L-fuzzy interiors and L-fuzzy closures
Fuzzy邻近空间(Ⅱ)(英文)
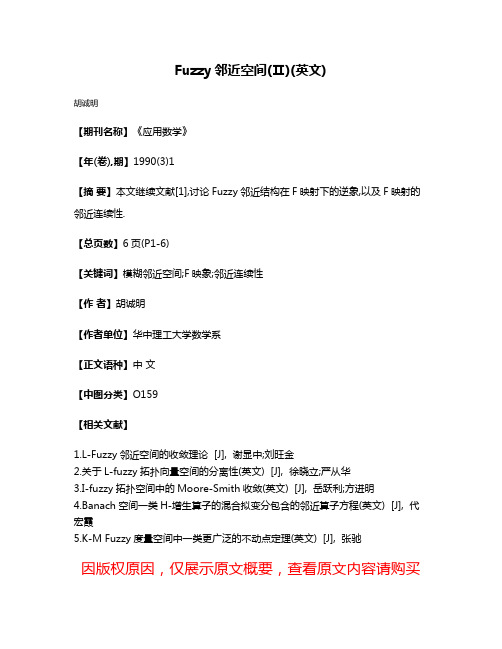
Fuzzy邻近空间(Ⅱ)(英文)
胡诚明
【期刊名称】《应用数学》
【年(卷),期】1990(3)1
【摘要】本文继续文献[1],讨论Fuzzy邻近结构在F映射下的逆象,以及F映射的邻近连续性.
【总页数】6页(P1-6)
【关键词】模糊邻近空间;F映象;邻近连续性
【作者】胡诚明
【作者单位】华中理工大学数学系
【正文语种】中文
【中图分类】O159
【相关文献】
1.L-Fuzzy邻近空间的收敛理论 [J], 谢显中;刘旺金
2.关于L-fuzzy拓扑向量空间的分离性(英文) [J], 徐晓立;严从华
3.I-fuzzy拓扑空间中的Moore-Smith收敛(英文) [J], 岳跃利;方进明
4.Banach空间一类H-增生算子的混合拟变分包含的邻近算子方程(英文) [J], 代宏霞
5.K-M Fuzzy度量空间中一类更广泛的不动点定理(英文) [J], 张驰
因版权原因,仅展示原文概要,查看原文内容请购买。
IEEE International Conference on Fuzzy Systems, San Antonio,
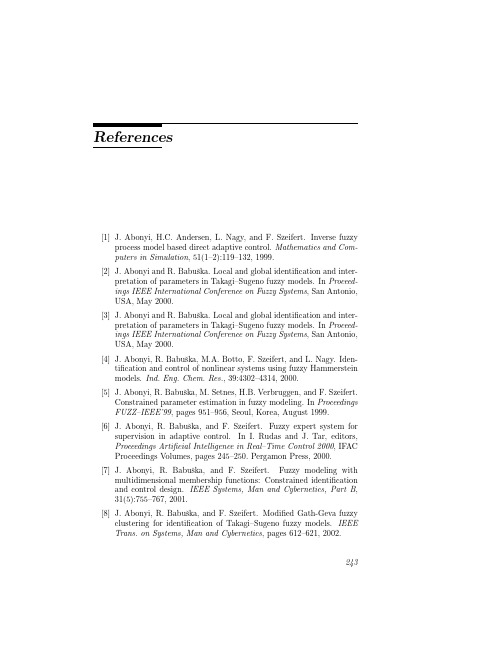
References[1]J.Abonyi,H.C.Andersen,L.Nagy,and F.Szeifert.Inverse fuzzyprocess model based direct adaptive control.Mathematics and Com-puters in Simulation,51(1–2):119–132,1999.[2]J.Abonyi and R.Babuˇs ka.Local and global identification and inter-pretation of parameters in Takagi–Sugeno fuzzy models.In Proceed-ings IEEE International Conference on Fuzzy Systems,San Antonio, USA,May2000.[3]J.Abonyi and R.Babuˇs ka.Local and global identification and inter-pretation of parameters in Takagi–Sugeno fuzzy models.In Proceed-ings IEEE International Conference on Fuzzy Systems,San Antonio, USA,May2000.[4]J.Abonyi,R.Babuˇs ka,M.A.Botto,F.Szeifert,and L.Nagy.Iden-tification and control of nonlinear systems using fuzzy Hammerstein models.Ind.Eng.Chem.Res.,39:4302–4314,2000.[5]J.Abonyi,R.Babuˇs ka,M.Setnes,H.B.Verbruggen,and F.Szeifert.Constrained parameter estimation in fuzzy modeling.In Proceedings FUZZ–IEEE’99,pages951–956,Seoul,Korea,August1999.[6]J.Abonyi,R.Babuˇs ka,and F.Szeifert.Fuzzy expert system forsupervision in adaptive control.In I.Rudas and J.Tar,editors, Proceedings Artificial Intelligence in Real–Time Control2000,IFAC Proceedings Volumes,pages245–250.Pergamon Press,2000.[7]J.Abonyi,R.Babuˇs ka,and F.Szeifert.Fuzzy modeling withmultidimensional membership functions:Constrained identification and control design.IEEE Systems,Man and Cybernetics,Part B, 31(5):755–767,2001.[8]J.Abonyi,R.Babuˇs ka,and F.Szeifert.Modified Gath-Geva fuzzyclustering for identification of Takagi–Sugeno fuzzy models.IEEE Trans.on Systems,Man and Cybernetics,pages612–621,2002.243244References [9]J.Abonyi,R.Babuˇs ka,F.Szeifert,L.Nagy,and H.B.Verbruggen.Design and application of block–oriented fuzzy models–fuzzy Ham-merstein model.In Y.Suzuki,S.Ovaska,and T.Furuhashi,editors, Soft Computing in Industrial Applications.Springer,London,UK, September2000.[10]J.Abonyi,R.Babuˇs ka,H.Verbruggen,and ing apriori knowledge in fuzzy model identification.International Journal of Systems Science,31:657–667,2000.[11]J.Abonyi,R.Babuˇs ka,L.F.A.Wessels,H.B.Verbruggen,andF.Szeifert.Fuzzy modeling and model based control with use of apriori knowledge.In3rd IMACS symposium on Mathematical Mod-elling,MATHMOD2000,pages769–772,Vienna,Austria,March 2000.[12]J.Abonyi,A.Bodizs,L.Nagy,and F.Szeifert.Hybrid fuzzy con-volution model based predictor corrector controller.In M.Mohama-dian,editor,Computational Intelligence for Modelling Control and Automation,pages265–270.IOS Press,Holland,ISBN9–051–99474–51999.[13]J.Abonyi,A.Bodizs,L.Nagy,and F.Szeifert.Predictor correc-tor controller using Wiener Fuzzy Convolution Model.Hungarian Journal of Industrial Chemistry,27(3):227–233,1999.[14]J.Abonyi,A.Bodizs,L.Nagy,and F.Szeifert.Hybrid fuzzy con-volution model and its application in predictive control.Chemical Engineering Research and Design,78:597–604,2000.[15]J.Abonyi,T.Chovan,L.Nagy,and F.Szeifert.Hybrid convolutionmodel and its application in predictive pH puters and Chemical Engineering,S:221–224,1999.[16]J.Abonyi,L.Nagy,and F.Szeifert.Fuzzy control of batch polymer-ization reactors.In IEEE International Conference on Intelligent Systems,INES’97,pages251–255,Budapest,Hungary,September 1997.[17]J.Abonyi,L.Nagy,and F.Szeifert.Takagi–Sugeno fuzzy control ofbatch polymerization reactors.In P.K.Chawdry,R.Roy,and R.K.Plant,editors,Soft Computing In Engineering Design and Manufac-turing,pages420–429.Springer,London,UK,ISBN3–540–76214–0 1997.[18]J.Abonyi,L.Nagy,and F.Szeifert.Adapive Sugeno fuzzy control:A case study.In R.Roy,editor,Advances in Soft Computing–En-gineering Design and Manufacturing.Springer,London,UK,ISBN 1–852–33062–7,1998.References245 [19]J.Abonyi,L.Nagy,and F.Szeifert.Adaptive fuzzy inference systemand its application in modelling and model based control.Chemical Engineering Research and Design,77A:281–290,1999.[20]J.Abonyi,L.Nagy,and F.Szeifert.Letter to the editor:Com-ments on block–oriented NARMAX models with output multiplici-ties.AIChE Journal,45:(8):1838–1839,1999.[21]J.Abonyi,L.Nagy,and F.Szeifert.Hybrid fuzzy convolution mod-elling and identification of chemical process systems.International Journal of Systems Science,31:457–466,2000.[22]J.Abonyi,L.Nagy,and F.Szeifert.Fuzzy model based predic-tive control by instantaneous linearisation.Fuzzy Sets and Systems, 120(1):109–122,2001.[23]J.Abonyi,H.Roubos,R.Babuˇs ka,and F.Szeifert.Identificationof semi-mechanistic models with interpretable ts-fuzzy submodels by clustering,ols andfis model reduction.In F.Herrera L.Magdalena J.Casillas,O.Cordon,editor,Trade-offbetween Accuracy and Inter-pretability in Fuzzy Rule-Based Modelling.Studies in Fuzziness and Soft Computing,Physica-Verlag,London,UK,2002.[24]J.Abonyi and J.A.Roubos.Initialization of fuzzy classification rules.In5th Online World Conference on Soft Computing in Industrial Applications,WSC5,Internet,September2000.[25]bining neural and conventional paradigms for mod-elling,prediction and control.International Journal of Systems Sci-ence,28(1):65–81,1997.[26]H.C.Andersen,A.Lotfi,and A.C.Tsoi.A new approach to adaptivefuzzy control:The Controller Output Error Method.IEEE Trans-actions on Systems,Man,and Cybernetics,SMC–27–B(4),August 1997.[27]H.C.Andersen,A.Lotfi,and ments on‘func-tional equivalence between radial basis function networks and fuzzy inference systems’.IEEE Transactions on Neural Networks,May 1998.[28]T.Anderson and P.Pucar.Estimation of residence time in continu-ousflow systems with dynamics.Journal of Process Control,5:9–17, 1995.[29]M.R.Arahal,M.Berenguel,and E.F.Camacho.Neural identificationapplied to predictive control of a solar plant.Control Engineering Practice,6:334–344,1998.246References [30] C.Arbeithuber,H.P.Jorgl,and H.Raml.Fuzzy control of an ironore sintering plant.Control Engineering Practice,3:1669–1674,1995.[31]P.Arva and S.Nemeth.Learning algorithm in fuzzy control.AnnalesUniv.Sci.Budapest p.,12:25–32,1991.[32]K.J.˚A str¨o m and B.Wittenmark.Adaptive Control.Addison Wesley,Reading,MA,USA,1989.[33]K.J.˚A str¨o m and puter Controlled Systems:Theory and Design.Prentice–Hall International,Inc.,London,1990.[34]M.Athans.Debate with Prof.LotfiA.Zadeh.In7th EuropeanConference on Intelligent Techniques and Soft Computing(EUFIT ’99).Aachen,Germany,Sep1999.[35]R.Babuˇs ka.Fuzzy Modeling for Control.Kluwer Academic Publish-ers,Boston,1998.[36]R.Babuˇs ka,C.Fantuzzi,U.Kaymak,and H.B.Verbruggen.Im-proved inference for Takagi–Sugeno models.In Proceedings Fifth IEEE International Conference on Fuzzy Systems,pages701–706, New Orleans,USA,Sep1996.[37]R.Babuˇs ka,J.A.Roubos,and H.B.Verbruggen.Identification ofMIMO systems by input–output TS fuzzy models.In Proceedings FUZZ–IEEE’98,pages657–662,Anchorage,USA,1998.[38]R.Babuˇs ka,J.M.Sousa,and H.B.Verbruggen.Inverse fuzzy modelbased predictive control.In D.Driankov and R.Palm,editors,Ad-vances in Fuzzy Control,pages129–154.Springer,Heidelberg,Ger-many,1998.[39]R.Babuˇs ka,H.A.B.te Braake, A.J.Krijgsman,and H.B.Ver-parison of intelligent control schemes for real–time pressure control.Control Engineering Practice,4(11):1585–1592, 1996.[40]R.Babuˇs ka and H.B.Verbruggen.An overview of fuzzy modelingfor control.Control Engineering Practice,4(11):1593–1606,1996. [41]R.Babuˇs ka and H.B.Verbruggen.Fuzzy identification of Hammer-stein systems.In Proceedings Seventh IFSA World Congress,vol-ume II,pages348–353,Prague,Czech Republic,June1997.[42]R.Babuˇs ka and H.B.Verbruggen.Fuzzy set methods for local mod-eling and identification.In R.Murray-Smith and T.A.Johansen, editors,Multiple Model Approaches to Nonlinear Modeling and Con-trol,pages75–100.Taylor&Francis,London,UK,1997.References247 [43]R.Babuˇs ka,H.B.Verbruggen,and H.J.L.van Can.Fuzzy modelingof enzymatic penicillin–G conversion.Engineering Applications of Artificial Intelligence,12(1):79–92,1999.[44]T.C biningfirst principles dynamic modelling and plantidentification for design of an industrial MPC application to a poly-mer reactor.In Proceedings of the14th Triennieal IFAC World Congress,pages N–7a–03–4.1999.[45]V.Balakrishnan,A.Zheng,and M.Morari.Constrained stabilizationof discrete–time systems.Advances in MBPC.Oxford University Press,1994.[46] A.Banerjee and Y.Arkun.Model predictive control of plant transi-tions using a new identification technique for interpolating nonlinear models.Journal of Process Control,8:441–457,1998.[47]P.Baranyi,I.M.Bavelaar,R.Babuˇs ka,L.T.K´o czy,A.Titli,andH.B.Verbruggen.A method to invert a linguistic fuzzy model.In-ternational Journal of Systems Science,29(7):711–721,1998. [48]G.Barko,J.Abonyi,and J.Hlavay.Application of fuzzy clusteringand piezoelectric chemical sensor array for investigation on organic compounds.Analitica Chimica Acta,398(2–3):219–222,1999. [49] B.W.Bequette.Nonlinear control of chemical processes:A review.Industrial Engineering Chemical Research,30:1391–1413,1991. [50]M.Berger.Self–tuning of a PI controller using fuzzy logic for aconstruction unit testing apparatus.Control Engineering Practice, 4:785–790,1996.[51]N.V.Bhat and e of neural nets for dynamic mod-elling and control of chemical process puters and Chem-ical Engineering,4/5:573–585,1990.[52]N.V.Bhat and T.J.McAvoy.Determining model structure for neuralmodels by network puters and Chemical Engineering, 16:271–281,1992.[53]N.V.Bhat,P.A.Minderman,T.J.McAvoy,and N.S.Wang.Mod-elling chemical process systems via neural computation.IEEE Con-trol Systems Magazine,April:24–30,1990.[54]K.K.Biassizo,I.Skrjanc,and D.Matko.Fuzzy predictive control ofhighly nonlinear pH puters and Chemical Engineering, 21/S:613–618,1997.