概率论第8讲
概率论第8章
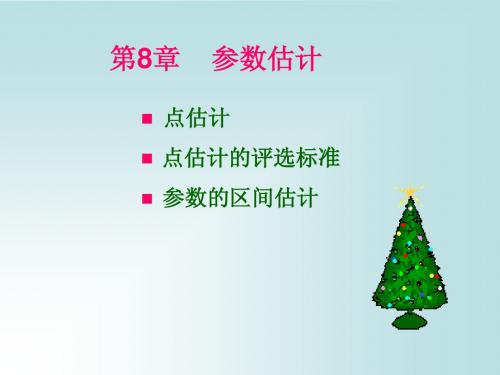
三、极大似然估计法(最先出现的是概率最大的)
定义
总体 的概率函数为 f x , 为未知参数
。
x1 , x 2 , , x n 是取自总体
的一个样本观察值,
如 = ˆ时, x1 , x 2 , , x n 被取到的概率最大,
即使似然函数
L 取到极大值。则称
ˆ为 的
x 1 x
, x 0 ,1
p 的似然函数为 L xi , p
i 1
n
p
xi
1 p 1 x
i
p
xi
i 1
n
1 p n x
i 1
n
i
令y
n
x i,得:
i 1
ln L x i , p y ln p n y ln 1 p 由对数似然方程 d ln L dp y p n y 1 p 0
参数估计
在实际问题中,对于一个总体ξ往往是 仅知其分布的类型,而其中所含的一个或 几个参数的值却是未知的,因此只有在确 定这些参数后,才能通过其分布来计算概 率,如何确定这些参数的数值呢?这就是 统计推断中的“参数估计”问题。
本章只研究总体分布是连续型或离散型两种情
形。为简便起见,我们引入一个对这两种情形通
2
这说明 是 的无偏估计
. S 是 的无偏估计。
2 2
~2 2 2 2 但 S 不是 的无偏估计。因此,一 般用 S 作为 的估计, ~2 2 但在 n 很大时, S 与 S 相差不大,这时二者就 不加以 区别了。
例2
设总体 有 E , D
2
, 1 , 2 , , n 是 的样本,
概率论与数理统计第8章
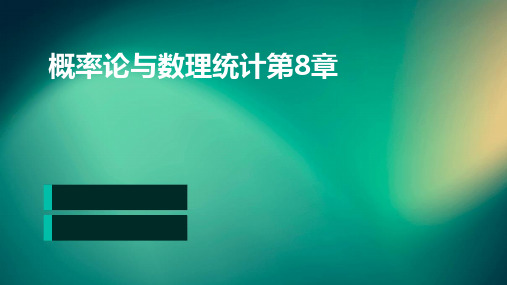
实验目的
通过实际数据验证概率论与数理统计中的理论 和方法,提高对理论知识的理解和应用能力。
01
1. 数据收集
从相关领域获取实际数据,确保数据 质量和代表性。
03
3. 理论应用
根据实验目的选择合适的理论和方法,进行 数据分析和解读。
05
02
实验方法
收集相关领域的实际数据,运用概率论与数 理统计中的理论和方法进行分析,如概率分 布、参数估计、假设检验等。
无偏性、有效性和一致性。
有效性
在所有无偏估计量中,有效性 是指方差最小的估计量。
点估计
用样本统计量来估计未知参数 的过程。
无偏性
估计量的期望值等于被估计的 参数值。
一致性
随着样本容量的增加,估计量 的值应趋近于被估计的参数值。
区间估计
区间估计
根据样本数据推断未知参数的可能取值范围。
置信区间和置信水平
本章内容主要包括贝叶斯推断的基本概念、贝叶斯推断的数学基础、贝叶斯推断 在参数估计和假设检验中的应用,以及贝叶斯推断的优缺点和与其他统计方法的 比较。
学习目标
01
掌握贝叶斯推断的基本原理和方法,理解贝叶斯推 断的数学基础。
02
学会使用贝叶斯方法进行参数估计和假设检验,了 解贝叶斯推断在实践中的应用。
案例总结
总结案例分析的成果,提炼出具有指导意义的结论和建议,为实际工 作提供参考和借鉴。
THANKS FOR WATCHING
感谢您的观看
置信区间是参数可能取值的范围,置信水平是该区间包含参数真 值的概率。
区间估计的步骤
确定置信水平、构造合适的统计量、计算置信区间。
假设检验的基本概念与步骤
假设检验
概率论与数理统计第八讲
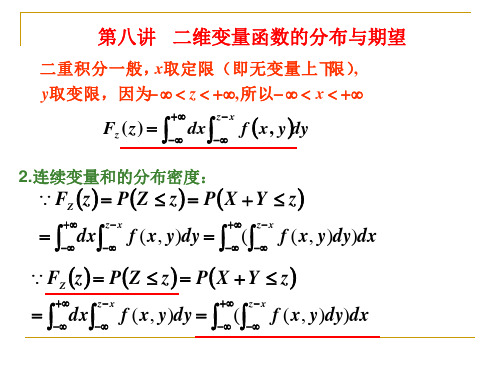
[(2
z)x
1
z 1
x2]
1
( z 2
4z
3)
20 2
2 z 3时 ,z 2 x 1;即 :
1
1
fz (z)
z2 f X ( x) fY (z x)dx
(2 z x)dx
z2
1 z2 3z 9
2
2
第八讲 二维变量函数的分布与期望
因 为1 z 2的 区 域 有2块 , 根 据fZ (z)
z
1 2
z2
z2
3z
3 2
综合以上几步,得:
f
Z
(z)
1 2
z2 z2
,
3z
3 2
,
0 z 1 1 z2
1 2
z
2 0,
3z
9 2
,
2 z 3 其它
第八讲 二维变量函数的分布与期望
例8-1-2(07数学一,11分)
已 知(
X ,Y
)的 概 率 密 度 为f
( x,
y)
2
x 0,
z 1
z1
2z z2
即:fZ (z) (2 z)2
0,
0 z 1 1 z2
其它
第八讲 二维变量函数的分布与期望
2. 平方和的分布
设二维连续随机变量 (X ,Y ) 的概率密度为 f (x, y), 寻求
Z X 2 Y 2 的分布。
考虑 Z 的分布函数:
FZ z PZ z PX 2 Y 2 z
第八讲 二维变量函数的分布与期望
FY1 ( y) P(Y1 y) P[min(X11, X12 , X13 ) y] 由 最 小 大 于 号 :FY1 ( y) 1 P[min(X11, X12 , X13 ) y]
概率论课件第8讲

正态分布的边缘分布仍为正态分布
fX (x)
fY ( y)
1
e
(
x1 212
)2
,
x
2 1
1
e
(
y2
2
2 2
)2
,
y
2 2
在这一讲中,我们与一维情形相对照, 介绍了二维随机变量的联合分布、边缘分布.
请注意联合分布和边缘分布的关系:
由联合分布可以确定边缘分布; 但由边缘分布一般不能确定联合分布.
(
x,
y
)
cy(
2 0
x ), ,
0 x 1,0 y x 其它
求 (1) c的值; (2)两个边缘密度。
解:(1)
f ( x,x)dy]dx
0
c
1
[
x2
(2
x)
/
2]dx
=5c/24=1,
0
y=x
1
x
c =24/5
f
(
x,
y)
cy(2
f (x, y)dy
1 r 2 x2
dy
r r 2 x2
2
2
r2 x2
r 2
故X的边缘密度函数为
r xr
f
X
(x)
2
r2 x2
r 2
0
r xr 其他
由对称性可得Y的边缘密度函数为
fY
(
x)
2
r2 y2
r 2
0
r yr 其他
(5) 若二维随机变量(X,Y)具有概率密度
(4) P{X 2 Y};
e(x y) , x 0, y 0
f (x, y)
8讲独立试验序列概型

伯努利定理) 定理 (伯努利定理 伯努利定理 设一次试验中事件A发生的概率 设一次试验中事件 发生的概率 重伯努利试验中, 为p(0<p<1), 则n重伯努利试验中 事 重伯努利试验中 恰好发生k次的概率用 表示, 件A恰好发生 次的概率用 n(k)表示 恰好发生 次的概率用p 表示 则
概率论与数理统计
第8讲 讲
2010-7-6
1
独立试验序列概型
2010-7-6
2
事件运算的最小项 任给n个事件 个事件A 取这n个事件 任给 个事件 1,A2,…,An, 取这 个事件 中的每一个,然后将其中的一些取逆 然后将其中的一些取逆, 中的每一个 然后将其中的一些取逆 则由 A1,A2,…,An产生的任何逻辑式都可由这 个 产生的任何逻辑式都可由这n个 事件中取逆的和不取逆的事件相交再相并 得到, 且称它们为这n个事件的一个最小项. 个事件的一个最小项 得到 且称它们为这 个事件的一个最小项 给定n个事件可产生多个不同的最小项 个事件可产生多个不同的最小项, 给定 个事件可产生多个不同的最小项 各 个最小项之间是互不相容的. 个最小项之间是互不相容的 而这n个事件能够逻辑上构成的任何事 而这 个事件能够逻辑上构成的任何事 可以由若干个最小项的并构成. 件, 可以由若干个最小项的并构成
P(B0 ) = P( ABC) = P( A)P(B)P(C) = 0.83 P(B ) = P( ABC + ABC + ABC ) 1 = P( ABC) + P( ABC) + P( ABC ) = 3×0.2×0.82 P(B2 ) = P( A BC + ABC + AB C ) = 3×0.2 ×0.8
