A MILP model concerning the optimisation of penalty factors for the short-term distribution..
外企英语笔试题及答案
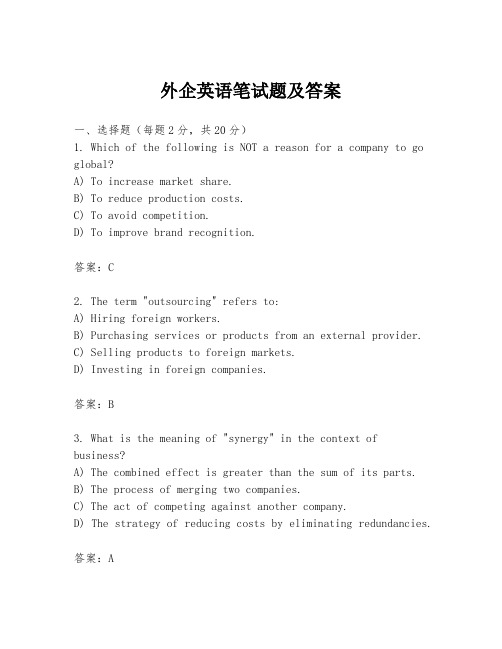
外企英语笔试题及答案一、选择题(每题2分,共20分)1. Which of the following is NOT a reason for a company to go global?A) To increase market share.B) To reduce production costs.C) To avoid competition.D) To improve brand recognition.答案:C2. The term "outsourcing" refers to:A) Hiring foreign workers.B) Purchasing services or products from an external provider.C) Selling products to foreign markets.D) Investing in foreign companies.答案:B3. What is the meaning of "synergy" in the context of business?A) The combined effect is greater than the sum of its parts.B) The process of merging two companies.C) The act of competing against another company.D) The strategy of reducing costs by eliminating redundancies. 答案:A4. In business, "due diligence" usually refers to:A) The process of paying close attention to details.B) The legal obligation to be honest in business dealings.C) The investigation of a potential investment or acquisition.D) The requirement to have a certain level of education.答案:C5. Which of the following is an example of a non-tangible asset?A) Land.B) Machinery.C) Trademark.D) Inventory.答案:C6. What does "B2B" stand for in business?A) Business to Business.B) Business to Consumer.C) Business to Government.D) Business to Retailer.答案:A7. If a company is "vertically integrated," it means that:A) It owns all the stages of the supply chain.B) It specializes in one particular product line.C) It operates in multiple industries.D) It is heavily reliant on external suppliers.答案:A8. The acronym "ROI" stands for:A) Return on Investment.B) Risk of Investment.C) Rate of Interest.D) Revenue of Investment.答案:A9. A "franchise" is a type of business arrangement where:A) A company sells its products to another company.B) A company licenses its business model to another entity.C) A company acquires another company.D) A company merges with another company.答案:B10. What is "SWOT analysis"?A) A method for evaluating a company's strengths, weaknesses, opportunities, and threats.B) A type of financial statement.C) A way to measure customer satisfaction.D) A tool for predicting market trends.答案:A二、填空题(每题1分,共10分)1. The process of a company expanding its business to foreign countries is known as ______.答案:internationalization2. When a company's stock price rises above its initialoffering price, it is said to be ______.答案:above the market3. A ______ is a document that outlines the business's goals, strategies, and plans for achieving those goals.答案:business plan4. The term "blue-chip" refers to stocks that are consideredto be ______.答案:highly stable and reliable5. In finance, "leverage" refers to the use of borrowed money to ______.答案:increase potential returns6. The ______ is the process of identifying, analyzing, and mitigating potential risks.答案:risk management7. A ______ is a financial instrument that represents ownership in a company.答案:share8. The ______ is the highest level of management in a company, responsible for setting overall direction and strategy.答案:board of directors9. A ______ is a type of investment that is not easily converted to cash.答案:illiquid asset10. The ______ is the process of identifying and analyzing the needs and problems of potential customers and creating products or services to meet those needs.答案:market research三、简答题(每题5分,共30分)1. What are the benefits of a company engaging in international trade?答案:The benefits of international trade for a company include access to new markets, economies of scale, diversification of risk, and the ability to source raw materials and labor at lower costs.2. Explain the concept of "corporate social responsibility" (CSR).答案:Corporate social responsibility (CSR) refers to a company's commitment to manage its impact on the environment, consumers, employees, communities, stakeholders, and all other parts of society. It involves a company's efforts to improve society by engaging in ethical practices, community development, and environmental sustainability.3. What is the difference between a "sole proprietorship" anda "partnership"?答案:A sole proprietorship is a type of business owned by one person who has complete control and unlimited liability for the business's debts. A partnership is a business owned by two or more people who share the profits, management, and legal responsibilities of the business。
A micro-constraint model of Optimality Theory Do infants
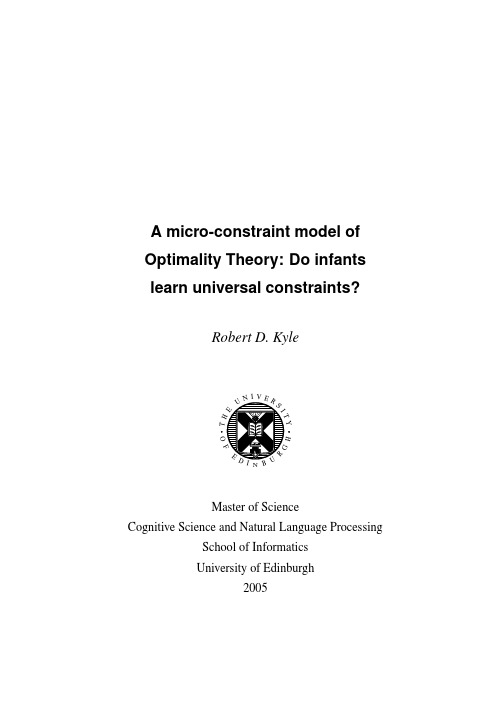
A micro-constraint model of Optimality Theory:Do infantslearn universal constraints?Robert D.KyleVNMaster of ScienceCognitive Science and Natural Language ProcessingSchool of InformaticsUniversity of Edinburgh2005AbstractDoes Optimality Theory offer a basis for a neurologically plausible model of language?This paper examines the case for Optimality Theory as a plausible model of language,and suggests modifications to the theory that would improve the biological plausibility,learnability,and co-herence of the model.The micro-constraint model which emerges from these modifications, suggests that language,and linguistic universal behaviour is learned by settling upon an arbitrary set micro-constraints,rather than acquiring or ranking innate constraints as specified by Opti-mality Theory.The validity of this micro-constraint model is tested using a Simple Recurrent Network model of speech syllabification,and some support is found for the claim that arbitrary micro-constraints might reproduce universal constraints.However,we suggest that further work, with fewer assumptions about the nature of the speech stream is needed in order to improve the conclusiveness of the result.iAcknowledgementsI would like to thank my supervisor,Mark Steedman,for his helpful guidance and advice throughout the project.I would also like to thank Mits Ota,for his considerable help on aspects of Optimality Theory, and insightful comments on the experiment.Last but not least,thanks to Lexi and Sara,whose presence in the MSc lab helped while away the three months spent on this project.Their lunchtime banter and considerable knowledge of esoteric perl and latex syntax was instrumental to the success of this project.iiDeclarationI declare that this thesis was composed by myself,that the work contained herein is my own except where explicitly stated otherwise in the text,and that this work has not been submitted for any other degree or professional qualification except as specified.(Robert D.Kyle)iiiTable of Contents1Introduction11.1Overview (1)1.2The structure of the thesis (2)2Background42.1Optimality Theory:a neurologically plausible model of language? (4)2.1.1Why Optimality Theory is deeply connected to connectionism (4)2.1.2Why Optimality Theory has nothing to do with connectionism (8)2.1.3Is strict domination an empirical fact? (11)2.1.4Are universal constraints and strict domination necessary? (11)2.2Universal constraints:more trouble than they are worth? (12)2.2.1The learnability of universal constraints (12)2.2.2The neurological plausibility of universal constraints (13)2.2.3The biological plausibility of universal constraints (14)2.3Micro-constraints:a solution to OT’s problems? (14)2.3.1Optimality Theory at the microscopic level (14)2.3.2How learned micro-constraints might imitate universal macro-constraints152.3.3How micro-constraints offer a simplified architecture (17)2.3.4Stochastic interpretations of Optimality Theory (17)2.4Recapitulation:The features of a micro-constraint model of Optimality Theory.183Design193.1The theoretical claim (19)3.2How the claim can be tested (19)iv3.3The syllabification problem (21)3.4Experimental hypothesis (22)4Method234.1Assembly of the corpora (23)4.2Experimental method (25)5Results275.1Assessing acquisition of phonology (27)5.2Assessing syllabification performance (27)5.2.1Test of the experimental hypothesis (30)6Discussion346.1Support for the hypothesis (34)6.1.1How should syllabification be measured? (34)6.1.2The difficulty of”doing Optimality Theory in a straitjacket” (35)6.1.3A preference for words with no coda (36)6.2Methodological Issues (36)6.2.1The corpus (36)6.2.2The model (38)6.3Interpretation of the results (39)6.4What is the use of a micro-constraint model of Optimality Theory? (40)7Future Work427.1Unsupervised learning of phonology (42)8Conclusion44 Bibliography45A Phonological key48B Phonetic feature table50vChapter1Introduction1.1OverviewThis thesis is focused upon constraint-based approaches to language,and in particular,the neu-rological plausibility of existing linguistic theories,such as Optimality Theory.Constraint based approaches to language offer an interesting basis for exploration of the plausibility of linguis-tic theories,because they describe language using a framework which has its roots in neural network research.This thesis examines the case for Optimality Theory as a neurologically plau-sible model of language,and suggests that the plausibility of the model would be improved if claims of universal constraints and strict domination were dropped.We argue that the intro-duction of universal constraints,which in turn motivates strict domination,serves to make the theory less biologically plausible,and reduces learnability of languages in the resulting model. We propose,that Optimality Theory is better conceived at the microscopic level,and that a more coherent model of language emerges if we consider the overall behaviour of Optimality Theory constraints to be as a result of the interaction of microscopic constraints at the neural level.This micro-constraint model of Optimality Theory implies that the universal constraints observed at a higher level,are in fact learned,and can be produced by any combination of micro-constraints which provide functionally equivalent behaviour to the high-level macro-constraints.This micro-constraint model of Optimality Theory is put to the test by examining whether a model that has learned to perform at a particular linguistic task by modifying internal micro-constraints,actu-ally displays behaviour,which at a higher-level of analysis can be attributed to a knowledge of the universal macro-constraints.We describe such an experiment by training a Simple Recur-1rent Network to predict syllable boundaries,and examining whether its syllabification behaviour implies implicit knowledge of Optimality Theory constraints relevant to syllabification.The test of the experimental hypothesis at the syllable level returns an inconclusive result, do to the considerable simplifying assumptions that were made in constructing the model and corpus.However,a test of the experimental hypothesis at the word level supports the hypothesis, and implies that the model has indeed learned a set of micro-constraints that display universal macro-constraint behaviour.We conclude by suggesting ways in which a more conclusive result might be reached,and discuss future work which could be used to further investigate this model.1.2The structure of the thesisChapter2In this chapter we introduce Optimality Theory in its historical context,and examine the properties which may or may not offer the model some neurological plausibility.We suggest areas in which the model might be modified to improve its coherence,and assess the consequences of making these modifications.Chapter3In the Design chapter we state an experimental claim motivated by the evidence described in the previous chapter.This claim is used to design an experimental test using a model of speech syllabification.The chapter concludes with an experimental hypothesis.Chapter4Chapter4describes experimental method,including the generation of the training data from a corpus of Child-directed speech,and the construction of the syllabification model.Chapter5In chapter5we report the model’s performance on predicting the next phoneme ina speech stream,and evaluate the model’s syllabification preference.The model’s perfor-mance on syllabification at word and syllable levels is used to examine the experimental hypothesisChapter6In this chapter we analyse the results reported in the previous chapter,and discuss on what grounds the experimental hypothesis is supported.We point out shortcomings of the model both theoretical and methodological,and we suggest ways in which the corpus and model could be modified to provide more conclusive evidence.We also discuss the relevance of the experimental claim,and the utility of the model proposed in chapter2.Chapter7This chapter includes a discussion of future work which might provide a more con-clusive test of the model.In particular we examine the possibility of constructing unsuper-vised models of phonological acquisition using recurrent self-organising maps.We also discuss previous language models which have made use of these architectures.Chapter8Conclusion.Chapter2Background2.1Optimality Theory:a neurologically plausible model oflanguage?This thesis is concerned with the neurological plausibility of existing linguistic theories,and for that reason we have chosen to examine the properties of Optimality Theory.Optimality Theory was chosen for study because it offers an interesting perspective on the issue for two reasons:1.It has been successful in phonology where generative models have failed.2.It describes language using mechanisms that are compatible with the way in which thebrain works.As such,it provides a