Providing Absolute Differentiated Services for Real-Time Applications in Static-Priority Sc
如何设置网络QoS:提升网络带宽利用率(六)
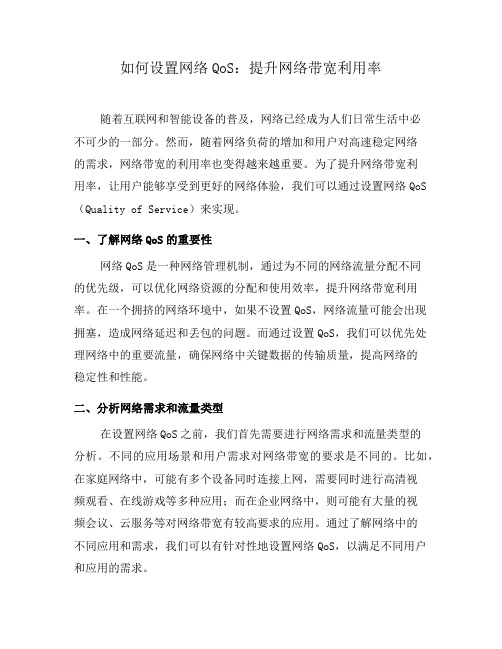
如何设置网络QoS:提升网络带宽利用率随着互联网和智能设备的普及,网络已经成为人们日常生活中必不可少的一部分。
然而,随着网络负荷的增加和用户对高速稳定网络的需求,网络带宽的利用率也变得越来越重要。
为了提升网络带宽利用率,让用户能够享受到更好的网络体验,我们可以通过设置网络QoS (Quality of Service)来实现。
一、了解网络QoS的重要性网络QoS是一种网络管理机制,通过为不同的网络流量分配不同的优先级,可以优化网络资源的分配和使用效率,提升网络带宽利用率。
在一个拥挤的网络环境中,如果不设置QoS,网络流量可能会出现拥塞,造成网络延迟和丢包的问题。
而通过设置QoS,我们可以优先处理网络中的重要流量,确保网络中关键数据的传输质量,提高网络的稳定性和性能。
二、分析网络需求和流量类型在设置网络QoS之前,我们首先需要进行网络需求和流量类型的分析。
不同的应用场景和用户需求对网络带宽的要求是不同的。
比如,在家庭网络中,可能有多个设备同时连接上网,需要同时进行高清视频观看、在线游戏等多种应用;而在企业网络中,则可能有大量的视频会议、云服务等对网络带宽有较高要求的应用。
通过了解网络中的不同应用和需求,我们可以有针对性地设置网络QoS,以满足不同用户和应用的需求。
三、设置不同的QoS策略根据网络需求和流量类型的分析结果,我们可以制定不同的QoS策略。
一般来说,网络中的数据流量可以分为三类:实时流量、敏感流量和普通流量。
实时流量包括视频通话、语音通话等需要即时传输的数据;敏感流量包括在线游戏、即时通信等对网络延迟要求较高的数据;而普通流量则包括普通网页浏览、电子邮件等对网络要求不高的数据。
我们可以根据不同流量的特点,设置不同的QoS策略。
对于实时流量和敏感流量,我们可以优先处理其数据包,确保其低延迟的传输。
可以通过设置优先级队列来确保这些流量的传输优先级高于其他流量。
而对于普通流量,则可以设置合适的带宽控制策略,根据网络带宽的实际情况和流量的需求来分配带宽,避免网络拥塞和带宽资源的浪费。
Qos
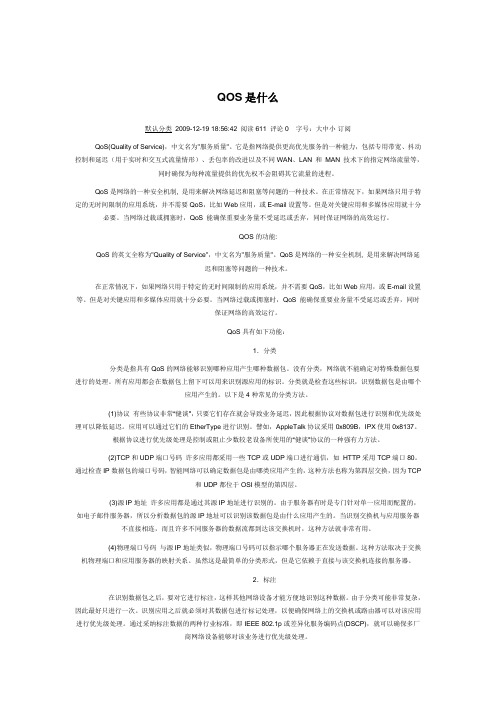
QOS是什么默认分类2009-12-19 18:56:42 阅读611 评论0 字号:大中小订阅QoS(Quality of Service),中文名为"服务质量"。
它是指网络提供更高优先服务的一种能力,包括专用带宽、抖动控制和延迟(用于实时和交互式流量情形)、丢包率的改进以及不同WAN、LAN 和MAN 技术下的指定网络流量等,同时确保为每种流量提供的优先权不会阻碍其它流量的进程。
QoS是网络的一种安全机制, 是用来解决网络延迟和阻塞等问题的一种技术。
在正常情况下,如果网络只用于特定的无时间限制的应用系统,并不需要QoS,比如Web应用,或E-mail设置等。
但是对关键应用和多媒体应用就十分必要。
当网络过载或拥塞时,QoS 能确保重要业务量不受延迟或丢弃,同时保证网络的高效运行。
QOS的功能:QoS的英文全称为"Quality of Service",中文名为"服务质量"。
QoS是网络的一种安全机制, 是用来解决网络延迟和阻塞等问题的一种技术。
在正常情况下,如果网络只用于特定的无时间限制的应用系统,并不需要QoS,比如Web应用,或E-mail设置等。
但是对关键应用和多媒体应用就十分必要。
当网络过载或拥塞时,QoS 能确保重要业务量不受延迟或丢弃,同时保证网络的高效运行。
QoS具有如下功能:1.分类分类是指具有QoS的网络能够识别哪种应用产生哪种数据包。
没有分类,网络就不能确定对特殊数据包要进行的处理。
所有应用都会在数据包上留下可以用来识别源应用的标识。
分类就是检查这些标识,识别数据包是由哪个应用产生的。
以下是4种常见的分类方法。
(1)协议有些协议非常“健谈”,只要它们存在就会导致业务延迟,因此根据协议对数据包进行识别和优先级处理可以降低延迟。
应用可以通过它们的EtherType进行识别。
譬如,AppleTalk协议采用0x809B,IPX使用0x8137。
qos处理流程

qos处理流程QoS(Quality of Service,服务质量)是一种网络技术,用于在网络中为不同类型的数据流提供不同的优先级和带宽分配,以保证网络的性能和服务质量。
QoS处理流程是指在网络中实现QoS的具体步骤和过程。
本文将从QoS处理流程的角度,介绍QoS的工作原理、常见的QoS技术和实施步骤。
一、QoS的工作原理QoS的工作原理是根据网络中各种数据流的特点和要求,对网络资源进行合理的调度和分配,以确保不同类型的数据流在网络中能够得到满足的服务质量。
QoS的核心目标是实现低延迟、高带宽、低丢包率和低抖动的网络传输。
QoS的工作原理主要包括以下几个方面:1. 流量分类:将网络中的数据流划分为不同的类别,如实时流、敏感流和普通流等,根据不同类型的数据流的特点和要求进行分类。
2. 流量标记:对不同类型的数据流进行标记,以便在后续的处理中根据标记对数据流进行不同的处理。
常见的标记方式包括802.1p和DiffServ等。
3. 流量调度:根据流量分类和标记的结果,对不同类型的数据流进行合理的调度和分配。
通过使用调度算法和队列管理策略,为不同类型的数据流提供优先级和带宽保障。
4. 流量控制:通过对数据流的速率进行限制和调整,控制网络中的流量,避免网络拥塞和资源浪费。
常用的流量控制技术包括令牌桶和漏桶算法等。
5. 流量保护:为关键数据流提供保护机制,防止其受到非关键数据流的影响。
常见的保护机制包括优先级队列和带宽保障等。
二、常见的QoS技术1. DiffServ(Differentiated Services):DiffServ是一种基于流量分类和标记的QoS技术,通过对数据流进行分类和标记,实现对不同类型的数据流进行不同的处理和调度。
2. RSVP(Resource Reservation Protocol):RSVP是一种用于实现网络资源预留的QoS技术,通过建立预留路径和预留资源,为特定的数据流提供优先级和带宽保障。
如何设置网络QoS:提升网络带宽利用率(二)
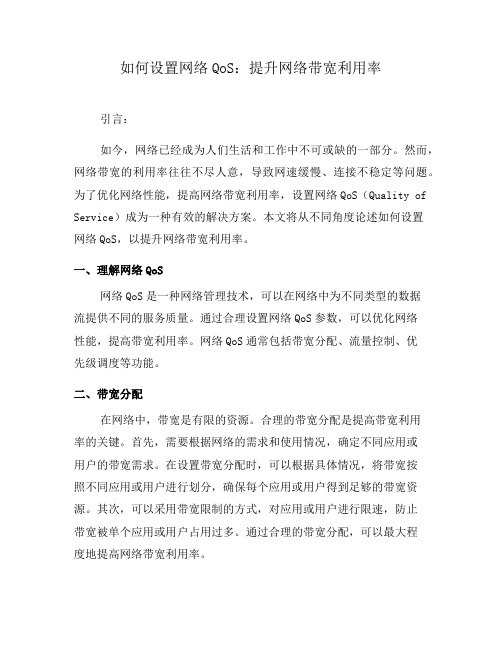
如何设置网络QoS:提升网络带宽利用率引言:如今,网络已经成为人们生活和工作中不可或缺的一部分。
然而,网络带宽的利用率往往不尽人意,导致网速缓慢、连接不稳定等问题。
为了优化网络性能,提高网络带宽利用率,设置网络QoS(Quality of Service)成为一种有效的解决方案。
本文将从不同角度论述如何设置网络QoS,以提升网络带宽利用率。
一、理解网络QoS网络QoS是一种网络管理技术,可以在网络中为不同类型的数据流提供不同的服务质量。
通过合理设置网络QoS参数,可以优化网络性能,提高带宽利用率。
网络QoS通常包括带宽分配、流量控制、优先级调度等功能。
二、带宽分配在网络中,带宽是有限的资源。
合理的带宽分配是提高带宽利用率的关键。
首先,需要根据网络的需求和使用情况,确定不同应用或用户的带宽需求。
在设置带宽分配时,可以根据具体情况,将带宽按照不同应用或用户进行划分,确保每个应用或用户得到足够的带宽资源。
其次,可以采用带宽限制的方式,对应用或用户进行限速,防止带宽被单个应用或用户占用过多。
通过合理的带宽分配,可以最大程度地提高网络带宽利用率。
三、流量控制流量控制是一种有效的网络QoS技术,可以避免过多的数据流拥塞网络,导致带宽浪费。
一种常见的流量控制方式是使用队列管理算法,如先进先出(FIFO)或最小二乘尺寸(MBS)等。
这些算法可以根据不同数据流的优先级和需求,按照一定的规则进行流量管理,确保网络中的数据传输稳定流畅。
此外,也可以利用流量控制技术在网络边界进行流量过滤和限制,减少无关流量的传输,提高带宽利用率。
四、优先级调度在网络中,不同类型的数据流有不同的优先级和重要性。
通过设置优先级调度,可以确保重要数据在带宽有限的情况下优先传输,提高网络的服务质量和带宽利用率。
一种常见的优先级调度方式是使用DiffServ(Differentiated Services)模型。
该模型将数据流划分为多个类别,每个类别具有不同的优先级,并为其分配相应的带宽资源。
QoS配置
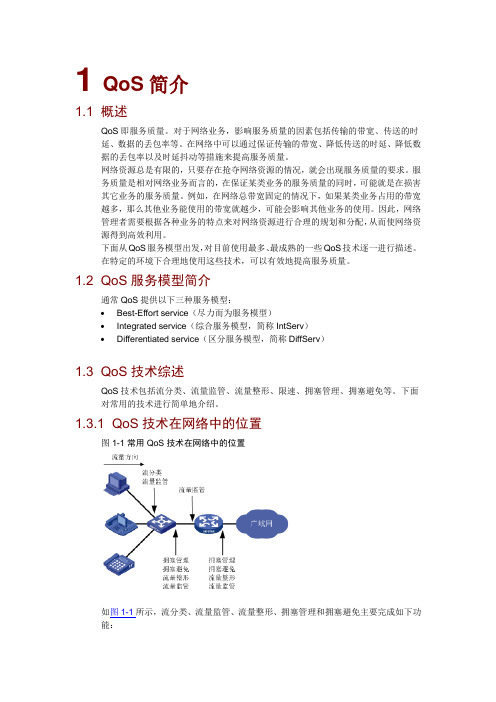
1 QoS简介1.1 概述QoS即服务质量。
对于网络业务,影响服务质量的因素包括传输的带宽、传送的时延、数据的丢包率等。
在网络中可以通过保证传输的带宽、降低传送的时延、降低数据的丢包率以及时延抖动等措施来提高服务质量。
网络资源总是有限的,只要存在抢夺网络资源的情况,就会出现服务质量的要求。
服务质量是相对网络业务而言的,在保证某类业务的服务质量的同时,可能就是在损害其它业务的服务质量。
例如,在网络总带宽固定的情况下,如果某类业务占用的带宽越多,那么其他业务能使用的带宽就越少,可能会影响其他业务的使用。
因此,网络管理者需要根据各种业务的特点来对网络资源进行合理的规划和分配,从而使网络资源得到高效利用。
下面从QoS服务模型出发,对目前使用最多、最成熟的一些QoS技术逐一进行描述。
在特定的环境下合理地使用这些技术,可以有效地提高服务质量。
1.2 QoS服务模型简介通常QoS提供以下三种服务模型:∙ Best-Effort service(尽力而为服务模型)∙ Integrated service(综合服务模型,简称IntServ)∙ Differentiated service(区分服务模型,简称DiffServ)1.3 QoS技术综述QoS技术包括流分类、流量监管、流量整形、限速、拥塞管理、拥塞避免等。
下面对常用的技术进行简单地介绍。
1.3.1 QoS技术在网络中的位置图1-1 常用QoS技术在网络中的位置如图1-1所示,流分类、流量监管、流量整形、拥塞管理和拥塞避免主要完成如下功能:∙流分类:采用一定的规则识别符合某类特征的报文,它是对网络业务进行区分服务的前提和基础。
∙流量监管:对进入或流出设备的特定流量进行监管,以保护网络资源不受损害。
可以作用在接口入方向和出方向。
∙流量整形:一种主动调整流的输出速率的流量控制措施,用来使流量适配下游设备可供给的网络资源,避免不必要的报文丢弃,通常作用在接口出方向。
计算机网络中的QoS保证方法及技术挑战
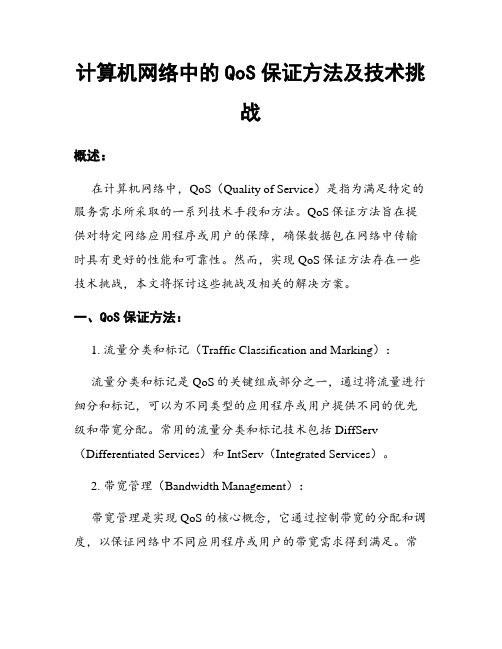
计算机网络中的QoS保证方法及技术挑战概述:在计算机网络中,QoS(Quality of Service)是指为满足特定的服务需求所采取的一系列技术手段和方法。
QoS保证方法旨在提供对特定网络应用程序或用户的保障,确保数据包在网络中传输时具有更好的性能和可靠性。
然而,实现QoS保证方法存在一些技术挑战,本文将探讨这些挑战及相关的解决方案。
一、QoS保证方法:1. 流量分类和标记(Traffic Classification and Marking):流量分类和标记是QoS的关键组成部分之一,通过将流量进行细分和标记,可以为不同类型的应用程序或用户提供不同的优先级和带宽分配。