[55]H.H.J.Bloemen and T.J.J.van den Boom.MPC for Wiener systems.In Proceedings of the38th Conference on Decision and Control,pages 4595–4600,Phoenix,Arizona,USA,Dec.1999.248References [56] A.Bodizs,F.Szeifert,and T.Chovan.Convolution model basedpredictive controller for a nonlinear process.Ind.Eng.Chem.Res., 38:154–161,1999.[57]J.D.Bomberger and D.E.Seborg.Determination of model order forNARX models directly from input–output data.Journal of Process Control,8:459–468,Oct–Dec1998.[58]K.M.Bossley.Neurofuzzy Modelling Approaches in System Identifi-cation.PhD thesis,University of Southampton,1997.[59]M.A.Botto,T.Boom,A.Krijgsman,and J.S´a Costa.Constrainednonlinear predictive control based on input–output linearization us-ing a neural network.International Journal of Control,72(17):1538–1554,1999.[60]G.E.P.Box and G.M.Jenkins.Time Series Analysis,Forecastingand Control.Holden Day,San Francisco,USA,1970.[61]S.P.Boyd,L.E.Ghaoui,and E.Feron.Linear Matrix Inequalities inSystems and Control Theory.SIAM,Philadelphia,1994.[62]H.A.B.T Braake,van H.J.L.Can,and H.B.Verbruggen.Semi–mechanistic modelling of chemical processes with neural networks.Engineering Applications Artificial Intelligence,11(4):507–515,1998.[63] D.M.Brengel and W.D.Sieder.Multistep nonlinear predictive con-troller.Ind.Eng.Chem.Res,28:1812–1822,1989.[64]L.Brieman.Classification,and function approximation.HingingHyperplanes for Regression,39(3):999–1013,1993.[65] A.Brooms and B.Kouvaritakis.Successive constrained optimiza-tion and interpolation in non–linear model based predictive control.International Journal of Control,68(3):599–623,2000.[66]M.Brown and C.Harris.Neurofuzzy Adaptive Modelling and Control.Prentice Hall,New York,1994.[67]M.Brown and C.J.Harris.A perspective and critique of adaptiveneurofuzzy systems used for modelling and control applications.In-ternational Journal of Neural Systems,6:197–220,1995.[68]M.Brown and parison of node insertion algorithmsfor Delaunay networks.In Proceedings of IMACS2nd MATHMOD Conference,pages445–780,Vienna,1997.[69]P.M.Brujin and H.B.Verbruggen.Model algorithmic control usingimpulse response model.Journal A,25(2):69–74,1984.References249 [70] A.E.Bryson and Y.C.Ho.Adaptive Optimal Control.HemispherePublishing Corporation,1975.[71] E.F.Camacho and C.Bordons.Model Predictive Control in the Pro-cess Industry.Springer Verlag,Berlin,Germany,1995.[72]H.J.L.Can,H.A.B.Braake te,C.Hellinga,K.Ch.A.M.Luyben,andJ.J.Heijnen.An efficient model development strategy for biopro-cesses based on neural networks in macroscopic balances.Biotech-nology and Bioengineering,54:549–566,1997.[73]H.J.L.Can,H.A.B.te Brake,C.Hellinga,A.J.Krisgsman,H.B.Ver-bruggen,K.Ch.A.M.Luyben,and J.J.Heijnen.Design and real time testing of neural model predictive controller for nonlinear systems.Chemical Engineering Science,50(15):2419–2430,1995.[74] C.T.Chou and M.Verhaegen.An indirect approach to closed–loopidentification of Wiener models.In Proceedings of the American Con-trol Conference,San Diego,USA,June1999.[75]M.Y.Chow,S.Altug,and H.J.Trussel.Heuristic constraints enforce-ment for training of and knowledge extraction from a fuzzy/neural arhitecture.IEEE Transactions on Fuzzy Systems,7(2):143–159, 1999.[76] D.W.Clarke and C.Mohtadi.Properties of generalized predictivecontrol.Automatica,25(6):859–875,1989.[77] D.W.Clarke,C.Mothadi,and P.S.C.Tuffs.Generalized predictivecontrol–Part I.The basic algorithm.Automatica,23:137–148,1989.[78] B.J.Cott,R.G.Durham,and G.R.Sullivan.Process model based en-puters and Chemical Engineering,13:973–984,1989.[79]M.G.Cox.Practical spline approximation.In Technical Report DITC1/82.National Physical Laboratory,USA,1982.[80] D.Cubanski and D.Cyganski.Multivariable classification throughadaptive Delaunay–based c spline approximation.IEEE Trans-actions on Pattern Analyzis and Machine Intelligence,17:403–417, 1995.[81]J.M.Dion,L.Dugard,A.Franco,Nguyen Minh Tri,and D.Rey.MIMO adaptive constrained predictive control case study:An envi-romental test chamber.Automatica,27:611–626,1991.[82] D.Dong and T.J.McAvoy.Nonlinear principal component analysis–based on principal curves and neural puters and Chemical Engineering,20(1):65–78,1996.250References [83] D.Driankov,H.Hellendoorn,and M.Reinfrank.An Introduction toFuzzy Control.Springer-Verlag,Heidelberg,Germany,1993.[84] D.Driankov,H.Hellendoorn,and M.Reinfrank.An Introduction toFuzzy Control.Springer,Berlin,1993.[85] D.Driankov and R.Palm,editors.Advances in Fuzzy Control.Springer,Heidelberg,Germany,1998.[86]H.Al.Duwaish and N.M.Karim.A new method for identification ofHammerstein model.Automatica,33(10):1871–1875,1997.[87]H.Al.Duwaish,N.M.Karim,and e of mul-tilayer feedforward neural networks in identification and control of Wiener model.IEE Proceedings of Control Theory and Applications, 143(3):255–258,1996.[88]J.W.Eaton and J.B.Rawlings.Feedback control of chemical pro-cesses using on–line optimization puters and Chem-ical Engineering,14:469–479,1990.[89] C.G.Economou,M.Morari,and B.O.Palsson.Internal model con-trol.5.Extension to nonlinear systems.Ind.Eng.Chem.Process Des.Dev.,25:403–411,1986.[90] E.Eskinat,S.H.Johnson,and e of Hammerstein mod-els in identification of nonlinear systems.AIChE Journal,37(2):255–268,1991.[91]J.J.Espinosa and J.Vandewalle.Predictive control using fuzzy mod-els.In R.Roy,editor,Advances in Soft Computing–Engineering Design and Manufacturing.Springer,London,UK,1998.[92] B.Feil,J.Abonyi,and F.Szeifert.Determining the model orderof nonlinear input-output systems by fuzzy clustering.In7th On-line World Conference on Soft Computing in Industrial Applications (WSC7).http://wsc7.ugr.es,London,UK,September2002.[93]M.Fischer and R.Isermann.Inverse fuzzy process models for robusthybrid control.In D.Driankov and R.Palm,editors,In Advances in Fuzzy Control,pages103–128.Physica–Verlag,Heildelberg,New York,1998.[94]M.Fischer and O.Nelles.Predictive control based on local linearfuzzy models.International Journal of Systems Science,29:679–697, 1998.[95]U.Forssell.Properties and Usage of Closed–loop identification meth-ods.Ph.D Thesis,Linkoping University,1997.References251 [96]T.R.Fortesque,L.S.Kershenbaum,and B.E.Ydstie.Implementa-tion of self tuning regulators with variable forgetting factor.Auto-matica,17:831–935,1981.[97] B.A.Foss,T.A.Johansen,and A.V.Sorensen.Nonlinear predictivecontrol using local models–applied to a batch fermentation pro-cesses.Control Engineering Practice,3:389–396,1995.[98]K.P.Fruzetti,A.Palazoglu,and K.A.McDonald.Nonlinear modelpredictive control using Hammerstein models.Journal of Process Control,7(1):31–41,1997.[99] C.Fu and M.Poch.Fuzzy modelling and pattern recognition for dy-namic processes and its application for an activated sludge processes.Chemical Engineering Science,50:3715–3725,1995.[100] C.E.Garcia and M.Morari.Internal model control: 1.A unifying review and some new results.Ind.Eng.Chem.Process Res.Dev., 21:308–323,1982.[101] C.E.Garcia and A.M.Morshedi.Quadratic programming solution of dynamic matrix control(QDMC).Chemical Engineering Commu-nications,46:73–87,1986.[102]S.E.Garcia,D.M.Prett,and M.Morari.Model predictive control: Theory and practice–a survey.Automatica,25(3):335–348,1989.[103] A.K.Garga and N.K.Bose.A neural network approach to the con-struction of Delaunay tessellation points in r d.IEEE Transactions on Circuits and Systems–I,41:611–613,1994.[104] E.P.Gatzke and F.Doyle III.Multiple model approach for CSTR control.In Proceedings of the14th Triennieal IFAC World Congress, pages N–7a–11–5.1999.[105]G.Goodwin and K.S.Sin.Adaptive Filtering Prediction and Control.Prentice-Hall Inc.,Englewood Cliffs,New Jersey,1984.[106] A.Grace.Optimization Toolbox User’s Guide.The Math Works Inc, Massachusetts,USA,1992.[107]P.Graham and R.B.Newel.Fuzzy identification and control of a liquid level rig.Fuzzy Sets and Systems,26:255–273,1988.[108]P.Graham and R.B.Newel.Fuzzy adaptive control of afirst order process.Fuzzy Sets and Systems,31:47–65,1989.[109] A.Grauel and H.Mackenberg.Mathematical analysis of the Sugeno controller leading to general design rules.Fuzzy Sets and Systems, 85:165–175,1997.252References [110] F.Guely and P.Siarry.Gradient descent method for optimizing various fuzzy rule bases.In Proc.of the IEEE–Fuzz–93,pages1241–1246.1993.[111] F.Guely and P.Siarry.A centred formulation of Takagi–Sugeno rules for improved learning efficiency.Fuzzy Sets and Systems,62:277–285, 1994.[112] E.J.Hartman,J.D.Keeler,and yered Neural Networks with Gaussian Hidden Units As Universal Approximations.Neural Computation,2:210–215,1990.[113]H.Hellendoorn and D.Driankov,editors.Fuzzy Model Identification: Selected Approaches.Springer,Berlin,Germany,1997.[114]M.Henson and D.E.Seborg.Nonlinear Process Control.Prentice Hall,Englewood Cliffs N.J.,USA,1997.[115]M.A.Henson.Nonlinear model predictive control:Current status and future puters and Chemical Engineering,23:187–202,1998.[116] E.Hernandez and Y.Arkun.Study of control–relevant properties of backpropagation neural network models of nonlinear dynamical puters and Chemical Engineering,16(4):227–240,1992.[117] E.Hernandez and Y.Arkun.Control of nonlinear systems using polynomial ARMA models.AICHE Journal,39(3):446–460,1993.[118]J.Q.Hu and E.Rose.Predictive fuzzy control applied to the sinter strand process.Control Engineering Practice,5:247–253,1997. [119]K.J.Hunt,R.Haas,and R.Murray-Smith.Extending the functional equivalence of radial basis function networks and fuzzy inference sys-tems.IEEE Transactions on Neural Networks,7(3):776–781,1996.[120]K.J.Hunt and T.A.Johansen.Design and analysis of gain–scheduled control using local controller networks.International Journal of Con-trol,66(5):619–651,1997.[121]K.J.Hunt and D.Sbarbaro.Neural networks for nonlinear internal model control.IEE Proceedings–D.,138:431–438,1991.[122]K.J.Hunt,D.Sbarbaro,R.Zbikowki,and P.J.Gawthrop.Neural networks for control systems—A survey.IEEE Transactions on Neural Networks,28:1083–1112,1992.[123]W.H.Hwang,J.I.Chey,and J.I.Rhee.Modelling and control of continuous stirred tank reactor for thermal copolymerisation.In-ternational Journal of Applied Polymerisation Science,67:921–931, 1998.References253 [124] E.Ikonen,K.Najim,and U.Kortela.Identification of non–linear processes using steady–state models with linear FIR dynamics.In Proceedings of the14th Triennieal IFAC World Congress,pages H–3a–02–3.1999.