interesting avenue to explore neurologically plausible descriptions of lan-guage.In this chapter we will examine the case for Optimality Theory as a neurologically plau-sible model of language,and we will suggest modifications that would improve its learnability and biological plausibility.2.1.1Why Optimality Theory is deeply connected to connectionism1Although Optimality Theory wasfirst introduced in1993(Prince and Smolensky,1993)and is a relatively new model,the roots of the theory stretch back further,to Harmonic Grammar(Smolensky et al.,1992),a model of language that was inspired by computational mechanisms developed in neural network research.Rather than describe language as a generative formal system which defines the legal forms of a language,Optimality Theory and Harmonic Grammar both describe language as a set of constraints which interact to determine the optimal parse.In Harmonic Grammar,the optimal parse is the one for which the numerical rankings of the violated constraints sum to the lowest value.Optimality Theory and Harmonic Grammar both claim that the constraints that are needed to adequately describe the empirical data,are a set of constraints that are universal to all languages. In these models,the differences between languages arise as a result of speakers’ranking the con-straints in subtly different ways.The difference between the Optimality Theory and Harmonic Grammar is that Harmonic Grammar originally claimed that constraints could potentially take any ranking value,whilst Optimality Theory has since proposed that the universal constraints are ranked ordinally,and obey a principle known as strict domination.The optimal version of any parse in Harmonic Grammar or Optimality Theory is often calculated using a constraint tableau, such as the one shown infigure2.1.Candidate words or utterances are written on one side of the tableau,and the constraints which they violate are marked in the appropriate columns.The two theories differ in the way that the constraints interact to determine which candidate is optimal.The system of interacting constraints described by Harmonic Grammar was directly inspired by the behaviour of weights in Neural Networks,which act as constraints upon the activation of neurons.Prior to introducing Harmonic Grammar,Smolensky developed Harmony Theory (Smolensky,1986),a model of the activation dynamics that occur in certain classes of neural networks.The analysis introduced by Harmony Theory implied that networks with an architec-ture comparable to biological networks2were extremely well designed to rapidly solve constraint satisfaction problems.Perhaps it was reasoned,that if language had developed by exploiting the computational abilities of the brain,then the mechanisms of constraint satisfaction might provide a useful framework in which to understand language.The extent to which Harmonic Grammar and Optimality Theory are inspired by connectionist research can be seen in the use of the term Harmony to describe the degree of optimality of each parse.Harmony,from Harmony Theory,is a quantitative value which defines the degree to which the constraints(competing weights)in a neural network are satisfied at any point inFigure2.1:A constraint tableau in Optimality Theory.The table shows a list of candidates on the left hand side,and relevant constraints along the top.Asterisks indicate a violation of the con-straint,with an exclamation indicating the fatal violation.PARSE is greyed out where the violation of a higher ranked constraint has ruled out this candidate.The optimal candidate is the one which least violates the constraints in order of their ranking.Figure2.2:The way in which Optimality Theory and Harmonic Grammar define language can be viewed as a set of constraints which define the space of permissible utterances in a particular language.In this view,constraints are circles demarcating the space of utterances that each constraint allows.Each circle has a radius r n which reflects the ranking of the constraint.An utterance within a particular language may fall anywhere within the total space,and its Harmony can be calculated by summing the radius’of the circles the utterance falls within(the constraints which permit this utterance).In this example,the Harmony of utterance a r1,b r1r2, c r1r2r3,and d r3time.Harmony in a neural network increases monotonically towards attractor states,which once reached,are stable enough to drive activation in other areas of the network,much in the same way as stable perception of a particular concept can lead to a linguistic,motor,or other cognitive response.These harmony dynamics are present in all bi-directionally connected networks,and so given their close links with biological networks,these mechanisms provide a plausible basis from which to build a model of language.2.1.2Why Optimality Theory has nothing to do with connectionism3There are two distinct arguments as to why Optimality Theory is incompatible with connectionist descriptions of language.1.Purely connectionist approaches to language are incompatible with the goals of the In-tegrated connectionist/symbolic(ICS)paradigm in Cognitive Science(Smolensky et al., 1992).As a result,proponents of ICS have attempted to distance themselves from connec-tionist interpretations of Optimality Theory.2.Strict domination of constraints cannot plausibly occur at the neural level.2.1.2.1Optimality Theory and the Integrated Connectionist-Symbolic paradigmIn order to understand the unusual position taken by Optimality Theory,the argument must be viewed in the context of the Integrated connectionist/symbolic(ICS)paradigm.The ICS paradigm stems from the belief that in order to explain fully the nature of cognition,a theory must provide a description at both the symbolic and sub-symbolic level.Explanations which favour one level,and eliminate the other are unacceptable to the proponents of ICS.Since the introduction of Optimality Theory,this stance has forced the model to develop its symbolic credentials in order to improve its standing as an integrative theory.At the present time,the overwhelming majority of work within Optimality Theory has concentrated on its validity as a symbolic model of language,and little attention has been paid to its decreasing plausibility at the neural level.In fact,modifications to the theory motivated by symbolic considerations have rendered Optimality Theory incompatible with simple connectionist models,let alone the complex networks that occur in the brain.ICS’s stance as to what a valid model of cognition must consist of means that connectionist interpretations of Optimality Theory are typically viewed as either implementationist or elimina-tivist:either the model merely implements symbolic linguistic processing,and therefore by itself is an incomplete model,or it eliminates the need for a symbolic description,and is therefore not a valid model of cognition.Catch22!”All of the prototypical objectives in eliminativist research are completely anti-thetical to Optimality Theory.And as for the implementationalist approach,ratherthan arguing for the contribution of Optimality Theory based on issues of connec-tionist implementation,we have not even entertained the question.”(Prince andSmolensky,1993)This kind of logic has discouraged researchers working outside of the ICS paradigm from contributing to thefield-any attempts to suggest sub-symbolic modifications to the theory are likely to be ruled out a priori,as an attempt to eliminate the symbolic level of description.2.1.2.2Differences between Optimality Theory and Harmonic GrammarOriginally,Harmonic Grammar was introduced as a model of language alongside the ICS paradigm, but developments in Harmonic Grammar eventually led to the introduction of Optimality The-ory,a special case of Harmonic Grammar in which constraints are ranked ordinally,rather than numerically4.”Phonological applications of Harmonic Grammar led Alan Prince and myself to a remarkable discovery:in a broad set of cases,at least,the relative strengthsof constraints need not be specified numerically.For if the numerically weightedconstraints needed in these cases are ranked from strongest to weakest,it turns outthat each constraint is stronger than all the weaker constraints combined.That is,thenumerical strengths are so arranged that each constraint can never be overruled byweaker constraints,no matter how many.”(Smolensky,1995)While the introduction of strict domination might have been empirically motivated,it quite clearly damages Optimality Theory’s validity as a sub-symbolic model.For reasons we shall discuss later,strict domination of constraints is not something which can plausibly occur in biological networks of neurons.Figure2.3:Now we can see more clearly how Optimality Theory differs from Harmonic Grammar usingfigure2.2.Optimality Theory can be seen as a special case of Harmonic Grammar where the rankings of the constraints(the radius’of the circles)are distributed along an exponential scale.The result is that no combination of lower ranked constraints can produce a Harmony value greater than a highly ranked constraint.For example,the ranking of permissible constraints is defined so that r3r2r2r1,and therefore d b aSo does strict domination conclusively count out Optimality Theory as a neurally plausible model?Possibly not.The fact that connectionism is antithetical to ICS should not discourage re-searchers from pursuing the consequences of a connectionist interpretation of the theory.Those who do not accept the necessity of integrated connectionist/symbolic explanations in Cognitive Science need not be concerned that a neural interpretation of Optimality Theory might not grant symbolic structures the same ontological relevance as the standard model.Also,the argument that strict domination renders Optimality Theory incompatible with connectionist models of lan-guage is far from sound.The argument depends upon the following assumptions1.That strict domination is an empirical fact2.That the constraints in Optimality Theory are universalIn the next section we will examine the evidence for these two assumptions.2.1.3Is strict domination an empirical fact?Although the introduction of strict domination was motivated by observations in phonology,there are now well established cases where strict domination is broken(Joanisse,2000).One example of this is local conjunction(Ito and Mester,1998),whereby a violation of a conjunction of lower ranked constraints is enough to overrule a more highly ranked constraint.Mechanisms such as Sympathy Theory(McCarthy,1999)have been suggested as modifications to Optimality Theory that would allow for strict domination to be broken occasionally.But these modifications should ring alarm bells when claims are made of empirical support for strict domination.2.1.4Are universal constraints and strict domination necessary?Despite the example of local conjunction,there are actually relatively few case of strict domina-tion being broken.Perhaps the question we should be asking is”Why are there so few examples of strict domination being broken?”The answer to this question might lie in the considerable controversy over what can be con-sidered a constraint in Optimality Theory(Eisner,1999).It is not clear on which basis the Universal constraint set(CON)was discovered or chosen-were constraints that might break strict domination discarded in the initial definition of CON?Obviously,if we are free to choose any constraints,then it should always be possible to pick a set of constraints which will display strictdomination.The arbitrary nature of CON has also been questioned by Ellison,who has suggested that the Universal constraint is better supported as a convention,rather than fact(Ellison,2000). One of the major criticisms of Optimality Theory is aimed at the extremeflexibility of its choice of constraints.There are no obvious reasons why absurd constraints such as PALINDROMIC5 (Eisner,1999)or FRENCH6should be any less valid than those in the Universal constraint set.The existence and significance of linguistic universals is a controversial topic,but there is enough evidence to suggest that many of these linguistic universals can be regarded as epiphe-nomenal features of a shared biological,social,and linguistic heritage.Or even as universal solutions to the problem of efficient communication in a noisy environment.If there were a set of linguistic universals,it is not clear why they should be described at the level of folk-psychological linguistic units,as they are in Optimality Theory.If linguistic univer-sals have emerged as a by-product of agents with a shared cognitive apparatus communicating in a noisy environment,there is no obvious reason why these constraints should only be defined in terms of arbitrary linguistic units,rather than a more messy set of constraints which are related to the biological apparatus of speech and cognition.To conclude,Optimality Theory’s claim to a language-specific universal constraint set can be called into question,without having to deny the existence of universal linguistic behaviour.We will now turn our attention to Optimality Theory’s claim of a universal constraint set,and the effect this has on the learnability and plausibility of the model.2.2Universal constraints:more trouble than they are worth?If the legitimacy of the universal constraint set is still an open question,perhaps we should re-examine this assumption,and ask whether or not universal constraints and strict domination are a worthy exchange for the models neurological plausibility.2.2.1The learnability of universal constraintsOne way in which we can observe the effect of universal constraints is the learnability of language within the Optimality Theory framework.Figure2.4:The architecture of Optimality Theory.A mechanism GEN generates candidate sets based upon the input,and these sets are evaluated tofind the most optimal parse,which is passed on to the output.By requiring infants tofind a particular ranking of a specific set of universal constraints, theorists have introduced a massive learning problem.Even if the universal constraints were somehow innate,there are a factorial number of possible rankings.Despite this apparent learning problem,Smolensky and Tesar have proposed the Constraint Demotion algorithm,which given CON,can rank the constraints,and learn certain classes of grammars within N(N-1)informative examples,where N is the number of constraints(Tesar and Smolensky,2000).However,this work assumes that the universal constraint set is innate,and is also depen-dent upon an architectural model which has no biological plausibility(something which ICS proponents believe is not a pressing concern).The constraint demotion algorithm suggested by Smolensky and Tesar also experiences problems with empirical data,as it cannot account for the way in which stages of language acquisition overlap,and the learners tendency towards regres-sion.