常用的流量分类和标记技术包括DiffServ (Differentiated Services)和IntServ(Integrated Services)。
2. 带宽管理(Bandwidth Management):带宽管理是实现QoS的核心概念,它通过控制带宽的分配和调度,以保证网络中不同应用程序或用户的带宽需求得到满足。
常见的带宽管理技术包括流量调度算法、公平队列调度算法(Fair Queuing)和权重公平队列调度算法(Weighted Fair Queuing)等。
3. 拥塞控制(Congestion Control):拥塞控制是保证网络中数据传输性能的关键技术之一。
通过动态监测网络状态和流量负载情况,拥塞控制机制可以在网络拥塞前提前发现并采取相应的措施,如降低传输速率或丢弃数据包,以防止拥塞的发生。
常用的拥塞控制技术包括TCP拥塞控制机制和主动队列管理算法(Active Queue Management)。
4. 延迟和丢包控制(Delay and Loss Control):对于某些网络应用程序,如实时音视频传输、在线游戏等,延迟和丢包是非常敏感的因素。
QoS保证方法需要通过适当的技术手段来控制网络中的延迟和丢包,以提供较低的时延和更可靠的传输。
CISCO交换机基本配置和使用概述

CISCO交换机基本配置和使用概述CISCO交换机是一种常用的网络设备,用于构建局域网(Local Area Network,LAN)。
它可以通过物理线路的连接,将多台计算机或其他网络设备连接到同一个网络中,实现数据的传输和共享。
CISCO交换机的基本配置包括IP地址的配置、VLAN的配置、端口配置、安全性配置等。
接下来,我们将对这些配置进行详细说明。
首先,IP地址的配置是CISCO交换机的基本操作之一。
通过配置IP地址,我们可以对交换机进行管理和监控。
具体的配置步骤如下:1. 进入交换机的配置模式。
在命令行界面输入"enable"命令,进入特权模式。
2. 进入全局配置模式。
在特权模式下输入"configure terminal"命令,进入全局配置模式。
3. 配置交换机的IP地址。
在全局配置模式下输入"interfacevlan 1"命令,进入虚拟局域网1的接口配置模式。
然后输入"ip address 192.168.1.1 255.255.255.0"命令,配置交换机的IP地址和子网掩码。
4. 保存配置并退出。
在接口配置模式下输入"exit"命令,返回到全局配置模式。
然后输入"exit"命令,返回到特权模式。
最后输入"copy running-config startup-config"命令,保存配置到闪存中。
其次,VLAN的配置是CISCO交换机的关键配置之一。
通过配置VLAN,我们可以将交换机的端口划分为不同的虚拟局域网,实现数据的隔离和安全。
1. 进入交换机的配置模式。
同样,在特权模式下输入"configure terminal"命令,进入全局配置模式。
2. 创建VLAN。
在全局配置模式下输入"vlan 10"命令,创建一个编号为10的VLAN。
移动通信中的QoS解析

移动通信中的QoS解析移动通信中的QoS(Quality of Service)是指在移动网络中对通信服务质量进行度量和管理的一种方式。
QoS是移动通信领域中的一个重要概念,它直接影响着用户体验、网络性能和网络资源的利用效率。
本文将对移动通信中的QoS进行解析,包括QoS的定义、分类、度量方法以及在移动通信系统中的应用。
1. QoS的定义QoS是指在移动通信网络中,通过对数据传输延迟、丢包率、带宽等参数进行度量和控制,来保证通信服务质量的一种机制。
它是指网络能够为特定的服务类型提供有限的资源,并满足一定的性能需求,如延迟、带宽、可靠性等。
2. QoS的分类QoS可以分为两种类型:差别化服务(Differentiated Services)和综合服务(Integrated Services)。
差别化服务是指通过对数据流进行分类、标记和排队,为不同的数据流提供不同的服务质量。
常用的差别化服务标记包括DiffServ(Differentiated Services Point)和MPLS (Multiprotocol Label Switching)。
综合服务是指为每一个数据流预留一定的资源,并通过嵌入式协议进行控制和管理,以实现对报文的优先级处理和拥塞控制。
3. QoS的度量方法在移动通信中,常用的QoS度量方法有以下几种:数据传输延迟(Delay):指数据包从发送端到接收端的传输时间。
数据传输速率(Throughput):指单位时间内传输的数据量。
丢包率(Packet Loss Rate):指传输过程中丢失的数据包占总发送数据包的比例。
抖动(Jitter):指数据包在传输过程中的时延变化量。
这些度量方法可以通过网络测量工具进行测试和分析,并用来评估和监控QoS的表现。
4. QoS的应用QoS在移动通信系统中有广泛的应用,例如:语音通信:通过提供低丢包率和低延迟的服务,保证方式通话的质量。
视频流媒体:通过提供足够的带宽和低延迟的服务,保证视频流的流畅播放。
- 1、下载文档前请自行甄别文档内容的完整性,平台不提供额外的编辑、内容补充、找答案等附加服务。
- 2、"仅部分预览"的文档,不可在线预览部分如存在完整性等问题,可反馈申请退款(可完整预览的文档不适用该条件!)。
- 3、如文档侵犯您的权益,请联系客服反馈,我们会尽快为您处理(人工客服工作时间:9:00-18:30)。
Providing Absolute Differentiated Services for Real-Time Applications in Static-PriorityScheduling NetworksShengquan Wang,Dong Xuan,Riccardo Bettati,and Wei ZhaoAbstract—In this paper,we propose and analyze a methodology for providing absolute differentiated services for real-time applications in net-works that use static-priority schedulers.We extend previous work on worst-case delay analysis and develop a method that can be used to derive delay bounds without specific information onflow population.With this new method,we are able to successfully employ a utilization-based admis-sion control approach forflow admission.This approach does not require explicit delay computation at admission time and hence is scalable to large systems.We assume the underlying network to use static-priority sched-ulers.We design and analyze several priority assignment algorithms,and investigate their ability to achieve higher utilization bounds.Traditionally, schedulers in differentiated services networks assign priorities on a class-by-class basis,with the same priority for each class on each router.In this paper,we show that relaxing this requirement,that is,allowing different routers to assign different priorities to classes,achieves significantly higher utilization bounds.Keywords—absolute differentiated services,static-priority scheduling, utilization-based admission control,priority assignment,delay bound.I.I NTRODUCTIONHE differentiated service(DiffServ)Internet model is aimed at supporting service differentiation for aggregated traffic in a scalable manner.Many approaches have been pro-posed to realize this model.At one end of the spectrum, absolute differentiated services[16],[17],[19]seek to pro-vide IntServ-type end-to-end absolute performance guaran-tees without per-flow state in the network core.The user re-ceives an absolute service profile(e.g.,guarantees on band-width,or end-to-end delay).For example,assuming that no dynamic routing occurs,the premium service can offer the user a performance level that is similar to that of a leased line,as long as the user’s traffic is limited to a given bandwidth[16]. At the other end of spectrum,relative differentiated services seek to provide per-hop,per-class relative services[9].Con-sequently,the network cannot provide end-to-end guarantees. Instead,each router only guarantees that the service invariant is locally maintained,even though the absolute end-to-end service might vary with networking conditions.Many real-time applications,such as,V oice over