[125]S.R Inamdar and M.S.Chiu.Fuzzy logic control of an unstable bio-logical reactor.Chemical Engineering Technology,20:414–418,1997.[126]R.Isermann and chmann.Parameter adaptive control with configurational aids and supervision functions.Automatica, 21(6):625–638,1985.[127]H.Ishibuchi,T.Nakashima,and T.Murata.Performance evaluation of fuzzy classifier systems for multidimensional pattern classification problems.IEEE Transaction on Systems,Man,and Cybernetics: Part B,29:601–618,1999.[128]H.Ishibuchi,K.Nozaki,H.Tanaka,Y.Hosaka,and M.Matsuda.Empirical study on learning in fuzzy systems by rice taste analysis.Fuzzy Sets and Systems,64:129–144,1994.[129]R.A.Jacobs and M.I.Jordan.Learning piecewise control strategies in a modular neural network architecture.IEEE Transactions on Systems,Man,and Cybernetics,23(2):337–345,1993.[130]R.Jager.Fuzzy logic in control.Delft University of Technology,The Netherlands,1995.[131]J.-S.R.Jang and Sun C.-T.Functional Equivalence Between Ra-dial Basis Function Networks and Fuzzy Inference Systems.IEEE Transactions on Neural Networks,4(1):156–159,Jan.1993. [132]J.-S.R.Jang.Input selection for anfis learning.In Proceedings of the IEEE International Conference on Fuzzy Systems,Orlando,USA, 1994.[133]J.-S.R.Jang,C.-T.Sun,and E.Mizutani.Neuro–Fuzzy and Soft Computing;a Computational Approach to Learning and Machine In-telligence.Prentice–Hall,Upper Sadle River,1997.[134]J.S.R.Jang.ANFIS:Adaptive–network–based fuzzy inference sys-tems.IEEE Transactions on Systems,Man and Cybernetics,23:665–685,1993.[135]J.S.R.Jang and C.T.Sun.Neuro–fuzzy modelling and control.Pro-ceedings of the IEEE,83:378–406,1995.[136] C.Jian.A predicting system based on combining an adaptive pre-dictor and a knowledge base as applied to a blast furnace.Journal of Forecasting,12:93–102,1993.254References [137]Y.Jin.Fuzzy modeling of high–dimensional systems.IEEE Trans-actions on Fuzzy Systems,8:212–221,2000.[138]Z.Jinxiang,Z.Zaiman,Q.Yanbin,Y.Linru,W.Suqin,and L.Lianyu.pH fuzzy control of automated industrial electroplating of gold.Chemical Engineering Technology,20:576–580,1997.[139]T.A.Johansen.Operating regime based process modeling and identi-fication.PhD thesis,Department of Engineering Cybernetics,Nor-wegian Institute of Technology,University of Trondheim,Norway, 1994.[140]T.A.Johansen.Identification of non–linear systems using empirical data and a priori knowledge–an optimisation approach.Automatica, 32:337–356,1996.[141]T.A.Johansen.Constrained and regularized system identification.Modeling,Identification and Control,19(2):109–116,1998.[142]T.A.Johansen,R.Shorten,and R.Murray-Smith.On the inter-pretation and identification of Takagi–Sugeno fuzzy models.IEEE Transactions on Fuzzy Systems,8:297–313,2000.[143] C.R.Johnson and E.Tse.Adaptive implementation of one–step–ahead optimal control via input matching.IEEE Transactions on Automatic Control,AC–23(5):865–872,October1978.[144] C.R.Johnson.Lectures on Adaptive Parameter Estimation.Prentice Hall,Englewood Cliffs,1988.[145]S.B.Jorgensen and K.M.Hangos.Grey box modelling for control: Qualitative models as a unifying framework.International Journal of Adaptive Control and Signal Processing,9:547–562,1995. [146]M.Karny,A.Haluskova,and P.Nedoma.Recursive approximation by ARX model:A tool for grey–box modelling.Int.J.Adaptive Control and Signal Processing,9:525–546,1995.[147]T.Kavli.ASMOD–an algorithm for adaptive spline modelling of observation data.International Journal of Control,58(4):947–967, 1993.[148]T.Kavli and G.T.Lines.A unifying framework for mechanistic: Fuzzy and data driven modelling.In EUFIT’96,pages1241–1246.Aachen,Germany,Sep1996.[149]R.M.C.Keyser,G.A.Velde,and F.A.G.Dumortier.A comparative study of self–adaptive long–range predictive control methods.Auto-matica,24(2):149–163,1988.References255 [150] E.Kim,M.Park,S.Kim,and M.Park.A transformed input–domain approach to fuzzy modeling.IEEE Transactions on Fuzzy Systems, 6:596–604,1998.[151]G.J.Klir and B.Yuan.Fuzzy sets and fuzzy logic;theory and appli-cations.Prentice Hall,1995.[152]M.V.Kothare,V.Balakrihnan,and M.Morari.Robust constrained predictive control using linear matrix inequalities.Automatica, 32(10):1361–1379,1996.[153] C.W.Koung and J.F.MacGregor.Design of identification exper-iments for robust control.A geometric approach for bivariate pro-cesses.Ind.Eng.Chem.Res.,32:1658–1666,1993.[154] A.Kraslawski,W.Pedrycz,T.Koiranen,and L.Nystrom.Hybrid knowledge–based tool for mixing equipment selection–the fuzzy neural network.Chemical Engineering Technology,19:233–239,1996.[155] A.Kroll.Identification of functional fuzzy models using multidimen-sional reference sets.Fuzzy Sets and Systems,80:149–158,1996.[156]M.J.Kurtz and M.A.Henson.Input–output linearizing control of constrained nonlinear processes.Journal of Process Control,7:3–17, 1997.[157] gerberg and C.Breitholtz.A study of gain scheduling control applied to an exothermic CSTR.Chemical Engineering Technology, 20:435–444,1997.[158] D.Lee and T.Kang.Adaptive fuzzy control of the molten steel level in a strip–casting process.Control Engineering Practice,4:1511–1520,1996.[159]J.H.Lee.Modeling for nonlinear model predictive control:Re-quirements,current status and future research needs.In Allgower and A.Zheng,editors,Nonlinear Model Predictive Control,Progress in Systems and Control Theory Series,volume26,pages348–353.Birkh¨a user Verlag,Basel,2000.[160]J.H.Lee and Z.H.Yu.Tuning of model predictive controllers for ro-bust puters and Chemical Engineering,18(1):15–37,1994.[161]R.Leitch.Artificial intelligence in control:Some myths,some fears but plenty puting and Control Engineering Journal, July:153–163,1992.[162] D.J.Leith and W.E.Leithead.Analytic framework for blended mul-tiple model systems using linear local models.International Journal of Control,72:605–619,May1999.256References [163]I.J.Leontaritis and S.A.Billings.Experimental design and identifi-ably for nonlinear systems.International Journal of Systems Science, 18:189–202,1987.[164]W.C.Li and L.T.Biegler.Multistep,Newton–type control strategies for constrained,nonlinear processes.Chem.Eng.Res.Des.(Trans IChemE),67:532–,1989.[165]G.Lightbody,P.O’Reilly,K.Kelly,and J.McCormick.Neural mod-elling of chemical plant using MLP and B–spline networks.Control Engineering Practice,5(11):150–1515,1997.[166]P.Lindskog.Fuzzy identification from a grey box modeling point of view.In H.Hellendoorn and D.Driankov,editors,Fuzzy Model Identification:Selected Approaches.Springer,Berlin,Germany,1997.[167]P.Lindskog and L.Ljung.Tools for semiphysical modelling.Interna-tional Journal of Adaptive Control and Signal Processing,9(6):509–523,1995.[168] D.A.Linkens and S.Kandiah.Long–range predictive control using fuzzy process models.Chem.Eng.Res.Des.(Trans IChemE),74:77–88,1996.[169]L.Ljung.System Identification,Theory for the User.Prentice–Hall, New Jersey,1987.[170]L.Ljung and T.S¨o derstr¨o m.Theory and practice of recursive iden-tification.MIT Press,Cambridge,Massachusetts,1983.[171] A.Lofti,H.C.Andersen,and A.C.Tsoi.Interpretation preserva-tion of adaptive fuzzy inference systems.International Journal of Approximate Reasoning,15(4):379–394,1996.[172]R.F Luo and Z.J.Zhang.Fuzzy–neural–net–based inferential control for a high–purity distillation column.Control Engineering Practice, 3:31–40,1995.[173]W.Luo,M.N.Karim,A.J.Morris,and E.B.Martin.A control rele-vant identification of a pH waste water neutralization process using adaptive radial basis function puters and Chemical Engineering,20/S:1017–1022,1996.[174]M.M.Mahesh and M.Chidambaram.Fuzzy state estimator for semi-batch copolimerisation reactor.Chemical Engineering Technology, 15:395–400,1992.[175] E.H.Mamdani.Advances in the linguistic synthesis of fuzzy con-trollers.International Journal of Man–Machine Studies,8:669–678, 1976.。
L—fuzzy层次拓扑空间的Ir—可数性
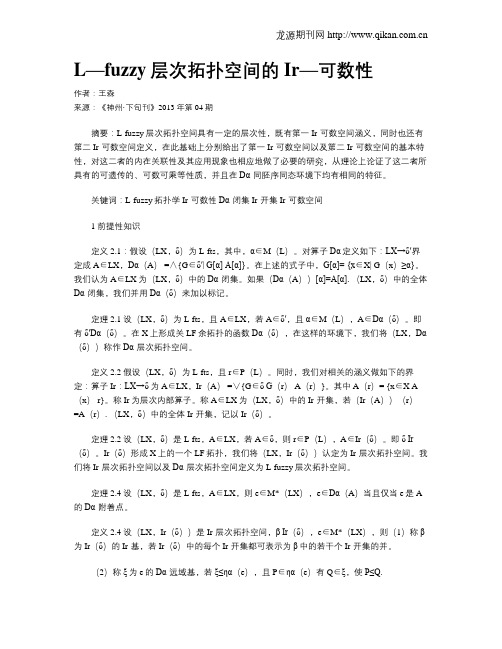
L—fuzzy层次拓扑空间的Ir—可数性作者:王森来源:《神州·下旬刊》2013年第04期摘要:L-fuzzy层次拓扑空间具有一定的层次性,既有第一Ir-可数空间涵义,同时也还有第二Ir-可数空间定义,在此基础上分别给出了第一Ir-可数空间以及第二Ir-可数空间的基本特性,对这二者的内在关联性及其应用现象也相应地做了必要的研究,从理论上论证了这二者所具有的可遗传的、可数可乘等性质,并且在Dα-同胚序同态环境下均有相同的特征。
关键词:L-fuzzy拓扑学 Ir-可数性Dα-闭集 Ir-开集 Ir-可数空间1 前提性知识定义2.1:假设(LX,δ)为L-fts,其中,α∈M(L)。
对算子Dα定义如下:LX→δ′界定成A∈LX,Dα(A) =∧{G∈δ′| G[α] A[α]}。
在上述的式子中,G[α]= {x∈X| G(x)≥α}。
我们认为A∈LX为(LX,δ)中的Dα-闭集。
如果(Dα(A))[α]=A[α]. (LX,δ)中的全体Dα-闭集,我们并用Dα(δ)来加以标记。
定理2.1 设(LX,δ)为L-fts,且A∈LX,若A∈δ′,且α∈M(L),A∈Dα(δ)。
即有δ′Dα(δ)。
在X上形成关LF余拓扑的函数Dα(δ),在这样的环境下,我们将(LX,Dα(δ))称作Dα-层次拓扑空间。
定义2.2 假设(LX,δ)为L-fts,且r∈P(L)。
同时,我们对相关的涵义做如下的界定:算子Ir:LX→δ为A∈LX,Ir(A) =∨{G∈δ G(r) A(r)}。
其中A(r)= {x∈X A (x) r}。
称Ir为层次内部算子。
称A∈LX为(LX,δ)中的Ir-开集,若(Ir(A))(r)=A(r). (LX,δ)中的全体Ir-开集,记以Ir(δ)。
定理2.2 设(LX,δ)是L-fts,A∈LX,若A∈δ,则 r∈P(L),A∈Ir(δ)。
即δ Ir (δ)。
Ir(δ)形成X上的一个LF拓扑,我们将(LX,Ir(δ))认定为Ir-层次拓扑空间。
Construction of fuzzy automata from fuzzy regular expressions