(Curtin,2000).2.2.2The neurological plausibility of universal constraintsIf we accept the claim of an innate universal constraint set,and therefore the existence of strict domination,it is very difficult to see how Optimality Theory might relate to neural processing.At the neural level,’innately specified’weights between neurons would need to line up along an exponential scale,so that no combination of lower ranked constraints could overcome a higher ranked constraint(Smolensky et al.,ress).Although this is highly implausible at a low level,itis not infeasible that moreflexible low-level constraints might display strict domination-like behaviour at a macroscopic level-we will explore this possibility later.2.2.3The biological plausibility of universal constraintsFinally,and most significantly,given the difficulty of learning specific universal constraints, the suggestion that the problem may be solved by claiming that children are born with these constraints is not a biologically plausible hypothesis.There is no biological or neurological evidence that could support the claim that a linguistic-specific set of constraints are innate,in fact many developmental neuroscientists reject the very notion of innate behaviour(Elman et al., 1996).As discussed in section2.1.4,there are plenty of ways in which linguistic universals might emerge,without having to rely upon a specific set of linguistic constraints.Given the long list of theoretical problems introduced by claiming universal constraints,we suggest that the theory would be better off dropping the requirement of a universal constraint set,and allowing any set of constraints that provide functionally equivalent behaviour to that observed by linguists.2.3Micro-constraints:a solution to OT’s problems?2.3.1Optimality Theory at the microscopic levelAs we have discussed in section2.1.1,Optimality Theory borrows much of its computational mechanisms from Harmony Theory,a model of the activation dynamics that occur in bi-directionally connected neural networks.Harmony measures the extent to which the constraints(or weights) learned by the network are satisfied by a particular input.Harmony is defined by the following equation2.5.1HFigure2.5:At t0,Unit C has an activation of1,and units A and B an activation of0.The competing constraints defined by the weights interact tofind the most Harmonious state as t∞. Note how quickly the Harmony reaches its maximum value-these networks are almost optimally designed for this task.units.Harmony in these networks increases monotonically to a state1that is stable in the absenceof further input to the network.These stable states are hypothesised to drive activation in other areas,so can be viewed as representing the optimally Harmonic state,or at a much higher level,as representing an interpretation of the input utterance,given the constraints learned by the network.The process of parallel constraint satisfaction,and the monotonic increase in harmony is showninfigure2.5.These bi-directionally connected networks display behaviour which makes them almost opti-mally adapted to the problem of dealing with linguistic input-their ability to resolve ambiguous input in parallel is an inherent feature of their architecture.2.3.2How learned micro-constraints might imitate universal macro-constraintsWe’ve seen how activation dynamics at the neural level provide the basis for the Optimality Theory framework,and so the question that we must now address is whether the macroscopic behaviour observed in Optimality Theory needs strict domination and a universal constraint setin order to reproduce the behaviour observed by linguists.。
大学英语b2试题及答案

大学英语b2试题及答案一、听力理解(共20分)1. What is the man doing?A) Reading a bookB) Watching TVC) Cooking dinnerD) Listening to music答案:C2. Where does the conversation most likely take place?A) In a libraryB) In a restaurantC) At a bus stopD) In a classroom答案:B3. What does the woman mean?A) She is not interested in the movie.B) She has seen the movie before.C) She wants to watch the movie again.D) She is looking for the movie ticket.答案:A4. How much does the woman need to pay for the shirt?A) $20B) $40C) $60D) $80答案:B5. What is the relationship between the two speakers?A) Husband and wifeB) Teacher and studentC) Doctor and patientD) Salesperson and customer答案:A二、阅读理解(共30分)6. According to the passage, which of the following is true?A) The company has been in business for over 50 years.B) The company was founded by John Smith.C) The company has a global presence.D) The company specializes in technology products.答案:C7. What is the main purpose of the article?A) To advertise a new product.B) To inform about a company's history.C) To persuade readers to invest in the company.D) To announce a new partnership.答案:B8. What can be inferred from the text?A) The author is satisfied with the current situation.B) The author is concerned about the future.C) The author is optimistic about the company's prospects.D) The author is critical of the company's management.答案:B9. What does the author suggest as the best approach to solving the problem?A) Implementing new technology.B) Increasing government funding.C) Encouraging public awareness.D) Conducting further research.答案:D10. Which of the following is NOT a benefit mentioned in the article?A) Improved efficiency.B) Reduced costs.C) Enhanced safety.D) Increased job opportunities.答案:D三、词汇与语法(共20分)11. The company has ________ the contract for three years.A) signedB) sealedC) ratifiedD) canceled答案:A12. Despite the heavy rain, they ________ their journey to the mountain.A) postponedB) abandonedC) resumedD) continued答案:D13. The professor's lecture was so ________ that it put everyone to sleep.A) boringB) fascinatingC) engagingD) enlightening答案:A14. She was ________ to find that her favorite author would be at the book fair.A) surprisedB) delightedC) disappointedD) annoyed答案:B15. The new policy will ________ a significant impact on the environment.A) haveB) holdC) takeD) make答案:A四、翻译(共30分)16. 请将以下句子翻译成英文:“这个项目的成功在很大程度上取决于团队的合作。
托福听力测试题及答案
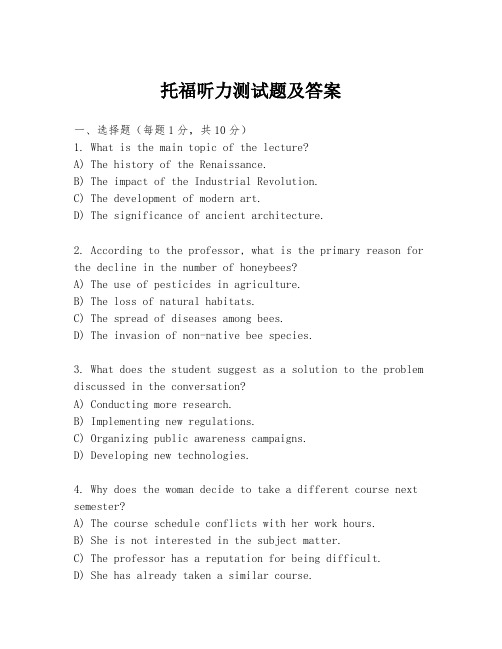
托福听力测试题及答案一、选择题(每题1分,共10分)1. What is the main topic of the lecture?A) The history of the Renaissance.B) The impact of the Industrial Revolution.C) The development of modern art.D) The significance of ancient architecture.2. According to the professor, what is the primary reason for the decline in the number of honeybees?A) The use of pesticides in agriculture.B) The loss of natural habitats.C) The spread of diseases among bees.D) The invasion of non-native bee species.3. What does the student suggest as a solution to the problem discussed in the conversation?A) Conducting more research.B) Implementing new regulations.C) Organizing public awareness campaigns.D) Developing new technologies.4. Why does the woman decide to take a different course next semester?A) The course schedule conflicts with her work hours.B) She is not interested in the subject matter.C) The professor has a reputation for being difficult.D) She has already taken a similar course.5. What is the main purpose of the campus tour mentioned in the lecture?A) To introduce new students to the campus facilities.B) To highlight the university's academic achievements.C) To promote the university to potential students.D) To raise funds for campus improvements.二、填空题(每题1分,共5分)6. The speaker mentions that the _______ is a key factor in determining the success of a business.7. In the dialogue, the man suggests that they should _______ before making a decision.8. The professor explains that the _______ theory has been widely accepted in the field of psychology.9. The woman is concerned about the _______ of the new policy on the local community.10. The student is looking for a part-time job that offers_______ and flexible hours.三、简答题(每题2分,共4分)11. Summarize the main points of the lecture on environmental conservation.12. What are the potential benefits of the proposed research project discussed in the conversation?四、论述题(每题3分,共3分)13. Discuss the role of technology in modern education andits implications for the future of learning.答案:1-5: D A C A C6. innovation7. consult with an expert8. cognitive dissonance9. impact10. health insurance11. The lecture covered the importance of biodiversity, the threats to natural habitats, and the need for sustainable practices.12. The research could lead to new treatments for diseases, improve public health, and contribute to scientific knowledge.13. Technology has revolutionized education by providing access to a wealth of information and interactive learning tools. However, it also raises concerns about the potential loss of human interaction and the digital divide.。
委托代理模型的连续时间版本(Sannikov)
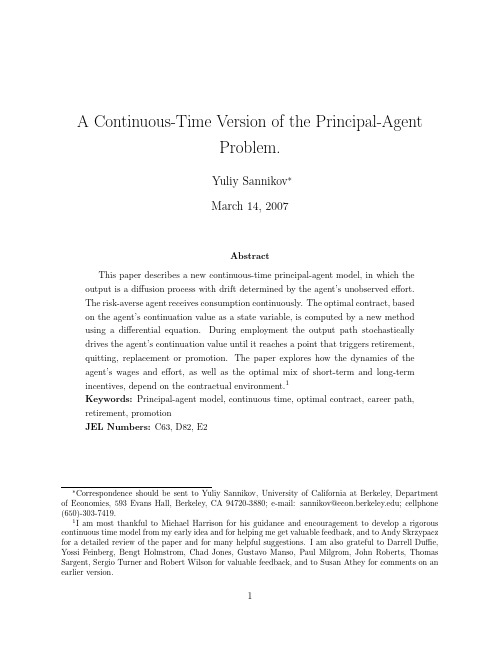
A Continuous-Time Version of the Principal-AgentProblem.Yuliy Sannikov∗March14,2007AbstractThis paper describes a new continuous-time principal-agent model,in which the output is a diffusion process with drift determined by the agent’s unobserved effort.The risk-averse agent receives consumption continuously.The optimal contract,basedon the agent’s continuation value as a state variable,is computed by a new methodusing a differential equation.During employment the output path stochasticallydrives the agent’s continuation value until it reaches a point that triggers retirement,quitting,replacement or promotion.The paper explores how the dynamics of theagent’s wages and effort,as well as the optimal mix of short-term and long-termincentives,depend on the contractual environment.1Keywords:Principal-agent model,continuous time,optimal contract,career path,retirement,promotionJEL Numbers:C63,D82,E2∗Correspondence should be sent to Yuliy Sannikov,University of California at Berkeley,Department of Economics,593Evans Hall,Berkeley,CA94720-3880;e-mail:sannikov@;cellphone (650)-303-7419.1I am most thankful to Michael Harrison for his guidance and encouragement to develop a rigorous continuous time model from my early idea and for helping me get valuable feedback,and to Andy Skrzypacz for a detailed review of the paper and for many helpful suggestions.I am also grateful to Darrell Duffie, Yossi Feinberg,Bengt Holmstrom,Chad Jones,Gustavo Manso,Paul Milgrom,John Roberts,Thomas Sargent,Sergio Turner and Robert Wilson for valuable feedback,and to Susan Athey for comments on an earlier version.1Introduction.The understanding of dynamic incentives is central in economics.How do companies moti-vate their workers through piecerates,bonuses,and promotions?How is income inequality connected with productivity,investment and economic growth?How dofinancial contracts and capital structure give incentives to the managers of a corporation?The methods and results of this paper provide important insights to many such questions.This paper introduces a continuous-time principal-agent model that focuses on the dynamic properties of optimal incentive provision.We identify factors that make the agent’s wages increase or decrease over time.We examine the degree to which current and future outcomes motivate the agent.We provide conditions under which the agent eventually reaches retirement in the optimal contract.We also investigate how the costs of creating incentives and the dynamic properties of the optimal contract depend on the contractual environment:the agent’s outside options,the difficulty of replacing the agent, and the opportunities for promotion.Our new dynamic insights are possible due to technical advantages of the continuous-time methods over the traditional discrete-time ones.Continuous time leads to a much simpler computational procedure tofind the optimal contract by solving an ordinary differ-ential equation.This equation highlights the factors that determine optimal consumption and effort.The dynamics of the agent’s career path is naturally described by the drift