest in a Teapot:Compromising Reflections Revisited.Michael Backes,Tongbo Chen,Markus Duermuth,Hendrik P.A.Lensch,Martin Welk22.Blueprint:Robust Prevention of Cross-site Scripting Attacks for Existing Browsers.Mike Ter Louw,V.N.Venkatakrishnan23.Pretty-Bad-Proxy:An Overlooked Adversary in Browsers’HTTPS Deployments.Shuo Chen,Ziqing Mao,Yi-Min Wang,Ming Zhang24.Secure Content Sniffing for Web Browsers,or How to Stop Papers from Reviewing Themselves.Adam Barth,Juan Caballero,Dawn Song25.It’s No Secret:Measuring the Security and Reliability of Authentication via’Secret’Questions.Stuart Schechter,A.J.Bernheim Brush,Serge Egelman26.Password Cracking Using Probabilistic Context-Free Grammars.Matt Weir,Sudhir Aggarwal,Bill Glodek,Breno de MedeirosUSENIX Security2009promising Electromagnetic Emanations of Wired and Wireless Keyboards.(Outstanding Student Paper)Martin Vuagnoux,Sylvain Pasini2.Peeping Tom in the Neighborhood:Keystroke Eavesdropping on Multi-User Systems.Kehuan Zhang,XiaoFeng Wang3.A Practical Congestion Attack on Tor Using Long Paths,Nathan S.Evans,Roger Dingledine,Christian Grothoff4.Baggy Bounds Checking:An Efficient and Backwards-Compatible Defense against Out-of-Bounds Errors.Periklis Akritidis,Manuel Costa,Miguel Castro,Steven Hand5.Dynamic Test Generation to Find Integer Bugs in x86Binary Linux Programs.David Molnar,Xue Cong Li,David A.Wagner6.NOZZLE:A Defense Against Heap-spraying Code Injection Attacks.Paruj Ratanaworabhan,Benjamin Livshits,Benjamin Zorn7.Detecting Spammers with SNARE:Spatio-temporal Network-level Automatic Reputation Engine.Shuang Hao,Nadeem Ahmed Syed,Nick Feamster,Alexander G.Gray,Sven Krasser8.Improving Tor using a TCP-over-DTLS Tunnel.Joel Reardon,Ian Goldberg9.Locating Prefix Hijackers using LOCK.Tongqing Qiu,Lusheng Ji,Dan Pei,Jia Wang,Jun(Jim)Xu,Hitesh Ballani10.GATEKEEPER:Mostly Static Enforcement of Security and Reliability Policies for JavaScript Code.Salvatore Guarnieri,Benjamin Livshits11.Cross-Origin JavaScript Capability Leaks:Detection,Exploitation,and Defense.Adam Barth,Joel Weinberger,Dawn Song12.Memory Safety for Low-Level Software/Hardware Interactions.John Criswell,Nicolas Geoffray,Vikram Adve13.Physical-layer Identification of RFID Devices.Boris Danev,Thomas S.Heydt-Benjamin,Srdjan CapkunCP:Secure Remote Storage for Computational RFIDs.Mastooreh Salajegheh,Shane Clark,Benjamin Ransford,Kevin Fu,Ari Juels15.Jamming-resistant Broadcast Communication without Shared Keys.Christina Popper,Mario Strasser,Srdjan Capkun16.xBook:Redesigning Privacy Control in Social Networking Platforms.Kapil Singh,Sumeer Bhola,Wenke Lee17.Nemesis:Preventing Authentication and Access Control Vulnerabilities in Web Applications.Michael Dalton,Christos Kozyrakis,Nickolai Zeldovich18.Static Enforcement of Web Application Integrity Through Strong Typing.William Robertson,Giovanni Vigna19.Vanish:Increasing Data Privacy with Self-Destructing Data.(Outstanding Student Paper)Roxana Geambasu,Tadayoshi Kohno,Amit A.Levy,Henry M.Levy20.Efficient Data Structures for Tamper-Evident Logging.Scott A.Crosby,Dan S.Wallach21.VPriv:Protecting Privacy in Location-Based Vehicular Services.Raluca Ada Popa,Hari Balakrishnan,Andrew J.Blumberg22.Effective and Efficient Malware Detection at the End Host.Clemens Kolbitsch,Paolo Milani Comparetti,Christopher Kruegel,Engin Kirda,Xiaoyong Zhou,XiaoFeng Wang 23.Protecting Confidential Data on Personal Computers with Storage Capsules.Kevin Borders,Eric Vander Weele,Billy Lau,Atul Prakash24.Return-Oriented Rootkits:Bypassing Kernel Code Integrity Protection Mechanisms.Ralf Hund,Thorsten Holz,Felix C.Freiling25.Crying Wolf:An Empirical Study of SSL Warning Effectiveness.Joshua Sunshine,Serge Egelman,Hazim Almuhimedi,Neha Atri,Lorrie Faith Cranor26.The Multi-Principal OS Construction of the Gazelle Web Browser.Helen J.Wang,Chris Grier,Alex Moshchuk,Samuel T.King,Piali Choudhury,Herman VenterACM CCS20091.Attacking cryptographic schemes based on”perturbation polynomials”.Martin Albrecht,Craig Gentry,Shai Halevi,Jonathan Katz2.Filter-resistant code injection on ARM.Yves Younan,Pieter Philippaerts,Frank Piessens,Wouter Joosen,Sven Lachmund,Thomas Walter3.False data injection attacks against state estimation in electric power grids.Yao Liu,Michael K.Reiter,Peng Ning4.EPC RFID tag security weaknesses and defenses:passport cards,enhanced drivers licenses,and beyond.Karl Koscher,Ari Juels,Vjekoslav Brajkovic,Tadayoshi Kohno5.An efficient forward private RFID protocol.Come Berbain,Olivier Billet,Jonathan Etrog,Henri Gilbert6.RFID privacy:relation between two notions,minimal condition,and efficient construction.Changshe Ma,Yingjiu Li,Robert H.Deng,Tieyan Li7.CoSP:a general framework for computational soundness proofs.Michael Backes,Dennis Hofheinz,Dominique Unruh8.Reactive noninterference.Aaron Bohannon,Benjamin C.Pierce,Vilhelm Sjoberg,Stephanie Weirich,Steve Zdancewicputational soundness for key exchange protocols with symmetric encryption.Ralf Kusters,Max Tuengerthal10.A probabilistic approach to hybrid role mining.Mario Frank,Andreas P.Streich,David A.Basin,Joachim M.Buhmann11.Efficient pseudorandom functions from the decisional linear assumption and weaker variants.Allison B.Lewko,Brent Waters12.Improving privacy and security in multi-authority attribute-based encryption.Melissa Chase,Sherman S.M.Chow13.Oblivious transfer with access control.Jan Camenisch,Maria Dubovitskaya,Gregory Neven14.NISAN:network information service for anonymization networks.Andriy Panchenko,Stefan Richter,Arne Rache15.Certificateless onion routing.Dario Catalano,Dario Fiore,Rosario Gennaro16.ShadowWalker:peer-to-peer anonymous communication using redundant structured topologies.Prateek Mittal,Nikita Borisov17.Ripley:automatically securing web2.0applications through replicated execution.K.Vikram,Abhishek Prateek,V.Benjamin Livshits18.HAIL:a high-availability and integrity layer for cloud storage.Kevin D.Bowers,Ari Juels,Alina Oprea19.Hey,you,get offof my cloud:exploring information leakage in third-party compute clouds.Thomas Ristenpart,Eran Tromer,Hovav Shacham,Stefan Savage20.Dynamic provable data possession.C.Christopher Erway,Alptekin Kupcu,Charalampos Papamanthou,Roberto Tamassia21.On cellular botnets:measuring the impact of malicious devices on a cellular network core.Patrick Traynor,Michael Lin,Machigar Ongtang,Vikhyath Rao,Trent Jaeger,Patrick Drew McDaniel,Thomas Porta 22.On lightweight mobile phone application certification.William Enck,Machigar Ongtang,Patrick Drew McDaniel23.SMILE:encounter-based trust for mobile social services.Justin Manweiler,Ryan Scudellari,Landon P.Cox24.Battle of Botcraft:fighting bots in online games with human observational proofs.Steven Gianvecchio,Zhenyu Wu,Mengjun Xie,Haining Wang25.Fides:remote anomaly-based cheat detection using client emulation.Edward C.Kaiser,Wu-chang Feng,Travis Schluessler26.Behavior based software theft detection.Xinran Wang,Yoon-chan Jhi,Sencun Zhu,Peng Liu27.The fable of the bees:incentivizing robust revocation decision making in ad hoc networks.Steffen Reidt,Mudhakar Srivatsa,Shane Balfe28.Effective implementation of the cell broadband engineTM isolation loader.Masana Murase,Kanna Shimizu,Wilfred Plouffe,Masaharu Sakamoto29.On achieving good operating points on an ROC plane using stochastic anomaly score prediction.Muhammad Qasim Ali,Hassan Khan,Ali Sajjad,Syed Ali Khayam30.On non-cooperative location privacy:a game-theoretic analysis.Julien Freudiger,Mohammad Hossein Manshaei,Jean-Pierre Hubaux,David C.Parkes31.Privacy-preserving genomic computation through program specialization.Rui Wang,XiaoFeng Wang,Zhou Li,Haixu Tang,Michael K.Reiter,Zheng Dong32.Feeling-based location privacy protection for location-based services.Toby Xu,Ying Cai33.Multi-party off-the-record messaging.Ian Goldberg,Berkant Ustaoglu,Matthew Van Gundy,Hao Chen34.The bayesian traffic analysis of mix networks.Carmela Troncoso,George Danezis35.As-awareness in Tor path