Available online at Fuzzy Sets and Systems199(2012)1–27/locate/fss Construction of fuzzy automata from fuzzy regular expressionsଁAleksandar Stamenkovi´c,Miroslav´Ciri´c∗University of Niš,Faculty of Sciences and Mathematics,Višegradska33,18000Niš,SerbiaReceived31May2011;received in revised form7January2012;accepted13January2012Available online4February2012AbstractLi and Pedrycz have proved fundamental results that provide different equivalent ways to represent fuzzy languages with mem-bership values in a lattice-ordered monoid,and generalize the well-known results of the classical theory of formal languages.In particular,they have shown that a fuzzy language over an integral lattice-ordered monoid can be represented by a fuzzy regular expression if and only if it can be recognized by a fuzzyfinite automaton.However,they did not give any efficient method for constructing an equivalent fuzzyfinite automaton from a given fuzzy regular expression.In this paper we provide such an efficient method.Transforming scalars appearing in a fuzzy regular expression into letters of the new extended alphabet,we convert the fuzzy regular expression to an ordinary regular expression R.Then,starting from an arbitrary nondeterministicfinite automaton A that recognizes the language R represented by the regular expression R,we construct fuzzyfinite automata A and A r with the same or even less number of states than the automaton A,which recognize the fuzzy language represented by the fuzzy regular expression .The starting nondeterministicfinite automaton A can be obtained from R using any of the well-known constructions for converting regular expressions to nondeterministicfinite automata,such as Glushkov–McNaughton–Yamada’s position automaton,Brzozowski’s derivative automaton,Antimirov’s partial derivative automaton,or Ilie–Yu’s follow automaton.©2012Elsevier B.V.All rights reserved.Keywords:Fuzzy automata;Fuzzy regular expressions;Nondeterministic automata;Regular expressions;Position automata;State reduction;Right invariant equivalences;Lattice-ordered monoids1.IntroductionStudy of fuzzy automata and languages was initiated in1960s by Santos[66–68],Wee[73],Wee and Fu[74], and Lee and Zadeh[45].From late1960s until early2000s mainly fuzzy automata and languages with membership values in the Gödel structure have been considered(see for example[25,28,54]).The idea of studying fuzzy automata with membership values in some structured abstract set comes back to Wechler[72],and in recent years researcher’s attention has been aimed mostly to fuzzy automata with membership values in complete residuated lattices,lattice-ordered moinoids,and other kinds of lattices.Fuzzy automata taking membership values in a complete residuated lattice werefirst studied in[61,62],where some basic concepts have been discussed,and later,extensive research of these fuzzy automata has been carried out in[63,64,75–79].From a different point of view,fuzzy automata with membershipଁResearch supported by Ministry of Education and Science,Republic of Serbia,Grant no.174013.∗Corresponding author.Tel.:+38118224492;fax:+38118533014.E-mail addresses:aca@pmf.ni.ac.rs(A.Stamenkovi´c),miroslav.ciric@.rs,mciric@pmf.ni.ac.rs(M.´Ciri´c).0165-0114/$-see front matter©2012Elsevier B.V.All rights reserved.doi:10.1016/j.fss.2012.01.0072 A.Stamenkovi´c,M.´Ciri´c/Fuzzy Sets and Systems199(2012)1–27values in a complete residuated lattice were studied in[20,21,31–34,70].Fuzzy automata with membership values ina lattice-ordered monoid have been investigated in[46,47,50,52],fuzzy automata over other types of lattices were thesubject of[24,42,43,49,51,56–59],and automata which generalize fuzzy automata over any type of lattices,as well asweighted automata over semirings,have been studied recently in[15,23,41].It is worth noting that fuzzy automata andlanguages are widely used in lexical analysis,description of natural and programming languages,learning systems,control systems,neural networks,clinical monitoring,pattern recognition,databases,discrete event systems,and manyother areas.Li and Pedrycz[50]have proved fundamental results that provide different equivalent ways to represent fuzzylanguages with membership values in a lattice-ordered monoid,e.g.,by fuzzyfinite automata,crisp-deterministicfuzzyfinite automata,fuzzy regular expressions,and fuzzy regular grammars.These results generalize the well-knownresults of the classical theory of formal languages.In particular,they have shown that a fuzzy language over an integrallattice-ordered monoid can be represented by a fuzzy regular expression if and only if it can be recognized by a fuzzyfinite automaton.However,Li and Pedrycz did not give any efficient method for constructing an equivalent fuzzyfiniteautomaton from a given fuzzy regular expression.The purpose of the present paper is to provide such an efficientmethod.Our basic idea is to convert a fuzzy regular expression into an ordinary regular expression R,transforming scalarsappearing in the fuzzy regular expression into letters of the new extended alphabet.Then,starting from an arbitrarynondeterministicfinite automaton A that recognizes the language R represented by the regular expression R,we construct a fuzzyfinite automaton A with the same number of states as the automaton A,which recognizes thefuzzy language represented by the fuzzy regular expression .Moreover,we construct a reduced version A r ofthe fuzzy automaton A ,a fuzzyfinite automaton which also recognizes the fuzzy language and can have evensmaller number of states than A .The method is generic,which means that it can be used in combination with anymethod for constructing a nondeterministicfinite automaton from an ordinary regular expression.In the past,manydifferent techniques for constructing nondeterministicfinite automata from regular expressions have been proposed.Besides Thompson’s construction[71],which builds nondeterministicfinite automata with -transitions,other well-known constructions build nondeterministicfinite automata without -transitions.The best known and most used suchconstructions are the position automaton,discovered independently by Glushkov[27]and McNaughton and Yamada[55],Brzozowski’s derivative automaton[6],Antimirov’s partial derivative automaton[1],and Ilie and Yu’s followautomaton[35–38].Each of these constructions can serve as a basis for the construction of our fuzzyfinite automata. More information on the algorithms for building small nondeterministicfinite automata from regular expressions can be found in[37].It should be noted that the same idea of treating scalars appearing in a fuzzy regular expression as the letters of a newextended alphabet,and then treating a fuzzy regular expression as an ordinary regular expression over a larger alphabet,has been recently used by Kuske[44]in the context of weighted regular expressions and weightedfinite automataover semirings.However,there are some significant differences between his and our approach.First,Kuske consideredonly weighted regular expressions that define proper power series,i.e.,power series with zero as the coefficient of theempty word.In terms of the theory of fuzzy languages,these are fuzzy languages which(absolutely)do not containthe empty word.There is one even more important difference.In the mentioned paper[44],Kuske gave a new proofof the famous Schützenberger’s theorem[26,65]which asserts that the behaviors of weightedfinite automata over anarbitrary semiring are precisely the rational formal power series,i.e.,formal power series defined by weighted regularexpressions.In his proof,Kuskefirst converts a weighted regular expression E to a regular expression E ,then he startsfrom an arbitrary deterministicfinite automaton that recognizes the language defined by E ,and from this automaton heconstructs a weightedfinite automaton whose behavior is the formal power series defined by E.However,the number ofstates of deterministicfinite automata obtained from regular expressions can be exponentially larger than the lengths ofthe corresponding regular expressions.For this reason,regular expressions are more often converted to nondeterministicfinite automata,and the above-mentioned constructions output nondeterministicfinite automata whose number of statesis equal to the length of the regular expression plus one,or even less than that number.In addition,our constructionsoutput fuzzyfinite automata with the same or even smaller number of states than the original nondeterministicfiniteautomaton.As we have said,the size of an automaton obtained from a regular expression plays a very important role,and for thatreason regular expressions are mostly converted to nondeterministicfinite automata.On the other hand,for practicalapplications deterministicfinite automata are usually needed,but determinization of a nondeterministicfinite automatonA.Stamenkovi´c,M.´Ciri´c/Fuzzy Sets and Systems199(2012)1–273 can cause an exponential blow up in the number of states.That is why the number of states of a nondeterministicfiniteautomaton has to be reduced prior to determinization.As the minimization of nondeterministicfinite automata iscomputationally hard,we must be satisfied with the methods for reducing the number of states that do not necessarilygive a minimal automaton,but rather provide a reasonably small automaton that can be efficiently computed.Suchreduction methods have been recently investigated in[8,10,36–40],in the context of nondeterministicfinite automata,and in[20,21,70],in the context of fuzzyfinite automata(see also[16,18,19]).A key role in the state reduction ofnondeterministicfinite automata is played by the right and left invariant equivalences,which have been generalizedin the fuzzy framework as right and left invariant fuzzy equivalences(cf.[20,21,70]).It is worth noting that the rightand left invariant(fuzzy)equivalences are also known as the forward and backward bisimulation(fuzzy)equivalences(cf.