and volatility of the agent’s payoffs.The geometry of solutions to the differential equation allows for easy comparisons to see how the agent’s wages,effort and incentives depend on the contractual environment.Finally,continuous time highlights many essential features of the optimal contract,including the agent’s eventual retirement.In our benchmark model a risk-averse agent is tied to a risk-neutral principal forever after employment starts.The agent influences output by his continuous unobservable effort input.The principal sees only the output:a Brownian motion with a drift that depends on the agent’s effort.The agent dislikes effort and enjoys consumption.We assume that the agent’s utility function has the income effect,that is,as the agent’s income increases it becomes costlier to compensate him for effort.Also,we assume that the agent’s utility of consumption is bounded from below.At time0the principal can commit to any history-dependent contract.Such a contract specifies the agent’s consumption at every moment of time contingent on the entire past output path.The agent demands an initial reservation utility from the entire contract inorder to begin,and the principal offers a contract only if he can derive a positive profit from it.After we solve our benchmark model,we examine how the optimal contract changes if the agent may quit,be replaced or promoted.As in related discrete-time models,the optimal contract can be described in terms of the agent’s continuation value as a single state variable,which completely determines the agent’s effort and consumption.After any history of output the agent’s continuation value is the total future expected utility.The agent’s value depends on his future wages and effort.While in discrete time the optimal contract is described by cumbersome functions that map current continuation values and output realizations into future continuation values and consumption,continuous time offers more natural descriptors of employment dynamics: the drift and volatility of the agent’s continuation value.The volatility of the agent’s continuation value is related to effort.The agent has incentives to put higher effort when his value depends more strongly on output.Thus, higher effort requires a higher volatility of the agent’s value.The agent’s optimal effort varies with his continuation value.To determine optimal effort,the principal maximizes expected output minus the costs of compensating the agent for effort and the risk required by incentives.If the agent is very patient,so that incentive provision is costless,the optimal effort decreases with the agent’s continuation value due to the income effect.Apart from this extreme case,the agent’s effort is typically nonmonotonic because of the costs of exposing the agent to risk.The drift of the agent’s value is related to the allocation of payments over time.The agent’s value has an upward drift when his wages are backloaded,i.e.his current consump-tion is small relative to his expected future payoff.A downward drift of the agent’s value corresponds to frontloaded payments.The agent’s intertemporal consumption is distorted to facilitate the provision of incentives.The drift of the agent’s value always points in the direction where it is cheaper to provide the agent with incentives.Unsurprisingly,when the agent gets patient,so that incentive provision is costless,his continuation value does not have any drift.Over short time intervals,our optimal contract resembles that of Holmstrom and Mil-grom(1987)(hereafter HM),who study a simple continuous-time model in which the agent gets paid at the end of afinite time interval.HM show that optimal contracts are linear in aggregate output when the agent has exponential utility with a monetary cost of effort.2 2Many other continuous-time papers have extended the linearity results of HM.Schattler and Sung (1993)develop a more general mathematical framework for such results,and Sung(1995)allows the agentThese preferences have no income effect.According to Holmstrom and Milgrom(1991), the model of HM is“especially well suited for representing compensation paid over short period.”Therefore,it is not surprising that the optimal contract in our model is also approximately linear in incremental output over short time periods.In the long-run,the optimal contract involves complex nonlinear patterns of the agent’s wages and effort.In our benchmark setting,where the contract binds the agent to the principal forever,the agent eventually reaches retirement.After retirement,which occurs when the agent’s continuation value reaches a low endpoint or a high endpoint,the agent receives a constant stream of consumption and stops putting effort.Why must the agent retire when his continuation value reaches either endpoint?For the low retirement point,our assumption that the agent’s consumption utility is bounded from below implies that payments to the agent must stop when his value reaches the lower bound. On the other hand,when the agent’s continuation value becomes very high,although it is possible to keep the agent actively employed,retirement becomes optimal due to the income effect.When the agent’s consumption is high,it costs too much to compensate him for positive effort.Spear and Wang(2005)provide similar intuition in a two-period model.This intuition alone does not imply eventual retirement.There are many contracts,in which the agent suspends effort only temporarily and never retires.However,our results imply that those contracts are suboptimal.In the optimal contract,the agent’s continuation value has a strictly positive volatility,which eventually drives the agent to retirement with probability1.3Of course,retirement and other dynamic properties of the optimal contract depend on the contractual environment.The agent cannot be forced to stop consuming at the low retirement point if he has acceptable outside opportunities.Then,the agent quits instead of retiring at the low endpoint.If the agent is replaceable,the principal hires a new agent when the old agent reaches retirement.The high retirement point may also be replaced with promotion,an event that allows the agent to gain greater human capital,and manage larger and more important projects with higher expected output.4The contractual envirnoment matters for the dynamics of the agent’s wages.We already to control volatility as well.Hellwig and Schmidt(2002)look at the conditions for a discrete-time principal-agent model to converge to the HM solution.See also Bolton and Harris(2001),Ou-yang(2003)and Detemple,Govindaraj and Loewenstein(2001)for further generalization and analysis of the HM setting.3Eventual retirement in the optimal contract depends on the assumption that the agent is equally patient as the principal.See DeMarzo and Sannikov(2006),Farhi and Werning(2006a)and Sleet and Yeltekin(2006)for examples where the agent is less patient than the principal.4I thank the editor Juuso Valimaki for encouraging me to investigate this possibility.mentioned that the drift of the agent’s continuation value always points in the direction where it is cheaper to create incentives.Since better outside options make it more difficult to motivate and retain the agent,it is not surprising that wages become more backloaded with better outside options.Lower payments up front cause the agent’s continuation value to drift up,away from the point where he is forced to quit.When the employer can offer better promotion opportunities,the agent’s wages also become backloaded in the optimal contract.The agent is willing to work for lower wages up front when he is motivated by future promotions.Thesefindings highlight the factors that may affect the agent’s wage structure in internal labor markets.What matters more for the agent’s incentives:immediate outcomes or those in distant future?Contracts in practice use both short-term incentives,as piecework and bonuses, and long-term ones,as promotions and permanent wage increases.In our model,the ratio of the volatilities of the agent’s consumption and his continuation value measures the mix of short-term and long-term incentives.Wefind that the optimal contract uses stronger short-term incentives when the agent has better outside options,which interfere with the agent’s incentives in the long run.In contrast,when the principal has greaterflexibility to replace or promote the agent,the optimal contract uses stronger long-term incentives and keeps the agent’s wages more constant in the short run.Wefind that the agent puts higher effort and the principal gets greater profit when the optimal contract relies on stronger long-term incentives.It is difficult to study these dynamic properties of the optimal contract using discrete-time models.Without theflexibility of the differential equation that describes the dynamics of the optimal contract in continous time,traditional discrete-time models produce a more limited set of results.Spear and Srivastava(1987)show that how to analyze dynamic principal-agent problems in discrete time using recursive methods,with the agent’s contin-uation value as a state variable.5Assuming that the agent’s consumption is nonnegative and that his utility is separable in consumption and effort,that paper shows that inverse of the agent’s marginal utility of consumption is a martingale.An earlier paper of Rogerson (1985)demonstrates this result on a two-period model.6However,this restriction is not very informative about the optimal path of the agent’s wages,since a great diversity of 5Abreu,Pearce and Stacchetti(1986)and(1990)study the recursive structure of general repeated games.6This condition,called the inverse Euler equation,has received a lot of attention in recent macroeco-nomics literature.For example,see Golosov,Kocherlakota and Tsyvinski(2003)and Farhi and Werning (2006b).consumption profiles in different contractual environments we study satisfy this restriction.In its early stage,this paper was inspired by Phelan and Townsend(1991)who develop a method for computing optimal long-term contracts in discrete time.There are strong similarities between our continuous-time solutions and their discrete-time example,imply-ing that ultimately the two approaches are different ways of looking at the same thing. Their computational method relies on linear programming and multiple iterations to con-verge to the principal’s value function.While their method is quiteflexible and applicable to a wide range of settings,it is far more computationally intensive than our method of solving a differential equation.Because general discrete-time models are difficult to deal with,one may be tempted to restrict attention to the special tractable case when the agent is patient.As the agent’s discount rate converges to0,efficiency becomes attainable,as shown in Radner(1985)and Fudenberg,Holmstrom and Milgrom(1990).7However,wefind that optimal contracts when the agent is patient do not deliver many important dynamic insights:the agent’s continuation value becomes driftless,and the agent’s effort,determined without taking the cost of incentives into account,is decreasing in the agent’s value.Concurrently with this paper,Williams(2003)also develops a continuous-time principal-agent model.The aim of that paper is to present a general characterization of the optimal contract using a partial differential equation and forward and backward stochastic differ-ential equations.The resulting contract is written recursively using several state variables, but due to greater generality,the optimal contract is not explored in as much detail.More recently,a number of other papers started using continuous-time methodology. DeMarzo and Sannikov(2006)study the optimal contract in a cash-flow deversion model using the methods from this paper.Biais et al.