概率论讲义Lecture8
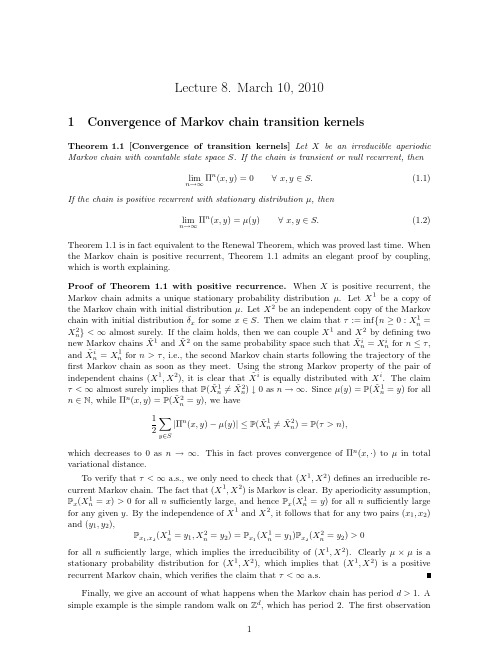
Lecture8.March10,20101Convergence of Markov chain transition kernelsTheorem1.1[Convergence of transition kernels]Let X be an irreducible aperiodic Markov chain with countable state space S.If the chain is transient or null recurrent,thenlimn→∞Πn(x,y)=0∀x,y∈S.(1.1) If the chain is positive recurrent with stationary distributionµ,thenlimn→∞Πn(x,y)=µ(y)∀x,y∈S.(1.2)Theorem1.1is in fact equivalent to the Renewal Theorem,which was proved last time.When the Markov chain is positive recurrent,Theorem1.1admits an elegant proof by coupling, which is worth explaining.Proof of Theorem1.1with positive recurrence.When X is positive recurrent,the Markov chain admits a unique stationary probability distributionµ.Let X1be a copy of the Markov chain with initial distributionµ.Let X2be an independent copy of the Markov chain with initial distributionδx for some x∈S.Then we claim thatτ:=inf{n≥0:X1n= X2n}<∞almost surely.If the claim holds,then we can couple X1and X2by defining two new Markov chains˜X1and˜X2on the same probability space such that˜X i n=X i n for n≤τ, and˜X i n=X1n for n>τ,i.e.,the second Markov chain starts following the trajectory of the first Markov chain as soon as they ing the strong Markov property of the pair of independent chains(X1,X2),it is clear that˜X i is equally distributed with X i.The claim τ<∞almost surely implies that P(˜X1n=˜X2n)↓0as n→∞.Sinceµ(y)=P(˜X1n=y)for all n∈N,whileΠn(x,y)=P(˜X2n=y),we have1 2y∈S|Πn(x,y)−µ(y)|≤P(˜X1n=˜X2n)=P(τ>n),which decreases to0as n→∞.This in fact proves convergence ofΠn(x,·)toµin total variational distance.To verify thatτ<∞a.s.,we only need to check that(X1,X2)defines an irreducible re-current Markov chain.The fact that(X1,X2)is Markov is clear.By aperiodicity assumption, P x(X1n=x)>0for all n sufficiently large,and hence P x(X1n=y)for all n sufficiently large for any given y.By the independence of X1and X2,it follows that for any two pairs(x1,x2) and(y1,y2),P x1,x2(X1n=y1,X2n=y2)=P x1(X1n=y1)P x2(X2n=y2)>0for all n sufficiently large,which implies the irreducibility of(X1,X2).Clearlyµ×µis a stationary probability distribution for(X1,X2),which implies that(X1,X2)is a positive recurrent Markov chain,which verifies the claim thatτ<∞a.s.Finally,we give an account of what happens when the Markov chain has period d>1.A simple example is the simple random walk on Z d,which has period2.Thefirst observationis that the state space S can be partitioned into d disjoint classes S0,S1,···,S d−1,and the Markov chain simply marches through these d classes sequentially.Let us make this statement more precise.Lemma1.2For x,y∈S,let D x,y={n≥0:Πn(x,y)>0}.Then d divides m−n for any m,n∈D x,y.Proof.By irreducibility,there exists k∈N withΠk(y,x)>0.ThereforeΠk+m(y,y)≥Πk(y,x)Πm(x,y)>0and k+m∈D y.Similarly k+n∈D y,which implies that d divides m−n.By Lemma1.2,given an x∈S,each y∈S is associated with an r y∈{0,1,···,d−1}, where r y is the residue modulo d of any n∈D x,y.Let S i:={y∈S:r y=i}for i= 0,1,···,d−1.Then S0,···,S d−1gives a disjoint union of S,and clearly the Markov chain marches through S0,S1,···,S d−1in this order cyclically.Theorem1.3[Convergence of transition kernels:periodic case]Let X be an irre-ducible Markov chain with countable state space S and period d>1.If the chain is transient or null recurrent,thenlimΠn(x,y)=0∀x,y∈S.