[16,18,19]).In particular,it has been proved in[11–13,35–38]that both the partial derivative automaton and thefollow automaton are factor automata of the position automaton with respect to certain right invariant equivalences.Thestate reduction of fuzzyfinite automata by means of right invariant fuzzy and crisp equivalences will be also consideredin this paper.Let us also note that the above-mentioned determinization problem has been recently investigated in thefuzzy framework in[3,15,32,41,50].Our main results are the following.We start from a given fuzzy regular expression over an alphabet X and alattice-ordered monoid L=(L,∧,∨,⊗,0,1,e),and we define an ordinary regular expression R over a newalphabet X∪Y,where Y consists of the letters associated with different scalars appearing in .The mapping of X∪Y to L,which maps all letters from X to e,and letters from Y to related scalars appearing in ,can be extended in a natural way to a homomorphism ∗ of the free monoid(X∪Y)∗to the monoid(L,⊗,e).In the casewhen L is an integral lattice-ordered monoid,using this homomorphism we establish a relationship between the fuzzylanguage represented by and the language R represented by R(cf.Theorem3.6),and starting from any nondeterministicfinite automaton A that recognizes the language R we define the fuzzy automaton A associated with A and ,and we prove that A recognizes the fuzzy language represented by the fuzzy regular expression (cf.Theorem3.7).However,the aforementioned definition of the fuzzy automaton A is not sufficiently constructive,because thecomputation of the fuzzy transition relation and the fuzzy set of terminal states of A requires the computation ofminimal words in certain infinite languages with respect to the embedding order,which might be a problem.We solvethis problem introducing a reflexive and transitive fuzzy relation R A on the set of states of the starting nondeterministicfinite automaton A,which can be efficiently computed as the n-th power of an easily computable fuzzy relation,wheren is the number of states of A.We express the fuzzy relation R A in terms of the homomorphism ∗ and the transitionrelation of A(cf.Theorem4.3),and then we express the fuzzy transition relation and the fuzzy set of terminal states of A in terms of the fuzzy relation R A,the transition relation of A,and the set of terminal states of A(cf.Theorem4.4). This result provides an efficient construction of the fuzzyfinite automaton A associated with A and the fuzzy regular expression .Using the fuzzy relation R A we also construct a version A r of the fuzzyfinite automaton A which can have evensmaller number of states than the fuzzy automaton A and the automaton A,and recognizes the same fuzzy language (cf.Theorem5.1).We show by an example that the number of states of A r can be strictly smaller than the number of states of A and A .We also discuss the state reduction of the fuzzy automaton A by means of right invariant crisp equivalences,and we show that even if the starting automaton A is a minimal deterministic automaton,the number of states of the fuzzy automaton A could be reduced.Finally,we describe certain properties of fuzzy automata obtained from the position and follow automaton.The structure of the paper is as follows.In Section2we recall some basic definitions and results concerning fuzzysets and relations over lattice ordered monoids,nondeterministic and fuzzy automata,and regular and fuzzy regularexpressions.In Section3we give the basic construction of a fuzzyfinite automaton A associated with a fuzzy regularexpression and a nondeterministicfinite automaton A recognizing the language R .Section4addresses the issue of the efficient construction of the fuzzy automaton A ,and in Section5we deal with the version of this construction that gives a fuzzy automaton with a reduced number of states with respect to the original construction.Finally,in Section6we discuss the problem of the reduction of the number of states of fuzzyfinite automata constructed from fuzzy regular expressions.Finally,note that our main results are proven under the assumption that the underlying -monoid is integral.Thisassumption is crucial in the proof Lemma3.5,and consequently,it is necessary in all the theorems that are based onLemma3.5and Theorem3.6(which also uses Lemma3.5).4 A.Stamenkovi´c ,M.´Ciri´c /Fuzzy Sets and Systems 199(2012)1–272.PreliminariesIn this section we recall some basic definitions and results concerning fuzzy sets and relations over lattice ordered monoids,nondeterministic and fuzzy automata,and regular and fuzzy regular expressions.ttice-ordered monoidsA lattice-ordered monoid or an -monoid [47,48,50,69]is an algebra L =(L ,∧,∨,⊗,0,1,e )such that(L1)(L ,∧,∨,0,1)is a lattice with the least element 0and the greatest element 1,(L2)(L ,⊗,e )is a monoid with the unit e ,(L3)x ⊗0=0⊗x =0,for every x ∈L ,(L4)x ⊗(y ∨z )=x ⊗y ∨x ⊗z ,(x ∨y )⊗z =x ⊗z ∨y ⊗z ,for all x ,y ,z ∈L .The operation ⊗is called the multiplication .In addition,if (L ,∧,∨,0,1)is a complete lattice and satisfies the following infinite distributive laws x ⊗ i ∈I x i = i ∈I (x ⊗x i ), i ∈I x i ⊗x = i ∈I(x i ⊗x ),(1)then L is called a quantale .In the general case,in an -monoid L =(L ,∧,∨,⊗,0,1,e )the greatest element 1of the lattice (L ,∧,∨,0,1)and the unit element e of the monoid (L ,⊗,e )are different.If 1and e coincide,then L is called an integral -monoid .Note that some authors understand the notion of a lattice-ordered monoid as having the multiplication distributive over the meet as well,i.e.,not only over the join,as we have in our definition.These concepts are different because the distributivity over the meet does not follow from the one over the join.It can be easily verified that with respect to ≤,the multiplication ⊗in an -monoid is isotone in both arguments,i.e.,for all x ,y ,z ∈L we havex ≤y implies x ⊗z ≤y ⊗z and z ⊗x ≤z ⊗y .(2)An integral quantale with commutative multiplication is known as a complete residuated lattice (cf.[4,5]).The most studied and applied kinds of complete residuated lattices,with the support [0,1],x ∧y =min(x ,y )and x ∨y =max(x ,y ),are the Lukasiewicz structure ,with the multiplication defined by x ⊗y =max(x +y −1,0),the Goguen or product structure ,with x ⊗y =x ·y ,and the Gödel structure ,with x ⊗y =min(x ,y ).The fourth important type of complete residuated lattices is the two-element Boolean algebra of classical logic with the support {0,1},called the Boolean structure .In the further text,if not noted otherwise,L will be an -monoid.A fuzzy subset of a set A is defined as any mapping from A into L .Ordinary crisp subsets of A are considered as fuzzy subsets of A taking membership values in the set {0,e }⊆L .Let f and g be two fuzzy subsets of A .The equality of f and g is defined as the usual equality of functions,i.e.,f =g if and only if f (x )=g (x ),for every x ∈A .The inclusion f ≤g is also defined pointwise:f ≤g if and only if f (x )≤g (x ),for every x ∈A .Endowed with this partial order the set L A of all fuzzy subsets of A forms a lattice,in which the meet (intersection)f ∧g and the join (union)f ∨g of any fuzzy subsets f ,g of A are also fuzzy subsets of A over L defined by(f ∧g )(x )=f (x )∧g (x ),(f ∨g )(x )=f (x )∨g (x ).(3)for each x ∈L .The crisp part of a fuzzy subset f ∈L A is a crisp subset f ={a ∈A |f (a )=e }of A .We will also consider f as a mapping f :A →L defined by f (a )=e ,if f (a )=e ,and f (a )=0,otherwise.A fuzzy relation on A is any fuzzy subset of A ×A .The equality,inclusion and ordering of fuzzy relations are defined as for fuzzy sets.For fuzzy relations R and S on a set A ,their composition R ◦S is the fuzzy relation on A defined by(R ◦S )(a ,b )=c ∈AR (a ,c )⊗S (c ,b ),(4)A.Stamenkovi´c,M.´Ciri´c/Fuzzy Sets and Systems199(2012)1–275 for all a,b∈A,and for a fuzzy subset f of A and a fuzzy relation R on A,the compositions f◦R and R◦f are fuzzy subsets of A defined,for any a∈A,by(f◦R)(a)=b∈A f(b)⊗R(b,a),(R◦f)(a)=b∈AR(a,b)⊗f(b).(5)For fuzzy subsets f and g we writef◦g=a∈Af(a)⊗g(a).(6) It is well known that the composition of fuzzy relations is associative.Moreover(f◦R)◦S=f◦(R◦S),(R◦S)◦f=R◦(S◦f),(f◦R)◦g=f◦(R◦g),(7) for all fuzzy subsets f and g of A,and fuzzy relations R and S on A.If A is afinite set with n elements,then R and S can be treated as n×n matrices over L,and R◦S is their matrix product,whereas f◦R can be treated as the product of the1×n matrix f and the n×n matrix R,and R◦f as the product of the n×n matrix R and the n×1matrix f t (the transpose of f).For afinite set A and an fuzzy relation R on A,a fuzzy relation R n is defined inductively as follows:R0is the crisp equality on A,and R n+1=R n◦R,for n∈N∪{0}.A fuzzy relation R on A is said to be(R)reflexive if R(a,a)=e,for every a∈A;(S)symmetric if R(a,b)=R(b,a),for all a,b∈A;(T)transitive if for all a,b,c∈A we have R(a,b)⊗R(b,c)≤R(a,c).It is easy to check that a reflexive fuzzy relation R is transitive if and only if R2=R,and then R n=R,for every n∈N.A reflexive,symmetric and transitive fuzzy relation is called a fuzzy equivalence.For a fuzzy equivalence E on A and a∈A we define a fuzzy subset E a of A by E a(x)=E(a,x),for every x∈A.We call E a the equivalence class of E determined by a.The set A/E={E a|a∈A}is called the factor set of A with respect to E(cf.[4,5,17]).We use the same notation for crisp equivalences,i.e.,for an equivalence on A,the related factor set is denoted by A/ ,the equivalence class of an element a∈A is denoted by a.A fuzzy equivalence E on a set A is called a fuzzy equality if for all x,y∈A,E(x,y)=e implies x=y.In other words,E is a fuzzy equality if and only if its crisp part E is a crisp equality.2.2.Fuzzy regular expressionsLet X be a non-empty set,which is called an alphabet and whose elements are called letters,and let X∗be the free monoid over X,i.e.,the set of allfinite sequences of letters from X,including the empty sequence,equipped with the concatenation operation.Elements of X∗are called words,and the empty sequence is denoted by and called the empty word.A fuzzy language in X∗is defined as any fuzzy subset of X∗.A language in X∗is a fuzzy language in X∗taking membership values in the set{0,e}.For a fuzzy language f and a scalar ∈L,the scalar multiplication ⊗f is the fuzzy language in X∗defined by( ⊗f)(u)= ⊗f(u),for any u∈X∗.The union(join)f∨g of fuzzy languages f and g is defined as the union of fuzzy subsets f and g. The concatenation(product)fg of fuzzy languages f and g is defined by(f g)(u)=u=vwf(v)⊗g(w).6 A.Stamenkovi´c ,M.´Ciri´c /Fuzzy Sets and Systems 199(2012)1–27The concatenation of fuzzy languages is an associative operation,and for n ∈N ,the n-th power of a fuzzy language f is defined inductively by f 0=f ,where f is a characteristic function of the empty word ,i.e.,f (u )= e if u = ,0otherwise ,(8)and f n +1=f n f ,for each n ∈N ∪{0}.The Kleene closure of a fuzzy language f ,denoted by f ∗,is defined byf =n ∈N ∪{0}f n .Recall the following result proved in [50].Proposition 2.1.If L is an integral -monoid ,then for any fuzzy language f ,the Kleene closure is well defined .The family LR of fuzzy regular expressions over a finite alphabet X is defined inductively in the following way (cf.[47,50]):(i)∅∈LR ;(ii)∈LR ;(iii)x ∈LR ,for all x ∈X ;(iv)( )∈LR ,for all ∈L and ∈LR (scalar multiplication );(v)( 1+ 2)∈LR ,for all 1, 2∈LR (addition );(vi)( 1 2)∈LR ,for all 1, 2∈LR (concatenation );(vii)( ∗)∈LR ,for all ∈LR (star operation );(viii)There are no other fuzzy regular expressions than those given in steps (i)–(viii).In order to avoid parentheses it is assumed that the star operation has the highest priority,then concatenation and then addition.For any fuzzy regular expression ∈LR ,the fuzzy language determined by is defined inductively as follows (cf.