IP,DoD’s C4I,or industrial control systems,demand efficient and effec-tive communication services.In this context,by real-time we mean that a packet is delivered from its source to the destination within a predefined end-to-end deadline.Packets delivered be-yond these end-to-end deadlines are considered useless.Within a differentiated-services framework,real-time applications must rely on absolute differentiated services in order to have a guar-antee on the end-to-end delay.Consequently,in this paper,we The authors are with the Department of Computer Science,Texas A&M University,College Station,TX77843.E-mail:swang,dxuan,bettati, zhao@.will focus on a quality-of-service(QoS)architecture that pro-vides end-to-end absolute differentiated services.Progress has been made to provide absolute differentiated services for real-time applications in networks with rate-based scheduling algorithms[19].In this paper,we consider networks that use static-priority schedulers.This type of scheduler is sup-ported in many current routers,and our approaches can therefore be easily realized within existing networks.In order to provide service guarantees,an admission control mechanism has to be in place,which makes sure that enough sources are available to satisfy the requirements of both the new and the existing connections after the new connection has been admitted.In order to keep steps with the scalability require-ments for differentiated services networks,any admission con-trol mechanism must be light-weight so that it can be realized in a scalable fashion.We show how,through appropriate system (re-)configuration steps,the delay guarantee test at run time is reduced to a simple utilization-based test:As long as the utiliza-tion of links along the path of aflow is not beyond a given bound, the performance guarantee can be met.The value of the utiliza-tion bound is verified at system(re-)configuration time.Once verified,the use of this utilization bound is relatively simple at flow admission time:Upon the arrival of aflow establishment request,the admission control admits theflow if the utilization values of links along the path of the newflow are no more than the bound.Thus,this approach(called Utilization-Based Ad-mission Control–UBAC–in the following)eliminates explicit delay analysis at admission time,and renders the system scal-able.Utilization-based admission control is not new to networks. Thefluid-flow model in the IntServ framework,for exam-ple,allows various forms of utilization based admission control [18].Such approaches cannot be used in a DiffServ frame-work,however,because they rely on guaranteed-rate schedulers, which need to maintainflow information.The challenge of us-ing the UBAC method is how to verify the correctness of a uti-lization bound at the configuration time.Obviously,the verifica-tion will have to rely on a delay analysis method.We will follow the approach proposed by Cruz[6]for analyzing delays.Cruz’s approach must be adapted to be applicable in aflow-unaware environment.The delay analysis proposed in[6]depends on the information aboutflow population,i.e.,the number offlows at input links and the traffic characteristics(e.g.,the average rate and burst size)offlows.In our case the delay analysis is done at system configuration time when the information aboutflow population is not available.We will develop a method that al-lows us to analyze delays without depending on the dynamicinformation aboutflows.Priority Assignment is an inherent issue in the networks with static priority scheduling.As priority assignment has direct im-pact on the delay performance of individual packets,it must be carefully addressed.In the DiffServ domain,applications are differentiated by their classes.Accordingly,many previ-ous studies assume that priorities are assigned on a class ba-sis only,where all theflows in a class are assigned the same priority[4].We study more generalized priority assignment al-gorithms,where theflows in a class may be assigned different priorities andflows from different classes may share a same pri-ority.While the proposed algorithms are still relatively simple and efficient,wefind that they are effective in achieving higher utilization bounds.The rest of the paper is organized as follows.In Section II we describe previous related work.The underlying network and traffic models for this study are introduced in Section III.In Sec-tion IV,we introduce our proposed architecture for providing absolute differentiated services in networks with static-priority scheduling.In Section V,we derive a delay computation for-mula that is insensitive to theflow population.In Section VI, we discuss our heuristic algorithms for priority assignment.In Section VII,we illustrate with extensive experimental data that the utilization achieved by our new algorithms is much higher than traditional methods.A summary of this paper and motiva-tion of future work are given in Section VIII.II.P REVIOUS W ORKSA good survey on recent work in absolute differentiated ser-vices and relative differentiated services has been done in[17]. Here,we compare our work with others from the view point of providing absolute differentiated services.Nichols et.al.