(1.3)n→∞If the chain is positive recurrent with stationary distributionµ,thenΠn(x,y)=dµ(y)∀x,y∈S.(1.4)limn→∞r x+n≡r y(mod d)Proof.Let us consider the transition kernel˜Π=Πd.Clearly˜Π(x,y)>0if and only if x,y belongs to the same S r for some0≤r≤d−1.Restricted to each S r,the associated Markov chain is irreducible,and furthermore,aperiodic.In fact,it is simply X n restricted to a d-periodic subsequence of times.We can then apply Theorem1.1.The result follows once we observe that the stationary distribution˜µr(·)for the Markov chain on S r with transition kernel˜Πmust equal dµ(·)restricted to S r.2Perron-Frobenius TheoremWhen the state space S isfinite,everything boils down to the study of thefinite-dimensional transition matrixΠ.WhenΠhas positive entries,Perron’s theorem asserts that1is the dominant eigenvalue with a positive eigenvector.Theorem2.1[Perron’s Theorem]Let P be an n by n matrix with positive entries.Then P has a dominant eigenvalueλsuch that(i)λ>0and the associated eigenvector h has positive entries.(ii)λis a simple eigenvalue.(iii)Any other eigenvalueκof P satisfies|κ|<λ.(iv)P has no other eigenvector with non-negative entries.Proof.Let T :={t ≥0:P v ≥tv for some v ∈[0,∞)n ,v ≡0}.Then min 1≤i,j ≤n P ij ∈T since P e i ≥P ii e i ,and T ⊂[0, 1≤i,j ≤n P ij ]since |P v |∞≤ P ij |v |∞.Furthermore,T is a closed set since if there exists t n →t and P v n ≥t n v n ,where without loss of generality we may assume |v n |1=1,we can find a subsequence n i such that v n i converges to a limitingnon-negative vector v ∞with |v ∞|1=1.Then we see that P v ∞≥tv ∞,which proves that T is closed.Let λ>0be the maximum in T ,and let P v ≥λv for some non-negative vector v =0.We claim that in fact P v =λv and v ∈(0,∞)n .Indeed,if P v −λv ≡0,then by the positivity assumption on entries of P ,we have P 2v −λP v >0,which implies that there exists some λ >λwith P 2v −λ P v ≥0.Since P v ≥0,this implies that λ ∈T ,contradicting our assumption that λis the maximum in T .Therefore P v =λv .Since P has positive entries and v ≡0,λv =P v >0,which proves (i).If P w =λw for an eigenvector w distinct from any constant multiple of v ,then there exists c ∈R such that w +cv ≥0,w +cv ≡0and w +cv has zero components.Since P (w +cv )=λ(w +cv )>0by the positivity of P ,this creates a contradiction.To conclude that λis a simple eigenvalue of P ,it remains to rule out the existence of a generalized eigenvector w with eigenvalue λ,i.e.,P w =λw +cv for some c =0.Changing w if necessary,we can assume c >0.Replacing w by w +bv if necessary,we can guarantee that w >0.Then P w =λw +cv implies that max T >λ,a contradiction.Let P w =κw for some κ=λ,where κand w could both be complex.Then|P w |=|κ||w |≤P |w |,(2.5)where |w |=|(w (1),···,w (n ))|=(|w (1)|,···,|w (n )|).Therefore |κ|∈T and |κ|≤λ.The inequality in (2.5)is in fact strict,which implies |κ|<λ,unless w =e iθw for some θ∈R and w ≥0,in which case κ=λ.By (i)–(iii)applied to P T ,which has the same eigenvalues as P ,there exists a non-negative and non-trivial w such that P T w =λw .Suppose that h be a non-negative eigenvector of P with eigenvalue λ =λ.Thenλ w,h = w,P h = P T w,h =λ w,h .Since h =h ,we have w,h =0,which is not possible if h is non-negative.Remark 2.2For a transition probability matrix Π,clearly |Πv |∞≤|v |∞for any vector v ,and Π1=1.Therefore 1is an eigenvalue of Πand all other eigenvalues κof Πhas |κ|≤1.When Πis the transition matrix of an irreducible aperiodic Markov chain,there exists n 0∈N such that for all n ≥n 0,Πn has positive entries.Therefore by Perron’s Theorem,1is a simple dominant eigenvalue of Πn for n ≥n 0.It is then easy to see that 1is also a simple dominant eigenvalue of Π,since the eigenvectors and generalized eigenvectors are the same for Πand Πn .Remark 2.3Perron’s Theorem applied to the transpose of a positive transition matrix Πimplies the existence of a stationary positive probability distribution µ.The fact that 1is a simple dominant eigenvalue of ΠT implies that starting from any probability measure νon {1,···,n },(ΠT )n νconverges to µexponentially fast.The case when P is only assumed to be non-negative is covered by Frobenius’s Theorem.Theorem2.4[Frobenius’Theorem]Let P be an n by n matrix with non-negative entries. Then P has an eigenvalueλwith the following properties:(i)λ>0and there exists an associated eigenvector with non-negative entries.(ii)Any other eigenvalueκof P satisfies|κ|≤λ.(iii)If|κ|=λ,thenκ=e2πik/mλfor some k,m∈N with m≤n.Remark2.5IfΠis the transition matrix of an irreducible Markov chain with period d,then Πd is of block diagonal form withΠd(i,j)>0if and only if i and j belong to the same class, and there are exactly d such classes.Restricted to each class S i⊂{1,···,n},Πd is a positive matrix and therefore by Perron’s Theorem has1as a simple dominant eigenvalue.Therefore Πd has1as the dominant eigenvalue with multiplicity d.Consequently,counting multiplicity,Πhas exactly d eigenvalues of modulus1,and any other eigenvalueκofΠhas|κ|<1.We claim that these eigenvalues are precisely e2πik/d with k=0,1,···,d−1.Indeed,(Πd)T has d linearly independent eigenvectors,which are just the restriction of the invariant measureµof the Markov chain to the d classes of states S i,0≤i≤d−1.Denote the restriction ofµto S i byµi.ThenΠTµi=µi+1for0≤i≤d−1andΠTµd−1=µ0.The space V spanned by(µi)0≤i≤d−1is preserved byΠT,and on V,if we chooseµi to be the basis vectors,thenΠT is a permutation matrix and its characteristic polynomial isλd−1=0.Therefore the set of eigenvalues ofΠT restricted to V is precisely e2πik/d for0≤k≤d−1.This exhausts the possible eigenvalues of modulus1forΠT,and hence alsoΠ.For a proof of the Frobenius theorem,see Lax[1,Chapter16].There are also infinite-dimensional versions of the Perron-Frobenius Theorem for compact positive operators.3Reversible Markov chainsWe now consider a special class of Markov chains called reversible Markov chains.Definition3.1[Reversible Markov chains]A Markov chain with countable state space S and transition matrixΠis called reversible,if it admits a stationary measureµ,called a reversible measure,which satisfiesµ(x)Π(x,y)=µ(y)Π(y,x)∀x,y∈S.(3.6)Remark.In physics literature,(3.6)is called the detailed balance condition.Heuristically,µ(x)Π(x,y)represents the probabilityflow from x to y in equilibrium.