selection.Matthew Edman,Paul F.Syverson36.Membership-concealing overlay networks.Eugene Y.Vasserman,Rob Jansen,James Tyra,Nicholas Hopper,Yongdae Kim37.On the difficulty of software-based attestation of embedded devices.Claude Castelluccia,Aurelien Francillon,Daniele Perito,Claudio Soriente38.Proximity-based access control for implantable medical devices.Kasper Bonne Rasmussen,Claude Castelluccia,Thomas S.Heydt-Benjamin,Srdjan Capkun39.XCS:cross channel scripting and its impact on web applications.Hristo Bojinov,Elie Bursztein,Dan Boneh40.A security-preserving compiler for distributed programs:from information-flow policies to cryptographic mechanisms.Cedric Fournet,Gurvan Le Guernic,Tamara Rezk41.Finding bugs in exceptional situations of JNI programs.Siliang Li,Gang Tan42.Secure open source collaboration:an empirical study of Linus’law.Andrew Meneely,Laurie A.Williams43.On voting machine design for verification and testability.Cynthia Sturton,Susmit Jha,Sanjit A.Seshia,David Wagner44.Secure in-VM monitoring using hardware virtualization.Monirul I.Sharif,Wenke Lee,Weidong Cui,Andrea Lanzi45.A metadata calculus for secure information sharing.Mudhakar Srivatsa,Dakshi Agrawal,Steffen Reidt46.Multiple password interference in text passwords and click-based graphical passwords.Sonia Chiasson,Alain Forget,Elizabeth Stobert,Paul C.van Oorschot,Robert Biddle47.Can they hear me now?:a security analysis of law enforcement wiretaps.Micah Sherr,Gaurav Shah,Eric Cronin,Sandy Clark,Matt Blaze48.English shellcode.Joshua Mason,Sam Small,Fabian Monrose,Greg MacManus49.Learning your identity and disease from research papers:information leaks in genome wide association study.Rui Wang,Yong Fuga Li,XiaoFeng Wang,Haixu Tang,Xiao-yong Zhou50.Countering kernel rootkits with lightweight hook protection.Zhi Wang,Xuxian Jiang,Weidong Cui,Peng Ning51.Mapping kernel objects to enable systematic integrity checking.Martim Carbone,Weidong Cui,Long Lu,Wenke Lee,Marcus Peinado,Xuxian Jiang52.Robust signatures for kernel data structures.Brendan Dolan-Gavitt,Abhinav Srivastava,Patrick Traynor,Jonathon T.Giffin53.A new cell counter based attack against tor.Zhen Ling,Junzhou Luo,Wei Yu,Xinwen Fu,Dong Xuan,Weijia Jia54.Scalable onion routing with torsk.Jon McLachlan,Andrew Tran,Nicholas Hopper,Yongdae Kim55.Anonymous credentials on a standard java card.Patrik Bichsel,Jan Camenisch,Thomas Gros,Victor Shouprge-scale malware indexing using function-call graphs.Xin Hu,Tzi-cker Chiueh,Kang G.Shin57.Dispatcher:enabling active botnet infiltration using automatic protocol reverse-engineering.Juan Caballero,Pongsin Poosankam,Christian Kreibich,Dawn Xiaodong Song58.Your botnet is my botnet:analysis of a botnet takeover.Brett Stone-Gross,Marco Cova,Lorenzo Cavallaro,Bob Gilbert,MartinSzydlowski,Richard A.Kemmerer,Christopher Kruegel,Giovanni VignaNDSS20101.Server-side Verification of Client Behavior in Online Games.Darrell Bethea,Robert Cochran and Michael Reiter2.Defeating Vanish with Low-Cost Sybil Attacks Against Large DHTs.S.Wolchok,O.S.Hofmann,N.Heninger,E.W.Felten,J.A.Halderman,C.J.Rossbach,B.Waters,E.Witchel3.Stealth DoS Attacks on Secure Channels.Amir Herzberg and Haya Shulman4.Protecting Browsers from Extension Vulnerabilities.Adam Barth,Adrienne Porter Felt,Prateek Saxena,and Aaron Boodman5.Adnostic:Privacy Preserving Targeted Advertising.Vincent Toubiana,Arvind Narayanan,Dan Boneh,Helen Nissenbaum and Solon Barocas6.FLAX:Systematic Discovery of Client-side Validation Vulnerabilities in Rich Web Applications.Prateek Saxena,Steve Hanna,Pongsin Poosankam and Dawn Song7.Effective Anomaly Detection with Scarce Training Data.William Robertson,Federico Maggi,Christopher Kruegel and Giovanni Vignarge-Scale Automatic Classification of Phishing Pages.Colin Whittaker,Brian Ryner and Marria Nazif9.A Systematic Characterization of IM Threats using Honeypots.Iasonas Polakis,Thanasis Petsas,Evangelos P.Markatos and Spiros Antonatos10.On Network-level Clusters for Spam Detection.Zhiyun Qian,Zhuoqing Mao,Yinglian Xie and Fang Yu11.Improving Spam Blacklisting Through Dynamic Thresholding and Speculative Aggregation.Sushant Sinha,Michael Bailey and Farnam Jahanian12.Botnet Judo:Fighting Spam with Itself.A.Pitsillidis,K.Levchenko,C.Kreibich,C.Kanich,G.M.Voelker,V.Paxson,N.Weaver,S.Savage13.Contractual Anonymity.Edward J.Schwartz,David Brumley and Jonathan M.McCune14.A3:An Extensible Platform for Application-Aware Anonymity.Micah Sherr,Andrew Mao,William R.Marczak,Wenchao Zhou,Boon Thau Loo,and Matt Blaze15.When Good Randomness Goes Bad:Virtual Machine Reset Vulnerabilities and Hedging Deployed Cryptography.Thomas Ristenpart and Scott Yilek16.InvisiType:Object-Oriented Security Policies.Jiwon Seo and Monica m17.A Security Evaluation of DNSSEC with NSEC3.Jason Bau and John Mitchell18.On the Safety of Enterprise Policy Deployment.Yudong Gao,Ni Pan,Xu Chen and Z.Morley Mao19.Where Do You Want to Go Today?Escalating Privileges by Pathname Manipulation.Suresh Chari,Shai Halevi and Wietse Venema20.Joe-E:A Security-Oriented Subset of Java.Adrian Mettler,David Wagner and Tyler Close21.Preventing Capability Leaks in Secure JavaScript Subsets.Matthew Finifter,Joel Weinberger and Adam Barth22.Binary Code Extraction and Interface Identification for Security Applications.Juan Caballero,Noah M.Johnson,Stephen McCamant,and Dawn Song23.Automatic Reverse Engineering of Data Structures from Binary Execution.Zhiqiang Lin,Xiangyu Zhang and Dongyan Xu24.Efficient Detection of Split Personalities in Malware.Davide Balzarotti,Marco Cova,Christoph Karlberger,Engin Kirda,Christopher Kruegel and Giovanni VignaOakland20101.Inspector Gadget:Automated Extraction of Proprietary Gadgets from Malware Binaries.Clemens Kolbitsch Thorsten Holz,Christopher Kruegel,Engin Kirda2.Synthesizing Near-Optimal Malware Specifications from Suspicious Behaviors.Matt Fredrikson,Mihai Christodorescu,Somesh Jha,Reiner Sailer,Xifeng Yan3.Identifying Dormant Functionality in Malware Programs.Paolo Milani Comparetti,Guido Salvaneschi,Clemens Kolbitsch,Engin Kirda,Christopher Kruegel,Stefano Zanero4.Reconciling Belief and Vulnerability in Information Flow.Sardaouna Hamadou,Vladimiro Sassone,Palamidessi5.Towards Static Flow-Based Declassification for Legacy and Untrusted Programs.Bruno P.S.Rocha,Sruthi Bandhakavi,Jerry I.den Hartog,William H.Winsborough,Sandro Etalle6.Non-Interference Through Secure Multi-Execution.Dominique Devriese,Frank Piessens7.Object Capabilities and Isolation of Untrusted Web Applications.Sergio Maffeis,John C.Mitchell,Ankur Taly8.TrustVisor:Efficient TCB Reduction and Attestation.Jonathan McCune,Yanlin Li,Ning Qu,Zongwei Zhou,Anupam Datta,Virgil Gligor,Adrian Perrig9.Overcoming an Untrusted Computing Base:Detecting and Removing Malicious Hardware Automatically.Matthew Hicks,Murph Finnicum,Samuel T.King,Milo M.K.Martin,Jonathan M.Smith10.Tamper Evident Microprocessors.Adam Waksman,Simha Sethumadhavan11.Side-Channel Leaks in Web Applications:a Reality Today,a Challenge Tomorrow.Shuo Chen,Rui Wang,XiaoFeng Wang Kehuan Zhang12.Investigation of Triangular Spamming:a Stealthy and Efficient Spamming Technique.Zhiyun Qian,Z.Morley Mao,Yinglian Xie,Fang Yu13.A Practical Attack to De-Anonymize Social Network Users.Gilbert Wondracek,Thorsten Holz,Engin Kirda,Christopher Kruegel14.SCiFI-A System for Secure Face Identification.