[47,50]):(i) ∅ (u )=0,for every u ∈X ∗;(ii)For ∈X ∪{ }, =f ,where f is the characteristic function of defined byf (u )= e if u = ,0otherwise ;(iii)= ⊗ for all ∈L and ∈LR ;(iv)( 1+ 2) = 1 ∨ 2 ,for all 1, 2∈LR ;(v)( 1 2) = 1 2 ,for all 1, 2∈LR ;(v) ∗ = ∗,for all ∈LR .For a fuzzy regular expression over X ,by | |X we denote the number of occurrences of letters from X in .The length of ,denoted by | |,is the sum | |=| |X +| |L ,where | |L is the number of scalars appearing in .A fuzzy regular expression which does not contain any occurrence of an element of L is called a regular expression over an alphabet X .In other words,regular expressions are fuzzy regular expressions without scalar multiplication.Consequently,the length of a regular expression is equal to | |X .Note also that the fuzzy language defined by a regular expression takes membership values in the set {0,e },and thus,it can be considered as an ordinary subset of X ∗.For the free monoid X ∗we set X +=X ∗\{ }.The length of a word u ∈X ∗,in notation |u |,is the number of appearances of the letters from X in u .The embedding order relation ≤em is defined on X ∗byu ≤em v ⇔u =u 1u 2···u n and v =v 0u 1v 1u 2···v n −1u n v n ,(9)where n ∈N and u ,v,u 1,u 2,...,u n ,v 0,v 1,...,v n ∈X ∗.A.Stamenkovi´c ,M.´Ciri´c /Fuzzy Sets and Systems 199(2012)1–277Proposition 2.2(Haines [29],Higman [30]).For any finite alphabet X ,≤em is a partial order on X ∗.Any set of pairwise incomparable words in the partially ordered set (X ∗,≤em )is finite .Consequently,for any U ⊆X ∗,the set M (U )of all minimal words from U with respect to ≤em is finite.Throughout the paper,the set of all minimal words from U ⊆X ∗with respect to the embedding order ≤em will be denoted by M (U ),as in the previous proposition.2.3.Fuzzy automataLet L be an -monoid.A fuzzy automaton (over L )is defined as a five-tuple A =(A ,X , A , A , A ),where A and X are non-empty sets,called respectively the set of states and the input alphabet , A :A ×X ×A →L is a fuzzy subset of A ×X ×A ,called the fuzzy transition relation , A ∈L A is the fuzzy set of initial states ,and A ∈L A is the fuzzy set of terminal states .We will assume that the input alphabet X is always finite.A fuzzy automaton whose set of states is finite is called a fuzzy finite automaton .Since all fuzzy automata considered in this paper will be finite,we will speak simply fuzzy automaton instead of fuzzy finite automaton.Cardinality of a fuzzy automaton A ,in notation |A |,is defined as the cardinality |A |of its set of states A .The fuzzy transition relation A can be extended up to a mapping A ∗:A ×X ∗×A →L in the following way:If a ,b ∈A and ∈X ∗is the empty word,then A ∗(a , ,b )= e if a =b ,0otherwise ,(10)and if a ,b ∈A ,u ∈X ∗and x ∈X ,then A ∗(a ,ux ,b )= c ∈AA ∗(a ,u ,c )⊗ A (c ,x ,b ).(11)Without danger of confusion we shall write just A instead of A ∗.By (L4)and Theorem 3.1in [50]we have that A (a ,u v,b )=c ∈A A (a ,u ,c )⊗ A (c ,v,b ),(12)for all a ,b ∈A and u ,v ∈X ∗.For any u ∈X ∗we define a fuzzy relation A u ∈L A ×A ,called the fuzzy transition relation determined by u ,by A u (a ,b )= A (a ,u ,b ),for all a ,b ∈A .Then for all u ,v ∈X ∗,the equality (12)can be written as A u v = A u ◦ A v .The fuzzy language recognized by a fuzzy automaton A =(A ,X , A , A , A ),denoted by L (A ),is the fuzzy language in X ∗defined by L (A )(u )=a ,b ∈AA (a )⊗ A (a ,u ,b )⊗ A (b ),(13)or equivalently,L (A )(u )= A ◦ A u ◦ A = A ◦ A x 1◦ A x 2◦···◦ A x n ◦ A ,(14)for any u =x 1x 2...x n ∈X ∗with x 1,x 2,...,x n ∈X .In particular,if A =(A ,X , A ,a 0, A )is a fuzzy automaton having a single crisp initial state a 0,then the fuzzy language L (A )recognized by A is given by L (A )(u )=a ∈AA (a 0,u ,a )⊗ A (a ),(15)or equivalently,L (A )(u )=( A u ◦ A )(a 0)=( A x 1◦ A x 2◦···◦ A x n ◦ A )(a 0),(16)for any u =x 1x 2...x n ∈X ∗with x 1,x 2,...,x n ∈X .8 A.Stamenkovi´c,M.´Ciri´c/Fuzzy Sets and Systems199(2012)1–27In the further text,ordinary nondeterministic automata will be considered as fuzzy ly,by a nonde-terministic automaton we mean a fuzzy automaton A=(A,X, A, A, A)such that A x is a fuzzy relation taking values in the set{0,e},for each x∈X,and A and A are fuzzy sets also taking values in{0,e}.In this case,the fuzzy language recognized by A is a crisp language,and it is exactly the language recognized by a nondeterministic automaton in the sense of the well-known definition from the classical theory of nondeterministic automata.Let A=(A,X, A, A, A)be a fuzzy automaton and let E be a fuzzy equivalence on A.Without any restriction on the fuzzy equivalence E,we define a fuzzy transition relation A/E:A/E×X×A/E→L by A/E(E a,x,E b)=a ,b ∈AE(a,a )⊗ (a ,x,b )⊗E(b ,b)=(E◦ x◦E)(a,b)=E a◦ x◦E b,(17) and fuzzy sets A/E∈L A/E and E∈L A/E of initial and terminal states byA/E(E a)=a ∈AA(a )⊗E(a ,a)=( A◦E)(a)= A◦E a,(18)A/E(E a)=a ∈AA(a )⊗E(a ,a)=( A◦E)(a)= A◦E a,(19)for any a∈A.Evidently, A/E, A/E and A/E are well-defined,and A/E=(A/E,X, A/E, A/E, A/E)is a fuzzy automaton,called the factor fuzzy automaton of A with respect to E.2.4.Position automataIn this section we recall the construction of the position automaton from a regular expression[27,55].Let be a regular expression over an alphabet X.Denote by the expression obtained from by marking each letter in with its position.For example,if =x+xy∗,then =x1+x2y∗3.The same notation will be used for removing indices,that is,for a regular expression we put = .We define the following sets:(i)pos0( )={0,1,...,| |X},(ii)f irst( )={i|x i u∈ },(iii)last( )={i|ux i∈ },(iv)f ollo w( ,i)={j|ux i x j v∈ },i>0,(v)f ollo w( ,0)=f irst( ),(vi)last0( )=last( ), /∈ , last( )∪{0}, ∈ .Define pos⊆pos0( )×X×pos0( )by(i,x,j)∈ pos⇔x j=x and j∈f ollo w( ,i).Then A pos( )=(pos0( ),X, pos,0,last0( ))is a nondeterministic automaton called the position automaton of . It was shown by Glushkov[27]and McNaughton and Yamada[55]that L(A pos( ))= .For the sake of simplicity,instead of A pos( )=(pos0( ),X, pos,0,last0( )),in the further text we will write A p( )=(A p,X, A p,0, A p).Let us illustrate the construction of the position automaton by the following simple example.Consider again the regular expression =x+xy∗.The marked version of this expression is =x1+x2y∗3. Next,we have the following:(i)A p=pos0( )={0,1,2,3}is the set of states of A p( ),(ii)f irst( )=f ollo w( ,0)={1,2},(iii)last( )=last0( )={1,2,3},(iv)f ollo w( ,1)=∅,f ollo w( ,2)=f ollo w( ,3)={3}.The transition set pos in this example is pos={(0,x,1),(0,x,2),(2,y,3),(3,y,3)}.。
On interval fuzzy S-implications

On interval fuzzy S-implicationsB.C.Bedregal a,*,G.P.Dimuro b ,R.H.N.Santiago a ,R.H.S.Reiser ca Universidade Federal do Rio Grande do Norte,Departamento de Informática e Matemática Aplicada,Campus Universitário,59072-970Natal,BrazilbUniversidade Federal do Rio Grande,Programa de Pós-Graduação em Modelagem Computacional,Campus Carreiros,96201-090Rio Grande,Brazilc Universidade Católica de Pelotas,Programa de Pós-Graduação em Informática,Rua Felix da Cunha 412,96010-000Pelotas,Brazil a r t i c l e i n f o Article history:Received 6March 2008Received in revised form 21August 2009Accepted 21November 2009Keywords:Fuzzy logic Interval mathematicsInterval representationInterval fuzzy implicationInterval S-implicationInterval automorphisma b s t r a c tThis paper presents an analysis of interval-valued S-implications and interval-valued auto-morphisms,showing a way to obtain an interval-valued S-implication from two S-implica-tions,such that the resulting interval-valued S-implication is said to be obtainable .Someconsequences of that are:(1)the resulting interval-valued S-implication satisfies the cor-rectness property,and (2)some important properties of usual S-implications are preservedby such interval representations.A relation between S-implications and interval-valued S-implications is outlined,showing that the action of an interval-valued automorphism on aninterval-valued S-implication produces another interval-valued S-implication.Ó2009Elsevier Inc.All rights reserved.1.IntroductionFuzzy set theory was introduced by Zadeh [76],allowing the development of soft computing techniques centered on the idea that computation,reasoning and decision making should exploit,whenever possible,the tolerance for imprecision and uncertainty [78].Like classical set theory,the corresponding fuzzy logic has been developed as formal deductive systems,but with a com-parative notion of truth that formalizes deduction under vagueness.It provides tools for approximate reasoning and decision making together with a framework to deal with imprecision,uncertainty,incompleteness of information,conflicting infor-mation,partiality of truth and partiality of possibility [79],improving the design of flexible information processing systems[51].It has been applied in several areas,such as control systems [18],decision making [17],expert systems [67],pattern recognition [19,50],etc.On the other hand,fuzzy logic may be viewed as an attempt to formalize/mechanize the human capability to perform a wide variety of physical and mental tasks without any measurements or computations [79].Fuzzy sets were originally defined by membership functions of the form l A :X !