[16] propose the premium service model,which provides the equiv-alent of a dedicated link between two access routers.It pro-vides absolute differentiated services in priority-driven schedul-ing networks with two priorities,in which the high priority is re-served for premium service.The algorithm in[7]provides both guaranteed and statistical rate and delay bounds,and addresses scalability through traffic aggregation and statistical multiplex-ing.Stoica and Zhang describe an architecture to provide guar-anteed service without per-flow state management by using a technique called dynamic packet state(DPS)[19].Our work is based on static priority scheduling algorithm,which is relatively simple and widely supported.Admission control has been investigated widely[8],[10], [15].The various approaches differ from each other in that they may require different scheduling schemes and so can be of vastly different complexity.For example,traditional admission control in networks with static priority scheduling is very com-plicated.Due to absence offlow separation,for any newflow re-quest,admission control needs to explicitly compute and verify delays for the new and existingflows.This procedure is very ex-pensive with increasing numbers offlows.The utilization-based admission control adopted dramatically reduces this complexity. In its basic form,UBAC wasfirst proposed in[14]for pre-emptive scheduling of periodic tasks on a simple processor.A number of utilization-based tests are known for centralized sys-tems(e.g.,69%and100%utilization bounds for periodic tasks on a single server using rate-monotonic and earliest-deadline-first scheduling,respectively[14]),or distributed systems(such as33for synchronous traffic over FDDI networks[23]).In this paper,we adopt utilization-based tests in providing differ-entiated services in static priority scheduling networks.Flow-population-insensitive delay analysis has been recently studied in[3]for the case of aggregate scheduling.Lower bounds on the worst-case delay are derived.These bounds are a function of network utilization,maximum hop count of any flow,and the shaping parameters at the entrance to the network. The work in[3]only considers FIFO scheduling.Also,delay bounds are not tight,although almost independent of the net-work topology.In this paper,we will derive a better delay bound in static-priority scheduling networks.This paper focuses on priority assignment in static priority scheduling networks for real-time communication applications within DiffServ domains.In[6],Cruz proposed a two-priority assignment scheme for a ring network.The work in [13]described and examined various priority assignment meth-ods for ATM networks.Our work is the veryfirst on priority assignment for absolute differentiated services.III.N ETWORK AND T RAFFIC M ODELSIn this section,we describe the model and define the termi-nology that will be used in the rest of this paper.work ModelThe DiffServ architecture distinguishes two types of routers:Edge routers are located at the boundary of the net-work,and provide support for traffic policing.Core routers are inside the network.A router is connected to other routers or hosts through its input and output links.For the purpose of de-lay computation,we follow standard practice and model a router as a set of servers,one for each router component,where packets can experience delays.Packets are typically queued at the out-put buffers,where they compete for the output link.We there-fore model a router as a set of output link servers.All other servers(input buffers,non-blocking switch fabric,wires,etc.) can be eliminated from the delay analysis by appropriately sub-tracting constant delays incurred on them from the deadline re-quirements of the traffic.We assume there are input links for Server,and all output links are of capacity,in bits per second.Consequently,the network can be modeled as a graph with connected link servers.The link servers in the server graph are connected through either links in the network or paths within routers,which both make up the set of edges in the graph.B.Traffic ModelWe call a stream of packets between a sender and receiver aflow.Packets of aflow are transmitted along a single path, which we model as a sequence of link servers.Following the DiffServ architecture,flows are partitioned into classes.QoS requirements and traffic specifications offlows are defined on a class-by-class basis.We use to denote the total number of classes in a network.We assume that at each link server,a cer-tain