2012年美国大学生数学建模竞赛B题特等奖文章翻译要点
美国数学建模题目2001至2012翻译
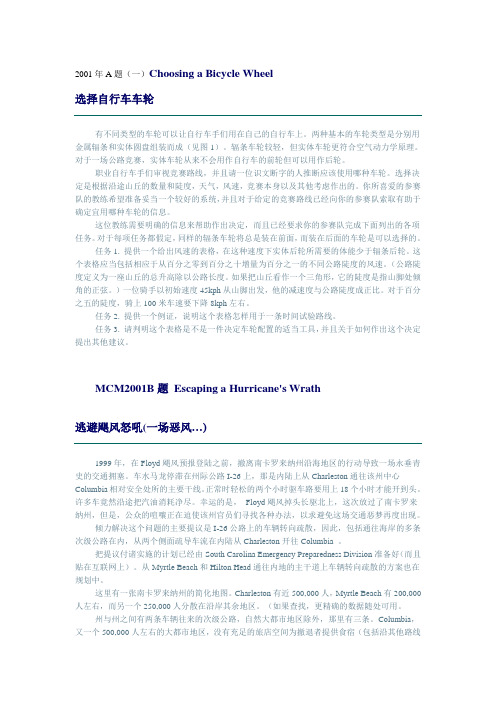
2001年A题(一)Choosing a Bicycle Wheel选择自行车车轮有不同类型的车轮可以让自行车手们用在自己的自行车上。
两种基本的车轮类型是分别用金属辐条和实体圆盘组装而成(见图1)。
辐条车轮较轻,但实体车轮更符合空气动力学原理。
对于一场公路竞赛,实体车轮从来不会用作自行车的前轮但可以用作后轮。
职业自行车手们审视竞赛路线,并且请一位识文断字的人推断应该使用哪种车轮。
选择决定是根据沿途山丘的数量和陡度,天气,风速,竞赛本身以及其他考虑作出的。
你所喜爱的参赛队的教练希望准备妥当一个较好的系统,并且对于给定的竞赛路线已经向你的参赛队索取有助于确定宜用哪种车轮的信息。
这位教练需要明确的信息来帮助作出决定,而且已经要求你的参赛队完成下面列出的各项任务。
对于每项任务都假定,同样的辐条车轮将总是装在前面,而装在后面的车轮是可以选择的。
任务1. 提供一个给出风速的表格,在这种速度下实体后轮所需要的体能少于辐条后轮。
这个表格应当包括相应于从百分之零到百分之十增量为百分之一的不同公路陡度的风速。
(公路陡度定义为一座山丘的总升高除以公路长度。
如果把山丘看作一个三角形,它的陡度是指山脚处倾角的正弦。
)一位骑手以初始速度45kph从山脚出发,他的减速度与公路陡度成正比。
对于百分之五的陡度,骑上100米车速要下降8kph左右。
任务2. 提供一个例证,说明这个表格怎样用于一条时间试验路线。
任务3. 请判明这个表格是不是一件决定车轮配置的适当工具,并且关于如何作出这个决定提出其他建议。
MCM2001B题Escaping a Hurricane's Wrath逃避飓风怒吼(一场恶风…)1999年,在Floyd飓风预报登陆之前,撤离南卡罗来纳州沿海地区的行动导致一场永垂青史的交通拥塞。
车水马龙停滞在州际公路I-26上,那是内陆上从Charleston通往该州中心Columbia相对安全处所的主要干线。
正常时轻松的两个小时驱车路要用上18个小时才能开到头。
2012年美国数学建模竞赛

2012年美国数学建模竞赛(MCM/ICM)将于2.10(正月十九)—2.13(共4天)举行,我们邀请冯国灿教授介绍如何准备美国数学建模竞赛,内容包括:1、报名注册注意事项2、写作技巧3、数学建模知识的准备4、典型案例分析欢迎备战参赛的同学参加!时间:2011年12月21日(周三)晚上7:30地点:数学楼202【嘉宾简介】冯国灿:教授、博士生导师、中山大学数学与计算科学学院数学系副主任、广东省大学生数学建模竞赛组委会委员。
是十几年的数学建模竞赛的资深教练,经常参加全国、广东省大学生数学建模竞赛论文的评阅工作,指导数模美赛、国赛,获奖无数。
2012年美国(国际)大学生数学建模竞赛官方网站:/undergraduate/contests比赛时间:美国东部时间:2012年2月9日(星期四)下午8点-2月13日下午8点(共4天)北京时间:2012年2月10日(星期五)上午9点-2月14日上午9点农历:正月十九-正月二十三比赛之前注册报名1. 注意所有时间都是美国东部时间。
2. 参赛机构(institute)派出的参赛队伍没有数量限制。
3. 每支参赛队伍都必须有一位来自参赛机构(institute)的导师(faculty advisor),并由指导老师负责为其指导队伍注册报名,每位指导老师的账号最多可以注册两支队伍。
报名费用1. 每支队伍$100。
如果想要赛后的评语,可以再加$100(非必需)。
2.报名费用将在网上报名期间被扣除,缴费方式为Mastercard 或 VISA card。
请确认报名处有打印机,确认缴费后,组委会将在数秒内收到费用,为参赛队伍分配一个control number,请务必将此显示control number的网页打印出来,这将是参赛队唯一的注册证明,因为你将不会收到Email形式的注册认证。
这张纸上同时包含该队导师注册时使用的邮箱和密码,是整个比赛手续的必须信息。
3. 报名后,导师仍然可以登入系统,在比赛前可以修改参赛人员、报名地址、联系方式等信息。
2012年美国大学生数学建模竞赛B题特等奖文章翻译
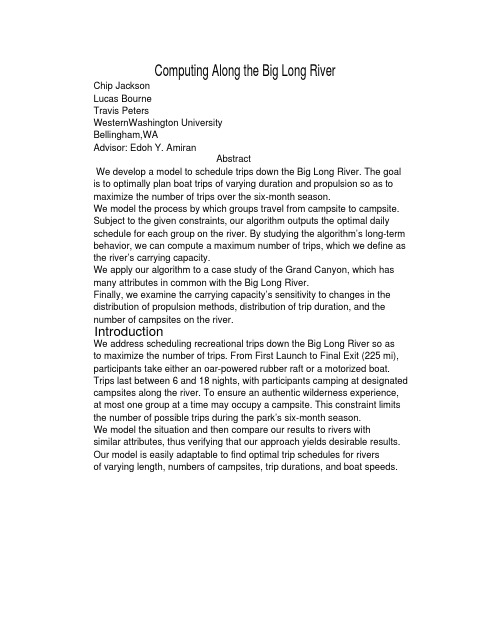
We develop a model to schedule trips down the Big Long River. The goalComputing Along the Big Long RiverChip JacksonLucas BourneTravis PetersWesternWashington UniversityBellingham,WAAdvisor: Edoh Y. AmiranAbstractis to optimally plan boat trips of varying duration and propulsion so as tomaximize the number of trips over the six-month season.We model the process by which groups travel from campsite to campsite.Subject to the given constraints, our algorithm outputs the optimal dailyschedule for each group on the river. By studying the algorithm’s long-termbehavior, we can compute a maximum number of trips, which we define asthe river’s carrying capacity.We apply our algorithm to a case study of the Grand Canyon, which hasmany attributes in common with the Big Long River.Finally, we examine the carrying capacity’s sensitivity to changes in thedistribution of propulsion methods, distribution of trip duration, and thenumber of campsites on the river.IntroductionWe address scheduling recreational trips down the Big Long River so asto maximize the number of trips. From First Launch to Final Exit (225 mi),participants take either an oar-powered rubber raft or a motorized boat.Trips last between 6 and 18 nights, with participants camping at designatedcampsites along the river. To ensure an authentic wilderness experience,at most one group at a time may occupy a campsite. This constraint limitsthe number of possible trips during the park’s six-month season.We model the situation and then compare our results to rivers withsimilar attributes, thus verifying that our approach yields desirable results.Our model is easily adaptable to find optimal trip schedules for riversof varying length, numbers of campsites, trip durations, and boat speeds.No two groups can occupy the same campsite at the same time.Campsites are distributed uniformly along the river.Trips are scheduled during a six-month period of the year.Group trips range from 6 to 18 nights.Motorized boats travel 8 mph on average.Oar-powered rubber rafts travel 4 mph on average.There are only two types of boats: oar-powered rubber rafts and motorizedTrips begin at First Launch and end at Final Exit, 225 miles downstream.*simulates river-trip scheduling as a function of a distribution of trip*can be applied to real-world rivers with similar attributes (i.e., the Grand*is flexible enough to simulate a wide range of feasible inputs; andWhat is the carrying capacity of the riverÿhe maximum number ofHow many new groups can start a river trip on any given day?How should trips of varying length and propulsion be scheduled toDefining the Problemmaximize the number of trips possible over a six-month season?groups that can be sent down the river during its six-month season?Model OverviewWe design a model thatCanyon);lengths (either 6, 12, or 18 days), a varying distribution of propulsionspeeds, and a varying number of campsites.The model predicts the number of trips over a six-month season. It alsoanswers questions about the carrying capacity of the river, advantageousdistributions of propulsion speeds and trip lengths, how many groups canstart a river trip each day, and how to schedule trips.ConstraintsThe problem specifies the following constraints:boats.AssumptionsWe can prescribe the ratio of oar-powered river rafts to motorized boats that go onto the river each day.There can be problems if too many oar-powered boats are launched with short trip lengths.The duration of a trip is either 12 days or 18 days for oar-powered rafts, and either 6 days or 12 days for motorized boats.This simplification still allows our model to produce meaningful results while letting us compare the effect of varying trip lengths.There can only be one group per campsite per night.This agrees with the desires of the river manager.Each day, a group can only move downstream or remain in its current campsiteÿt cannot move back upstream.This restricts the flow of groups to a single direction, greatly simplifying how we can move groups from campsite to campsite.Groups can travel only between 8 a.m. and 6 p.m., a maximum of 9hours of travel per day (one hour is subtracted for breaks/lunch/etc.).This implies that per day, oar-powered rafts can travel at most 36 miles, and motorized boats at most 72 miles. This assumption allows us to determine which groups can reasonably reach a given campsite.Groups never travel farther than the distance that they can feasibly travelin a single day: 36 miles per day for oar-powered rafts and 72 miles per day for motorized boats.We ignore variables that could influence maximum daily travel distance, such as weather and river conditions.There is no way of accurately including these in the model.Campsites are distributed uniformly so that the distance between campsites is the length of the river divided by the number of campsites.We can thus represent the river as an array of equally-spaced campsites.A group must reach the end of the river on the final day of its trip:A group will not leave the river early even if able to.A group will not have a finish date past the desired trip length.This assumption fits what we believe is an important standard for theriver manager and for the quality of the trips.MethodsWe define some terms and phrases:Open campsite: Acampsite is open if there is no groupcurrently occupying it: Campsite cn is open if no group gi is assigned to cn.Moving to an open campsite: For a group gi, its campsite cn, moving to some other open campsite cm ÿ= cn is equivalent to assigning gi to the new campsite. Since a group can