(Best Paper)Margarita Osadchy,Benny Pinkas,Ayman Jarrous,Boaz Moskovich15.Round-Efficient Broadcast Authentication Protocols for Fixed Topology Classes.Haowen Chan,Adrian Perrig16.Revocation Systems with Very Small Private Keys.Allison Lewko,Amit Sahai,Brent Waters17.Authenticating Primary Users’Signals in Cognitive Radio Networks via Integrated Cryptographic and Wireless Link Signatures.Yao Liu,Peng Ning,Huaiyu Dai18.Outside the Closed World:On Using Machine Learning For Network Intrusion Detection.Robin Sommer,Vern Paxson19.All You Ever Wanted to Know about Dynamic Taint Analysis and Forward Symbolic Execution(but might have been afraid to ask).Thanassis Avgerinos,Edward Schwartz,David Brumley20.State of the Art:Automated Black-Box Web Application Vulnerability Testing.Jason Bau,Elie Bursztein,Divij Gupta,John Mitchell21.A Proof-Carrying File System.Deepak Garg,Frank Pfenning22.Scalable Parametric Verification of Secure Systems:How to Verify Ref.Monitors without Worrying about Data Structure Size.Jason Franklin,Sagar Chaki,Anupam Datta,Arvind Seshadri23.HyperSafe:A Lightweight Approach to Provide Lifetime Hypervisor Control-Flow Integrity.Zhi Wang,Xuxian Jiang24.How Good are Humans at Solving CAPTCHAs?A Large Scale Evaluation.Elie Bursztein,Steven Bethard,John C.Mitchell,Dan Jurafsky,Celine Fabry25.Bootstrapping Trust in Commodity Computers.Bryan Parno,Jonathan M.McCune,Adrian Perrig26.Chip and PIN is Broken.(Best Practical Paper)Steven J.Murdoch,Saar Drimer,Ross Anderson,Mike Bond27.Experimental Security Analysis of a Modern Automobile.K.Koscher,A.Czeskis,F.Roesner,S.Patel,T.Kohno,S.Checkoway,D.McCoy,B.Kantor,D.Anderson,H.Shacham,S.Savage 28.On the Incoherencies in Web Browser Access Control Policies.Kapil Singh,Alexander Moshchuk,Helen J.Wang,Wenke Lee29.ConScript:Specifying and Enforcing Fine-Grained Security Policies for JavaScript in the Browser.Leo Meyerovich,Benjamin Livshits30.TaintScope:A Checksum-Aware Directed Fuzzing Tool for Automatic Software Vulnerability Detection.(Best Student Paper)Tielei Wang,Tao Wei,Guofei Gu,Wei Zou31.A Symbolic Execution Framework for JavaScript.Prateek Saxena,Devdatta Akhawe,Steve Hanna,Stephen McCamant,Dawn Song,Feng MaoUSENIX Security20101.Adapting Software Fault Isolation to Contemporary CPU Architectures.David Sehr,Robert Muth,CliffBiffle,Victor Khimenko,Egor Pasko,Karl Schimpf,Bennet Yee,Brad Chen2.Making Linux Protection Mechanisms Egalitarian with UserFS.Taesoo Kim and Nickolai Zeldovich3.Capsicum:Practical Capabilities for UNIX.(Best Student Paper)Robert N.M.Watson,Jonathan Anderson,Ben Laurie,Kris Kennaway4.Structuring Protocol Implementations to Protect Sensitive Data.Petr Marchenko,Brad Karp5.PrETP:Privacy-Preserving Electronic Toll Pricing.Josep Balasch,Alfredo Rial,Carmela Troncoso,Bart Preneel,Ingrid Verbauwhede,Christophe Geuens6.An Analysis of Private Browsing Modes in Modern Browsers.Gaurav Aggarwal,Elie Bursztein,Collin Jackson,Dan Boneh7.BotGrep:Finding P2P Bots with Structured Graph Analysis.Shishir Nagaraja,Prateek Mittal,Chi-Yao Hong,Matthew Caesar,Nikita Borisov8.Fast Regular Expression Matching Using Small TCAMs for Network Intrusion Detection and Prevention Systems.Chad R.Meiners,Jignesh Patel,Eric Norige,Eric Torng,Alex X.Liu9.Searching the Searchers with SearchAudit.John P.John,Fang Yu,Yinglian Xie,Martin Abadi,Arvind Krishnamurthy10.Toward Automated Detection of Logic Vulnerabilities in Web Applications.Viktoria Felmetsger,Ludovico Cavedon,Christopher Kruegel,Giovanni Vigna11.Baaz:A System for Detecting Access Control Misconfigurations.Tathagata Das,Ranjita Bhagwan,Prasad Naldurg12.Cling:A Memory Allocator to Mitigate Dangling Pointers.Periklis Akritidis13.ZKPDL:A Language-Based System for Efficient Zero-Knowledge Proofs and Electronic Cash.Sarah Meiklejohn,C.Chris Erway,Alptekin Kupcu,Theodora Hinkle,Anna Lysyanskaya14.P4P:Practical Large-Scale Privacy-Preserving Distributed Computation Robust against Malicious Users.Yitao Duan,John Canny,Justin Zhan,15.SEPIA:Privacy-Preserving Aggregation of Multi-Domain Network Events and Statistics.Martin Burkhart,Mario Strasser,Dilip Many,Xenofontas Dimitropoulos16.Dude,Where’s That IP?Circumventing Measurement-based IP Geolocation.Phillipa Gill,Yashar Ganjali,Bernard Wong,David Lie17.Idle Port Scanning and Non-interference Analysis of Network Protocol Stacks Using Model Checking.Roya Ensafi,Jong Chun Park,Deepak Kapur,Jedidiah R.Crandall18.Building a Dynamic Reputation System for DNS.Manos Antonakakis,Roberto Perdisci,David Dagon,Wenke Lee,Nick Feamster19.Scantegrity II Municipal Election at Takoma Park:The First E2E Binding Governmental Election with Ballot Privacy.R.Carback,D.Chaum,J.Clark,J.Conway,A.Essex,P.S.Herrnson,T.Mayberry,S.Popoveniuc,R.L.Rivest,E.Shen,A.T.Sherman,P.L.Vora20.Acoustic Side-Channel Attacks on Printers.Michael Backes,Markus Durmuth,Sebastian Gerling,Manfred Pinkal,Caroline Sporleder21.Security and Privacy Vulnerabilities of In-Car Wireless Networks:A Tire Pressure Monitoring System Case Study.Ishtiaq Rouf,Rob Miller,Hossen Mustafa,Travis Taylor,Sangho Oh,Wenyuan Xu,Marco Gruteser,Wade Trappe,Ivan Seskar 22.VEX:Vetting Browser Extensions for Security Vulnerabilities.(Best Paper)Sruthi Bandhakavi,Samuel T.King,P.Madhusudan,Marianne Winslett23.Securing Script-Based Extensibility in Web Browsers.Vladan Djeric,Ashvin Goel24.AdJail:Practical Enforcement of Confidentiality and Integrity Policies on Web Advertisements.Mike Ter Louw,Karthik Thotta Ganesh,V.N.Venkatakrishnan25.Realization of RF Distance Bounding.Kasper Bonne Rasmussen,Srdjan Capkun26.The Case for Ubiquitous Transport-Level Encryption.Andrea Bittau,Michael Hamburg,Mark Handley,David Mazieres,Dan Boneh27.Automatic Generation of Remediation Procedures for Malware Infections.Roberto Paleari,Lorenzo Martignoni,Emanuele Passerini,Drew Davidson,Matt Fredrikson,Jon Giffin,Somesh Jha28.Re:CAPTCHAs-Understanding CAPTCHA-Solving Services in an Economic Context.Marti Motoyama,Kirill Levchenko,Chris Kanich,Damon McCoy,Geoffrey M.Voelker,Stefan Savage29.Chipping Away at Censorship Firewalls with User-Generated Content.Sam Burnett,Nick Feamster,Santosh Vempala30.Fighting Coercion Attacks in Key Generation using Skin Conductance.Payas Gupta,Debin GaoACM CCS20101.Security Analysis of India’s Electronic Voting Machines.Scott Wolchok,Erik Wustrow,J.Alex Halderman,Hari Prasad,Rop Gonggrijp2.Dissecting One Click Frauds.Nicolas Christin,Sally S.Yanagihara,Keisuke Kamataki3.@spam:The Underground on140Characters or Less.Chris Grier,Kurt Thomas,Vern Paxson,Michael Zhang4.HyperSentry:Enabling Stealthy In-context Measurement of Hypervisor Integrity.Ahmed M.Azab,Peng Ning,Zhi Wang,Xuxian Jiang,Xiaolan Zhang,Nathan C.Skalsky5.Trail of Bytes:Efficient Support for Forensic Analysis.Srinivas Krishnan,Kevin Z.Snow,Fabian Monrose6.Survivable Key Compromise in Software Update Systems.Justin Samuel,Nick Mathewson,Justin Cappos,Roger Dingledine7.A Methodology for Empirical Analysis of the Permission-Based Security Models and its Application to Android.David Barrera,H.Gunes Kayacik,Paul C.van Oorschot,Anil Somayaji8.Mobile Location Tracking in Metropolitan Areas:malnets and others.Nathanial Husted,Steve Myers9.On Pairing Constrained Wireless Devices Based on Secrecy of Auxiliary Channels:The Case of Acoustic Eavesdropping.Tzipora Halevi,Nitesh Saxena10.PinDr0p:Using Single-Ended Audio Features to Determine Call Provenance.Vijay A.Balasubramaniyan,Aamir Poonawalla,Mustaque Ahamad,Michael T.Hunter,Patrick Traynor11.Building Efficient Fully Collusion-Resilient Traitor Tracing and Revocation Schemes.Sanjam Garg,Abishek Kumarasubramanian,Amit Sahai,Brent Waters12.Algebraic Pseudorandom Functions with Improved Efficiency from the Augmented Cascade.Dan Boneh,Hart Montgomery,Ananth Raghunathan13.Practical Leakage-Resilient Pseudorandom Generators.Yu Yu,Francois-Xavier Standaert,Olivier Pereira,Moti Yung14.Practical Leakage-Resilient Identity-Based Encryption from Simple Assumptions.Sherman S.M.Chow,Yevgeniy Dodis,Yannis Rouselakis,Brent Waters15.Testing Metrics for Password Creation Policies by Attacking Large Sets of Revealed Passwords.Matt Weir,Sudhir Aggarwal,Michael Collins,Henry Stern16.The Security of Modern Password Expiration:An Algorithmic Framework and Empirical Analysis.Yinqian Zhang,Fabian Monrose,Michael K.Reiter17.Attacks and Design of Image Recognition CAPTCHAs.Bin Zhu,JeffYan,Chao Yang,Qiujie Li,Jiu Liu,Ning Xu,Meng Yi18.Robusta:Taming the Native Beast of the JVM.Joseph Siefers,Gang Tan,Greg Morrisett19.Retaining Sandbox Containment Despite Bugs in Privileged