portion of bandwidth is reserved for each traffic class sep-arately.Let denote the portion of bandwidth reserved for Class at Server.We assume static-priority schedulers withsupport for distinct priorities in the routers.The bandwidth assigned to Class at Server is further partitioned into por-tions,one for each priority traffic of Class at that server. We note that(the question of how much band-width to assign to each priority will be discussed in Section VI.). We aggregateflows into group offlows.Allflows of Class with priority going through Server from input link form a group offlows and allflows of all classes with priority going through Server from input link form a group offlows. In order to appropriately characterize traffic both at the ingress router and within the network,we use a general traffic descriptor in form of traffic functions and their time independent counterpart,constraint traffic functions[6].Definition1:The traffic function is defined as the amount of the traffic in a group offlows during time interval.The function is called the traffic constraint function of if(1) for any and.In this paper,we use and to express the traffic constraint function for group offlowsand group offlows respectively.We assume that the source traffic of aflow in Class is con-trolled by a leaky bucket with burst size and average rate. Define as the source traffic function for any class-traffic flow,which is constrained at the entrance to the network by(2) Since the QoS requirements offlows(in our case,end-to-end delay requirements)are specified on a class-by-class basis as well,we can,where we define the end-to-end deadline require-ment of class-traffic to be and use a triple to represent class-traffic.As no distinction is made betweenflows belonging to the same class,allflows in the same class are guar-anteed the same delay.In the following,we use to denote the local worst-case delay suffered byflows with priority at Server.IV.A Q O S A RCHITECTURE FOR A BSOLUTED IFFERENTIATED S ERVICESIn this section,we propose an architecture to provide ab-solute differentiated services in static priority scheduling net-works.This architecture consists of three major modules: Utilization bound verification:In order to allow for a utilization-based admission control to be used at run time,safe utilization levels on all links must be determined during system confiing aflow-population-insensitive delay com-putation method,a delay upper bound is determined for each priority traffic at each router.This module then verifies whether the end-to-end delay bound in each feasible path of the network satisfies the deadline requirement,as long as the bandwidth us-age on the path is within a pre-defined limit–the utilization bound.This is also the point when priorities are assigned within classes and when bandwidth is assigned to classes and to prior-ities.We will discuss bandwidth and priority assignment algo-rithm later.Utilization-based admission control:Once safe utilization levels have been verified at configuration time,the admission control only needs to check if the necessary bandwidth is avail-able along the path of the newflow.Packet forwarding:In a router,packets are transmitted ac-cording to their priorities,which can be derived from the(pos-sibly extended)class identifier in the header.Within the same priority,packets are served in FIFO order.While utilization-based admission control significantly re-duces the admission control overhead compared to traditional approaches that require explicit delay computation,excessive connection establishment activity can still add substantial strain to the admission control components.In[5]we describe ways to distribute the load for admission control by appropriately pre-allocating resources and give the control to ingress nodes to the domain.In the rest of this paper,we will focus onflow-population-insensitive delay computation analysis and on priority assign-ment.V.F LOW-P OPULATION-I NSENSITIVE D ELAYC OMPUTATIONIn this section,we will present a new delay computation for-mula,which is insensitive toflow population.We then discuss the approach with which this delay formula is derived.A.Main ResultSince static priority scheduling does not provideflow sepa-ration,the local delay at a server depends on detailed informa-tion(number and traffic characteristics)of otherflows both at the server under consideration and at servers upstream.There-fore,all theflows currently established in the network must be known in order to compute delays.Delay formulas for this type of systems have been derived for a variety of scheduling algo-rithms[13].While such formulas could be used(at quite some expense)forflow establishment at system run time,they are not applicable for delay computation during configuration time,as they rely on information aboutflow population.As this information is not available