move only downstream, or remain at their current campsite, we must have m ÿ n.Waitlist: The waitlist for a given day is composed of the groups that are not yet on the river but will start their trip on the day when their ranking onthe waitlist and their ability to reach a campsite c includes them in theset Gc of groups that can reach campsite c, and the groups are deemed “the highest priority.” Waitlisted groups are initialized with a current campsite value of c0 (the zeroth campsite), and are assumed to have priority P = 1 until they are moved from the waitlist onto the river.Off the River: We consider the first space off of the river to be the “final campsite” cfinal, and it is always an open campsite (so that any number of groups can be assigned to it. This is consistent with the understanding that any number of groups can move off of the river in a single day.The Farthest Empty CampsiteOurscheduling algorithm uses an array as the data structure to represent the river, with each element of the array being a campsite. The algorithm begins each day by finding the open campsite c that is farthest down the river, then generates a set Gc of all groups that could potentially reach c that night. Thus,Gc = {gi | li +mi . c},where li is the groupÿs current location and mi is the maximum distance that the group can travel in one day.. The requirement that mi + li . c specifies that group gi must be able to reach campsite c in one day.. Gc can consist of groups on the river and groups on the waitlist.. If Gc = ., then we move to the next farthest empty campsite.located upstream, closer to the start of the river. The algorithm always runs from the end of the river up towards the start of the river.. IfGc ÿ= ., then the algorithm attempts tomovethe groupwith the highest priority to campsite c.The scheduling algorithm continues in this fashion until the farthestempty campsite is the zeroth campsite c0. At this point, every group that was able to move on the river that day has been moved to a campsite, and we start the algorithm again to simulate the next day.PriorityOnce a set Gc has been formed for a specific campsite c, the algorithm must decide which group to move to that campsite. The priority Pi is a measure of how far ahead or behind schedule group gi is:. Pi > 1: group gi is behind schedule;. Pi < 1: group gi is ahead of schedule;. Pi = 1: group gi is precisely on schedule.We attempt to move the group with the highest priority into c.Some examples of situations that arise, and how priority is used to resolve them, are outlined in Figures 1 and 2.Priorities and Other ConsiderationsOur algorithm always tries to move the group that is the most behind schedule, to try to ensure that each group is camped on the river for aFigure 1. The scheduling algorithm has found that the farthest open campsite is Campsite 6 and Groups A, B, and C can feasibly reach it. Group B has the highest priority, so we move Group B to Campsite 6.Figure 2. As the scheduling algorithm progresses past Campsite 6, it finds that the next farthest open campsite is Campsite 5. The algorithm has calculated that Groups A and C can feasibly reach it; since PA > PC, Group A is moved to Campsite 5.number of nights equal to its predetermined trip length. However, in someinstances it may not be ideal to move the group with highest priority tothe farthest feasible open campsite. Such is the case if the group with thehighest priority is ahead of schedule (P <1).We provide the following rules for handling group priorities:?If gi is behind schedule, i.e. Pi > 1, then move gi to c, its farthest reachableopen campsite.?If gi is ahead of schedule, i.e. Pi < 1, then calculate diai, the number ofnights that the group has already been on the river times the averagedistance per day that the group should travel to be on schedule. If theresult is greater than or equal (in miles) to the location of campsite c, thenmove gi to c. Doing so amounts to moving gi only in such a way that itis no longer ahead of schedule.?Regardless of Pi, if the chosen c = cfinal, then do not move gi unless ti =di. This feature ensures that giÿ trip will not end before its designatedend date.Theonecasewhere a groupÿ priority is disregardedisshownin Figure 3.Scheduling SimulationWe now demonstrate how our model could be used to schedule rivertrips.In the following example, we assume 50 campsites along the 225-mileriver, and we introduce 4 groups to the river each day. We project the tripFigure 3. The farthest open campsite is the campsite off the river. The algorithm finds that GroupD could move there, but GroupD has tD > dD.that is, GroupD is supposed to be on the river for12 nights but so far has spent only 11.so Group D remains on the river, at some campsite between 171 and 224 inclusive.schedules of the four specific groups that we introduce to the river on day25. We choose a midseason day to demonstrate our modelÿs stability overtime. The characteristics of the four groups are:. g1: motorized, t1 = 6;. g2: oar-powered, t2 = 18;. g3: motorized, t3 = 12;. g4: oar-powered, t4 = 12.Figure 5 shows each groupÿs campsite number and priority value foreach night spent on the river. For instance, the column labeled g2 givescampsite numbers for each of the nights of g2ÿs trip. We find that each giis off the river after spending exactly ti nights camping, and that P ÿ 1as di ÿ ti, showing that as time passes our algorithm attempts to get (andkeep) groups on schedule. Figures 6 and 7 display our results graphically.These findings are consistent with the intention of our method; we see inthis small-scale simulation that our algorithm produces desirable results.Case StudyThe Grand CanyonThe Grand Canyon is an ideal case study for our model, since it sharesmany characteristics with the Big Long River. The Canyonÿs primary riverrafting stretch is 226 miles, it has 235 campsites, and it is open approximatelysix months of the year. It allows tourists to travel by motorized boat or byoar-powered river raft for a maximum of 12 or 18 days, respectively [Jalbertet al. 2006].Using the parameters of the Grand Canyon, we test our model by runninga number of simulations. We alter the number of groups placed on thewater each day, attempting to find the carrying capacity for the river.theFigure 7. Priority values of groups over the course of each trip. Values converge to P = 1 due to the algorithm’s attempt to keep groups on schedule.maximumnumber of possible trips over a six-month season. The main constraintis that each trip must last the group’s planned trip duration. Duringits summer season, the Grand Canyon typically places six new groups onthe water each day [Jalbert et al. 2006], so we use this value for our first simulation.In each simulation, we use an equal number of motorized boatsand oar-powered rafts, along with an equal distribution of trip lengths.Our model predicts the number of groups that make it off the river(completed trips), how many trips arrive past their desired end date (latetrips), and the number of groups that did not make it off the waitlist (totalleft on waitlist). These values change as we vary the number of new groupsplaced on the water each day (groups/day).Table 1 indicates that a maximum of 18 groups can be sent down theriver each day. Over the course of the six-month season, this amounts to nearly 3,000 trips. Increasing groups/day above 18 is likely to cause latetrips (some groups are still