(3.6)requires that the probabilityflow from x to y equals theflow from y to x,which is a sufficient condition forµto be stationary.Reversing theflow can be interpreted as reversing the time direction.Not all stationary measures are reversible measures,as can be seen for the uniform measureµ≡1 for an asymmetric simple random walk on Z.Note that the notion of a reversible Markov chain is accompanied by a reversible measure.Theorem3.2[Cycle condition for reversibility]Let X be an irreducible Markov chain with countable state space S and transition matrixΠ.A necessary and sufficient condition for the existence of a reversible measure for X is that(i)Π(x,y)>0if and only ifΠ(y,x)>0.(ii)For any loop x 0,x 1,···,x n =x 0with n i =1Π(x i −1,x i )>0,we haven i =1Π(x i −1,x i )Π(x i ,x i −1)=1.(3.7)Proof.Suppose µis a reversible measure for X and µ≡0.Then by irreducibility and the stationarity of µ,µ(x )>0for all x ∈S .The detailed balance condition (3.6)clearly implies (i).Similarly,reversibility implies that the probability flow along the cycle x 0,x 1,···,x n =x 0,i.e.,µ(x 0) n i =1Π(x i −1,x i ),equals the probability flow along the reversed cycle x n =x 0,x n −1,···,x 0,which is just µ(x 0) 1i =n Π(x i ,x i −1).This yields (3.7).Conversely,if Πsatisfies (i)and (ii),then for a given x ∈S ,we can define µ(x )=1,and for any y ∈S with a path of states z 0=x,z 1,···,z n =y connecting x and y such that Π(z i −1,z i )>0,we can define µ(y )=n i =1Π(z i −1,z i )Π(z i ,z i −1).Conditions (i)and (ii)guarantee that our definition of µis independent of the choice of path connecting x and y .It is easy to check that µsatisfies the detailed balance condition (3.6).Example 3.31An irreducible birth-death chain is reversible,since Theorem 3.2(i)fol-lows from irreducibility,and the cycle condition (3.7)is trivially satisfied due to the lack of cycles.Similarly,any irreducible Markov chain on a tree is reversible.2A random walk on a connected graph G =(V,E )with vertex set V and edge set E is a Markov chain with state space V and transition matrix Π(x,y )=1/d x for all y ∈V with {x,y }∈E ,where d x is the degree of x in G .It is easily seen that µ(x )=d x is a reversible measure for the walk with unit measure flow across each edge.More generally,if each edge {x,y }∈E is assigned a positive conductance C x,y =C y,x ,and Π(x,y )=C x,y z :{x,z }∈EC x,z ,then µ(x )= z :{x,z }∈E C x,z is a reversible measure for the walk with mass flow C x,y across each edge {x,y }∈E .We briefly explain the usefulness of reversibility.Let µbe a reversible measure for the Markov chain with transition matrix Π.Then Πis a self-adjoint operator on the Hilbert space L 2(S,µ)with inner product f,g µ:= x f (x )g (x )µ(x ).Indeed,formally for any f,g ∈L 2(S,µ),f,Πg µ= x ∈Sf (x ) y ∈SΠ(x,y )g (y )µ(x )= x,y ∈Sf (x )g (y )Π(y,x )µ(y )= Πf,g µ.All information about the Markov chain are encoded in its transition matrix.The self-adjointness of Πon L 2(µ,S )allows one to use spectral theory to study its spectrum,which otherwise is not possible when the Markov chain is irreversible.References[1]x.Linear Algebra .John Wiley &Sons,Inc.New York,1997.。
福大概率论第八章PPT课件
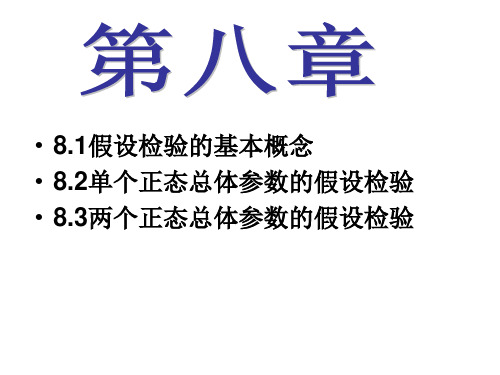
小概率事件在一次试验中基本上不会发生.