(2006)show that the contract of DeMarzo and Sannikov(2006)arises in the limit of discrete-time models as the agent’s actions become more frequent.Cvitanic,Wan and Zhang(2006)study optimal contracts when the agent gets paid once,and Westerfield(2006)develops an approach that uses the agent’s wealth, as opposed to his continuation value,as a state variable.The paper is organized as follows.Section2presents the benchmark setting and for-mulates the principal’s problem.Section3presents an optimal contract and discusses its properties:the agent’s effort and consumption,the drift and volatility of his continuation value and retirement points.The formal derivation of the optimal contract is deferred to the Appendix.Section4explores how the agent’s outside options and the possibilities for 7Also,Fudenberg,Levine and Maskin(1994)prove a Folk Theorem for general repeated games.replacement and promotion affect the dynamics of the agent’s wages,effort and incentives. Section5studies optimal contracts for small discount rates.Section6concludes the paper.2The Benchmark Setting.Consider the following dynamic principal-agent model in continuous time.A standard Brownian motion Z={Z t,F t;0≤t<∞}on(Ω,F,Q)drives the output process.The total output X t produced up to time t evolves according todX t=A t dt+σdZ t,where A t is the agent’s choice of effort level andσis a constant.The agent’s effort is a stochastic process A={A t∈A,0≤t<∞}progressively measurable with respect to F t, where the set of feasible effort levels A is compact with the smallest element0.The agent experiences cost of effort v(A t),measured in the same units as the utility of consumption, where v:A→ is continuous,increasing and convex.We normalize v(0)=0and assume that there isγ0>0such that v(a)≥γ0a for all a∈A.The output process X is publicly observable by both the principal and the agent.The principal does not observe the agent’s effort A,and uses the observations of X to give the agent incentives to make costly effort.Before the agent starts working for the principal,the principal offers him a contract that specifies a nonnegativeflow of consumption C t(X s;0≤s≤t)∈[0,∞)based on the principal’s observation of output.The principal can commit to any such contract.We assume that the agent’s utility is bounded from below and normalize u(0)=0.Also,we assume that u:[0,∞)→[0,∞)is an increasing,concave and C2function that satisfies u (c)→0as c→∞.For simplicity,assume that both the principal and the agent discount theflow of profit and utility at a common rate r.If the agent chooses effort level A t,0≤t<∞,his average expected utility is given byE r ∞0e−rt(u(C t)−v(A t))dt ,and the principal gets average profitE r ∞0e−rt dX t−r ∞0e−rt C t dt =E r ∞0e−rt(A t−C t)dt .Factor r in front of the integrals normalizes total payoffs to the same scale asflow payoffs.We say that an effort process{A t,0≤t<∞}is incentive compatible with respect to {C t,0≤t<∞}if it maximizes the agent’s total expected utility.2.1The Formulation of The Principal’s Problem.The principal’s problem is to offer a contract to the agent:a stream of consumption {C t,0≤t<∞}contingent on the realized output and an incentive-compatible advice of effort{A t,0≤t<∞}that maximizes the principal’s profitE r ∞0e−rt(A t−C t)dt (1) subject to delivering to the agent a required initial value of at leastˆWE r ∞0e−rt(u(C t)−v(A t))dt ≥ˆW.(2) We assume that the principal can choose not to employ the agent,so we are only interested in contracts that generate nonnegative profit for the principal.3The Optimal Contract.This section describes the optimal contract for the benchmark model and discusses its basic properties.Appendix A provides the formal derivation of the optimal contract.It turns out that under the optimal contract the principal retires the agent after some paths of output by giving the agent a constant stream of payments in return for zero effort. The principal’s retirement profit as a function of the agent’s value F0:[0,u(∞))→(−∞,0] is given byF0(u(c))=−c,where u(∞)=lim c→∞u(c)can be infinity or afinite number.Even though retiring the agent may appear wasteful,wefind that under the optimal contract the agent ends up in retirement infinite time with probability1.88While in our model the agent keeps producing output with zero drift after retirement,it may be more natural to think that instead the principal shuts down thefirm.Analytically,the two possibilities are equivalent.Defineγ(a)=min{y∈[0,∞):a∈arg maxa∈Aya−v(a)}(3) The optimal contract is characterized by the unique concave solution F≥F0of the HJB equationF (W)=mina>0,c F(W)−a+c−F (W)(W−u(c)+v(a))rσ2γ(a)2/2(4)that satisfies the boundary conditionsF(0)=0,F(W gp)=F0(W gp)and F (W gp)=F 0(W gp)(5) for some W gp≥0,where F (W gp)=F 0(W gp)is called the smooth-pasting condition.9Let functions c:(0,W gp)→0and a:(0,W gp)→0be the minimizers in equation(4)on (0,W gp).A typical form of the value function F(0)together with a(W),c(W)and the drift of the agent’s continuation value(see below)are shown in Figure1.Theorem1,which is proved in Appendix A,characterizes optimal contracts.Theorem1.The unique concave function F≥F0that satisfies(4)and(5)character-izes any optimal contract with positive profit to the principal.For the agent’s starting value of W0>W gp,F(W0)<0is an upper bound on the principal’s profit.If W0∈[0,W gp], then the optimal contract attains profit F(W0).Such contract is based on the agent’s continuation value as a state variable,which starts at W0and evolves according todW t=r(W t−u(C t)+v(A t))dt+rγ(A t)(dX t−A t dt)(6) under payments C t=c(W t)and effort A t=a(W t),until the retirement timeτ.Retirement occurs when W t hits0or W gp for thefirst time.After retirement the agent gets constant consumption of−F0(Wτ)and puts effort0.The agent’s continuation value is his future expected payoffafter a given history of output.The intuition why the agent’s continuation value W t completely summarizes the past history in the optimal contract is the same as in discrete time.The agent’s incentives are unchanged if we replace the continuation contract that follows a given history with a9If r is sufficiently large,then the solution of(4)with boundary conditions F(0)=0and F (0)=F(0) satisfies F(W)>F0(W)for all W>0.In this case W gp=0and contracts with positive profit do not exist.-0.2-0.4-0.6-0.8√c,v(a)=0.5a2+0.4a,r=0.1andσ=1.Point W∗is Figure1:Function F for u(c)=the maximum of F.different contract that has the same continuation value W t.10Therefore,to maximize the principal’s profit after any history,the continuation contract must be optimal given W t. It follows that the agent’s continuation value W t completely determines the continuation contract.Equation(6)that describes the evolution of W t satisfies three objectives:incentives, promise keeping and profit maximization.The agent’s incentives arise from the sensitivity rγ(a)of his promised value towards output.Intuitively,it is optimal for the agent to choose the effort level a that maximizes the expected change of his promised value due to effort 10This logic would fail if the agent had hidden savings.With hidden savings,the agent’s past incentives to save depend not only on his current promised value,but also on how his value would change with savings level.Therefore,the problem with hidden savings has a different recursive structure,as discussed in the conclusions.minus the cost of effortr(γ(a)a−v(a)),where a is the drift of output when the agent chooses effort a.Functionγ(a)defined by (3)motivates the agent to put effort a with minimal volatility of continuation values.Due to the concavity of the principal’s profit,it is optimal to expose agent to the least amount of risk for a given effort level.Functionγ(a)is increasing in a.For the binary action case A={0,a},γ(a)=v(a)/a.When A is an interval and v is a differentiable function,γ(a)=v (a)for a>0.When the agent takes the recommended effort A t,then the drift r(W t−u(C t)+v(A t)) of the agent’s continuation value accounts for promise keeping.In order for W t to correctly describe the principal’s debt to the agent,in expectation W t grows at the interest rate r and fall due to theflow of repayments r(u(C t)−v(A t)).The HJB equation,which is the continuous-time version of the Bellman equation,deliv-ers the optimal choice of payments C t=c(W t)and recommendations of effort A t=a(W t). Equation(4),which is suitable for computation because it isolates F (W)on the left hand side,follows from the standard formrF(W)=maxa,c r(a−c)+r(W−u(c)+v(a))F (W)+r2σ2γ(a)2F (W)2(7)This equation determines a(W)and c(W)by maximizing the sum of the principal’s current expected profitflow r(a−c)and the expected change of his future profit due to the drift and volatility of the agent’s continuation value.Together,they add up to the annuity value of total profit rF(W).The optimal effort maximizesra+rv(a)F (W)+r2σ2γ(a)2F (W)2,(8)where ra is the expectedflow of output,−rv(a)F (W)is the cost of compensating the agent for his effort,and−r2σ2γ(a)2F (W)is the cost of exposing the agent to income uncertainty to provide incentives.These two costs typically work in opposite directions,creating a complex effort profile(see Figure1).While F (W)decreases in W because F is concave, F (W)tends increases over some ranges of W.11However,as r→0,the cost of exposing11F (W)increases at least on the interval[0,W∗],where c=0and sign F (W)=sign(rW−u(c)+ v(a))>0(see Theorem2).When W is smaller,the principal faces a greater risk of triggering retirementthe agent to risk goes away and the effort profile becomes decreasing in W,except possibly near endpoints0and W gp(see Section5).The optimal choice of consumption maximizes−c−F (W)u(c).(9)Thus,the agent’s consumption is0when F (W)≥−1/u (0),in the probationary interval [0,W∗∗],and it is increasing in W according to F (W)=−1/u (c)above W∗∗.Intuitively, 1/u (c)and−F (W)are the marginal costs of giving the agent value through current con-sumption and through his continuation payoff,respectively.Those marginal costs must be equal under the optimal contract,except in the probationary interval.There,consumption zero is optimal because it maximizes the drift of W t away from the inefficient low retirement point.The drift of W t is connected with the allocation of the agent’s wages over time.Section 5shows that the drift of W t becomes zero when the agent become patient,to minimize intertemporal distortions of the agent’s consumption.In general,the drift of W t is nonzero in the optimal contract.Theorem2shows that the drift of W t always points in the direction where it is cheaper to provide incentives.Theorem2.Until retirement,the drift of the agent’s continuation value points in the direction in which F (W)is increasing.Proof.From(7)and the Envelope Theorem,we haver(W−u(c)+v(a))F (W)+r2σ2γ(a)2F (W)2=0(10)Since F (W)is always negative,W−u(c)+v(a)has the same sign as F (W).QEDBy Ito’s lemma,(10)is the drift of−1/u (C t)=F (W t)on[W∗∗,W gp].Thus,in our model the inverse of the agent’s marginal utility is a martingale when the agent’s consump-tion is positive.The analogous result in discrete time wasfirst discovered by Rogerson (1985).Under the optimal contract the agent keeps putting effort at all times until he is even-tually retired when W t reaches0or W gp.The principal must retire the agent when W t hits 0because the only way to deliver to the agent value0is to pay him0forever.Indeed,if the future payments were not always0,the agent can guarantee himself a strictly positive value by providing stronger incentives.by putting effort0.Why is it optimal for the principal to retire agent if his continuation payoffbecomes sufficiently large?This happens due to the income effect:when theflow of payments to the agent is large enough,it costs the principal too much to compensate the agent for his effort,so it is optimal to allow effort0.When the agent gets richer, the monetary cost of delivering utility to the agent rises indefinitely(since u (c)→0as c→∞)while the utility cost of output stays bounded above0since v(a)≥γ0a for all a. High retirement occurs even before the cost of compensating the agent for effort exceeds the expected output from effort,since the principal must compensate the agent not only for effort,but also for risk(see(8)).While it is necessary to retire the agent when W t hits 0and it is optimal to do so if W t reaches W gp,there are contracts that prevent W t from reaching0or W gp by allowing the agent to suspend effort temporarily.Those contracts are suboptimal:in the optimal contract the agent puts positive effort until he is retired forever.12In the next subsection we discuss the paths of the agent’s continuation value and income, and the connections between the agent’s incentives,productivity and income distribution in the example in Figure1.Before that,we make three remarks about possible extensions of our model.Remark1:Retirement.If the agent’s utility was unbounded from below(e.g.expo-nential utility),our differential equation would still characterize the optimal contract,but the agent may never reach retirement at the low endpoint.To take care of this possibility, the boundary condition F(0)=0would need to be replaced with a regularity condition on the asymptotic behavior of F.Of course,the low retirement point does not disappear if the agent has an outside option at all times(see Section4).Similarly,if the agent’s utility had no income effect,the high retirement point may disappear as well.This would be the case if we assumed exponential utility with a monetary cost of effort,as in Holmstrom and Milgrom(1987).13Remark2:Savings.We assume in this model that the agent cannot save or borrow, and is restricted to consume what the principal pays him at every moment of time.What 12This conclusion depends on the assumption that the agent’s discount rate is the same as that of the principal.If the agent’s discount rate was higher,the optimal contract may allow the agent to suspend effort temporarily.13DeMarzo and Sannikov(2006)study a dynamic agency problem without the income effect.In their setting the moral hazard problem is that the agent may secretly divert cash from thefirm,so his benefit from the hidden action is measured in monetary terms.The optimal contract has a low absorbing state, since the agent’s utility is bounded from below,but no upper absorbing state.。
my model的英语作文
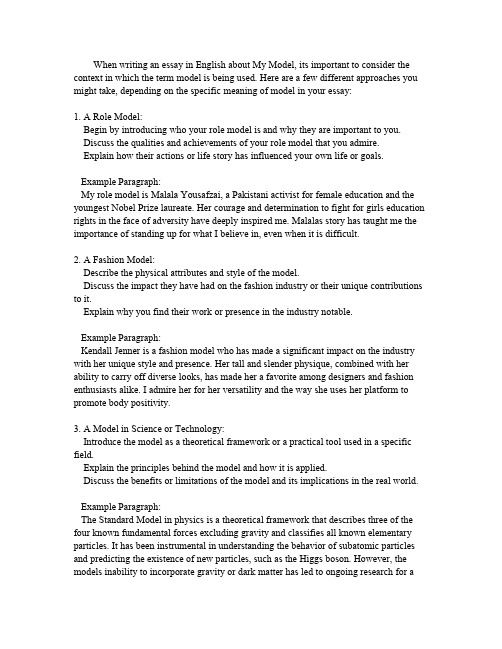