on the river when our simulation ends) and long waitlists. In Simulation 1, we send 1,080 groups down river (6 groups/day?80 days) but only 996 groups make it off; the other groups began near the end of the six-month period and did not reach the end of their trip beforethe end of the season. These groups have negligible impact on our results and we ignore them.Sensitivity Analysis of Carrying CapacityManagers of the Big Long River are faced with a similar task to that of the managers of the Grand Canyon. Therefore, by finding an optimal solutionfor the Grand Canyon, we may also have found an optimal solution forthe Big Long River. However, this optimal solution is based on two key assumptions:?Each day, we put approximately the same number of groups onto theriver; and?the river has about one campsite per mile.We can make these assumptions for the Grand Canyon because they are true for the Grand Canyon, but we do not know if they are true for the Big Long River.To deal with these unknowns,wecreate Table 3. Its values are generatedby fixing the number Y of campsites on the river and the ratio R of oarpowered rafts to motorized boats launched each day, and then increasingthe number of trips added to the river each day until the river reaches peak carrying capacity.The peak carrying capacities in Table 3 can be visualized as points ina three-dimensional space, and we can find a best-fit surface that passes (nearly) through the data points. This best-fit surface allows us to estimatethe peak carrying capacity M of the river for interpolated values. Essentially, it givesM as a function of Y and R and shows how sensitiveM is tochanges in Y and/or R. Figure 7 is a contour diagram of this surface.The ridge along the vertical line R = 1 : 1 predicts that for any givenvalue of Y between 100 and 300, the river will have an optimal value ofM when R = 1 : 1. Unfortunately, the formula for this best-fit surface is rather complex, and it doesn’t do an accurate job of extrapolating beyond the data of Table 3; so it is not a particularly useful tool for the peak carrying capacity for other values ofR. The best method to predict the peak carrying capacity is just to use our scheduling algorithm.Sensitivity Analysis of Carrying Capacity re R and DWe have treatedM as a function ofR and Y , but it is still unknown to us how M is affected by the mix of trip durations of groups on the river (D).For example, if we scheduled trips of either 6 or 12 days, how would this affect M? The river managers want to know what mix of trips of varying duration and speed will utilize the river in the best way possible.We use our scheduling algorithm to attempt to answer this question.We fix the number of campsites at 200 and determine the peak carrying capacity for values of R andD. The results of this simulation are displayed in Table 4.Table 4 is intended to address the question of what mix of trip durations and speeds will yield a maximum carrying capacity. For example: If the river managers are currently scheduling trips of length?6, 12, or 18: Capacity could be increased either by increasing R to be closer to 1:1 or by decreasing D to be closer to ? or 12.?12 or 18: Decrease D to be closer to ? or 12.?6 or 12: Increase R to be closer to 4:1.ConclusionThe river managers have asked how many more trips can be added tothe Big Long Riverÿ season. Without knowing the specifics ofhowthe river is currently being managed, we cannot give an exact answer. However, by applying our modelto a study of the GrandCanyon,wefound results which could be extrapolated to the context of the Big Long River. Specifically, the managers of the Big Long River could add approximately (3,000 - X) groups to the rafting season, where X is the current number of trips and 3,000 is the capacity predicted by our scheduling algorithm. Additionally, we modeled how certain variables are related to each other; M, D, R, and Y . River managers could refer to our figures and tables to see how they could change their current values of D, R, and Y to achieve a greater carrying capacity for the Big Long River.We also addressed scheduling campsite placement for groups moving down the Big Long River through an algorithm which uses priority values to move groups downstream in an orderly manner.Limitations and Error AnalysisCarrying Capacity OverestimationOur model has several limitations. It assumes that the capacity of theriver is constrained only by the number of campsites, the trip durations,and the transportation methods. We maximize the river’s carrying capacity, even if this means that nearly every campsite is occupied each night.This may not be ideal, potentially leading to congestion or environmental degradation of the river. Because of this, our model may overestimate the maximum number of trips possible over long periods of time. Environmental ConcernsOur case study of the Grand Canyon is evidence that our model omits variables. We are confident that the Grand Canyon could provide enough campsites for 3,000 trips over a six-month period, as predicted by our algorithm. However, since the actual figure is around 1,000 trips [Jalbert et al.2006], the error is likely due to factors outside of campsite capacity, perhaps environmental concerns.Neglect of River SpeedAnother variable that our model ignores is the speed of the river. Riverspeed increases with the depth and slope of the river channel, makingour assumption of constant maximum daily travel distance impossible [Wikipedia 2012]. When a river experiences high flow, river speeds can double, and entire campsites can end up under water [National Park Service 2008]. Again, the results of our model don’t reflect these issues. ReferencesC.U. Boulder Dept. of Applied Mathematics. n.d. Fitting a surface to scatteredx-y-z data points. /computing/Mathematica/Fit/ .Jalbert, Linda, Lenore Grover-Bullington, and Lori Crystal, et al. 2006. Colorado River management plan. 2006./grca/parkmgmt/upload/CRMPIF_s.pdf .National Park Service. 2008. Grand Canyon National Park. High flowriver permit information. /grca/naturescience/high_flow2008-permit.htm .Sullivan, Steve. 2011. Grand Canyon River Statistics Calendar Year 2010./grca/planyourvisit/upload/Calendar_Year_2010_River_Statistics.pdf .Wikipedia. 2012. River. /wiki/River .Memo to Managers of the Big Long RiverIn response to your questions regarding trip scheduling and river capacity,we are writing to inform you of our findings.Our primary accomplishment is the development of a scheduling algorithm.If implemented at Big Long River, it could advise park rangerson how to optimally schedule trips of varying length and propulsion. Theoptimal schedule will maximize the number of trips possible over the sixmonth season.Our algorithm is flexible, taking a variety of different inputs. Theseinclude the number and availability of campsites, and parameters associatedwith each tour group. Given the necessary inputs, we can output adaily schedule. In essence, our algorithm does this by using the state of theriver from the previous day. Schedules consist of campsite assignments foreach group on the river, as well those waiting to begin their trip. Given knowledge of future waitlists, our algorithm can output schedules monthsin advance, allowing managementto schedule the precise campsite locationof any group on any future date.Sparing you the mathematical details, allow us to say simply that ouralgorithm uses a priority