Memory-Safe Code.Justin Cappos,Armon Dadgar,JeffRasley,Justin Samuel,Ivan Beschastnikh,Cosmin Barsan,Arvind Krishnamurthy,Thomas Anderson20.A Control Point for Reducing Root Abuse of File-System Privileges.Glenn Wurster,Paul C.van Oorschot21.Modeling Attacks on Physical Unclonable Functions.Ulrich Ruehrmair,Frank Sehnke,Jan Soelter,Gideon Dror,Srinivas Devadas,Juergen Schmidhuber22.Dismantling SecureMemory,CryptoMemory and CryptoRF.Flavio D.Garcia,Peter van Rossum,Roel Verdult,Ronny Wichers Schreur23.Attacking and Fixing PKCS#11Security Tokens.Matteo Bortolozzo,Matteo Centenaro,Riccardo Focardi,Graham Steel24.An Empirical Study of Privacy-Violating Information Flows in JavaScript Web Applications.Dongseok Jang,Ranjit Jhala,Sorin Lerner,Hovav Shacham25.DIFC Programs by Automatic Instrumentation.William Harris,Somesh Jha,Thomas Reps26.Predictive Black-box Mitigation of Timing Channels.Aslan Askarov,Danfeng Zhang,Andrew Myers27.In Search of an Anonymous and Secure Lookup:Attacks on Structured Peer-to-peer Anonymous Communication Systems.Qiyan Wang,Prateek Mittal,Nikita Borisov28.Recruiting New Tor Relays with BRAIDS.Rob Jansen,Nicholas Hopper,Yongdae Kim29.An Improved Algorithm for Tor Circuit Scheduling.Can Tang,Ian Goldberg30.Dissent:Accountable Anonymous Group Messaging.Henry Corrigan-Gibbs,Bryan Ford31.Abstraction by Set-Membership—Verifying Security Protocols and Web Services with Databases.Sebastian Moedersheim。
Scalability Solutions in Operating Systems

Scalability Solutions in OperatingSystemsScalability in operating systems refers to the ability of an operating system to handle an increasing workload or its ability to accommodate growth in terms of users, requests, or transactions without compromising performance or reliability. As technology continues to advance and more and more data is being generated, scalability has become a crucial aspect of operating systems. In this article, we will explore some of the scalability solutions that are commonly implemented in operating systems.One of the key scalability solutions in operating systems is parallel processing. Parallel processing involves breaking down a task into smaller sub-tasks that can be processed simultaneously by multiple processors. This allows for faster execution of tasks and better utilization of system resources. Operating systems that support parallel processing can handle a higher workload and provide better performance as compared to systems that rely on a single processor.Another scalability solution is load balancing. Load balancing involves distributing incoming requests evenly across multiple servers or nodes in a network. This ensures that no single server is overloaded with requests, thereby improving the overall performance and reliability of the system. Operating systems that implement load balancing algorithms can efficiently manage resources and handle a larger number of users or requests without any degradation in performance.Virtualization is another important scalability solution in operating systems. Virtualization allows multiple virtual machines to run on a single physical machine, enabling efficient utilization of resources and easier management of hardware. By virtualizing resources, operating systems can dynamically allocate resources based on workload demands and scale up or down as needed. Virtualization also enhances system reliability and fault tolerance by isolating applications from the underlying hardware.Containerization is also a popular scalability solution in modern operating systems. Containers encapsulate applications and their dependencies in a lightweight and portable package, allowing them to run consistently across different environments. Containers are highly scalable and can be easily deployed and managed, making them ideal for modern cloud-based applications. Operating systems that support containerization enable developers to quickly scale their applications and services without the need for complex configurations.Furthermore, microservices architecture is another scalability solution that is gaining popularity in operating systems. Microservices break down applications into smaller, independently deployable services that can be scaled horizontally to meet growing demand. This modular approach allows for better resource utilization, fault isolation, and improved system performance. Operating systems that support microservices architecture enable developers to build scalable and resilient applications that can adapt to changing workloads.In conclusion, scalability solutions play a crucial role in ensuring the performance, reliability, and efficiency of operating systems in today's rapidly evolving technological landscape. By implementing parallel processing, load balancing, virtualization, containerization, and microservices architecture, operating systems can effectively handle increasing workloads, accommodate growth, and provide seamless user experiences. As the demand for scalable and high-performing systems continues to grow, it is essential for operating systems to embrace these scalability solutions to stay competitive and meet the needs of modern computing environments.。
boot项目的概念
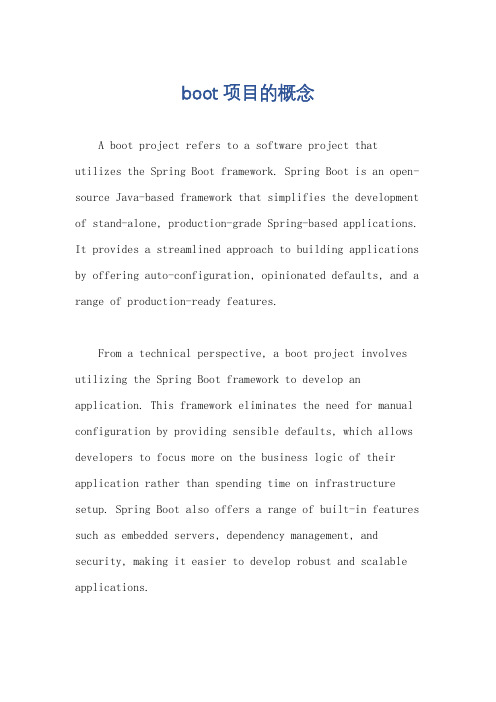
boot项目的概念A boot project refers to a software project thatutilizes the Spring Boot framework. Spring Boot is an open-source Java-based framework that simplifies the development of stand-alone, production-grade Spring-based applications. It provides a streamlined approach to building applications by offering auto-configuration, opinionated defaults, and a range of production-ready features.From a technical perspective, a boot project involves utilizing the Spring Boot framework to develop an application. This framework eliminates the need for manual configuration by providing sensible defaults, which allows developers to focus more on the business logic of their application rather than spending time on infrastructure setup. Spring Boot also offers a range of built-in features such as embedded servers, dependency management, and security, making it easier to develop robust and scalable applications.From a developer's point of view, working on a boot project can be a pleasant experience. The framework's simplicity and convention-over-configuration approach reduce the boilerplate code typically required intraditional Spring applications. This means less time spent on configuration and more time spent on actual development. Additionally, Spring Boot's extensive documentation and active community make it easy to find solutions to common problems and get support when needed.From a business perspective, boot projects can have several advantages. The streamlined development process offered by Spring Boot can lead to faster time-to-marketfor applications, allowing businesses to respond quickly to market demands. The framework's focus on production-ready features ensures that applications are built with scalability, robustness, and security in mind, reducing the risk of issues in production environments. Furthermore, Spring Boot's popularity and wide adoption in the industry mean that there is a large pool of skilled developers available, making it easier to build and maintain boot projects.Boot projects also have implications for the deployment and maintenance of applications. The framework's embedded server capability allows applications to be packaged as executable JAR files, making deployment and distribution straightforward. Spring Boot's Actuator module provides monitoring and management endpoints, enabling administrators to monitor the health and performance of applications. This simplifies the maintenance and troubleshooting process, as it provides valuable insights into the application's behavior.In conclusion, a boot project refers to a software project that utilizes the Spring Boot framework for developing stand-alone, production-grade applications. From a technical perspective, it involves leveraging the framework's features and auto-configuration capabilities to simplify development. For developers, boot projects offer a streamlined and efficient development experience, while businesses benefit from faster time-to-market, scalability, and a large pool of skilled developers. Additionally, boot projects simplify deployment and maintenance through theuse of embedded servers and monitoring endpoints. Overall, boot projects are a popular choice for building robust and scalable applications in the Java ecosystem.。