When writing an essay in English about My Model,its important to consider the context in which the term model is being used.Here are a few different approaches you might take,depending on the specific meaning of model in your essay:1.A Role Model:Begin by introducing who your role model is and why they are important to you. Discuss the qualities and achievements of your role model that you admire. Explain how their actions or life story has influenced your own life or goals.Example Paragraph:My role model is Malala Yousafzai,a Pakistani activist for female education and the youngest Nobel Prize laureate.Her courage and determination to fight for girls education rights in the face of adversity have deeply inspired me.Malalas story has taught me the importance of standing up for what I believe in,even when it is difficult.2.A Fashion Model:Describe the physical attributes and style of the model.Discuss the impact they have had on the fashion industry or their unique contributions to it.Explain why you find their work or presence in the industry notable.Example Paragraph:Kendall Jenner is a fashion model who has made a significant impact on the industry with her unique style and presence.Her tall and slender physique,combined with her ability to carry off diverse looks,has made her a favorite among designers and fashion enthusiasts alike.I admire her for her versatility and the way she uses her platform to promote body positivity.3.A Model in Science or Technology:Introduce the model as a theoretical framework or a practical tool used in a specific field.Explain the principles behind the model and how it is applied.Discuss the benefits or limitations of the model and its implications in the real world.Example Paragraph:The Standard Model in physics is a theoretical framework that describes three of the four known fundamental forces excluding gravity and classifies all known elementary particles.It has been instrumental in understanding the behavior of subatomic particles and predicting the existence of new particles,such as the Higgs boson.However,the models inability to incorporate gravity or dark matter has led to ongoing research for amore comprehensive theory.4.A Model in Business or Economics:Introduce the business or economic model and its purpose.Explain how the model works and the strategies it employs.Discuss the success or challenges associated with the model and its potential for future growth.Example Paragraph:The subscriptionbased business model has become increasingly popular in recent years, particularly in the software panies like Adobe have transitioned from selling packaged software to offering services on a subscription basis,allowing for continuous revenue streams and a more predictable income.This model has been successful in fostering customer loyalty and providing a steady income,although it requires ongoing innovation to maintain customer interest.5.A Model in Art or Design:Describe the aesthetic or functional qualities of the model.Discuss the creative process or design principles that inform the model.Explain the cultural or historical significance of the model and its influence on contemporary art or design.Example Paragraph:The Eames Lounge Chair,designed by Charles and Ray Eames,is a model of modern furniture that has become an icon of midcentury design.Its elegant form,made from molded plywood and leather,exemplifies the designers commitment to blending comfort with aesthetics.The chairs timeless appeal has made it a staple in both residential and commercial settings,influencing countless furniture designs that followed. Remember to structure your essay with a clear introduction,body paragraphs that develop your points,and a conclusion that summarizes your main e specific examples and evidence to support your claims,and ensure your writing is clear,concise, and engaging.。
高考英语阅读理解态度题单选题30题
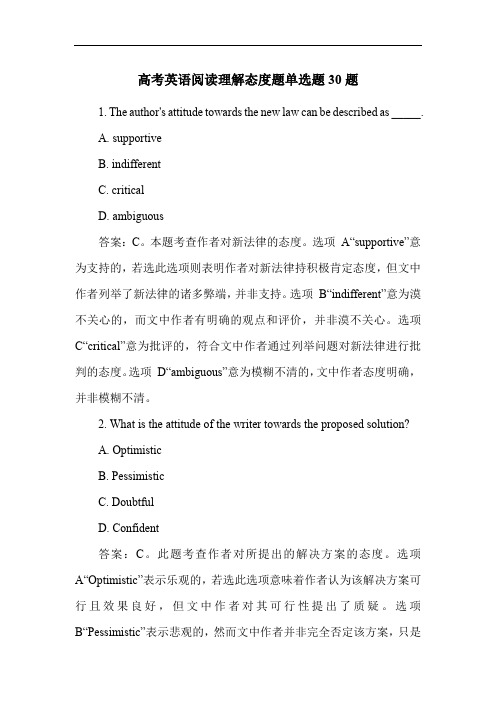
高考英语阅读理解态度题单选题30题1. The author's attitude towards the new law can be described as _____.A. supportiveB. indifferentC. criticalD. ambiguous答案:C。
本题考查作者对新法律的态度。
选项A“supportive”意为支持的,若选此选项则表明作者对新法律持积极肯定态度,但文中作者列举了新法律的诸多弊端,并非支持。
选项B“indifferent”意为漠不关心的,而文中作者有明确的观点和评价,并非漠不关心。
选项C“critical”意为批评的,符合文中作者通过列举问题对新法律进行批判的态度。
选项D“ambiguous”意为模糊不清的,文中作者态度明确,并非模糊不清。
2. What is the attitude of the writer towards the proposed solution?A. OptimisticB. PessimisticC. DoubtfulD. Confident答案:C。
此题考查作者对所提出的解决方案的态度。
选项A“Optimistic”表示乐观的,若选此选项意味着作者认为该解决方案可行且效果良好,但文中作者对其可行性提出了质疑。
选项B“Pessimistic”表示悲观的,然而文中作者并非完全否定该方案,只是存在怀疑。
选项C“Doubtful”意为怀疑的,符合文中作者对方案的态度,作者在文中指出了方案可能存在的问题和不确定性。
选项D“Confident”表示自信的,与文中作者的态度不符。
3. The tone of the passage when referring to the recent development is _____.A. excitedB. cautiousC. enthusiasticD. worried答案:B。
Kernel methods in machine learning

a rX iv:mat h /7197v3[mat h.ST]1J ul28The Annals of Statistics 2008,Vol.36,No.3,1171–1220DOI:10.1214/009053607000000677c Institute of Mathematical Statistics ,2008KERNEL METHODS IN MACHINE LEARNING 1By Thomas Hofmann,Bernhard Sch ¨o lkopf and Alexander J.Smola Darmstadt University of Technology ,Max Planck Institute for Biological Cybernetics and National ICT Australia We review machine learning methods employing positive definite kernels.These methods formulate learning and estimation problems in a reproducing kernel Hilbert space (RKHS)of functions defined on the data domain,expanded in terms of a kernel.Working in linear spaces of function has the benefit of facilitating the construction and analysis of learning algorithms while at the same time allowing large classes of functions.The latter include nonlinear functions as well as functions defined on nonvectorial data.We cover a wide range of methods,ranging from binary classifiers to sophisticated methods for estimation with structured data.1.Introduction.Over the last ten years estimation and learning meth-ods utilizing positive definite kernels have become rather popular,particu-larly in machine learning.Since these methods have a stronger mathematical slant than earlier machine learning methods (e.g.,neural networks),there is also significant interest in the statistics and mathematics community for these methods.The present review aims to summarize the state of the art on a conceptual level.In doing so,we build on various sources,including Burges [25],Cristianini and Shawe-Taylor [37],Herbrich [64]and Vapnik [141]and,in particular,Sch¨o lkopf and Smola [118],but we also add a fair amount of more recent material which helps unifying the exposition.We have not had space to include proofs;they can be found either in the long version of the present paper (see Hofmann et al.[69]),in the references given or in the above books.The main idea of all the described methods can be summarized in one paragraph.Traditionally,theory and algorithms of machine learning and2T.HOFMANN,B.SCH¨OLKOPF AND A.J.SMOLAstatistics has been very well developed for the linear case.Real world data analysis problems,on the other hand,often require nonlinear methods to de-tect the kind of dependencies that allow successful prediction of properties of interest.By using a positive definite kernel,one can sometimes have the best of both worlds.The kernel corresponds to a dot product in a(usually high-dimensional)feature space.In this space,our estimation methods are linear,but as long as we can formulate everything in terms of kernel evalu-ations,we never explicitly have to compute in the high-dimensional feature space.The paper has three main sections:Section2deals with fundamental properties of kernels,with special emphasis on(conditionally)positive defi-nite kernels and their characterization.We give concrete examples for such kernels and discuss kernels and reproducing kernel Hilbert spaces in the con-text of regularization.Section3presents various approaches for estimating dependencies and analyzing data that make use of kernels.We provide an overview of the problem formulations as well as their solution using convex programming techniques.Finally,Section4examines the use of reproduc-ing kernel Hilbert spaces as a means to define statistical models,the focus being on structured,multidimensional responses.We also show how such techniques can be combined with Markov networks as a suitable framework to model dependencies between response variables.2.Kernels.2.1.An introductory example.Suppose we are given empirical data (1)(x1,y1),...,(x n,y n)∈X×Y.Here,the domain X is some nonempty set that the inputs(the predictor variables)x i are taken from;the y i∈Y are called targets(the response vari-able).Here and below,i,j∈[n],where we use the notation[n]:={1,...,n}. Note that we have not made any assumptions on the domain X other than it being a set.In order to study the problem of learning,we need additional structure.In learning,we want to be able to generalize to unseen data points.In the case of binary pattern recognition,given some new input x∈X,we want to predict the corresponding y∈{±1}(more complex output domains Y will be treated below).Loosely speaking,we want to choose y such that(x,y)is in some sense similar to the training examples.To this end,we need similarity measures in X and in{±1}.The latter is easier, as two target values can only be identical or different.For the former,we require a function(2)k:X×X→R,(x,x′)→k(x,x′)KERNEL METHODS IN MACHINE LEARNING3Fig. 1.A simple geometric classification algorithm:given two classes of points(de-picted by“o”and“+”),compute their means c+,c−and assign a test input x to the one whose mean is closer.This can be done by looking at the dot product between x−c [where c=(c++c−)/2]and w:=c+−c−,which changes sign as the enclosed angle passes throughπ/2.Note that the corresponding decision boundary is a hyperplane(the dotted line)orthogonal to w(from Sch¨o lkopf and Smola[118]).satisfying,for all x,x′∈X,k(x,x′)= Φ(x),Φ(x′) ,(3)whereΦmaps into some dot product space H,sometimes called the featurespace.The similarity measure k is usually called a kernel,andΦis called its feature map.The advantage of using such a kernel as a similarity measure is that it allows us to construct algorithms in dot product spaces.For instance, consider the following simple classification algorithm,described in Figure1, where Y={±1}.The idea is to compute the means of the two classes inthe feature space,c+=1n− {i:y i=−1}Φ(x i), where n+and n−are the number of examples with positive and negative target values,respectively.We then assign a new pointΦ(x)to the class whose mean is closer to it.This leads to the prediction ruley=sgn( Φ(x),c+ − Φ(x),c− +b)(4)with b=1n+ {i:y i=+1} Φ(x),Φ(x i)k(x,x i)−12(1n2+ {(i,j):y i=y j=+1}k(x i,x j)).Let us consider one well-known special case of this type of classifier.As-sume that the class means have the same distance to the origin(hence, b=0),and that k(·,x)is a density for all x∈X.If the two classes are4T.HOFMANN,B.SCH¨OLKOPF AND A.J.SMOLAequally likely and were generated from two probability distributions that are estimatedp+(x):=1n− {i:y i=−1}k(x,x i),(6)then(5)is the estimated Bayes decision rule,plugging in the estimates p+ and p−for the true densities.The classifier(5)is closely related to the Support Vector Machine(SVM) that we will discuss below.It is linear in the feature space(4),while in the input domain,it is represented by a kernel expansion(5).In both cases,the decision boundary is a hyperplane in the feature space;however,the normal vectors[for(4),w=c+−c−]are usually rather different.The normal vector not only characterizes the alignment of the hyperplane, its length can also be used to construct tests for the equality of the two class-generating distributions(Borgwardt et al.[22]).As an aside,note that if we normalize the targets such thatˆy i=y i/|{j:y j= y i}|,in which case theˆy i sum to zero,then w 2= K,ˆyˆy⊤ F,where ·,· F is the Frobenius dot product.If the two classes have equal size,then up to a scaling factor involving K 2and n,this equals the kernel-target alignment defined by Cristianini et al.[38].2.2.Positive definite kernels.We have required that a kernel satisfy(3), that is,correspond to a dot product in some dot product space.In the present section we show that the class of kernels that can be written in the form(3)coincides with the class of positive definite kernels.This has far-reaching consequences.There are examples of positive definite kernels which can be evaluated efficiently even though they correspond to dot products in infinite dimensional dot product spaces.In such cases,substituting k(x,x′) for Φ(x),Φ(x′) ,as we have done in(5),is crucial.In the machine learning community,this substitution is called the kernel trick.Definition1(Gram matrix).Given a kernel k and inputs x1,...,x n∈X,the n×n matrixK:=(k(x i,x j))ij(7)is called the Gram matrix(or kernel matrix)of k with respect to x1,...,x n.Definition2(Positive definite matrix).A real n×n symmetric matrix K ij satisfyingi,j c i c j K ij≥0(8)for all c i∈R is called positive definite.If equality in(8)only occurs for c1=···=c n=0,then we shall call the matrix strictly positive definite.KERNEL METHODS IN MACHINE LEARNING 5Definition 3(Positive definite kernel).Let X be a nonempty set.A function k :X ×X →R which for all n ∈N ,x i ∈X ,i ∈[n ]gives rise to a positive definite Gram matrix is called a positive definite kernel .A function k :X ×X →R which for all n ∈N and distinct x i ∈X gives rise to a strictly positive definite Gram matrix is called a strictly positive definite kernel .Occasionally,we shall refer to positive definite kernels simply as kernels .Note that,for simplicity,we have restricted ourselves to the case of real valued kernels.However,with small changes,the below will also hold for the complex valued case.Since i,j c i c j Φ(x i ),Φ(x j ) = i c i Φ(x i ), j c j Φ(x j ) ≥0,kernels of the form (3)are positive definite for any choice of Φ.In particular,if X is already a dot product space,we may choose Φto be the identity.Kernels can thus be regarded as generalized dot products.While they are not generally bilinear,they share important properties with dot products,such as the Cauchy–Schwarz inequality:If k is a positive definite kernel,and x 1,x 2∈X ,thenk (x 1,x 2)2≤k (x 1,x 1)·k (x 2,x 2).