system. It prioritizes groups who are behindschedule by allowing them to move to further campsites, and holds backgroups who are ahead of schedule. In this way, it ensures that all trips willbe completed in precisely the length of time the passenger had planned for.But scheduling is only part of what our algorithm can do. It can alsocompute a maximum number of possible trips over the six-month season.We call this the carrying capacity of the river. If we find we are below ourcarrying capacity, our algorithm can tell us how many more groups wecould be adding to the water each day. Conversely, if we are experiencingriver congestion, we can determine how many fewer groups we should beadding each day to get things running smoothly again.An interesting finding of our algorithm is how the ratio of oar-poweredriver rafts to motorized boats affects the number of trips we can send downstream. When dealing with an even distribution of trip durations (from 6 to18 days), we recommend a 1:1 ratio to maximize the river’s carrying capacity.If the distribution is skewed towards shorter trip durations, then ourmodel predicts that increasing towards a 4:1 ratio will cause the carryingcapacity to increase. If the distribution is skewed the opposite way, towards longer trip durations, then the carrying capacity of the river will always beless than in the previous two cases—so this is not recommended.Our algorithm has been thoroughly tested, and we believe that it isa powerful tool for determining the river’s carrying capacity, optimizing daily schedules, and ensuring that people will be able to complete their trip as planned while enjoying a true wilderness experience.Sincerely yours,Team 13955。
2012数学建模B题解答
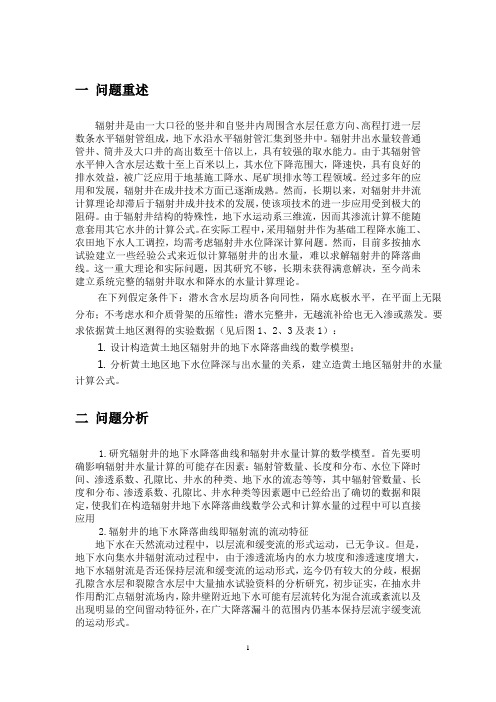
一问题重述辐射井是由一大口径的竖井和自竖井内周围含水层任意方向、高程打进一层数条水平辐射管组成,地下水沿水平辐射管汇集到竖井中。
辐射井出水量较普通管井、筒井及大口井的高出数至十倍以上,具有较强的取水能力。
由于其辐射管水平伸入含水层达数十至上百米以上,其水位下降范围大,降速快,具有良好的排水效益,被广泛应用于地基施工降水、尾矿坝排水等工程领域。
经过多年的应用和发展,辐射井在成井技术方面已逐渐成熟。
然而,长期以来,对辐射井井流计算理论却滞后于辐射井成井技术的发展,使该项技术的进一步应用受到极大的阻碍。
由于辐射井结构的特殊性,地下水运动系三维流,因而其渗流计算不能随意套用其它水井的计算公式。
在实际工程中,采用辐射井作为基础工程降水施工、农田地下水人工调控,均需考虑辐射井水位降深计算问题。
然而,目前多按抽水试验建立一些经验公式来近似计算辐射井的出水量,难以求解辐射井的降落曲线。
这一重大理论和实际问题,因其研究不够,长期未获得满意解决,至今尚未建立系统完整的辐射井取水和降水的水量计算理论。
在下列假定条件下:潜水含水层均质各向同性,隔水底板水平,在平面上无限分布;不考虑水和介质骨架的压缩性;潜水完整井,无越流补给也无入渗或蒸发。
要求依据黄土地区测得的实验数据(见后图1、2、3及表1):1.设计构造黄土地区辐射井的地下水降落曲线的数学模型;1.分析黄土地区地下水位降深与出水量的关系,建立造黄土地区辐射井的水量计算公式。
二问题分析1.研究辐射井的地下水降落曲线和辐射井水量计算的数学模型。
首先要明确影响辐射井水量计算的可能存在因素:辐射管数量、长度和分布、水位下降时间、渗透系数、孔隙比、井水的种类、地下水的流态等等,其中辐射管数量、长度和分布、渗透系数、孔隙比、井水种类等因素题中已经给出了确切的数据和限定,使我们在构造辐射井地下水降落曲线数学公式和计算水量的过程中可以直接应用2.辐射井的地下水降落曲线即辐射流的流动特征地下水在天然流动过程中,以层流和缓变流的形式运动,已无争议。
2012年全国数学建模B题优秀论文
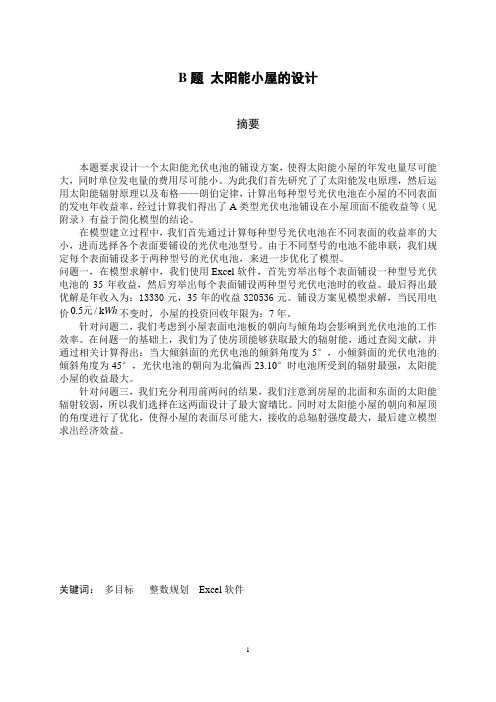
B 题 太阳能小屋的设计摘要本题要求设计一个太阳能光伏电池的铺设方案,使得太阳能小屋的年发电量尽可能大,同时单位发电量的费用尽可能小。
为此我们首先研究了了太阳能发电原理,然后运用太阳能辐射原理以及布格——朗伯定律,计算出每种型号光伏电池在小屋的不同表面的发电年收益率,经过计算我们得出了A 类型光伏电池铺设在小屋顶面不能收益等(见附录)有益于简化模型的结论。
在模型建立过程中,我们首先通过计算每种型号光伏电池在不同表面的收益率的大小,进而选择各个表面要铺设的光伏电池型号。
由于不同型号的电池不能串联,我们规定每个表面铺设多于两种型号的光伏电池,来进一步优化了模型。
问题一,在模型求解中,我们使用Excel 软件,首先穷举出每个表面铺设一种型号光伏电池的35年收益,然后穷举出每个表面铺设两种型号光伏电池时的收益。
最后得出最优解是年收入为:13330元,35年的收益320536元。
铺设方案见模型求解,当民用电价Wh k /5.0元不变时,小屋的投资回收年限为:7年。
针对问题二,我们考虑到小屋表面电池板的朝向与倾角均会影响到光伏电池的工作效率。
在问题一的基础上,我们为了使房顶能够获取最大的辐射能,通过查阅文献,并通过相关计算得出:当大倾斜面的光伏电池的倾斜角度为5°,小倾斜面的光伏电池的倾斜角度为45°,光伏电池的朝向为北偏西23.10°时电池所受到的辐射最强,太阳能小屋的收益最大。
针对问题三,我们充分利用前两问的结果,我们注意到房屋的北面和东面的太阳能辐射较弱,所以我们选择在这两面设计了最大窗墙比。
同时对太阳能小屋的朝向和屋顶的角度进行了优化,使得小屋的表面尽可能大,接收的总辐射强度最大,最后建立模型求出经济效益。
关键词: 多目标 整数规划 Excel 软件一、问题重述在设计太阳能小屋时,需在建筑物外表面(屋顶及外墙)铺设光伏电池,光伏电池组件所产生的直流电需要经过逆变器转换成220V 交流电才能供家庭使用,并将剩余电量输入电网。
2012美国大学生数学建模竞赛_MCM_A题

SummaryMany scholars conclude that leaf shape is highly related with the veins. Based on this theory, we assume the leaf growth in each direction satisfies a function. For the leaves in the same tree, the parameters are different; for those of separate trees, the function mode is different. Thus the shape of leaf differs from that of another. In the end of section 3, we simulate one growing period and depict the leaf shape.Through thousands of years of evolution, the leaves find various wa ys to make a full use of natural resources, including minimizing overlapping individual shadows. In order to find the main factors promoting the evolution of leaves, we analyze the distribution of adjacent leaves and the equilibrium point of photosynthesis and respiration. Besides, we also make a coronary hierarchical model and transmission model of the solar radiation to analyze the influence of the branches.As to the tree structure and the leaf shape, first we consider one species. Different tree shapes have different space which is built up by the branch quality and angle, effect light distribution, ventilation and humidity and concentration of CO2 in the tree crown. These are the factors which affect the leaf shape according to the model in section 1. Here we analyze three typical tree shapes: Small canopy shape, Open center shape and Freedom spindle shape, which can be described by BP network and fractional dimension model. We find that the factors mainly affect the function of Sthat affects the additional leaf area. Factors are assembled in different ways to create different leaf shapes. So that the relationship between leaf shape and tree profile/branching structure is proved.Finally we develop a model to calculate the leaf mass from the basic formula of . By adjusting the crown of a tree to a half ellipsoid, we first define thefunction of related factors,such as the leaf density and the effective ratio of leaf area. Then we develop the model using calculus. With this model, weapproximately evaluate the leaf mass of a middle-sized tree is 141kg.Dear editor,How much the leaves on a tree weigh is the focus of discussion all the time. Our team study on the theme following the current trend and we find something interesting in the process.The tree itself is component by many major elements. In our findings, we analyze the leaf mass with complicated ones, like leaf shape, tree structure and branch characteristics, which interlace with each other.With the theory that leaf shape is highly related with the veins, we assume theleaf growth in each direction satisfies a function . For the leaves in thesame tree, the parameters are different; for those of separate trees, the function mode is di fferent. That’s why no leaf shares the same shape. Also, we simulate one growing period and depict the leaf shape.In order to find the main factors promoting the evolution of leaves, we analyze the distribution of adjacent leaves and the equilibrium point of photosynthesis and respiration. Besides, we also make a coronary hierarchical model and transmission model of the solar radiation to analyze the influence of the branches.As to the tree structure and the leaf shape, different tree shapes have differe nt space which is built up by the branch quality and angle, effect light distribution, ventilation and humidity and concentration of CO2 in the tree crown that affect leaf shapes. Here we analyze three typical tree shapes which can be described by BP network and fractional dimension model. We find that the factors mainly affect the function of Sthat affects the additional leaf area. Factors are assembled in different ways to create different leaf shapes. So that the relationship between leaf shape and tree profile or branching structure is proved.Finally we develop the significant model to calculate the leaf mass from thebasic formula of . By adjusting the crown of a tree to a half