- 1、下载文档前请自行甄别文档内容的完整性,平台不提供额外的编辑、内容补充、找答案等附加服务。
- 2、"仅部分预览"的文档,不可在线预览部分如存在完整性等问题,可反馈申请退款(可完整预览的文档不适用该条件!)。
- 3、如文档侵犯您的权益,请联系客服反馈,我们会尽快为您处理(人工客服工作时间:9:00-18:30)。
A Scalable Framework for Information VisualizationMatthias Kreuseler,Norma López,Heidrun Schumann{mkreusel,lopez,schumann}@informatik.uni-rostock.de University of Rostock,Dept.of Computer Science,18051Rostock,GermanyAbstractThis paper describes major concepts of a scalable information visualization framework.We assume that the exploration of hete-rogenous information spaces at arbitrary levels of detail requires a suitable preprocessing of information quantities,the combination of different graphical interfaces and the illustration of the frame of reference of given information sets.The innovative features of our system include dynamic hierarchy computation and user con-trolled refinement of those hierarchies for preprocessing unstruc-tured information spaces,a new Focus+Context technique for vi-sualizing complex hierarchy graphs,a new paradigm for visuali-zing information structures within their frame of reference and a new graphical interface that utilizes textual similarities to arrange objects of high dimensional information space in3-dimensional visualization space.1.IntroductionVisual exploration of complex information spaces has become one of the”hot topics”in computer graphics research.A vari-ety of novel visualization paradigms and frameworks have been developed in recent years.Nevertheless achievingflexible visuali-zations i.e.,preprocessing large quantities of unstructured hetero-geneous information,displaying information context(e.g.frame of spatial or domain references)and supporting a variety of explo-ration tasks carry over entirely new qualities of problems.Some of the most important ones can be summarized as follows: Reducing information and Obtaining structure:The explo-ration of large unstructured information spaces requires in-formation preprocessing in order to reduce the active data size to processible levels.In this regard"filtering out unin-teresting items"and merging similar objects into groups are necessary.Suitable metrics have to be applied for obtaining similarities and structures in high-dimensional feature space.Furthermore the degree of abstraction has to be controlled in-teractively in order to browse information space at arbitrary levels of detail.Visualizing information sets:The success of visualization depends very much on its ability of supporting a variety of exploration tasks(e.g.overview,zoom in on items of inte-rest or details on demand).Different visualization methodsare required for revealing information structure and informa-tion contents(e.g.attribute values).Furthermore novel in-teraction techniques are needed for controlling the degree of abstraction within visual representations and for providing navigational aids in information space.Visualizing the frame of reference:Effective explorations of spatially referenced information(e.g.health data in certain areas)require the combination of an adequate display of the spatial frame of reference with the visualization of complex information structures.It is necessary tofind an appropriate mapping between information and frame of reference.This problem hasn’t been widely studied yet in the case of dis-playing complex graphs such as large hierarchies over geo-graphical maps.A variety of visualization methods have been developed in dif-ferent domains.Among these are techniques for visualizing and interacting with hierarchies like Cone Trees[6]or Disc Trees [13]which use horizontal and vertical cones or discs to layout hierarchies.FSN[22]and Information Pyramids[1]exploit the metaphor of3D information landscapes to depict large hierarchi-cal information spaces.Other approaches such as Treemaps[14] and CHEOPS[5]are well known2D techniques which use avail-able screen space very effectively.Several techniques have been developed for visualizing multi-dimensional information.These methods try to map correlations of objects in high dimensional information space to spatial corre-lations in a2D or3D presentation space.Among these are ap-proaches like IVORY[8],VR-VIBE[4]and Narcissus[10]which exploit spring models to place objects according to their similari-ties,whereby similar objects are placed spatially close together. Other systems like Lyberworld[9]and SPIRE[24]use different vi-sual metaphors like Relevance Spaces[9],Information Galaxies or Themescapes[24]in order to visualize document collections or re-sults from data base retrieval.FOCUS[21]is an interactive table viewer which supports the exploration of complex object-attribute tables by a combination of a focus+context technique,a hierar-chical outliner for large attribute sets and a general easy-to-use dynamic query mechanism.Systems like Descartes[2]or Devise[7]provide solutions for visualizing geographically related information.Different types of icons,diagrams,colored faces and maps are used for depicting data within their spatial frame of reference.In contrast to that these systems do not support the visualization of rather complex information structures as for instance abstract node link graphs or hierarchies.Most of the systems mentioned above solve,each in its own manner,some of the single problems introduced earlier in this section.But,up to now,there are still open questions of how to provide aflexible framework for solving those problems in a more general way.We suggest a scalable visualization framework(cf.section2) in order to address the introduced problems.Basically our frame-work integrates a scalable preprocessing pipeline for organizing large unstructured high-dimensional information spaces(see sec-tion3)with several new scalable visualization techniques(cf.sec-tion4)for visualizing information structure along with informa-tion contents.We propose a new paradigm for integrating the visualization of information structures and their spatial frame of reference in section5.Future work and conclusions are covered in section6.2.Basic concept of a scalable frameworkThe design of a scalable visualization framework requires a formal and easily adaptable information model for describing in-formation units and the general characteristics of the information space.Therefore we introduce Information objects IO i as the basic elements of our information model.The term information object denotes a necessary abstraction of the data for specifying infor-mation units.Each information object is characterized by a set of attributes which can have arbitrary continuous ranges of values in order to describe object rmation objects IO i can be text documents,files or real world objects like cars,houses or cities.A more formal transcription of our information model is given by Wünsche[25].In order to solve the problems addressed in section1we propose a framework which integrates a scalable preprocessing pipeline and different visualization modules.Our preprocess-ing pipeline implements several algorithms(e.g.clustering,dy-namic hierarchy computation and neural networks)for analyzing unstructured information bining different techniques within aflexible framework helps to scale preprocessing with re-spect to the characteristics of the information space and users ex-ploration tasks.In order to display preprocessing results and to explore information space graphically the framework offers seve-ral new visualization