at configuration time,the worst-case delays must be determined assuming a worst-case combination offlows.Fortunately,the following theorem gives an upper bound on this worst-case delay without having to ex-haustively enumerate all theflow combinations.Theorem1:The worst-case queuing delay suffered by traffic with priority at Server is bounded by1where(5)(6) for,and(7)is the set of all paths passed by the packets of Class with priority before arriving at Server,then is the maximumof the worst-case delays experienced by allflows of Class withpriority before arriving at Server.is the available bandwidth for traffic with priority no higher than.Derivation of Inequality(3)will be discussed in Subsection V-B.At this point we would like to make the following observa-tions on Theorem1:In a previously derived delay computation formula in[4], there was an implicit limitation on the relationship of traffic classes and priorities:One traffic class can only have a sin-gle priority,and one priority can only be assigned to a single class traffic.The new delay formula removes this limitation,and gives moreflexibility when differentiating service.Our priority assignment algorithms will take advantage of thisflexibility to better utilize available resource.Usually a delay computation formula for a server would de-pend on the state of the server,i.e.,the number offlows that are admitted and pass through the server.We note that Inequality(3) is independent from this kind of information and just depends on ,,,and.The values of these parameters are avail-able at the time when the system is(re-)configured.Hence,the delay computation formula is insensitive to theflow population information.We define.We note that in Inequality(3)depends on.The value of,in turn,depends on the delays experienced at servers other than Server.In general,we have a circular dependency. Hence,the delay values depend on each other and must be com-puted simultaneously.We use the()-dimensional vector to denote the upper bounds of the delays suffered by the traffic with all priorities at all servers:(8) Define the right hand side of(3)as,and then define(9)The queuing delay bound vector can then be determined by iteratively solving the following vector equation:(10) In some special cases,closed-form solutions for the delay can be derived.This is the case,for example,in a network with a single real-time traffic class that is assigned a single priority in a net-work of identical servers and identical allocations of bandwidth to the class on all servers(in this case,we simplify the notation to let,and).The following corollary shows how a delay bound can be computed if we loose the bound on.Corollary1:Suppose is the worst-case delay bound across any node in the network,and the path of anyflow in the network traverses at most nodes,then we have.If,then(11) Therefore the end-to-end delay can be bounded by(12) This delay formula does not depend on topology of the network except for the length of the longestflow path.We note that a very similar result was derived using a different approach in[3].B.Deriving the Delay FormulaIn this subsection,we discuss how to derive the delay formula given in(3).We will start with a formula for delay computation that depends onflow population,which we call the general delay formula.We will describe how to remove its dependency on information offlow population.For Server,suppose that the group offlows,at some time moment,has trafficflows.The constraint function can be formulated as the summation of the constraint functions of individualflows,that is,(13)where is the constraint function forflow in.Fur-ther,the aggregate traffic of group offlows is constrained by(14)The worst-case delay of priority-flows at Server can then easily be formulated in terms of the aggregated traffic con-straint functions and the service rate of the server as follows [13]:on,the number offlows in,and on the traffic con-straint functions of the individualflows.This kind of dependency on the dynamic system status must be removed in order to perform delay computations at configuration time.In the following sections,we describe how wefirst elimi-nate the dependency on the traffic constraint functions.Then we eliminate the dependency on the number offlows on each input link.The result is a delay formula that can be applied without knowledge aboutflow population.B.1Removing the Dependency on Individual Traffic ConstraintFunctionsWe now show that the aggregated traffic functioncan be bounded by replacing the individual traffic constraintfunctions by a common upper bound,which is indepen-dent of input link.The delay on each server can now be formulated without re-lying on traffic constraint functions within the network of indi-vidualflows.The following theorem in fact states that the delay for eachflow on each server can be computed by using the con-straint