ellipsoid,we first define the function of related factors and then we develop the model using calculus. With this model, we approximately evaluate the leaf mass of a middle-sized tree is 141kg.We are greatly appreciated that if you can take our findings into consideration. Thank you very much for your precious time for reading our letter.Yours sincerely,Team #14749Contents1. Introduction (4)2. Parameters (4)3. Leaves have their own shapes (5)3.1 Photosynthesis is important to plants (5)3.2 How leaves grow? (6)3.3 Build our model (7)3.4 A simulation of the model (10)4. Do the shapes maximize exposure? (14)4.1 The optimum solution of reducing overlapping shadows (14)4.1.1 The distribution of adjacent leaves (14)4.1.2 Equilibrium point of photosynthesis and respiration (15)4.2 The influence of the “volume” of a tree and its branches (17)4.2.1 The coronary hierarchical model (17)4.2.2 Spatial distribution model of canopy leaf area (18)4.2.3 Transmission model of the solar radiation (19)5. Is leaf shape related to tree structure? (20)5.1 The experiment for one species (21)5.2 Different tree shapes affect the leaf shapes (23)5.2.1 The light distribution in different shapes (23)5.2.2 Wind speed and humidity in the canopy (23)5.2.3 The concentration of carbon dioxide (24)5.3 Conclusion and promotion (25)6. Calculus model for leaf mass (26)6.1 How to estimate the leaf mass? (26)6.2 A simulation of the model (28)7. Strengths and Weakness (29)7.1 Strengths (29)7.2 Weaknesses (30)8. Reference (30)1. IntroductionHow much do the leaves on a tree weigh? Why do leaves have the various shapes that they have? How might one estimate the actual weight of the leaves? How might one classify leaves?We human-beings have never stopped our steps on exploring the natural world. But, as a matter of fact, the answer to those questions is still unresolved. Many scientists continue to study on this area. Recently , Dr. Benjamin Blonder (2010) achieved a new breakthrough on the venation networks and the origin of the leaf econo mics spectrum. They defined a standardized set of traits – density , distance and loopiness and developed a novel quantitative model that uses these venation traits to model leaf-level physiology .Now, it is commonly thought that there are four key leaf functional traits related to leaf economics: net carbon assimilation rate, life span, leaf mass per area ratio and nitrogen content.2. Parametersthe area a leaf grows decided by photosynthesisthe additional leaf area in one growing periodthe leaf growing obliquity Pthe total photosynthetic rate 0d R the dark respiration rate of leavesn P the net photosynthetic rateh the height of the canopyd the distance between two branchesdi the illumination intensity of scattered light from a given directionthe solar zenith angleh the truck highh the crown high13. Leaves have their own shapes3.1 Photosynthesis is important to plantsIt is widely accepted that two leaves are different, no matter where they are chosen from; even they are from the very tree. To understand how leaves grow is helpful to answer why leaves have the various shapes that they have.The canopy photosynthesis and respiration are the central parts of most biophysical crop and pasture simulation models. In most models, the acclamatory responses of protein and the environmental conditions, such as light, temperature and CO2 concentration, are concerned[1].In 1980, Farquhar et al developed a model named FvCB model to describe photosynthesis[2]:The FvCB model predicts the net assimilation rate by choosing the minimum between the Rubisco-limited net photosynthetic rate and the electron transport-limited net photosynthetic rate.Assume A n, A c, A j are the symbols for net assimilation rate, the Rubisco-limited net photosynthetic rate and the electron transport-limited net photosynthetic rate respectively, and the function can be described as:(1)(2)where and are the intercellular partial pressures of CO2 and O2,respectively, and are the Michaelis–Menten coefficients ofRubisco for CO2and O2, respectively, is the CO2compensation point inthe absence of (day respiration in andis the photosystem II electron transport rate that is used for CO2fixation and photorespiration[3][4].We apply the results of this model to build the relationship between the photosynthesis and the area a leaf grows during a period of time. It can be released as:(3)and are the area of the target leaf and the period of time it grows.is a function which can transfer the amount ofCO2into the area the leaf grows and the are parameters which affect S p. S p can be used as a constraint condition in our model.3.2 How leaves grow?As the collocation of computer hardware and software develops, people can refer to bridging biology, morphogenesis, applied mathematics and computer graphics to simulate living organisms[5], thus how to model leaves is of great challenge. In 2001, Dengler and Kang[6]brought up the thought that leaf shape is highly related to venation patterns. Recently, Runions[7] brought up a method to portray the leaf shape by analyzing venation patterns. Together with the Lindenmayer system (L-system), an advanced venation model can adjust the growth better that it solved the problem occurred in the previous model that the secondary veins are retarded.We knowleaves have various shapes.For example, leaves can be classified in to simple leaves which have an undivided blade and compound leaves whose blade is divided into two or more distinctleaflets such as the Fabaceae. As to the shape of a leaf, it may have marginal dentations of the leaf blades or not, and like a palm with various fingers or an elliptical cake. Judd et al defined a set of terms which describe the shape of leaves as follows [8]:We chose entire leaves to produce this model as a simplification. What’s more, they confirmed again that the growth of venations relates with that of the leaf.To disclose this relationship, Relative Elementary Rate of Growth (RERG) can be introduced to depict leaves growth [9]. RERG is defined as the growth rate per distance, in the definitive direction l at a point p of the growing object, yielding(4)Considered RERG , the growth patterns of leaves are also different. Roth-Nebelsick et al brought up four styles in their paper [10]:3.3 Build our modelWe chose marginal growth to build our model. Amid all above-mentioned studies, weFigure 3.1 Terms pertinent to the description of leaf shapes.Figure 3.2 A sample leaf (a) and the results of its: (b) marginal growth, (c) uniform isotropic (isogonic) growth, (d) uniform anisotropic growth, and (e) non-uniform anisotropic growth.Figure 3.3 The half of a leaf is settled in x-y plane like this with primary vein overlapping x-axis. The leaf grows in the direction of .assume that the leaf produce materials it needs to grow by photosynthesis to expand its leaf area from its border and this process is only affected by what we have discussed in the previous section about photosynthesis. The border can be infinitesimally divided into points. Set as the angle between the x-axis and thestraight line connecting the grid origin and one point on the curve,andas the growth distance in the direction of . To simplify the model, we assume that the leaf grows symmetrical. We put half of the leaf into the x-y plane and make the primary vein overlap x-axis.This is how we assume the leaf grows.In one circle of leaf growth, anything that photosynthesis provided transfers into theadditional leaf area, which can be described as:(5)while in the figure.In this case, we can simulate leaf growth thus define the leaf shape by using iterative operations the times N a leaf grow in its entire circle ①.