techniques as well.Scalable PreprocessingPreprocessing information in order to gain structure,e.g.iden-tifying groups of related information objects or forming meaning-ful subsets of the given data is a non-trivial task because there is no general mathematical framework or paradigm on how to build those groups or subsets.Basically our approach exploits similarities between information objects in high dimensional fea-ture space.Therefore we have to provide adequate measures S i j S IO i IO j for calculating similarities between information objects IO i and IO j.As stated in[3]computing similarity measures is rather com-plicated because similarity can be defined in various ways,and of-ten domain specific expertise is required for determining appropri-ate measures.Furthermore the decision if two objects are similar or not is specific to user goals.Lets consider an example.A num-ber offirms are described by the volume of sales over a period of several years.As it is the objective to group thosefirms with simi-lar sales rates within this time period Euclidean Distance or some Minkowski Distances[15]are sufficient measures.In contrast to that the Dot product or a Correlation coefficient[15]are appropri-ate if it is the intention groupingfirms with similar sales growth within that period of time.Thus either of the different measures might be appropriate in certain cases.Furthermore the applicability of a specific similarity measure depends on the basic data types of the information object’s at-tribute values.Thus similarities might have to be computed from variables that are binary,nominal,ratio scaled or a combination of these(cf.[15]for further information about these data types).Summarizing the discussion above we conclude that provid-ing a single similarity measure is not sufficient for aflexible pre-processing of complex information spaces.Therefore our pre-processing pipeline offers a variety of different metrics and simi-larity measures:Euclidean distance,L p-metric,Mahalonobis distance,Dot product,Normalized dot product,Correlation co-efficient,General M-coefficient and M-coefficient.Moreover the pipeline can easily be extended by additional measures.In addi-tion toflexibility regarding similarity measures our pipeline sup-ports different algorithms for preprocessing information.Depend-ing on exploration tasks the user can choose one of the following techniques:Self Organizing Maps[16]which are suitable for determin-ing an overview of the entire collection and revealing the overall similarity structure between information objects in information spaceDynamic hierarchy computation which can be controlled in-teractively in order to achieve sophisticated organizations of complex data sets and to reveal patterns and relationships among the dataScalable VisualizationThe effective presentation of different aspects of a given infor-mation set(e.g.visualization of information structure or display of concrete attribute values)requires the combination of differ-ent visualization methods respectively scalable techniques which can be adopted to specific exploration goals.Our scalable vi-sualization framework provides several visualization techniques. Beneath Highfields(cf.Figure1),KOAN[18]and Parallel Co-ordinates[12]we introduce the new techniques Magic-Eye-View for displaying complex graphs and ShapeVis for depicting multi-dimensional information sets.Furthermore we propose a new ap-proach which we named Marching Sphere for visualizing complex information structures with spatial dependencies.3.Structuring and preprocessing informationExploring information collections becomes increasingly diffi-cult as the volume of information grows.Major problems arise due to visual clutter and the limited screen space as the number of objects exceeds some limits.Hence it is indispensable to apply suitable preprocessing for gaining structures,extracting relevant subsets of the information and for reducing the active data size to processible levels.Figure1.Example of information organization based onself-organizing maps3.1.Self-organizing mapsSelf-organizing maps(SOM)as introduced by Kohonen[16] provide an effective mechanism for preprocessing and organizing unstructured data.SOMs are able to extract groups of similar in-formation objects and can be described as nonlinear projection from n-dimensional input space onto two-dimensional visualiza-tion space.A self organizing map consists of a two-dimensional network of neurons typically arranged on a regular lattice.Each cell is associated with a single randomly initialized n-dimensional reference vector.In the basic SOM algorithm the map is trained with a set of input vectors several times.For each input vector the map is searched for the most similar reference vector,called the winning vector.The winning vector is updated such that it more closely represents the input vector.Along with that the reference vectors in the neighborhood around the winning vector are also adjusted in response to the actual input vector.After the training phase reference vectors in adjacent cells represent input vectors which are close(i.e.similar)in information space.Thus SOMs provide a useful topological arrangement of information objects in order to display clusters of similar objects in information space.Figure1illustrates the use of SOMs for structuring unorga-nized information spaces in our framework.The picture was gen-erated from a car data set with6dimensions.Each peak in the map displays a cluster of similar objects.The number of objects within a single cluster is mapped onto the height of the peak.Color is used for displaying similarities between adjacent clusters where bright intensities denote a higher degree of dissimilarity.Thus SOMs are suitable for providing an overview of the entire infor-mation space.3.2.Dynamic hierarchy computationThe dynamic hierarchy computation is another possible method to achieve predictable presentations of unstructured infor-mation spaces,even if the given data set is not a"natural"hierar-chy.If an abstraction is used to organize data,it is important to remember that users may have different requirements when merg-ing objects into groups.Thus we do not compute afixed number of static groups.Instead,a nested sequence of groups is determined and organized into a hierarchy,whereby the requirements accord-ing to the similarity of the objects within those groups increase as the hierarchy is descended.Dynamic hierarchy computation is carried out by adapted ag-glomerative clustering algorithms[15].Based on one of the al-gorithms Single Linkage,Complete Linkage,Average Linkage, Ward,Median,Flexible Strategy and Zentroid[15],informationobjects IO are merged into groups according to their similarities in information space.Therefore a symmetric n x n similarity matrix S is computed(with n number of information objects IO ininformation space)based on a single or on a combination of the similarity measures enumerated in section2.SS11S n1S n1S n nwhere S i j S j i i j1n andS i i1i1nThe similarity matrix serves as a basis for a bottom up creation of a binary dendrogram(cf.Figure2left).Figure2.Construction of thefinal Hierarchy tree with3levels based on the binary dendrogramIn thefirst step we start merging the two most similar infor-mation objects IO i IO j,i.e.where S i j max into thefirst group. Subsequently a new n1x n1similarity matrix is calculated and the next two closest objects(groups)are merged.This pro-cess continues until all information objects IO i are processed and the binary dendrogram is determined completely.A heterogene-ity value,which denotes the average dissimilarity within a single group of objects,is calculated for each node in the binary dendro-gram.The hierarchy computation within our framework is scalable in terms of several similarity measures(c.f.section2)and clustering algorithms.Furthermore it is our objective to generate dynamic hierarchies under different