traffic functions at the entrance to the network only. Theorem2:The aggregated traffic of the group offlows is constrained by(16) where(19) where(20)(21)(22)(27) To maximize the right hand side of(19),we should maximize and minimize,,and.Under the constraint of(26),these parameters can be bounded for all possible distri-bution of numbers of activeflows on all input links,as the following theorem shows:Theorem3:If the worst-case queuing delay is experienced by the traffic with priority at Server,then,(28)(29)(30) andmembership)that is available before run time.For a network withfixed routers,flows can be classified at each server by their class identification,the source and the destination identification. In the following we use class and path(in form of source and destination identification)information to assign priorities, where allflows in the same class with the same source and des-tination have the same priority.This approach has two advan-tages over more dynamic ones.First,the priority assignment can be done before run time and thus does not burden the ad-mission control procedure at establishment time.Second,the static-priority schedulers need no dynamic information at run time,as the priority mapping for each packet is fully defined by its class identification,and its source and destination identifica-tions.No additionalfields in packet headers are needed.A.Outline of AlgorithmsMapping with increasing complexity can be used to assign priorities toflows:One-to-One Mapping:All theflows in a class are assigned the same priority.Flows in different classes are mapped into dif-ferent priorities.A simple deadline-based mapping can be used to assign priorities to classes with the least deadline getting the highest priority.The advantage of this method is its simplicity. Obviously,this does not take into account more detailed infor-mation such as topology and others.We use this mapping as baseline for the comparison with others.One-to-Many Mapping:Classes may be partitioned into sub-classes for priority assignment purposes,withflows from a class assigned different priorities.Flows in different classes,however, may not share a priority.In Subsection VI-B we present a ver-sion of this algorithm.This algorithm can recognize the differ-ent requirements offlows in a class and assign them different priorities,hence improving the network performance.The algo-rithm is still relatively simple,but it may use too many priorities given that it does not allow priorities to be shared byflows from different classes.Many-to-Many Mapping:The priority assignment is not con-strained by class membership,andflows from different classes can be assigned the same priority.Given its generality,this map-ping can achieve better performance than the other two.B.Details of AlgorithmsWe willfirst focus on Algorithm One-to-Many.We will then show that Algorithms One-to-One and Many-to-Many are a spe-cial case and generalization of Algorithm One-to-Many,respec-tively.Given the limited space,there will be no need to present the other two algorithms in details.The purpose of the static priority assignment algorithm is to generate a priority assign-ment table,which then is used by admission control and is loaded into routers for scheduling purposes.The priority as-signment table(see Table I for an example)consists of entries of type.The priority as-signment then maps from thefirst threefields in the entry to the priorityfield.Figure1shows our One-to-Many priority assignment algo-rithm.The algorithm uses a stack to store subsets of entries toTABLE IA E XAMPLE OF P RIORITY A SSIGNMENT T ABLEClass Destinationnode22node43......node61Fig.1.Algorithm One-to-Manywhich the priorityfields are to be assigned.Entries in each sub-set can potentially assigned to the same priority.The subsets are ordered in the stack in accordance to their real-time require-ments.The subset with entries that representflows with the most tight deadline and/or laxity is at the top of the stack.After its initialization,the algorithm works iteratively.At each iteration,the algorithmfirst checks whether enough un-used priorities are available.If not,the program stops and de-clares“failure”(Step4.2).Otherwise,a subset is popped from the stack.The algorithm then assigns the best(highest)avail-able priority to the entries in the subset if the deadlines of the flows represented by those entries can be met.However,if some of the deadline tests cannot be passed,Procedure Bi-Partition is called to partition the entries in the subset into two subsets based on their laxity.The idea here is that if we assign a higher pri-ority to entries with little laxity,we may pass the deadline tests for all entries.This is realized by pushing two new subsets into the stack in the proper order and by letting the future iteration deal with the priority assignment.Procedure Bi-Partition also assigns