① For instance, if the vegetative circle of a leaf is 20 weeks on average, the times of iterative operations N can beset as 20 when we calculate on a weekly basis.Figure 3.4 The curves of the adjacent growing period and their relationship.First, we pre-establish the border shape of a leaf in the x-y plane, yielding . where , the relevant satisfies:(6)(7) In the first growing period, assume , the growth distance in the direction of ,satisfies:(8)(9) It releases the relationship of the coordinates in the adjacent growing period. In this case, we can use eq.(9)to predict the new border of the leaf after one period of growth②:(10)And the average simple recursions are③:②That means, in the end of period 1.③As we both change the x coordinate and the y coordinate, in the new period, these two figures relate through those in the last period in the functions.(11)After simulate the leaf borders of the interactive periods, use definite integral ④ to settle parametersin the eq.(8) then can be calculated in each direction of , thus the exact shape of a leaf in the next period is visible.When the number of times N the leaf grows in its life circle applies above-mentioned recursions to iterate N times and the final leaf shape can be settled.By this model, we can draw conclusions about why leaves have different shapes. For the leaves on the same tree, they share the same method of expansion which can be described as the same type of function as Eq.(8). The reason why they are different, not only in a sense of big or small, is that in each growing period they acquire different amount of materials used to expand its own area. In a word, the parameters in the fixedly formed eq.(8) are different for any individual leaf on the same tree. For the leaves of different tree species, the corresponding forms of eq.(8) are dissimilar. Some are linear, some are logarithmic, some are exponential or mixtures of that, which settle the totally different expansion way of leaf, are related with the veins. On that condition, the characters can be divided by a more general concept such as entire or toothed.3.4 A simulation of the modelWe set the related parameters by ourselves to simulate the shape of a leaf and to express the model better.First we initialize the leaf shape by simulating the function of a leaf border at the④The relationship must meet eq.(5).Figure 3.5 and the recurrence relations.beginning of growing period 1 in the x-y plane. By observation, we assume that themovement of the initial leaf border satisfied:(12)Suppose the curve goes across the origin of coordinates, then the constraint conditionscan be:(13)Thus the solution to eq.(13) is:(14)By using Mathematica we calculatewhereandthe area of the half leaf is: (15)Wesettleaccording to the research by S. V . Archontoulisin et al [11] in eq.(3). On a weekly basis, theparameter. In eq.(15), we have .Figure 3.6When assume ,thus constraint condition eq.(5) becomes: (16)When eq.(8) is linear and after referring to Runions’s paper, we assume that Y -valuedecreases when X-value increases, which means the leaf grows faster at the end of theprimary vein. If the grow rate at the end of the primary vein is 0,as ,eq.(8) can be described as: (17)where b is decided by eq.(16).To settle the value of b , we calculate multiple sets of data by Excel then use a planecurve to trace them and get the approximation of b . In this method, we use grid toapproximate .Apparently,is monotone.When b is 2: The square of each square is 0.0139cm 2, and the total number of the squares in theadditional area is about 240. SoFigure 3.7When b is 2.5:Thesquare of each squareis 0.0240cm 2, and the total number of the squares in theadditional area is about 201. SoWhen b is 3:The square of each square is 0.0320cm 2, and the total number of the squares in the additional area is about 193. SoAfter comparing, we can draw a conclusion that fit eq.(16)best; accordingly, in period 1: (18)In each growing period, may be different for the amount of material produced isFigure 3.9Figure 3.8related with various factors, such as the change of relative location and CO 2 or O 2concentration, and other reasons. By using the same method, the leaf shape in period2 or other period can be generated on the basis of the previous growing period untilthe end of its life circle.What’s more, when eq.(8) is remodeled, the corresponding leaf shape can be changed.With , the following figure shows the transformationof a leaf shape in the firstgrowing period with the samesettled above and we can see that the shape will be dissected in the end.4. Do the shapes maximize exposure?4.1 The optimum solution of reducing overlapping shadows4.1.1 The distribution of adjacent leavesV ein is the foundation of the leaves. With the growing of veins, the leaves graduallyexpand around. The distribution of main and lateral veins plays an important decisiverole in the shapes of leaves. The scientists created a mathematical model which usesthree decisive factors - the relationship between the rate of photosynthesis, leaf life,carbon consumption or nitrogen consumption, to simulate the leaves’ shape. Becauseof carbon consumption is a constant for one tree, and we take the neighboring leavesin the same growth cycle to observe. So, we can only focus on one factor - the rate ofphotosynthesis.Figure 3.10The shape differs from figure 3.7-3.9, as the kind offunction of is different. In this case, it is a cubic model while a linear model in figure 3.7-3.9.Through the observation of dicotyledon, leaves on a branch will grow in a staggered way that can reduce the overlapping individual shadows of adjacent leaves and make them get more sunlight (Figure 4.1).Figure 4.1 The rotation distribution of leavesBase on the similar environment, we assume that adjacent leaves nearly have the same shape. From the perspective of looking down, the leaves grow from a point on the branch. So, we can simplify the vertical view of leaves as a circle of which the radius is the length of a vein which is represented with r. The width of the leaf is represented with w. The angle between two leaves is represented with β(Figure4.2).Figure 4.2 The vertical view of leavesThe leaves should use the space as much as possible, and for the leaf with one main vein, oval is the best choice. In general, βis between 15°and 90°. In this way, effectively reduce the direct overlapping area. According to the analysis of the first question, r and w are determined by the rate of photosynthesis and respiration. Besides, the width of leaf is becoming narrower when the main vein turns to be thinner.4.1.2 Equilibrium point of photosynthesis and respirationThe organism produced by photosynthesis firstly satisfies needs of leaf itself. Then the remaining organism delivered to the root to meet the growth needs of the tree. As we know, respiration needs to consume organism. If the light is not sufficient, organism produced by photosynthesis may no longer be able to afford the materials required for the growth of leaves. There should be an equilibrium point so as toprevent the leaf is behindhand in its circumstances.The formula [12] that describes the photosynthetic rate in response to light