(9)2.2.1.Construction of the reproducing kernel Hilbert space.We now de-fine a map from X into the space of functions mapping X into R ,denoted as R X ,viaΦ:X →R X where x →k (·,x ).(10)Here,Φ(x )=k (·,x )denotes the function that assigns the value k (x ′,x )to x ′∈X .We next construct a dot product space containing the images of the inputs under Φ.To this end,we first turn it into a vector space by forming linear combinationsf (·)=n i =1αi k (·,x i ).(11)Here,n ∈N ,αi ∈R and x i ∈X are arbitrary.Next,we define a dot product between f and another function g (·)= n ′j =1βj k (·,x ′j )(with n ′∈N ,βj ∈R and x ′j ∈X )asf,g :=n i =1n ′j =1αi βj k (x i ,x ′j ).(12)To see that this is well defined although it contains the expansion coefficients and points,note that f,g = n ′j =1βj f (x ′j ).The latter,however,does not depend on the particular expansion of f .Similarly,for g ,note that f,g = n i =1αi g (x i ).This also shows that ·,· is bilinear.It is symmetric,as f,g =6T.HOFMANN,B.SCH¨OLKOPF AND A.J.SMOLAg,f .Moreover,it is positive definite,since positive definiteness of k implies that,for any function f,written as(11),we havef,f =ni,j=1αiαj k(x i,x j)≥0.(13)Next,note that given functions f1,...,f p,and coefficientsγ1,...,γp∈R,we havepi,j=1γiγj f i,f j = p i=1γi f i,p j=1γj f j ≥0.(14)Here,the equality follows from the bilinearity of ·,· ,and the right-hand inequality from(13).By(14), ·,· is a positive definite kernel,defined on our vector space of functions.For the last step in proving that it even is a dot product,we note that,by(12),for all functions(11),k(·,x),f =f(x)and,in particular, k(·,x),k(·,x′) =k(x,x′). (15)By virtue of these properties,k is called a reproducing kernel(Aronszajn [7]).Due to(15)and(9),we have|f(x)|2=| k(·,x),f |2≤k(x,x)· f,f .(16)By this inequality, f,f =0implies f=0,which is the last property that was left to prove in order to establish that ·,· is a dot product. Skipping some details,we add that one can complete the space of func-tions(11)in the norm corresponding to the dot product,and thus gets a Hilbert space H,called a reproducing kernel Hilbert space(RKHS).One can define a RKHS as a Hilbert space H of functions on a set X with the property that,for all x∈X and f∈H,the point evaluations f→f(x) are continuous linear functionals[in particular,all point values f(x)are well defined,which already distinguishes RKHSs from many L2Hilbert spaces]. From the point evaluation functional,one can then construct the reproduc-ing kernel using the Riesz representation theorem.The Moore–Aronszajn theorem(Aronszajn[7])states that,for every positive definite kernel on X×X,there exists a unique RKHS and vice versa.There is an analogue of the kernel trick for distances rather than dot products,that is,dissimilarities rather than similarities.This leads to the larger class of conditionally positive definite kernels.Those kernels are de-fined just like positive definite ones,with the one difference being that their Gram matrices need to satisfy(8)only subject toni=1c i=0.(17)KERNEL METHODS IN MACHINE LEARNING7 Interestingly,it turns out that many kernel algorithms,including SVMs and kernel PCA(see Section3),can be applied also with this larger class of kernels,due to their being translation invariant in feature space(Hein et al.[63]and Sch¨o lkopf and Smola[118]).We conclude this section with a note on terminology.In the early years of kernel machine learning research,it was not the notion of positive definite kernels that was being used.Instead,researchers considered kernels satis-fying the conditions of Mercer’s theorem(Mercer[99],see,e.g.,Cristianini and Shawe-Taylor[37]and Vapnik[141]).However,while all such kernels do satisfy(3),the converse is not true.Since(3)is what we are interested in, positive definite kernels are thus the right class of kernels to consider.2.2.2.Properties of positive definite kernels.We begin with some closure properties of the set of positive definite kernels.Proposition4.Below,k1,k2,...are arbitrary positive definite kernels on X×X,where X is a nonempty set:(i)The set of positive definite kernels is a closed convex cone,that is, (a)ifα1,α2≥0,thenα1k1+α2k2is positive definite;and(b)if k(x,x′):= lim n→∞k n(x,x′)exists for all x,x′,then k is positive definite.(ii)The pointwise product k1k2is positive definite.(iii)Assume that for i=1,2,k i is a positive definite kernel on X i×X i, where X i is a nonempty set.Then the tensor product k1⊗k2and the direct sum k1⊕k2are positive definite kernels on(X1×X2)×(X1×X2).The proofs can be found in Berg et al.[18].It is reassuring that sums and products of positive definite kernels are positive definite.We will now explain that,loosely speaking,there are no other operations that preserve positive definiteness.To this end,let C de-note the set of all functionsψ:R→R that map positive definite kernels to (conditionally)positive definite kernels(readers who are not interested in the case of conditionally positive definite kernels may ignore the term in parentheses).We defineC:={ψ|k is a p.d.kernel⇒ψ(k)is a(conditionally)p.d.kernel},C′={ψ|for any Hilbert space F,ψ( x,x′ F)is(conditionally)positive definite}, C′′={ψ|for all n∈N:K is a p.d.n×n matrix⇒ψ(K)is(conditionally)p.d.},whereψ(K)is the n×n matrix with elementsψ(K ij).8T.HOFMANN,B.SCH¨OLKOPF AND A.J.SMOLAProposition5.C=C′=C′′.The following proposition follows from a result of FitzGerald et al.[50]for (conditionally)positive definite matrices;by Proposition5,it also applies for (conditionally)positive definite kernels,and for functions of dot products. We state the latter case.Proposition6.Letψ:R→R.Thenψ( x,x′ F)is positive definite for any Hilbert space F if and only ifψis real entire of the formψ(t)=∞ n=0a n t n(18)with a n≥0for n≥0.Moreover,ψ( x,x′ F)is conditionally positive definite for any Hilbert space F if and only ifψis real entire of the form(18)with a n≥0for n≥1.There are further properties of k that can be read offthe coefficients a n:•Steinwart[128]showed that if all a n are strictly positive,then the ker-nel of Proposition6is universal on every compact subset S of R d in the sense that its RKHS is dense in the space of continuous functions on S in the · ∞norm.For support vector machines using universal kernels,he then shows(universal)consistency(Steinwart[129]).Examples of univer-sal kernels are(19)and(20)below.•In Lemma11we will show that the a0term does not affect an SVM. Hence,we infer that it is actually sufficient for consistency to have a n>0 for n≥1.We conclude the section with an example of a kernel which is positive definite by Proposition6.To this end,let X be a dot product space.The power series expansion ofψ(x)=e x then tells us thatk(x,x′)=e x,x′ /σ2(19)is positive definite(Haussler[62]).If we further multiply k with the positive definite kernel f(x)f(x′),where f(x)=e− x 2/2σ2andσ>0,this leads to the positive definiteness of the Gaussian kernelk′(x,x′)=k(x,x′)f(x)f(x′)=e− x−x′ 2/(2σ2).(20)KERNEL METHODS IN MACHINE LEARNING9 2.2.3.Properties of positive definite functions.We now let X=R d and consider positive definite kernels of the form(21)k(x,x′)=h(x−x′),in which case h is called a positive definite function.The following charac-terization is due to Bochner[21].We state it in the form given by Wendland [152].Theorem7.A continuous function h on R d is positive definite if and only if there exists afinite nonnegative Borel measureµon R d such thath(x)= R d e−i x,ω dµ(ω).(22)While normally formulated for complex valued functions,the theorem also holds true for real functions.Note,however,that if we start with an arbitrary nonnegative Borel measure,its Fourier transform may not be real. Real-valued positive definite functions are distinguished by the fact that the corresponding measuresµare symmetric.We may normalize h such that h(0)=1[hence,by(9),|h(x)|≤1],in which caseµis a probability measure and h is its characteristic function.For instance,ifµis a normal distribution of the form(2π/σ2)−d/2e−σ2 ω 2/2dω, then the corresponding positive definite function is the Gaussian e− x 2/(2σ2); see(20).Bochner’s theorem allows us to interpret the similarity measure k(x,x′)= h(x−x′)in the frequency domain.The choice of the measureµdetermines which frequency components occur in the kernel.Since the solutions of kernel algorithms will turn out to befinite kernel expansions,the measureµwill thus determine which frequencies occur in the estimates,that is,it will determine their regularization properties—more on that in Section2.3.2 below.Bochner’s theorem generalizes earlier work of Mathias,and has itself been generalized in various ways,that is,by Schoenberg[115].An important generalization considers Abelian semigroups(Berg et al.[18]).In that case, the theorem provides an integral representation of positive definite functions in terms of the semigroup’s semicharacters.Further generalizations were given by Krein,for the cases of positive definite kernels and functions with a limited number of negative squares.See Stewart[130]for further details and references.As above,there are conditions that ensure that the positive definiteness becomes strict.Proposition8(Wendland[152]).A positive definite function is strictly positive definite if the carrier of the measure in its representation(22)con-tains an open subset.10T.HOFMANN,B.SCH¨OLKOPF AND A.J.SMOLAThis implies that the Gaussian kernel is strictly positive definite.An important special case of positive definite functions,which includes the Gaussian,are radial basis functions.These are functions that can be written as h(x)=g( x 2)for some function g:[0,∞[→R.They have the property of being invariant under the Euclidean group.2.2.4.Examples of kernels.We have already seen several instances of positive definite kernels,and now intend to complete our selection with a few more examples.In particular,we discuss polynomial kernels,convolution kernels,ANOVA expansions and kernels on documents.Polynomial kernels.From Proposition4it is clear that homogeneous poly-nomial kernels k(x,x′)= x,x′ p are positive definite for p∈N and x,x′∈R d. By direct calculation,we can derive the corresponding feature map(Poggio [108]):x,x′ p= d j=1[x]j[x′]j p(23)= j∈[d]p[x]j1·····[x]j p·[x′]j1·····[x′]j p= C p(x),C p(x′) ,where C p maps x∈R d to the vector C p(x)whose entries are all possible p th degree ordered products of the entries of x(note that[d]is used as a shorthand for{1,...,d}).The polynomial kernel of degree p thus computes a dot product in the space spanned by all monomials of degree p in the input coordinates.Other useful kernels include the inhomogeneous polynomial, (24)k(x,x′)=( x,x′ +c)p where p∈N and c≥0,which computes all monomials up to degree p.Spline kernels.It is possible to obtain spline functions as a result of kernel expansions(Vapnik et al.[144]simply by noting that convolution of an even number of indicator functions yields a positive kernel function.Denote by I X the indicator(or characteristic)function on the set X,and denote by ⊗the convolution operation,(f⊗g)(x):= R d f(x′)g(x′−x)dx′.Then the B-spline kernels are given by(25)k(x,x′)=B2p+1(x−x′)where p∈N with B i+1:=B i⊗B0.Here B0is the characteristic function on the unit ball in R d.From the definition of(25),it is obvious that,for odd m,we may write B m as the inner product between functions B m/2.Moreover,note that,for even m,B m is not a kernel.KERNEL METHODS IN MACHINE LEARNING11 Convolutions and structures.Let us now move to kernels defined on struc-tured objects(Haussler[62]and Watkins[151]).Suppose the object x∈X is composed of x p∈X p,where p∈[P](note that the sets X p need not be equal). For instance,consider the string x=AT G and P=2.It is composed of the parts x1=AT and x2=G,or alternatively,of x1=A and x2=T G.Math-ematically speaking,the set of“allowed”decompositions can be thought of as a relation R(x1,...,x P,x),to be read as“x1,...,x P constitute the composite object x.”Haussler[62]investigated how to define a kernel between composite ob-jects by building on similarity measures that assess their respective parts; in other words,kernels k p defined on X p×X p.Define the R-convolution of k1,...,k P as[k1⋆···⋆k P](x,x′):=¯x∈R(x),¯x′∈R(x′)P p=1k p(¯x p,¯x′p),(26)where the sum runs over all possible ways R(x)and R(x′)in which we can decompose x into¯x1,...,¯x P and x′analogously[here we used the con-vention that an empty sum equals zero,hence,if either x or x′cannot be decomposed,then(k1⋆···⋆k P)(x,x′)=0].If there is only afinite number of ways,the relation R is calledfinite.In this case,it can be shown that the R-convolution is a valid kernel(Haussler[62]).ANOVA kernels.Specific examples of convolution kernels are Gaussians and ANOVA kernels(Vapnik[141]and Wahba[148]).To construct an ANOVA kernel,we consider X=S N for some set S,and kernels k(i)on S×S,where i=1,...,N.For P=1,...,N,the ANOVA kernel of order P is defined as k P(x,x′):= 1≤i1<···<i P≤N P p=1k(i p)(x i p,x′i p).