假设其中真有99个白球, 摸出红球的概率只有1/100, 这是小概率事件.
小概率事件在一次试验中竟然发生了,不能不 使人怀疑所作的假设.
这个例子中所使用的推理方法,可以称为
带概率性质的反证法
不妨称为概率反证法.
它不同于一般的反证法
(3) 左侧检验:检验假设H0: μ1 ≥ μ2
PU u
当U u时,拒绝H0 , 否则,接受H0.
例1. 从甲、乙两厂所生产的钢丝总体 X、Y中 各取50束作拉力强度试验, 得X 1208mPa,Y 1284mPa,
已知 X 80mPa,Y 94mPa
甲乙两厂钢丝的抗拉强度是否有显著差异?(α=0.05)
当T t 2 (m n 2)时,拒绝H0 ,
否则,接受H0.
(2) 右侧检验:检验假设H0: μ1≤ μ2
PT t (m n 2)
当T t (m n 2)时,拒绝H0, 否则,接受H0.
(3) 左侧检验:检验假设H0: μ1 ≥ μ2
PT t (m n 2)
当T t (m n 2)时,拒绝H0, 否则,接受H0.
一般的反证法要求在原假设成立的条件下 导出的结论是绝对成立的,如果事实与之矛盾, 则完全绝对地否定原假设.
概率反证法的逻辑是:如果小概率事件 在一次试验中居然发生,我们就以很大的把 握否定原假设.
在假设检验中,我们称这个小概率为显
著性水平,用 表示.
的选择要根据实际情况而定。
常取 0.1, 0.01, 0.05.
↓ A没发生→接受H0
关于原假设H0的拒绝域:拒绝原假设H0的区间 关于原假设H0的接受域:接受原假设H0的区间 双侧检验:拒绝域在两侧
《8.1.1 条件概率》 讲义
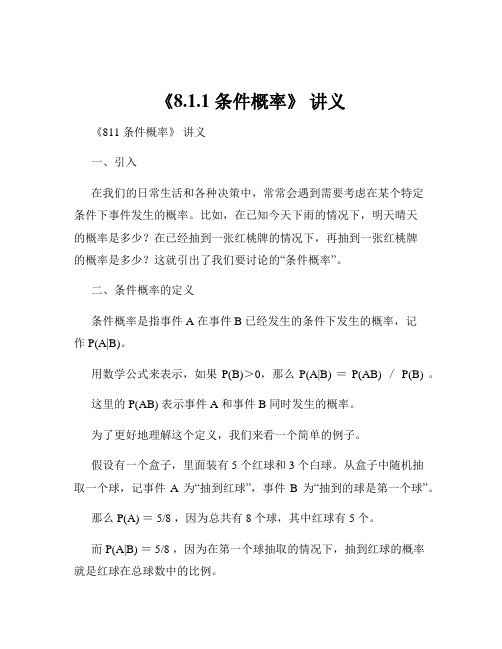
《8.1.1 条件概率》讲义《811 条件概率》讲义一、引入在我们的日常生活和各种决策中,常常会遇到需要考虑在某个特定条件下事件发生的概率。
比如,在已知今天下雨的情况下,明天晴天的概率是多少?在已经抽到一张红桃牌的情况下,再抽到一张红桃牌的概率是多少?这就引出了我们要讨论的“条件概率”。
二、条件概率的定义条件概率是指事件 A 在事件 B 已经发生的条件下发生的概率,记作 P(A|B)。
用数学公式来表示,如果P(B)>0,那么P(A|B) =P(AB) /P(B) 。
这里的 P(AB) 表示事件 A 和事件 B 同时发生的概率。
为了更好地理解这个定义,我们来看一个简单的例子。
假设有一个盒子,里面装有 5 个红球和 3 个白球。
从盒子中随机抽取一个球,记事件A 为“抽到红球”,事件B 为“抽到的球是第一个球”。
那么 P(A) = 5/8 ,因为总共有 8 个球,其中红球有 5 个。
而 P(A|B) = 5/8 ,因为在第一个球抽取的情况下,抽到红球的概率就是红球在总球数中的比例。
三、条件概率的性质1、非负性:0 ≤ P(A|B) ≤ 1 。
2、规范性:如果 B 是必然事件,那么 P(A|B) = P(A) 。
四、计算条件概率的方法1、利用定义计算如前面提到的例子,先计算P(AB) 和P(B),然后相除得到P(A|B) 。
2、利用缩小样本空间法还是以盒子抽球为例,如果已知事件 B 发生了,那么我们可以把 B 当作新的样本空间,然后计算在这个新样本空间中事件A 发生的概率。
比如已知第一个球抽到的是红球,那么在剩下的 7 个球中,再计算抽到红球的概率。
五、条件概率的应用1、在医疗诊断中的应用假设某种疾病在人群中的发病率为 01% ,而某种检测方法对患有该疾病的人检测结果为阳性的概率为 99% ,对未患该疾病的人检测结果为阳性的概率为 1% 。
现在有一个人的检测结果为阳性,那么他真正患有该疾病的概率是多少?设事件 A 为“患有疾病”,事件 B 为“检测结果为阳性”。