intensity with gradual exponential growth index can be expressed as:(19) Where P is total photosynthetic rate, m ax P is maximum photosynthetic rate ofleaves, a is initial solar energy utilization and I is photosynthetic photon quanta flux density .The respiration rate is affected by temperature ,using the formal of index to describe as follows:025102T d d R R -=⋅ (20)Where T is temperature and 0d R is the dark respiration rate of leaves, when the temperature is 25 degrees. As a model parameter, 0d R can be determined bynonlinear fitting. Therefore, the net photosynthetic rate can be expressed in the indexform as follows:(21)Where n P is the net photosynthetic rate, which does not include the concentration ofcarbon dioxide and other factors. The unit of n P is 21m ol m s μ--⋅⋅.We assume that the space for leaf growth is limited, the initial area of the leaf is 0S . At this point, the entire leaf happens to be capable of receiving sunlight. If the leaf continue to grow, some part of the leaf will be in the shadows and the area in the shadows is represented with x . Ignore the fluctuation cycle of photosynthesis and respiration, on average, the duration of photosynthesis is six hours per day . In the meanwhile, the respiration is ongoing all the time. When the area increased to S , we established an equation as follows:(22)where μ is the remaining organism created by the leaf per day . And from where 0μ=, which means the organism produced by photosynthesis has all been broken down completely in the respiration, we get the equilibrium point.4n n d S P x P R ⋅=+ (23) The proportion of the shaded area in the total area:4nn d P xS P R =+ (24)Cite an example of oak trees, we found the following data (Figure 4.3), which shows the relationship between net photosynthesis and dark respiration during 160 days. Thehabitat of the trees is affected by the semi-humid monsoon climate.Figure 4.3 The diurnal rate of net photosynthesis and dark respiration [13]In general, 217n P m ol m s μ--=⋅⋅,214d R m ol m s μ--=⋅⋅. Taking the given numbersinto the equation, we can get the result.0025xS =From the result, we can see that in the natural growth of leaves, with the weakening of photosynthesis, the leaves will naturally stop growing once they come across the blade between blocked. Therefore, the leaves can always keep overlapping individual shadows about 25%, so as to maximize exposure.4.2 The influence of the “volume” of a tree and its branches4.2.1 The coronary hierarchical modelMr. Bōken and Dr. J. Fischer (1987) found that in order to adequate lighting, leaves have different densities and the branches is distributed according to certain rules. They observed tropical plants in Miami, found that the ratio of main branch and two side branches is 1:0.94:0.87, and the angles between them are 24.4°and 36.9°. According to the computer simulation, the two angles can maximize the exposure of leaves.For simply, we use hemisphere to simulate the shape of the canopy. According to the light transmittance rate, we can divide the canopy into outer and inner two layers(Figure 4.3). The volume of the hemisphere depends on the size and distribution of branches.Figure 4.3 The coronary hierarchical modelThe angle between the branches will affect the depth of penetration of sun radiation and the distribution of leaves. Besides, thickness of the branches will affect the transfer of nutrients to the leaves.4.2.2 Spatial distribution model of canopy leaf areaAssume that the distribution of leaves is uniform in the section xz but not uniform in the y direction, as the figure 4.3. Then, we can get the formula of leaf area index (LAI) as follows [14]:/20/21(,)h d d dz x z dx LAI d -∂=⎰⎰(25)Where h is the height of the canopy , d is the distance between two branches, and (,)x z ∂ is the leaf area density function of the micro-body at the point (,)x z . x a is the function of leaf area which means the distribution of cross-section of the X direction, and Its value is a dimensionless, defined as follows: /2/21()d x d a x dx LAI d -=⎰ (26)()()x s a x d LAI C x =⋅⋅ (27)For the canopy which is not uniform in the horizontal direction, the distribution of its leaves is not entirely clear. We use s C to represent the distribution function of thedensity of leaves. s C can be expressed as a quadratic function or a Gaussiandistribution function.4.2.3 Transmission model of the solar radiationAssume that the attenuation of the solar radiation accords with the law of Beer-Lambert. The attenuation value at a point of canopy where the light arrives with a certain angle of incidence and azimuth is proportional to the length of the path, and the length can be calculated by Goudriaan function. Approximate function of the G function is shown as follows [15]:00(12)cos G G k G θ=+- (28)where k is a parameter decided by different plants.Take a micro unit in the canopy . Direct sunlight intercepted in this micro unit can be expressed in the following form:()(,)b b L dI I z G z dLAI =-Θ (29)Where b I means the direct sunlight, Θ is the solar zenith angle and L dL A I is the LAI on the path of light.Figure 4.4 Micro unit of the canopyThrough the integral and the chain rule, we can get the transmittance at the point (',')x z as follows:(,)[()]tan sin ()(',')exp()()h b b z G z a x z a z I x z I h ΘΘ∆Φ+=-⎰ (30)Average direct transmission rate:/2/21(')(',')'d b b d t z t x z dx d --=⎰(31)Different with the direct light, the scattered light in all directions is intercepted by theleaf surface from the upper hemisphere. The irradiance d dI of scattering at the point(',')x z can be expressed as follows:'cos d d b dI i t d ωθ=⋅⋅ (32) where d i is the illumination intensity of scattered light from a given direction.The transmission rate of the sun scattered light is as follows:2/2'00(',')1sin cos ()d b d I x z t d d I h ππθθθϕπ=⎰⎰ (33) Average scattering transmission rate:/2/21(')(',')'d d d d t z t x z dx d --=⎰(34)Global solar radiation reaching the canopy with a given depth z :(')()[(1)(')(')]d b d d I z I h k t z k t z ---=-+ (35)According to eq.(30) and eq.(33), the maximum depth the solar radiation can reach has a major link with h and θ. The shape of leaves have a relationship with photosynthesis. So, the h and θ of branches do have an influence on the leaves. Results showed that the light level and light utilization of high stem and open ce nter shape as well as small and sparse canopy shape were better than others .Double canopy shape ,spindle shape and center shape took second place ,while big canopy shape had the lowest light distribution [16].5. Is leaf shape related to tree structure?Due to internal and external factors, there are many kinds of tree shapes in the nature. For example, the apple's tree shape is semi-ellipsoidal, the willow's is hemispherical, the peach's likes a cup, the pine's likes cone and so on. The shape of their leaves varies. The leaf shape of apple is oval, pine's is needle, and the Indus's is palm. Is leaf shape related to tree shape? Even for the same species, there are many kinds of tree shapes. For instance, Small canopy shape, Open center shape, Freedom spindle shape, high stem and open center shape, Double canopy shape and so on. The sizes of leaves are different. Does the tree profile/branching structure effects the leaf shape?5.1 The experiment for one speciesTo solve this problem, we first consider the relationship of one tree species such as apple, which is semi-ellipsoidal. According to the second question, the hemispherical model is further extended to the semi-ellipsoidal model. Every tree individual shows irregularly because of natural and man-made factors, which reflects on the diversity of the crown in the canopy height direction of the changing relationship between the crown and canopy height. Figure 5.1 shows the relationship.Figure5.1 Schematic diagram of tree crown contourWhere 0h means the truck high, 1h means the crown high, 8710,,,,d d d d ⋅⋅⋅ meanscrown diameter. Tree shape with the scale change can be characterized in fractal dimension. Across the same scales, a fixed fractal dimension indicates the boundary shape's self-similarity; on different scales, the change of fractal dimension means that different processes or limiting factor has superiority. (Wiens, 1989)[17] According to the application of BP network and fractional dimension, we describe the tree shapes.