aspects from the same information set. Therefore we need a basis which can be used effectively for a userdriven dynamic refinement of the hierarchy.The binary dendro-gram(cf.Figure2)which was computed previously provides such a basis.If the binary dendrogram has been determined thefinal hierarchy tree which represents the similarity structure of the in-formation space is derived form it(cf.Figure2).Therefore het-erogeneity values have to be assigned with each level of thefinal hierarchy tree.These values denote the allowed average dissimi-larities of the clusters at the levels in thefinal hierarchy tree(e.g., the maximum heterogeneity value H max is attached with the root node).These heterogeneity values can either be specified inter-actively by the user or determined automatically by our system in order to achieve optimized clustering of the data.Once the number of desired hierarchy levels and the heterogeneity values are speci-fied thefinal hierarchy is derived from the dendrogram according to the following algorithm:1.Create the root node of thefinal hierarchy tree(RHT)ac-cording to the dendrograms root node(RD).2.Test if the heterogeneity of RD’s children(max.2)are lessthen thefirst(current)element in the heterogeneity list.(a)If not,proceed with the node’s children at step2.(b)If yes,i.e.the heterogeneity of a child node in the bi-nary dendrogram is less than the current value in thelist,insert this node into thefinal hierarchy.The be-longing dendrogram’s node’s position of the insertednode is stored.3.All new inserted nodes form new sub-trees within thefinalhierarchy.Execute step1-2for all those stored nodes with the next value in the heterogeneity list.4.Iterate step1-3until the heterogeneity list is processed com-pletely.Using the binary dendrogram is very efficient.Once the den-drogram is created we do not need time consuming recomputations of the similarity matrices for refining the hierarchy tree.Thus complex information spaces can be browsed interactively in a top down like fashion by starting with an overview with only a few hierarchy levels(cf.Figure3)and refining embodiments by increasing the number of hierarchy levels for determining more subtle patterns in the data(cf.Figure4).Thefinal hierarchy tree contains information objects IO at its leaves.The remaining nodes represent clusters which fulfil the heterogeneity conditions associ-ated with each hierarchy level.The principle of hierarchy refine-ment is depicted in Figure3and Figure4.As the number of levels is increased,bigger clusters are split up into smaller sub-clusters (see Fig.3and4).Thus a stepwise exploration at arbitrary levels of detail is supported.4.VisualizationSupporting a variety of different exploration tasks(e.g.dis-playing different aspects of given information sets)as well as processing different types of information(e.g.hierarchical infor-mation structures or unstructured multi-dimensional information spaces)requires several visualization methods or a combination of these methods.Therefore our framework provides a range of different techniques.Beneath known techniques(c.f.section2)Figure3.Overview with3hierarchylevelsFigure4.Hierarchy refinement with7levelswe propose the new Focus+Context technique Magic-Eye-View for displaying complex hierarchy graphs and an adapted version of our ShapeVis for visualizing multidimensional information sets.4.1.Hierarchy VisualizationVisualizing the computed hierarchies becomes complicated as the number of levels and nodes increases.Standard2D hierarchy browsers can typically display about100nodes[17].Exceeding this number makes perceiving details difficult.Zooming and pan-ning do not provide a satisfying solution to this drawback due to loss of context information.In order to solve these problems sev-eral Focus+Context techniques have been developed,e.g.Graphi-cal Fisheye Views[20]or the Hyperbolic Browser[17]which ex-ploit distortion to enlarge a focus area while preserving context information.In order to achieve an additional degree of freedom for focussing arbitrary areas of the hierarchy graph,we propose the new Focus+Context technique Magic Eye View.Our approach maps a hierarchy graph onto the surface of a hemisphere.We then apply a projection in order to change the focus area interactively by moving the center of projection.Graph mapping onto the hemisphereLaying out the hierarchy tree is done with a simple2-d algorithm which is similar to the algorithm of Reingold and Tilford[19]. Thus we determine x y-coordinates for each node of the hier-archy within a Cartesian coordinate system.The graph is then mapped onto the surface of a hemisphere.Each point on a sphere can be described uniquely by two anglesλφ.Thus the deter-mined Cartesian coordinates can be mapped directly to spherical coordinates.plex hierarchy graph with p0at the origin plex hierarchy graph with enlarged focusregion4.2.Visualization of Multi-dimensional informationWe developed the new technique ShapeVis1for further explo-ration of multi-dimensional information sets(e.g.revealing at-tribute values of the data or determining object similarities withina cluster or at certain hierarchy levels).ShapeVis exploits an en-hanced spring model for arranging n-dimensional information ob-jects in2(3)-dimensional visualization space according to their at-tribute values.For reasons of readability,we briefly sketch thebasics of our model in this section.Enhanced spring modelInformation objects IO are described by a set of n attributes2which have continuous ranges of values.Thus each IO in then-dimensional information space is a n-tuple c1c n nwith c1c n0.The c i with i1n can be consideredas the coordinates of the IO in information space.(As an exam-ple,consider the IO as text documents and the attributes as certainkeywords.Then the coordinates c1c n of an IO are the fre-quencies of appearance of the key words in the document.)Several approaches(e.g.[11])use a classical spring model formapping objects from n-dimensional information space onto2(3)-dimensional visualization space.In the classical spring modelevery dimension of the information space is related to a pointd i23i1n in the visualization space.An infor-mation object IO c1c n is mapped to a point p in visuali-zation space using n springs-from each dimension point d i to p.The stiffness of the springs are set to the values c1c n.Thenthe location of p is searched where the spring model is in balance.1We use an adapted version of our technique introduced in[23]withinthe framework.2The terms data dimension and attribute are used exchangeable in thefollowing sections.Forfixed d i this location can be computed explicitly:p ∑n i1c i d i∑n i1c i(1)The location of p gives spatially intuitive information about the information objects,i.e.the bigger the value of a certain attribute (c i)the closer moves p towards d i.Furthermore objects with simi-lar properties are spatially close in the visualization.Beneath these advantages the classical spring model introduces two major draw-backs.1.ambiguity:Objects with different properties(coordinatesc1c n in information space)may collapse to the same point in visualization space(cf.