- 1、下载文档前请自行甄别文档内容的完整性,平台不提供额外的编辑、内容补充、找答案等附加服务。
- 2、"仅部分预览"的文档,不可在线预览部分如存在完整性等问题,可反馈申请退款(可完整预览的文档不适用该条件!)。
- 3、如文档侵犯您的权益,请联系客服反馈,我们会尽快为您处理(人工客服工作时间:9:00-18:30)。
解 按表(8),(9)分别得所要求的分布密度为
x
0 3 2 1 3 2 1 3
x
0 3 2 2
h
1
1
2
1 概率 3
h
概率
1 1 3 6 1 6
2 2 6
1 3 0 12 12 2 1 1 12 12 12 3 1 0 12 12
29 2013-7-15
设二维连续型随机变量(x,h)的分布密度 为j(x,y), 按式(6)有
1 2 1
2
1 2(1 2 )
( x 2 2 xy y 2 )
e
(13)
( x , y )
求关于x及关于h的边缘分布密度.
34 2013-7-15
解 按式(10)有
j1 ( x) j ( x, y )dy
1 2 1
37 2013-7-15
2003年考研数学试题(数一,一(5)): 设二维随机变量(X,Y)的概率密度为
6 x, 0 x y 1, f ( x, y ) 其他. 0,
则P{X+Y1}=_______. (分值: 4分) x+y=1 0xy1 y y=x
y=1
x
38 2013-7-15F1 ( x) x
j ( x, y)dy dx.
可见关于x的边缘分布也是连续型的, 它 的分布密度为
j1 ( x) j ( x, y)dy
(10)
30 2013-7-15
同理可得, 关于h的边缘分布也是连续型 的, 它的分布密度为
j 2 ( y) j ( x, y)dx
D
31 (6 x y )dy dx 0 28 3 8 (2) P{(x ,h ) D} j ( x, y )d
1
D
5 3 x 1 (6 x y )dy dx 0 24 2 8
1
22 2013-7-15
x
(7)
下面分别讨论离散型, 连续型分布中的 边缘分布.
25 2013-7-15
设(x,h)为二维离散型随机变量, 它的分 布密度如表(2)所示, 则 P{x ai } P{x ai ,h b1} P{x ai ,h b2 } P{x ai ,h b j }
D
(4)
其中D为xOy平面内任一区域.
18 2013-7-15
最常遇到的二维连续型分布是二维正态 分布. 它的分布密度为
j ( x, y )
1 2 1 2 1
2
e
( x a )2 2 ( x a )( y b ) ( y b ) 2 2 2 2 1 2 2(1 ) 1 2 1
第四节 边缘分布
23 2013-7-15
设(x,h)为一个二维随机变量. 事件{x<x} 就是指事件{x<x,h<+}. 由(x,h)的分布 函数可以定出x的分布函数: P{x<x}=P{x<x,h<+}. 这样定出的一维分布称为关于x的边缘 分布. 类似地,关于h的边缘分布的分布函数为 P{h<y}=P{x<+, h<y}.
9 2013-7-15
设二维离散型随机变量(x,h)可能取的值 为 (a1,b1),...,(a1,bj),...,(ai,b1),...,(ai,bj),..., 且事件{x=ai,h=bj}的概率为pij(i,j=1,2,...), 即 P{x=ai,h=bj}=pij,
10 2013-7-15
2
1 2(1 2 )
( x 2 2 xy y 2 )
x2 2
e
dy
e 2
1 1
2
( y x )2 2(1 2 )
e
dy.