½0;1 ,where any membership degree l A ðx Þwas a precise number.However,in some situations,we do not have precise knowledge about the membership function (or the membership degree)that should be taken into account.This consideration has led to some extensions of fuzzy sets,giving rise to type-n fuzzy sets [77],which incorporated uncertainty about membership functions and membership degrees into fuzzy set theory,where the ‘‘precise number”representing a membership degree was generalized to a value carrying its uncertainty.0020-0255/$-see front matter Ó2009Elsevier Inc.All rights reserved.doi:10.1016/j.ins.2009.11.035*Corresponding author.Tel.:+558432153814;fax:+558432153813.E-mail addresses:bedregal@dimap.ufrn.br (B.C.Bedregal),gracaliz@ (G.P.Dimuro),regivan@dimap.ufrn.br (R.H.N.Santiago),reiser@ucpel.tche.br (R.H.S.Reiser).Information Sciences 180(2010)1373–1389Contents lists available at ScienceDirectInformation Sciencesj o u r n a l ho m e p a g e :w w w.e l s e vier.c om/loc ate/ins1374 B.C.Bedregal et al./Information Sciences180(2010)1373–1389Type-2fuzzy sets have been largely applied since the works of Jerry Mendel[48]in the90s,with the increase of the the-oretical research on their properties[49,71].Interval-valued fuzzy sets are a particular case of type-2fuzzy sets with a rich structure provided by Interval Mathematics[52].Interval Mathematics is a mathematical theory that aims at the representation of uncertain input data and parameters, originally interested in the automatic and rigorous control of the errors that arise in numerical computations[37].It has been applied to deal with the uncertainties in the results of numerical algorithms in Engineering and Scientific Computing,with contributions1/interval-comp/.in several areas[40,41],such as electrical power systems[8],mechan-ical engineering[54],chemical engineering[68],artificial intelligence[38],multiagent systems[26]and geophysics[2].Interval mathematics is another form of information theory which is related to,but independent from,fuzzy logic.In one hand,intervals can be considered to be a particular type of fuzzy set.On the other hand,interval membership degrees can be used to represent the uncertainty and the difficulty of an expert to precisely determine the fairest membership degree of an element with respect to a linguistic term,as considered in interval-valued fuzzy sets.In this case,the radius of an interval is used as an error measure[57],providing an estimation of the uncertainty during membership assignment.Interval degrees can also be viewed as summarizing the opinions of several experts about the exact membership degree for an element with respect to a linguistic term.In this case,the left and right interval endpoints are,respectively,the least and the greatest de-grees provided by a group of experts[29,57,70].In both cases,the richness of interval structures provides tools to deal with such notions of uncertainty.Interval-valued fuzzy sets were introduced independently by Zadeh[77]and other authors in the70s(e.g.,[36,39,63]), allowing to deal not only with vagueness(lack of sharp class boundaries),but also with uncertainty(lack of information) [29,45].Since then,the integration of fuzzy theory with interval mathematics has been studied from different viewpoints, as properly pointed out by Lodwick[45],generating several different approaches(as in[21,27–29,33,44,45,53,56,57,71,75]).In this paper,we follow the approachfirst introduced in Bedregal and Takahashi’s works[12,13],which has already been applied in our previous papers,where we provided interval extensions for some fuzzy connectives(see[9,15,25,59])by con-sidering both correctness(accuracy)and optimality aspects of interval methods[37,64].In particular,we are interested in the investigations of interval extensions of the various types of fuzzy implications and their related properties.Fuzzy implications[4–7,11,31,46,47,58,61,62,66,65,74]play an important role in fuzzy logic.In a broad sense,fuzzy implications are important not only because they are used to formalize‘‘If...then”rules in fuzzy systems,but also because they have different meanings(e.g.,S-implications,R-implications,QL-implications,D-implications etc.)to be used in per-forming inferences in approximate reasoning and fuzzy control[46].The role of fuzzy implications on the development of applications also motivates the research in the narrow sense through the investigation of related logical aspects[47].The aim of this work is to introduce an interval generalization for a particular meaning of fuzzy implications,namely,S-implications[5,6,16,47,61,30,31].This generalization,which we call interval S-implication,satisfies the correctness property mentioned above.We present an analysis of interval S-implications and interval automorphisms,showing a way to obtain an interval S-implication from two S-implications,so that the resulting interval S-implication is said to be obtainable.We prove that interval S-implications are closed under the action of the interval-valued automorphisms introduced in[33,34].We also prove that several analogous important properties of S-implications are also valid for interval S-implications,showing their applicability on interval-based fuzzy systems.Thus,this work is an important step towards the fundamentals for the devel-opment of such interval-based fuzzy systems.The paper is organized as follows:Section2discusses the notions of interval representations of real functions providing the related definitions and results.The main results related to the interval extensions of fuzzy t-conorms introduced in pre-vious works[12–14]are presented in Section3.In Section4,we discuss the interval extensions of fuzzy negations.A brief review about fuzzy implications,and,in particular,S-implications,is presented in Section5,where the main properties of S-implications are presented.Section6introduces interval fuzzy implications and the definition of interval S-implications, showing that several analogous properties of S-implications also hold for interval S-implications.The action of an interval automorphism on an interval S-implication is analyzed in Section7.Section8concludes this paper,summarizing its main results,presenting somefinal remarks and pointing out future works.2.Interval representationsConsider the real unit interval U¼½0;1 #R and the set U¼f½a;b j06a6b61g of subintervals of U.The left and right projections of an interval½a;b 2U are given by the functions l;r:U!U,defined,respectively,bylð½a;b Þ¼a and rð½a;b Þ¼b:ð1ÞFor a given interval X2U;lðXÞand rðXÞare also denoted,respectively,by X and X.The following partial orders play important roles in this paper:(i)The product order(also called component-wise order or Kulisch-Miranker order),defined,for all X;Y2U,by:X6Y()X6Y^X6Y;ð2Þ1For a survey on applications of Interval Mathematics,see,e.g.,/interval-comp/.(ii)The inclusion order,defined,for all X ;Y 2U ,by:X #Y ()X P Y ^X 6Y :ð3ÞThese partial orders can be naturally extended to U n .For example,considering the product order defined in Eq.(2),forany ~X ¼ðX 1;...;X n Þ;~Y ¼ðY 1;...;Y n Þ2U n ,one has that ~X 6~Y ()X 16Y 1^ÁÁÁ^X n 6Y n :ð4ÞAn interval function F :U n !U is said to be strictly increasing if,for each ~X ;~Y 2U n ,whenever ~X <~Y (that is,~X 6~Y and ~X –~Y )it holds that F ð~X Þ<F ð~Y Þ.The notion of interval correctness plays a very important role in numerical computations [64].A correct interval method can always guarantee that if x 2X then f ðx Þ2F ðX Þ,where F is the interval method that evaluates a real function f .In [64],the notion of correctness is formalized by the so-called Interval Representation ,considering that interval methods are represen-tations of punctual methods.In what follows,we reproduce such definition,but,instead of considering the set of real num-bers R ,we consider the set U ¼½0;1 #R .Definition 1[64].An interval X 2U is said to be a representation for a real number a if a 2X .Considering two interval representations X and Y for a real number a ;X is said to be a better interval representation of a than Y ,denoted by Y v X ,if X #Y .The notion of better interval representation can also be easily extended for n -tuples of intervals.Definition 2[64].A function F :U n !U is said to be an interval representation of a real function f :U n !U if,for each ~X 2U n and ~x 2~X ;f ð~x Þ2F ð~X Þ.F is also said to be correct with respect to f .An interval function F :U n !U is said to be a better interval representation of a real function f :U n !U than an intervalfunction G :U n !U ,denoted by G v F ,if F ð~X Þ#G ð~X Þ,for each ~X 2U n [64].In [64],the notion of optimality of interval methods was formalized by the so-called canonical interval representations of real functions,also known by the best interval representations [12]of real functions:Definition 3[64].The best interval representation of a real function f :U n !U is the interval function b f :U n !U ,defined byb f ð~X Þ¼½inf f f ð~x Þj ~x 2~X g ;sup f f ð~x Þj ~x 2~X g :ð5ÞNotice that the interval function b f is well defined and it is clearly an interval representation of f .Moreover,for any other interval representation F of f ,F v b f .This means that b f always returns a narrower interval than the intervals produced by any other interval representation of f .Thus,b f has the optimality property of interval algorithms mentioned by Hickey et al.[37],when it is seen as an algorithm to compute a real function f .Observe that if the real function f is continuous in the usual sense then,for each ~X 2U n ,one has thatb f ð~X Þ¼f f ð~x Þj ~x 2~X g ¼f ð~X Þ;ð6Þthat is,the best interval representation b f of a real function f coincides with its range [64].Definition 4.An interval function F :U n !U is obtainable if there exist projections P 1;...;P 2n :U !U ,where P i 2f l ;r g ,for i ¼1;...;2n ,and functions f 1;f 2:U n !U such that,for each X 1;...;X n 2U ,it holds thatF ðX 1;...;X n Þ¼½f 1ðP 1ðX 1Þ;...;P n ðX n ÞÞ;f 2ðP n þ1ðX 1Þ;...;P 2n ðX n ÞÞ :ð7ÞThe concept of obtainable function generalizes the notion of representable function,as proposed by Deschrijver et al.[22–24,32]in the context of interval t-norms.On the other hand,observe that every obtainable interval function F is an interval representation of some real function f (at least f 1and f 2).However,the converse is not true.For example,the interval functionF :U n !U ,defined by F ðX Þ¼½max ð0;X ÀX Þ;min ð1;X þX 10Þ ,is an interval representation of the identity on U ,id U ðx Þ¼x ,but F is not obtainable.An interval function F :U n !U preserves degenerate intervals ,if it maps degenerate intervals into degenerate intervals,that is,if,for each x 1;...;x n 2U ,there exists y 2U such that F ð½x 1;x 1 ;...;½x n ;x n Þ¼½y ;y .Notice that the best interval representation of any real function is #-monotonic (inclusion-monotonic),obtainable and preserves degenerate intervals.In this paper,we adopt the following notions of continuity defined on the set U of subintervals of ½0;1 :(i)Moore continuity [52]:is defined as an extension of the continuity on the set of the real numbers by considering themetric given by the distance between two intervals X ;Y 2U ,which is defined by:d ðX ;Y Þ¼max fj X ÀY j ;j X ÀY jg .