(27)Note that if P=N,the sum consists only of the term for which(i1,...,i P)= (1,...,N),and k equals the tensor product k(1)⊗···⊗k(N).At the other extreme,if P=1,then the products collapse to one factor each,and k equals the direct sum k(1)⊕···⊕k(N).For intermediate values of P,we get kernels that lie in between tensor products and direct sums.ANOVA kernels typically use some moderate value of P,which specifiesthe order of the interactions between attributes x ip that we are interestedin.The sum then runs over the numerous terms that take into account interactions of order P;fortunately,the computational cost can be reduced to O(P d)cost by utilizing recurrent procedures for the kernel evaluation. ANOVA kernels have been shown to work rather well in multi-dimensional SV regression problems(Stitson et al.[131]).12T.HOFMANN,B.SCH¨OLKOPF AND A.J.SMOLABag of words.One way in which SVMs have been used for text categoriza-tion(Joachims[77])is the bag-of-words representation.This maps a given text to a sparse vector,where each component corresponds to a word,and a component is set to one(or some other number)whenever the related word occurs in the ing an efficient sparse representation,the dot product between two such vectors can be computed quickly.Furthermore, this dot product is by construction a valid kernel,referred to as a sparse vector kernel.One of its shortcomings,however,is that it does not take into account the word ordering of a document.Other sparse vector kernels are also conceivable,such as one that maps a text to the set of pairs of words that are in the same sentence(Joachims[77]and Watkins[151]).n-grams and suffix trees.A more sophisticated way of dealing with string data was proposed by Haussler[62]and Watkins[151].The basic idea is as described above for general structured objects(26):Compare the strings by means of the substrings they contain.The more substrings two strings have in common,the more similar they are.The substrings need not always be contiguous;that said,the further apart thefirst and last element of a substring are,the less weight should be given to the similarity.Depending on the specific choice of a similarity measure,it is possible to define more or less efficient kernels which compute the dot product in the feature space spanned by all substrings of documents.Consider afinite alphabetΣ,the set of all strings of length n,Σn,and the set of allfinite strings,Σ∗:= ∞n=0Σn.The length of a string s∈Σ∗is denoted by|s|,and its elements by s(1)...s(|s|);the concatenation of s and t∈Σ∗is written st.Denote byk(x,x′)= s#(x,s)#(x′,s)c sa string kernel computed from exact matches.Here#(x,s)is the number of occurrences of s in x and c s≥0.Vishwanathan and Smola[146]provide an algorithm using suffix trees, which allows one to compute for arbitrary c s the value of the kernel k(x,x′) in O(|x|+|x′|)time and memory.Moreover,also f(x)= w,Φ(x) can be computed in O(|x|)time if preprocessing linear in the size of the support vectors is carried out.These kernels are then applied to function prediction (according to the gene ontology)of proteins using only their sequence in-formation.Another prominent application of string kernels is in thefield of splice form prediction and genefinding(R¨a tsch et al.[112]).For inexact matches of a limited degree,typically up toǫ=3,and strings of bounded length,a similar data structure can be built by explicitly gener-ating a dictionary of strings and their neighborhood in terms of a Hamming distance(Leslie et al.[92]).These kernels are defined by replacing#(x,s)KERNEL METHODS IN MACHINE LEARNING13 by a mismatch function#(x,s,ǫ)which reports the number of approximate occurrences of s in x.By trading offcomputational complexity with storage (hence,the restriction to small numbers of mismatches),essentially linear-time algorithms can be designed.Whether a general purpose algorithm exists which allows for efficient comparisons of strings with mismatches in linear time is still an open question.Mismatch kernels.In the general case it is only possible tofind algorithms whose complexity is linear in the lengths of the documents being compared, and the length of the substrings,that is,O(|x|·|x′|)or worse.We now describe such a kernel with a specific choice of weights(Cristianini and Shawe-Taylor[37]and Watkins[151]).Let us now form subsequences u of strings.Given an index sequence i:= (i1,...,i|u|)with1≤i1<···<i|u|≤|s|,we define u:=s(i):=s(i1)...s(i|u|). We call l(i):=i|u|−i1+1the length of the subsequence in s.Note that if iis not contiguous,then l(i)>|u|.The feature space built from strings of length n is defined to be H n:=R(Σn).This notation means that the space has one dimension(or coordinate) for each element ofΣn,labeled by that element(equivalently,we can thinkof it as the space of all real-valued functions onΣn).We can thus describe the feature map coordinate-wise for each u∈Σn via(28)[Φn(s)]u:= i:s(i)=uλl(i).Here,0<λ≤1is a decay parameter:The larger the length of the subse-quence in s,the smaller the respective contribution to[Φn(s)]u.The sum runs over all subsequences of s which equal u.For instance,consider a dimension of H3spanned(i.e.,labeled)by the string asd.In this case we have[Φ3(Nasd as)]asd= 2λ5.In thefirst string,asd is a contiguous substring.In the second string,it appears twice as a noncontiguous substring of length5in lass das,the two occurrences are las as and la d。
- 1、下载文档前请自行甄别文档内容的完整性,平台不提供额外的编辑、内容补充、找答案等附加服务。
- 2、"仅部分预览"的文档,不可在线预览部分如存在完整性等问题,可反馈申请退款(可完整预览的文档不适用该条件!)。
- 3、如文档侵犯您的权益,请联系客服反馈,我们会尽快为您处理(人工客服工作时间:9:00-18:30)。
2013年3月第32卷第1期内蒙古科技大学学报Journal of Inner Mongolia University of Science and TechnologyMarch,2013Vol.32,No.1文章编号:2095-2295(2013)01-0054-05钢铁企业副产煤气系统优化研究*赵贤聪1,白皓1,李洪福1,李梦奇1,李涛2(1.北京科技大学冶金与生态工程学院,北京100083;2.北京大学城市与环境学院,北京100871)关键词:钢铁企业;煤气系统;优化;煤气热效率中图分类号:F426.3文献标识码:A摘要:钢铁企业副产煤气系统是钢铁企业能源系统的主要部分,通过建立模型,并设立目标函数、约束条件及相关参数,利用GAMS软件计算煤气的最优配比以达到煤气平均热效率的最大化.Research on optimizing distributionof byproduct gas in iron and steel enterpriseZHAO Xian-cong1,BAI Hao1,LI Hong-fu1,LI Meng-qi,Li Tao2(1.School of Metallurgical and Ecological Engineering,University of Science and Technology Beijing,Beijing100083,China;2.School of Urban and Environmental Sciences,Peking University,Beijing100871,China)Key words:iron and steel enterprise;byproduct gas system;optimizing;byproduct gas heat efficiencyAbstract:The byproduct gas system is the main part of energy system in iron and steel enterprise.By setting up objective function,constraint condition and related parameters,the GAMS calculating software was used to optimize the byproduct gas system as to obtain the maximization of the gas average heat efficiency.钢铁工业是能源密集型行业,2011年中国钢铁工业总能耗占全国总能耗的16.3%[1],节能减排任务艰巨.在钢铁生产过程中,煤炭是主要能源,而且其热量的34%会转换为副产煤气.因此,充分利用副产煤气资源对钢铁企业节能减排有重要意义.副产煤气优化分配是提高其利用水平的重要途径,前人已做出了许多探索[2-5].过去的研究多建立在满足用户煤气需求的基础上寻求总调度成本最低,或是能源使用量最小,鲜有研究者以提高煤气热效率为目标来构建模型.本文以国内某典型大型钢铁联合企业(以下简称J钢)为研究对象,以煤气热效率为优化目标,结合GAMS软件对J钢煤气系统进行优化研究.1钢铁企业副产煤气及其优化副产煤气是钢铁企业重要的二次能源,主要包括高炉煤气(BFG)、焦炉煤气(COG)和转炉煤气(LDG).高炉煤气是铁矿石和焦炭在高炉中发生还原反应的产物,主要成分为N2和CO,热值为3000 3800kJ/Nm3;焦炉煤气是洗精煤在焦炉中高温干馏产生的,主要成分为H2和CH4,热值为17000 19000kJ/Nm3;转炉煤气的主要成分为CO和CO2,热值为6000 8000kJ/Nm3.在钢铁生产过程中,大部分用户使用混合煤气,而且不同用户对煤气的热值要求是有差别的,为了*收稿日期:2012-12-06作者简介:赵贤聪(1990-),男,湖北潜江人,北京科技大学硕士研究生.赵贤聪,等:钢铁企业副产煤气系统优化研究保证正常生产,应尽量满足其不同的热值要求.对混合煤气用户而言,如果煤气混合的种类和比例不同,其对应的空气需要量、烟气产生量以及烟气成分会有较大差别,从而导致煤气使用的热效率发生变化.由于钢铁企业煤气系统庞大,用户数量多,合理地在煤气用户之间分配三种煤气,使煤气系统整体热效率达到最大,是一个系统优化的问题,可以通过建立煤气优化模型解决煤气的优化分配.采用运筹学计算软件GAMS (General Algebraic Modeling System )进行建模优化.通过建立J 钢煤气系统优化分配模型,利用GAMS 软件编程计算,得到最优煤气配比,从而提高煤气使用的平均热效率.2煤气系统优化模型2.1J 钢概况J 钢2010年生产700万t 生铁,700万t 粗钢和750万t 钢材.该企业有焦炉9座,高炉4座,转炉3座,煤气用户11个.高炉煤气、焦炉煤气和转炉煤气经单品种加压站及混合煤气加压站后送往烧结、球团、中板等煤气用户(见图1).图1J 钢煤气系统流程图Fig.1Flow chart of byproduct gas system in J-Steel2.2模型假设在建模时作如下简化:(1)燃料在炉内是完全燃烧的,烟气中不存在可燃成分;(2)忽略煤气柜对煤气系统的微调作用;(3)将炉体散热、冷却水带走的热量等热损失系数视为固定值,从总效率中扣除;(4)煤气用户的理论燃烧温度T c 同烟气温度T w 呈线性相关.2.3目标函数及约束条件2.3.1目标函数模型的目标是实现煤气平均热效率最大化.煤气平均热效率指的是煤气系统内所有煤气用户热效率的平均值.目标函数及相关变量的表达式见公式(1)至(7).max ηm =∑11j =1(1-Q w (j )+Q s (j )Q (j )+Q g (j )+Q c (j )),(1)Q w (j )=∑4k =1[∫T w (j )T 0C w (j ,k )d T ˑ100022.4ˑV w (j ,k )],(2)Q a (j )=∑3i =1[αi ˑC α(j )ˑT α(j )],(3)Q g (j )=∑3i =1[U ij ˑC g (j )ˑT g (j )],(4)Q c (j )=∑3i =1[U ij ˑH (i )],(5)Q all (j )=Q a (j )+Q g (j )+Q c (j ),(6)Q s (j )=r (j )ˑQ all (j ),(7)式中,ηm 为煤气平均热效率;i 为BFG ,COG ,LDG 三种煤气之一;j 代表11个煤气用户之一;k 为CO 2,H 2O ,N 2及O 2等烟气成分之一;Q w (j )表示烟气带走的物理热,见公式(2);C w (j ,k )表示煤气用户j 成分为k 的定压热容,J /(Nm 3·ħ);V w (j ,k )表示煤气用户j 成分为k 的烟气体积,Nm 3;T w (j )为烟气温度;Q a (j )为助燃空气的物理热;αi 表示第i 种煤气的实际空气需要量;U ij 表示用户j 使用的第i 种煤气量;C α(j )表示空气的定压热容,J /(Nm 3·ħ);T α(j )为空气温度;Q g (j )为煤气的物理热;C g (j )为煤气的定压热容,J /(Nm 3·ħ);T g (j )为煤气温度;Q c (j )为煤气的化学热;H (i )表示第i 种煤气的热值;总带入热Q all (j )为空气物理热Q α(j ),煤气物理热Q g (j )与煤气化学热Q c (j )之和;r (j )为热损失系数,不同用户有所不同;Q s (j )为炉体及冷却水系统的热损失.2.3.2约束条件(1)副产煤气的总量约束∑11j =1U (i ,j )≤C (i ),(8)式中,C (i )表示第i 种煤气产生量,m 3;∑11j =1U (i ,j )表示第i 种煤气消耗量,Nm 3.(2)煤气用户的热量约束55内蒙古科技大学学报2013年3月第32卷第1期∑3i=1[H(i)ˑU(i,j)]≥Q(j),(9)式中,H(i)表示第i种煤气的热值,kJ/Nm3;U(i,j)表示用户j使用的第i种煤气的体积,Nm3;q(j)表示优化前煤气总化学热,MJ/h.(3)煤气用户的热值约束∑3i=1[H(i)ˑU(i,j)]∑3 i=1U(i,j)≥q(j),(10)式中,H(i)表示第i种煤气的热值,kJ/Nm3;U(ij)表示用户j使用的第i种煤气的体积,m3;q(j)表示优化前煤气热值,kJ/Nm3.3结果与讨论3.1建模所用的基本参数表1为副产煤气的成分,表2为煤气用户的热量约束及热值约束,其值由该企业2010年的实际煤气消耗量代入公式(9)和(10)计算得到.此外,副产煤气的热值、实际空气需要量可根据副产煤气的成分计算得到[6].副产煤气的产生量取该企业2010年的实际值,空气和煤气温度取环境温度.表1副产煤气的成分(质量分数,%)Table1Byproduct gas compositionCO2CmHnO2CO CH4H2N2高炉煤气19.5900.3526.7002.5150.85焦炉煤气2.492.540.286.9823.4460.453.82转炉煤气15.3300.3254.970029.38表2煤气用户的热量约束与热值约束Table2Thermal constraint and calorific value constraint of gas user煤气用户热量约束/(GJ·h-1)热值约束/(MJ·Nm-3)煤气用户热量约束/(GJ·h-1)热值约束/(MJ·Nm-3)焦化928.455.38中板234.479.59烧结124.499.45中厚板276.279.77球团125.324.36宽厚板117.339.04高炉热风炉1976.273.73热轧薄板158.138.65炼钢车间129.696.08冷轧工序56.9610.9小型材115.173.64———将目标函数和约束条件转换为GAMS软件能识别的程序代码,输入已知的煤气参数等一系列初始值,可以计算出最佳的煤气配比以及对应的煤气热效率值.3.2计算结果3.2.1有热值约束时的优化结果由表3可知,优化后的平均热效率较优化前提高了0.44%.优化后的烟气物理热降低了1.88GJ,烟气平均温度降低了13.79ħ,它们是引起煤气平均热效率提高的主要原因.为了便于研究烟气温度与煤气热效率之间的关系,表4列出了各煤气用户优化前后的煤气热效率及烟气温度的数值.从整体看,优化后的煤气热效率较优化前有小幅度提高.从单个设备看,炼钢提高最大,达到了2.06%.所有设备中只有焦化热效率出现下降,具体原因将在后文阐述.表3优化前后主要计算结果比较Table3Comparison of main calculation results before and after optimization项目优化前优化后优化后-优化前煤气平均热效率/(GJ·h-1)68.9569.390.44总带入热/(GJ·h-1)6281.686281.710.03烟气物理热/(GJ·h-1)1027.391025.51-1.88烟气总量109/(Nm3·h-1)20.7120.69-0.02烟气平均温度/ħ327.76313.95-13.7965赵贤聪,等:钢铁企业副产煤气系统优化研究表4优化前后煤气热效率和烟气温度的比较Table4Comparison of gas heat efficiency and waste gas temperature before and after optimization煤气用户优化前煤气热效率/%优化后煤气热效率/%优化后-优化前/%优化前烟气温度/ħ优化后烟气温度/ħ优化后-优化前/ħ焦化50.8850.27-0.61349.81366.6816.87烧结74.7475.080.34400.89389.31-11.58球团80.7381.410.68199.65184.00-15.65高炉79.1279.130.01254.14253.91-0.23炼钢45.5047.502.00800.56735.81-64.75小型材77.4877.480150.60150.600中板77.6978.020.33150.94140.71-9.23中厚板73.3573.870.52150.83136.14-14.69宽厚板77.5677.560148.80148.800热轧薄板54.9455.490.55599.10579.58-19.52冷轧66.5267.440.92400.89367.99-32.90平均值68.9569.390.44327.75313.95-13.79表5为优化前后煤气分配比例.结合表4可以发现,凡是优化后煤气热效率下降的用户,其优化后的COG百分含量一定下降;凡是优化后煤气热效率上升的用户,其优化后的COG百分含量一定上升.因为同样体积的COG比BFG和LDG需要的空气更多,产生的烟气量也大.若优化后COG的百分含量提高,则烟气量V w(j)会增大,根据煤气理论燃烧温度计算公式[6],理论燃烧温度会随之降低,烟气温度也会随之降低.从优化结果看,只有焦化工序优化后的COG百分含量有所降低,因而此工序优化后的烟气温度较优化前升高,煤气热效率在优化后降低.表5优化前后煤气分配比例Table5Comparison of gas distribution rate before and after optimization煤气用户优化前优化后BFG/%COG/%LDG/%热值/(MJ·m-3)T理/ħBFG/%COG/%LDG/%热值/(MJ·m-3)T理/ħ焦化87.5812.4205.381347.2072.527.7619.725.381398.47烧结43.6337.0719.309.451700.5158.3641.6409.451661.89球团78.34021.664.361272.1594.885.1204.361211.98高炉99.140.590.273.731111.8699.350.6503.731111.11炼钢45.155.8748.986.081550.7982.5517.4506.081424.32小型材100003.641089.80100003.641089.80中板38.4136.8124.789.591716.2157.3342.6709.591666.85中厚板25.5734.5239.919.771757.9156.0443.9609.771678.09宽厚板61.3238.6809.041639.9761.3238.6809.041639.97热轧薄板46.1630.3623.488.651667.5464.0935.9108.651617.99冷轧1.5037.3661.1410.91843.9048.1851.82010.91727.2675内蒙古科技大学学报2013年3月第32卷第1期3.2.2无热值约束时的优化结果热值约束的存在会限制优化空间,为了研究模型的提效潜力,讨论取消煤气热值约束时的优化结果,优化前后的主要计算结果见表6.表6优化前后主要计算结果比较Table6Comparison of main calculation results before and after optimization项目优化前优化后优化后-优化前(无热值约束)优化后-优化前(有热值约束)煤气平均热效率/%68.9571.662.710.44总带入热/(GJ·h-1)6281.686281.55-0.130.03烟气物理热/(GJ·h-1)1027.391021.95-5.44-1.88烟气总量109/(m3·h-1)20.7120.710-0.02烟气平均温度/ħ327.76243.10-84.66-13.79由表6可知,无热值约束情形下的平均效率提高至2.71%,是有热值约束时的6.2倍.优化后的烟气物理热降低了5.44GJ,烟气平均温度降低了84.66ħ.可见,无热值约束时的优化效果要明显好于有热值约束时的优化效果.由表5可知,有热值约束时优化前后的煤气热值不变.因此,煤气用户只能在煤气热值不变的前提下通过改变三种煤气的配比来提高煤气的热效率,优化效果比较有限.无热值约束时煤气用户可以通过改变优化后的煤气热值来提高煤气的热效率,显然后者优化空间更大.进一步研究得知,除焦化工序外,优化后的煤气热值皆有所降低.其中:烧结、球团和高炉等8个用户优化后单烧BFG,中板用户优化后混烧BFG和LDG,中厚板用户优化后单烧LDG,焦化工序由于煤气平衡的原因,混烧COG和LDG.由此可知,取消热值约束之后,煤气用户倾向于使用高炉煤气,则理论燃烧温度会降低,烟气温度也会随之降低,煤气热效率得以提高.虽然有热值约束下的优化模型更贴近实际情况,但是无热值约束时的优化结果表明使用低热值煤气更有利于提高煤气使用的热效率.因此在钢铁生产中应尽量减少煤气用户对煤气热值的限制,积极开发低热值煤气燃烧技术,增加低热值煤气在煤气用户中的使用率,提高煤气使用的总体热效率.4结论(1)有热值约束时,优化后煤气的平均热效率较优化前提高了0.44%,优化后的烟气物理热和烟气平均温度较优化前分别降低1.88GJ及13.79ħ;优化前后的煤气热值保持不变,大部分用户优化后COG百分含量的增加是优化后煤气平均热效率提高的驱动力.(2)无热值约束时,优化后煤气的平均热效率提高至2.71%,是有热值约束时的6.2倍;优化后的烟气物理热降低了5.44GJ,烟气平均温度降低了84.66ħ;优化后的煤气热值降低趋势明显,煤气用户倾向于使用高炉煤气,因此在钢铁生产中应积极开发低热值煤气燃烧技术,增加低热值煤气在煤气用户中的使用率,提高煤气使用的热效率.参考文献:[1]王维兴.钢铁工业能耗现状和节能潜力分析[J].中国钢铁业,2011,(4):19-22.[2]Higashi I.Energy balance of steel mills and utilization of byproduct gases[J].Transactions of the Iron and Steel In-stitute of Japan,1982,22(1):57-65.[3]Fukuda K,Makino H,Suzuki Y.Optimal energy distri-bution control at the steel works[A].IFAC simulation ofcontrol systems[C].Austria:IFAC Publication,1986:337-342.[4]Akimoto K,Sannomiya N,Nishikawa Y,et al.An optimal gas supply for a power plant using a mixed integer pro-gramming model[J].Automatica,1991,27(3):513-518.[5]Sinha G P,Chandrasekaran B S.Strategic and operational management with optimization at tata steel[J].Interfaces,1995,25(1):6-19.[6]韩昭沧.燃料及燃烧[M].北京:冶金工业出版社,1994.85。