[18]According to Li Guodong and Zhang Junke's work which detected and evaluated in different tree shapes of ‘Fuji’ apple. We choose three kinds of tree shapes to study; they are Small canopy shape, Open center shape and Freedom spindle shape as figure5.2 shows.。
2016-MCM美国大学生数学建模竞赛B题中文
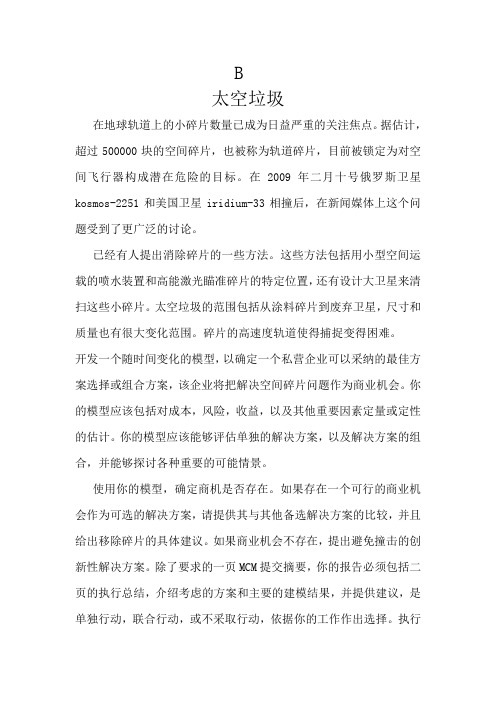
B
太空垃圾
在地球轨道上的小碎片数量已成为日益严重的关注焦点。
据估计,超过500000块的空间碎片,也被称为轨道碎片,目前被锁定为对空间飞行器构成潜在危险的目标。
在2009年二月十号俄罗斯卫星kosmos-2251和美国卫星iridium-33相撞后,在新闻媒体上这个问题受到了更广泛的讨论。
已经有人提出消除碎片的一些方法。
这些方法包括用小型空间运载的喷水装置和高能激光瞄准碎片的特定位置,还有设计大卫星来清扫这些小碎片。
太空垃圾的范围包括从涂料碎片到废弃卫星,尺寸和质量也有很大变化范围。
碎片的高速度轨道使得捕捉变得困难。
开发一个随时间变化的模型,以确定一个私营企业可以采纳的最佳方案选择或组合方案,该企业将把解决空间碎片问题作为商业机会。
你的模型应该包括对成本,风险,收益,以及其他重要因素定量或定性的估计。
你的模型应该能够评估单独的解决方案,以及解决方案的组合,并能够探讨各种重要的可能情景。
使用你的模型,确定商机是否存在。
如果存在一个可行的商业机会作为可选的解决方案,请提供其与其他备选解决方案的比较,并且给出移除碎片的具体建议。
如果商业机会不存在,提出避免撞击的创新性解决方案。
除了要求的一页MCM提交摘要,你的报告必须包括二页的执行总结,介绍考虑的方案和主要的建模结果,并提供建议,是单独行动,联合行动,或不采取行动,依据你的工作作出选择。
执行总结
是为不具备技术背景的高层决策者和新闻媒体分析人士写的。
2012数学建模B题解答过程要点
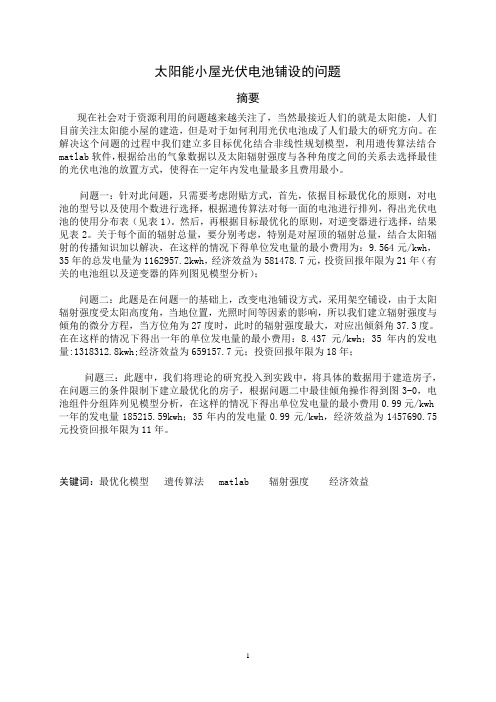
太阳能小屋光伏电池铺设的问题摘要现在社会对于资源利用的问题越来越关注了,当然最接近人们的就是太阳能,人们目前关注太阳能小屋的建造,但是对于如何利用光伏电池成了人们最大的研究方向。
在解决这个问题的过程中我们建立多目标优化结合非线性规划模型,利用遗传算法结合matlab软件,根据给出的气象数据以及太阳辐射强度与各种角度之间的关系去选择最佳的光伏电池的放置方式,使得在一定年内发电量最多且费用最小。
问题一:针对此问题,只需要考虑附贴方式,首先,依据目标最优化的原则,对电池的型号以及使用个数进行选择,根据遗传算法对每一面的电池进行排列,得出光伏电池的使用分布表(见表1)。
然后,再根据目标最优化的原则,对逆变器进行选择,结果见表2。
关于每个面的辐射总量,要分别考虑,特别是对屋顶的辐射总量,结合太阳辐射的传播知识加以解决,在这样的情况下得单位发电量的最小费用为:9.564元/kwh,35年的总发电量为1162957.2kwh,经济效益为581478.7元,投资回报年限为21年(有关的电池组以及逆变器的阵列图见模型分析);问题二:此题是在问题一的基础上,改变电池铺设方式,采用架空铺设,由于太阳辐射强度受太阳高度角,当地位置,光照时间等因素的影响,所以我们建立辐射强度与倾角的微分方程,当方位角为27度时,此时的辐射强度最大,对应出倾斜角37.3度。
在在这样的情况下得出一年的单位发电量的最小费用:8.437元/kwh;35年内的发电量:1318312.8kwh;经济效益为659157.7元;投资回报年限为18年;问题三:此题中,我们将理论的研究投入到实践中,将具体的数据用于建造房子,在问题三的条件限制下建立最优化的房子,根据问题二中最佳倾角操作得到图3-0,电池组件分组阵列见模型分析,在这样的情况下得出单位发电量的最小费用0.99元/kwh 一年的发电量185215.59kwh;35年内的发电量0.99元/kwh,经济效益为1457690.75元投资回报年限为11年。
- 1、下载文档前请自行甄别文档内容的完整性,平台不提供额外的编辑、内容补充、找答案等附加服务。
- 2、"仅部分预览"的文档,不可在线预览部分如存在完整性等问题,可反馈申请退款(可完整预览的文档不适用该条件!)。
- 3、如文档侵犯您的权益,请联系客服反馈,我们会尽快为您处理(人工客服工作时间:9:00-18:30)。
2012年美赛B题题目翻译:到Big Long River(225英里)游玩的游客可以享受那里的风景和振奋人心的急流。
远足者没法到达这条河,唯一去的办法是漂流过去。
这需要几天的露营。
河流旅行始于First Launch,在Final Exit结束,共225英里的顺流。
旅客可以选择依靠船桨来前进的橡皮筏,它的速度是4英里每小时,或者选择8英里每小时的摩托船。
旅行从开始到结束包括大约6到18个晚上的河中的露营。
负责管理这条河的政府部门希望让每次旅行都能尽情享受野外经历,同时能尽量少的与河中其他的船只相遇。
当前,每年经过Big Long河的游客有X组,这些漂流都在一个为期6个月时期内进行,一年中的其他月份非常冷,不会有漂流。
在Big Long上有Y处露营地点,平均分布于河廊。
随着漂流人数的增加,管理者被要求应该允许让更多的船只漂流。
他们要决定如何来安排最优的方案:包括旅行时间(以在河上的夜晚数计算)、选择哪种船(摩托还是桨船),从而能够最好地利用河中的露营地。
换句话说,Big Long River在漂流季节还能增加多少漂流旅行数?管理者希望你能给他们最好的建议,告诉他们如何决定河流的容纳量,记住任两组旅行队都不能同时占据河中的露营地。
此外,在你的摘要表一页,准备一页给管理者的备忘录,用来描述你的关键发现。
沿着大朗河露营摘要我们开发了一个模型来安排沿大河的行程。
我们的目标是为了优化乘船旅行的时间,从而使6个月的旅游旺季出游人数最大化。
我们模拟团体从营地到营地旅行的过程。
根据给定的约束条件,我们的算法输出了每组沿河旅行最佳的日程安排。
通过研究算法的长期反应,我们可以计算出旅行的最大数量,我们定义为河流的承载能力。
我们的算法适应于科罗多拉大峡谷的个案分析,该问题的性质与大长河问题有许多共同之处。
最后,我们考察当改变推进方法,旅程时间分布,河上的露营地数量时承载能力的变化的敏感性。
我们解决了使沿大朗河出游人数最大化的休闲旅行计划。
从首次启动到最终结束(225英里),参与者需使用桨供电的橡胶筏或机动船在指定的参与者露营地游玩6到18个晚上。
为了确保一个真实的荒野体验,一组在同一时间最多占据一个营地。
这个约束限制了公园的6个月的旅游旺季期间可能的旅行数量。
我们模拟情景,然后把我们相似特性的研究结果进行比较,从而验证了我们的方法是否能得到令人满意的结果。
我们的模型是适用于针对有着不同长度的河流、不同数量的露营地、不同的行程持续时间、以及不同的船的速度的情况中,找到最佳的行程安排。
问题重述•应该如何制定不同长度和推进过程的旅行计划,使其在6个月的旅行季中旅行可能数量最大化?•在任何时候,有多少新的组可以开始河上旅行?•什么是河流的承载能力——在六个月的旅行季中可以发送顺流而下的最大数量的组?模型概述我们设计了一个模型,•可以应用到具有相似属性的真实世界的河流(即,大峡谷);•足够灵活,以模拟各种可行的输入参数;•模拟河往返调度的关于旅行分布长度(无论是6,12,或18天)的推进,不同的分布速度和不同数量的露营地的函数。
该模型可以预测出这6个月的旅行季的旅游人数。
它还回答有关河流的承载能力,有利的推进速度和行程长度的分布,每一天可以有多少组开始河流之旅,以及如何安排行程。
约束条件问题指定了以下限制:•旅行在始发点开始并在终点结束,225公里的下游。
•只有两种船:桨供电的橡胶筏和电机化船。
•桨供电的橡皮筏平均每小时旅行4英里。
•电动船平均每小时旅行8英里。
•旅行时间范围是6至18晚。
•旅行安排在一年的6个月期间。
•露营地沿河均匀分布。
•没有两个组可以同时占据相同的营地。
问题假设•我们可以规定每天能到河上航行的桨供电河筏和机帆船的比例。
如果有太多的桨动力船在短时间内出行,有可能会出现问题。
•桨供电筏一趟的时间是12天或18天,机动船的为6天或12天。
这种简化使得我们的模型产生有意义的结果。
同时,让我们比较不同的行程长度的效果。
•每个营地每晚只能有一组。
这符合河川管理者的要求。
•每一天,一组只能向下游移动,或留在其目前的营地——不能向上游航行。
这将流动组限制在了一个单一的方向上,从而极大地简化了我们该如何移动营地与营地间的组。
•旅行组从上午8时至下午6时,每天最多只能航行9小时(减去一个小时休息/午餐/等)。
这意味着,每一天,桨动力筏旅行最多航行36英里,机动船最多72英里。
这种假设使我们可以确定哪些组可以合理地达到某一的营地。
•旅行团每天的航行里程不能超过他们合理的旅行距离:桨动力阀最多36英里每天,机动阀72英里每天。
•我们忽略可能影响最大出行距离的变量,如天气和河流条件。
没有办法将这些变量精确地包括在模型中。
•露营营地之间的距离均匀分布,这样营地间的距离就等于河流的长度除以营地的数量。
因此,我们可以将河流表示为一个等距离分布露营地的数列。
•A组必须在其行程的最后一天到达终点的河流:A组即使可以,也不会提前离开河流。
A组不会超出计划的旅行时间。
我们相信这个假设符合河流管理者以及旅行质量的标准。
模型建立我们定义一些术语和短语:开放的营地(open campsite):如果目前没有旅行团占用,营地是开放的:如果没有组被分配到,营地是开放的。
移动到一个开放的营地(moving to an open campsite):对于一组营地是的组,其移动到其他的开放营地,即,相当于组分配到新营地。
由于旅游团只可以向下游移动,或留在他们目前的营地,我们有。
等待名单(waitlist):某天的等待名单是那些在河上但还没有开始当天的旅行团组成,此时他们在等待名单上的排名和他们到达营地c的能力将他们包含在能够到达营地c的所有组的集合,这些组被视作有着最大的优先权。
等待名单上的组以当前的营地初值为,并且有着之为P=1的优先权知道他们从等待名单上移除,并到河上开始旅行。
离开河流(off the River):我们认为,河上第一个离开的营地是,它始终是一个开放的营地(因此,任何数量的组可以被分配给它。
这符合任何数量的旅行团都可以在任何一天离开河流的理解。
最远的空营地(the Farthest Empty Campsite):我们的调度算法使用一个数组作为数据结构来表示河流,数组的每个元素作为一个营地。
每天以找到在河上最远的开放营地c来开始该算法,然后生成一个集合,其中包含了所有在当晚可能到达c的组。
因此,Gc = {gi | li + mi ≥c},其中是该组的当前位置,是该小组可以在一天之内旅行的最大距离。
• 限定了组必须能够在一天之内到达营地ç。
• 是有在河上以及等待名单上的组构成。
•如果,那么我们可以移动到下一个最远的空营地——位于上流,并更接近于河流的起点。
该算法总是从河流的末端向河流的始端运行。
•如果,则算法试图将具有最高优先级的组移动到营地C。
该调度算法一直执行到最远的空营地是为止。
此时,每个能在河上继续航行的组被分配到一个营地,然后我们开始另一个算法来模拟第二天。
优先级:一旦集合已形成为特定的露营地Ç,算法必须决定哪个组移动到该营地。
优先级是一个衡量组落后或提前于计划的程度的量:衡量多远的前面或后面的计划组gi是:•>1:组进度落后;•<1:组提前;•=1:组恰恰是按计划进行。
我们尽量用最高的优先级别将组移动到c。
具体的例子,以及如何用优先级解决这些问题,在图1和图2中概述了。
优先顺序和其他注意事项:我们的算法总是试图移动落后于计划最多的组,来以确保每组都能在河上扎营,图1调度算法发现,最远的开放营地是营地6,组A,B,C可能达到。
B组具有最高优先级,所以我们移动B组到营地6。
图2由于调度算法的过程经过了营地6,它发现下一个最远开放式营地是营地5。
该算法得出A组和C能到达营地5;由于PA> PC,A组搬到营地5。
并且扎营的夜数就等于之前确定的旅行长度。
然而,在某些情况下,它可能无法以最高的优先级将组移到最远的可用的开放营地。
这种情况下,如果具有最高优先级的是提前与计划的(P<1)。
我们提供以下规则处理组优先级:•如果是落后进度,即>1,那么移动到c——其最远可达的开放营地。
•如果提前,即<1,然后计算——该组在河上已经度过的夜数乘以每天计划行进的平均距离。
如果结果是大于或等于(以英里为单位)的露营地c的位置,那么移动到c。
这样做可以让不再提前于计划。
•不管,如果选择,那么不移动,除非。
此功能可确保,的行程不会在其计划结束日期前结束。
在图3中示出了一个组的优先级被忽略的情况。
调度仿真:现在我们证明我们的模型可以用来安排河流上的旅行次数。
在下面的例子中,我们假设沿225英里的河流有50个露营地,我们设定每天河上有四个组。
我们为图3 最远的开放营地不在河上。
该算法找到,组D可以移动到那里,但D组有,即组D计划在河上待12晚,但到目前为止,只待了11晚——所以D组仍然在河上,在营地171和224(含)之间。
我们引进的四个特定组做了一个25天的行程计划。
我们选择了旺季中的一天,以证明我们的模型的时间稳定性。
四组的特性如下:•:机动,=6; •:桨供电,=18; •:机动,=12; •:桨供电,=12。
案例:(大峡谷)大峡谷是一个针对我们模型研究的理想情况,因为它和大朗河的许多特征相同。
峡谷的主要河流长为226英里,它拥有235个露营地,它在一年里大约有六个月都开放。
它可以让游客乘坐机动船或桨供电河筏分别最多航行12天或18天。
使用的大峡谷参数中,我们模拟了多次来测试我们的模型。
我们改变每天在河上的组的数量,想要得出河流的承载量——图5例如组推出的第25天时间表。
图6在图5的基础上顺流而下的组的运动。
组由不同的行程持续时间参数在不同的时间到达终点。
图7每组行进过程的优先级值。
由于算法为了保持组群按时进行,值收敛到P=1。
长达六个月的可能的最大旅行数量。
主要的制约因素是,每趟必须持续组的计划旅程时间。
在夏季,大峡谷一般会将新的六组置于水上[贾等人的水。
2006 ],所以我们使用这个值为我们的第一次模拟。
在每一次模拟中,我们使用同等数量的机帆船,桨动力筏,以及相等行程长度的分配。
我们的模型预测出成功离开河的组(已完成人数)的数量,以及超过他们预定的截止日期(逾期人数)的人的数量,以及没有离开候补名单的人的数量(候补名单上的总人数)。
这些值随着我们改变分配到水上的新团队的数量而变化(团队/天)。
表2每组每天的模拟结果仿真组/天模拟数每组每天载人数完成人数逾期人数等待人数表1表明了18个组中每天可分配到河上的最大人数。
在过去的六个月中,乘船人数近3000人次。
增加至18组/天以上,很可能导致迟到人次(我们的模拟结束时,一些团体仍然在河上)和长候补名单。