bandwidth to the different priorities in the class,that is, properly splits to reflect the partitioned subsets.The program iterates until either it exhausts all the subsets in the stack,in which case a successful priority assignment has been found and the program returns the assignment table or it must declare“failure”.The latter happens when either the program runs out of priorities or it cannot meet the timing re-quirments for a single entry in a subset.Because the size of a subset is halved at every iteration step, the worst-case time complexity of the algorithm is in the order of in the number of delay computations.We will show that this algorithm does perform reasonably well in spite of its low time complexity.Algorithm One-to-One is a special case of Algorithm One-to-Many presented in Figure1.For Algorithm One-to-One,no subset partition is allowed(otherwise entries in one class will be assigned to different priorities—a violation of the One-to-One principle).Thus,if we modify the code in Figure1,so that it returns“failure”whenever a failure on deadline test is found (Step4.7),it becomes the code for Algorithm One-to-One.On the other hand,we can generalize Algorithm One-to-Many to become Algorithm Many-to-Many.Recall that Algo-rithm Many-to-Many allows the priorities to be shared byflows in different classes.Note that sharing a priority is not necessary unless the priorities have been used up.Following this idea,we can modify the code in Figure1so that it becomes the code for Algorithm Many-to-Many:At Step4.2,when it is discovered that all the available priorities have been used up,do not return “failure”,but assign the entries with the priority that has just be used.In the case the deadline test fails,assign these entries with a higher priority(until the highest priority has been assigned).VII.E XPERIMENTAL E VALUATIONIn this section,we evaluate the performance of the systems that use our new delay analysis techniques and priority assign-ment algorithms discussed in the previous sections.Recall that we use a utilization-based admission control in our study:As long as the link utilization along the path of aflow does not exceed a given bound,the end-to-end deadline of theflow is guaranteed.The value of this bound therefore gives a good in-dication about how manyflows can be admitted by the network. We define the maximum usable utilization(MUU)to be summa-tion of the bandwidth portions that can be allocated to real-time traffic in all classes,and use this metric to measure the perfor-mance of the systems.For a given network and a given priority assignment algorithm,the value for the MUU is obtained by performing a binary search in conjunction with the priority as-signment algorithm discussed in Section VI.To illustrate the performance of our algorithms for different settings,we describe two experiments.In thefirst experiment, we use afixed network topology and compare the performance of the three algorithms presented in Section VI and measure how the algorithms perform for traffic with varying burstiness.In the second experiment,we measure how the three algorithms behave for networks with different topologies.In the following we describe the setup for the two experiments and discuss the results.A.Experiment1The underlying network topology in this experiment in the classical MCI network topology.All links in the network have a capacity of Mbps.All link servers in the simulated network use a static-priority scheduler with priorities.We assume that there are three classes of traffic:bits, bps,ms,bits,bps,ms,andbits,bps,ms,where each triple defines ,and the end-to-end delay requirement for the class.Any pair of nodes in the simulated networks may request aflow in any class.All the traffic will be routed along shortest paths in terms of number of hops from source to destination.The results of these simulations are depicted in thefirst row of Table II.In the subsequent rows of the table,the same simulation results are depicted for higher-burtiness traffic.In each row,the burstiness parameter is quadrupled.As expected,Table II shows that the MUU increases signifi-cantly with more sophisticated assignment algorithms.The per-formance improvement of algorithms One-to-Many and Many-to-Many over One-to-One remains constant for traffic with widely different burstiness.TABLE IIT HE C OMPARISON OF MUU FOR D IFFERENT B URSTY D ELAY IN THE MCIN ETWORKMaximum Usable UtilizationOne-to-Many0.630.08s0.260.430.141.28s0.0260.050From Table II,we also see that the traffic burstiness heavily impacts on the MUU.In fact,for very bursty traffic the MUU can get quite low.We would like to point out that,even for very bursty traffic,sufficient amounts of bandwidth can still be designated for real-time traffic.B.Experiment2In the second experiment we keep the setup of Experiment1, except that we do not vary the burst delay。