[23]).2.insensitivity against coordinate scalings:The informationobjects c1c n and c1k c n k with k0can-not be distinguished in the visualization because they are mapped to the same point.In order to solve the problems mentioned above we assign an information object IO not only with a point but with a small shape which is composed off basic geometric primitives.Size,location and orientation of these primitives are determined based on the following enhanced spring model.As in the classical spring model,we place afixed point d i 23for every dimension of the information space.We attach n springs with the constant stiffness c0to p.The other ends of the springs are named p1p n.Now we consider n more springs-from p i to d i with the stiffness c i.The points p p1p n are free moveable,the points d1d n arefixed.Then we search for the state of balance of this spring system.Figure8illustrates this principle.Applying this model,an information object IO c1c n is described by the n1points p p1p n which can be computed explicitly by solving the linear system of equations (2)-(4):p ∑n i1w i d i∑n i1w i(2)withw ic ic c if or i1n(3)Then p1p n are obtained by:p i c p c i d ic c ii1n(4)Obviously the locations of p p1p n depend on the attribute val-ues c1c n of the information object and on the value of con-stant c.Thus the points p p1p n describe an information ob-ject IO c1c n uniquely.Thus we solve the problems intro-duced by the classical spring model.Obtaining geometric objectsEven if the points p p1p n describe an object uniquely-n1 points are not suitable for visualizing information objects.We studied the use of small closed free-form-surfaces(c.f.[23])for obtaining an intuitive imagination of the locations of p p1p n. But approximating point locations using free-form-surfaces be-comes rather difficult when the number of information objectsisFigure8.Enhanced spring model for an informationobject IO in4-dimensional information space.Figure9.Visualizing an object(1,2,1,1,2)with differentparameters c.increased to several hundreds or thousands because of the large number of polygons required for generating smooth surfaces.(E.g, the geometric complexity of the visualization exceeded a million polygons when approximating500objects with a satisfying geo-metric resolution.)Therefore we propose basic primitives(n cylinders)for com-posing geometric objects out of the p p1p n.These n cylin-ders tie up p and p i in order to build the geometric shape which is assigned with the according information object.Lo-cation,Orientation and lengths of each cylinder depend on p p1p n and the constant c.Thus the geomteric objects de-scribe the information objects IO c1c n uniquely.This principle and the influence of parameter c are illustrated in Figure 9.The strength of the deformation(length of cylinders)decreases, if parameter c is increased.If the length of all cylinders of a geo-metric object is less than a certain threshold,we replace this object with a small sphere around p.In this case we have the classical spring model.The parameter controlled deformation is very use-ful for visualizing a higher number of objects.First we obtain a global impression by visualizing all objects with a high parameter c.The objects are small points and we try to detect clusters.If we found a cluster,we zoom into it and decrease c such that the deformation of the cylinders provides more information about the object properties(i.e.a long cylinder into the direction of a certain d i denotes large data value of the according c i of this object).Fig-ure10illustrates this principle.Our approach is applied to a data set which measures6demographic parameters of106countries. We placed one point d i for each dimension of the data set in anFigure10.Visualization of a demographic data set with6dimensions with deformable geometric objects. equidistant way on the surface of a sphere.The global clustering of the data can be obtained within the sphere.The objects in the upper right,which have big values in the dimensions Baby mor-tality and Birthrate move towards the according dimension points d i.Furthermore we can verify the assumption that these objects have big values in the dimensions Baby mortality and Birthrate by applying the deformation to the geometric objects.The cylinders which point towards the Baby mortality and Birthrate dimension points are much longer than the cylinders which point towards the remaining d i(c.f.Figure10magnification of the upper cluster).In contrast to that the cluster lower left is characterized by countries with much bigger values with respect to the dimensions Literacy and Gross Domestic Product while the values of Baby mortality and Birthrate are rather small.bination of techniquesThe techniques introduced above are combined in our frame-work in order to supportflexible visualizations at arbitrary levels of detail.Therefore arbitrary subsets of the hierarchy can be se-lected for further exploration.Selection of cluster nodes-Each cluster node of the hierar-chy tree can be selected.Color is used to distinguish between cluster nodes and object nodes,whereby the size of a cluster,i.e.the number of objects is mapped to the intensity of thenode’s color.All objects of a selected cluster are visualized with ShapeVis in a separate display area.Selection of hierarchy levels-A representative is determined for each cluster which resides at the selected level by calcu-lating mean values of the data of all cluster members.Shape-Vis is used to visualize those representatives and all remain-ing objects at the selected level.Exploring clusters and levels with ShapeVis reveals basic infor-mation about attribute values and similarities between clusters and information objects.In order to identify concrete information con-tents,i.e.real attribute values,arbitrary shape objects can be se-lected and visualized with parallel coordinates[12].Labeling the coordinate axis and displaying the data values provides more de-tailed information about each information object.Figure11illustrates the combination of ShapeVis,Magic-Eye-View and Parallel Coordinates applied to a data set which de-scribes2440houses withfive attributes.The left picture shows the2440houses with ShapeVis and reveals three visual clusters. Exploration of single objects is rather complicated because of the dense object cloud.Reducing the size of the objects and zoom-ing into the cluster is possible with ShapeVis but makes analysis difficult due to the vanishing dimension points.In this case it is more meaningful to preprocess the data as introduced in section 3in order to form manageable subsets.The picture in the middle of Figure11depicts the hierarchical representation of the data set. The three major clusters are represented by the hierarchy nodes at thefirst level.Furthermore the tree shows that these clusters are split up into smaller sub-clusters at the following levels.These sub-clusters can be selected for further exploration.We selected thefirst level of the hierarchy tree and obtained the picture upper right which shows one representative for each of the three major clusters.Thus we can explore the relationships between the three clusters very easily by size,location and defor-mation of its graphical objects.The picture lower right shows the use of parallel coordi-nates.In our example we selected a single information object (Haus_516)which belongs to the cluster CN.1.The diagram dis-plays the concrete attribute values of(Haus_516)compared to the data values of the object which represents the whole cluster CN.1.5.Marching SphereIn many application domains(e.g.demographic research, health monitoring etc.)complex information structures are given within a spatial frame of reference.In general the usability of visual representations of given information can be enhanced sig-nificantly by displaying these frame of reference.Geographic In-formation Systems(GIS)provide various functions for displaying this spatial frame of reference but do not offer the functionality for depicting information structures like complex graphs or hierarchy trees.We propose in our framework the Marching Sphere as a new approach for solving these drawback.The Marching Sphere com-bines the visualization of complex information structures and the display of the spatial frame of reference within the same visual representation.In order to achieve this we had to solve a range of problems:The visualization of spatially referenced information struc-tures is rather complicated because of the high display com-plexity,since the information structures have to be visualized along with the spatial frame of reference.Techniques which generate compact embodiments of the given information set have to be applied such that the vi-。