35 2013-7-15
作代换 v y x , 2
1
便得关于x的边缘分布密度为
e 1 j1 ( x) e dv 2 e 2 ( x ). x2 2 v2 2 x2 2
(9)
p1
p2
...
pj
...
其中
p j pij
i
( j 1, 2,)
27 2013-7-15
例3 设二维离散型随机变量(x,h)的分布 密度为 h
x
0 3 2 2 1 1 2 1 3 0 12 12 2 1 1 12 12 12 3 1 0 12 12
求关于x及关于h的边缘分布的分布密度
3 2013-7-15
在研究某族人的身长与体重之间的联系 时, 要从这族人中抽出若干个来, 测量他 们的身高与体重. 每抽一个人出来, 就有 一个由身长, 体重组成的有序数组(x,h). 这个有序数组是根据试验结果(抽到的 人)而确定的.
4 2013-7-15
一般地说, 如果由两个变量所组成的有 序数组即二维变量(x,h), 它的取值是随 着试验结果而确定的, 那末称这个二维 变量(x,h)为二维随机变量. 相应地, 称 (x,h)的取值规律为二维分布.
又(1)D为xOy平面内由不等式x<1,y<3所定 的区域; (2) D为xOy平面内由不等式x+y<3 所定的区域. 求P{(x,h)D}
20 2013-7-15
(1)和(2)中D的示意图 y y 4
4 3
2
3 2
O
1
2
x
O
1
2
x
21 2013-7-15
解 (1) P{(x ,h ) D} j ( x, y )d
即这边缘分布为N(0,1).
36 2013-7-15
按式(11), 同理可得关于h的边缘分布密度为
1 j 2 ( y) e 2
y2 2
( y ).
即这边缘分布也是N(0,1).
从这个例子可以看到:二维正态分布的两个 边缘分布都是一维正态分布, 而且这两个边 缘分布都不依赖于参数. 这一事实表明: 单 单由关于x及关于h的边缘分布, 一般说来是 不能确定二维随机变量(x,h)的分布的.
0 x 1, 其余地方.
按式(11), 得关于h的边缘分布密度为
y 1 2 y 1dx 1 , 0 y 2, j 2 ( y) 0 2 0, 其余地方.
33 2013-7-15
例5 设(x,h)服从二维正态分布, 它的分布 密度为
j ( x, y )
pi1 pi 2 pij pij pi (i 1, 2,)
j
因此x的分布密度为
x
概率
a1
p1
a2
p2
...
...
ai
pi
...
(8)
26 2013-7-15
同理可得, 关于h的边缘分布也是离散型 的, 且它的分布密度为
h
概率
b1 b2 ... bj ...
24 2013-7-15
设(x,h)的分布函数为F(x,y), 那末关于x 的边缘分布函数为
F1 ( x) P{x x} P{x x,h } lim F ( x, y ).
y
(6)
同理可得, 关于h的边缘分布函数为
F2 ( y ) lim F ( x, y )
解 所求概率为
P{ X Y 1}
0.5 0
1 x
f ( x, y )dydx
0.5 1 x 0
1 x
x
6 xdydx 6 x y x dx
那末(x,h)的分布密度为表格
x
a1
ai
h
b1
...
bj
...
p11
pi1
...
... ... ...
p1j
pij
...
... ... ...
(2)
0 pij 1,
p
i, j
i, j
1.
11 2013-7-15
例1 一口袋中有三个球, 它们依次标有数 字1,2,2. 从这袋中任取一球后, 不放回袋 中, 再从袋中任取一球. 设每次取球时, 袋中各个球被取到的可能性相同. 以x,h 分别记第一次, 第二次取得的球上标有 的数字, 求(x,h)的分布密度.
D
f ( x, y )d lim
D1 D
D1
f ( x, y )d ,
其中D1为D内的任意有界区域.
17 2013-7-15
二维分布密度具有下列性质: (1) j ( x, y ) 0;
(2)
j ( x, y )dydx 1;
(3) P{(x ,h ) D} j ( x, y )d ,
6 2013-7-15
与一维时相仿, 定义二维分布的分布函 数为 F(x,y)=P{x<x,h<y}, (1) 其中x,y是任意实数.
y
y
D
O 图6-1
x
x
7 2013-7-15
第二节 二维离散型随机变量
8 2013-7-15
设(x,h)为一个二维随机变量. 如果它可 能取的值的全体是有限个或可数多个数 组, 则称(x,h)为二维离散型随机变量, 称 它的分布为二维离散型分布.
5 2013-7-15
也就是说, 对于平面上任意一个集D, {(x,h)D}代表了一个随机事件. D确定 后, P{(x,h)D}随之唯一确定. 由这个对 应关系定出, 以平面上的集D为自变量, 函数值在区间[0,1]上的函数, 称为二维 随机变量(x,h)的分布. 它表明了二维随 机变量(x,h)取数组的规律. 简称二维随 机变量的分布为二维分布.