(ii)Scott continuity:is defined as an extension of the continuity on the set of the real numbers,considering the quasi-met-ric q ðX ;Y Þ¼max f Y ÀX ;X ÀY ;0g defined over U ,introduced in [1,64].An alternative way to define the Scott continu-ity on U is to consider the set U with the reverse inclusion order as a continuous domain [35],and a function B.C.Bedregal et al./Information Sciences 180(2010)1373–138913751376 B.C.Bedregal et al./Information Sciences180(2010)1373–1389f:ðU; Þ!ðU; Þis said to be Scott-continuous if it is monotonic and preserves the least upper bound of directed sets[35].2The main result in[64]can be adapted to our context,considering the set U instead of R,as shown in the following: Theorem5.Let f:U n!U be a real function.The following statements are equivalent:(i)f is continuous;(ii)b f is Scott-continuous;(iii)b f is Moore-continuous.3.Interval t-conormsA triangular conorm(t-conorm for short)is a function S:U2!U that is commutative,associative,monotonic and has an identity‘‘0”,generalizing the classical disjunction.Among several t-conorms,in this paper,we consider the maximum t-con-orm S M:U!U,defined asS Mðx;yÞ¼max f x;y g:ð8ÞAn interval generalization of t-conorms was introduced in[13],applying the principles discussed in Section2.The so-called interval t-conorm is defined as an interval representation of a t-conorm.This generalizationfits the idea of interval member-ship degrees as approximations of exact degrees.Definition6[13].A function S:U2!U is an interval t-conorm,whenever it is commutative,associative,monotonic with respect to the product and inclusion orders,and½0;0 is the identity element.In the following,the main results related to interval t-conorms are presented.Proposition7[13,Theorems5.1and5.2].If S is a t-conorm,then its best interval representation b S:U2!U is an interval t-conorm.For example,the supremum interval t-conorm S M:U2!U,defined byS MðX;YÞ¼sup f X;Y g;ð9Þis the best interval representation of the maximum t-conorm S Mðx;yÞ,given in Eq.(8),that is,S M¼c S M.3Proposition8[14,Corollary 5.3].The function S:U2!U is an interval t-conorm if,and only if,the real functions S;S:U2!U,defined bySðx;yÞ¼lðSð½x;x ;½y;y ÞÞand Sðx;yÞ¼rðSð½x;x ;½y;y ÞÞ;ð10Þare t-conorms andSðX;YÞ¼½SðX;YÞ;ð;;ð11Þwhere l and r are,respectively,the left and right projections defined in Eq.(1).Therefore,one has that interval t-conorms are obtainable.The following result is immediate:Corollary9.Let S:U2!U be an interval t-conorm and S:U2!U be a t-conorm.If S represents S then S6S6S. Given a t-conorm S,the interval t-conorm b S can be expressed by:b SðX;YÞ¼½SðX;YÞ;SðX;YÞ :ð12Þ4.Interval fuzzy negationsLike t-conorms,fuzzy negations generalize the classical negations.A function N:U!U is a fuzzy negation ifN1:Nð0Þ¼1and Nð1Þ¼0;N2:If x P y then NðxÞ6NðyÞ;8x;y2U.Fuzzy negations satisfying the involutive property N3are called strong fuzzy nega-tions[16,43]:N3:NðNðxÞÞ¼x;8x2U.In addition,a continuous fuzzy negation is strict whenN4:If x>y then NðxÞ<NðyÞ;8x;y2U.2A directed set ofðU; Þis a non-empty subset S#U such that every pair of intervals in S has an upper bound in S.3sup denotes the supremum related to the Kulisch-Miranker or product order.B.C.Bedregal et al./Information Sciences180(2010)1373–13891377As is well known,all strong fuzzy negations are strict.An element e2U is said to be an equilibrium point of a fuzzy negation N whenever NðeÞ¼e.If N is a strict fuzzy negation, then there exists a unique equilibrium point e N2U and it holds that NðxÞP e N,for all x6e N.Conversely,one has that NðxÞ6e N,for all x P e N.Definition10.An interval function N:U!U is an interval fuzzy negation if,for all X;Y in U,the following properties hold: N1:Nð½0;0 Þ¼½1;1 and Nð½1;1 Þ¼½0;0 ;N2a If X P Y then NðXÞ6NðYÞ;N2b If X#Y then NðXÞ#NðYÞ.If N also satisfies the involutive property N3,then it is said to be a strong interval fuzzy negation:N3:NðNðXÞÞ¼X;8X2U.A Moore and Scott-continuous interval fuzzy negation N is strict if it also satisfies the following properties:N4a If X<Y then NðYÞ<NðXÞ;N4b If X&Y then NðXÞ&NðYÞ.The concepts of interval representation and obtainability show their strength on the context of fuzzy negations in the fol-lowing results.We show that those concepts guarantee that punctual properties are preserved by the interval generalization of fuzzy negations.Let N:U!U be a fuzzy negation.The interval function b N can be expressed as:b NðXÞ¼½NðXÞ;NðXÞ :ð13ÞThe proofs of the next propositions in this section can be found in[10].Proposition11.A function N:U!U is a(strict)interval fuzzy negation if,and only if,the functions N;N:U!U,defined, respectively,byNðxÞ¼lðNð½x;x ÞÞand NðxÞ¼rðNð½x;x ÞÞ;ð14Þare(strict)fuzzy negations andNðXÞ¼½NðXÞ;NðXÞ ;ð15Þwhere l and r are,respectively,the left and right projections defined in Eq.(1).Remark12.If N¼N,then NðXÞ¼b NðXÞ¼b NðXÞ.Therefore,one has that(strict)interval fuzzy negations are obtainable.Proposition13.A function N:U!U is a strong interval fuzzy negation if,and only if,there exists a strong fuzzy negation N such that N¼b N.From Eq.(13)and Remark12,if the conditions of Proposition13hold,then it follows that N¼N¼N.From Propositions11and13,it is immediate that:Corollary14.Let N:U!U be a fuzzy negation.Then b N is an interval fuzzy negation.In addition,if N is a strong(strict)fuzzy negation then b N is a strong(strict)interval fuzzy negation.An interval E2U is an equilibrium point of an interval fuzzy negation N if NðEÞ¼E.Trivially,½0;1 is an equilibrium point of any interval fuzzy negation.Thus,if an equilibrium interval E is such that E–½0;1 then E is said to be a non-trivial equi-librium point.Proposition15.If N is a strong interval fuzzy negation,then N has a degenerate equilibrium.Moreover,it is the unique non-trivial equilibrium point.5.Fuzzy implications and S-implicationsSeveral definitions for fuzzy implications together with related properties have been given(see, e.g.,[4–7,16,30,31,46,47,58,61,60,62,66,65,72–74]).However,there is just one consensus on what a fuzzy implication should be, namely:‘‘a fuzzy implication should present the behavior of the classical implication when the crisp case is considered”[47].In other words,a function I:U2!U is a fuzzy implication whenever it satisfies the minimal boundary conditions:Ið1;1Þ¼Ið0;1Þ¼Ið0;0Þ¼1and Ið1;0Þ¼0:ð16ÞSeveral reasonable properties may be required for fuzzy implications,among them we consider the following: I1:If x6z then Iðx;yÞP Iðz;yÞ(first place antitonicity);I2:If y6z then Iðx;yÞ6Iðx;zÞ(second place isotonicity);I3:Ið1;xÞ¼x(left neutrality principle);I4:Iðx;Iðy;zÞÞ¼Iðy;Iðx;zÞÞ(exchange principle);I5:Iðx;yÞ¼Iðx;Iðx;yÞÞ(iterative boolean-like law);I6:Iðx;NðxÞÞ¼NðxÞ,where N is a strong fuzzy negation;I7:NðxÞ¼Iðx;0Þis a strong fuzzy negation;I8:Iðx;1Þ¼1(dominance of truth of consequent);I9:Iðx;yÞP y;I10:Iðx;yÞ¼IðNðyÞ;NðxÞÞ,where N is a strong fuzzy negation(contra-positive);I11:Ið0;xÞ¼1(dominance falsity).Some relations between classical implications and negations can be recovered for the fuzzy case.For example,if I:U2!U is a fuzzy implication satisfying the Property I1,then there is a fuzzy negation N I:U!U that can be defined by[6,Lemma2.1]:N IðxÞ¼Iðx;0Þ:ð17ÞAnother relation between negation and implication follows the opposite direction,showing that it is possible to define a fuz-zy implication from a fuzzy negation.Let S be a t-conorm and N be a fuzzy negation.An S-implication[5,6,16,30,31,47,61]is a fuzzy implication I S;N:U2!U defined byI S;Nðx;yÞ¼SðNðxÞ;yÞ:ð18ÞIn some texts(e.g.,[16,30,31]),the definition of an S-implication requires a strong fuzzy negation.Such S-implications are called here strong S-implications.Similar definitions can be introduced for continuous S-implications and strict S-implications.Trillas and Valverde[69,Theorem3.2](see also[30,Theorem1.13]and[6,Theorem1.6])provided the following char-acterization for strong S-implications:a function I:U2!U is a strong S-implication if,and only if,it satisfies the Properties I1–I4,and tely,Baczynsky and Jayaram[6,Theorem2.6])introduced a new characterization of strong S-implications, considering properties I1,I4and I7.Strong S-implications also satisfy the properties I8–I11and the following two extra properties below:I12:Iðx;yÞP N IðxÞ;I13:Iðx;yÞ¼0if,and only if,x¼1and y¼0.Notice that any S-implication I S;N satisfies the properties I1–I3,I8,I9,and I11.If a fuzzy implication I is an S-implication then N I,as given in Eq.(17),is the underlying negation of I,that is: N IS;NðxÞ¼I S;Nðx;0Þ¼SðNðxÞ;0Þ¼NðxÞ:ð19ÞTherefore,N I is a strict fuzzy negation if,and only if,I is a strict S-implication.Baczynsky and Jayaram[6,Theorem5.2]provided a characterization for strict S-implications,where an S-implication I S;N is strict if and only if N IS;Nis strict and the properties I1and I10also hold.If a fuzzy implication I:U2!U is a strong S-implication,then I satisfies I6if,and only if,the underlying t-conorm of I isthe maximum t-conorm S M,given in Eq.(8),and,therefore,one has I¼I SM;N ,where N is a strong fuzzy negation.The strong S-implication I SM ;Nalso satisfies the properties I1–I11.Moreover,it is the only S-implication satisfying I6.Given an equilibriumpoint e N,if x2U and x P e N then one has that I SM;Nðx;xÞ¼x.6.Interval fuzzy implicationsSince real numbers may be identified with degenerate intervals in the context of interval mathematics,the boundary con-ditions that must be satisfied by the classical fuzzy implications can be naturally extended to interval fuzzy degrees,when-ever degenerate intervals are considered.Then,a function I:U2!U is said to be an interval fuzzy implication if the following interval-based boundary conditions hold:(i)Ið½1;1 ;½1;1 Þ¼Ið½0;0 ;½0;0 Þ¼Ið½0;0 ;½1;1 Þ¼½1;1 ;(ii)Ið½1;1 ;½0;0 Þ¼½0;0 .The properties presented in Section5can then also be naturally extended to an interval-based approach:I1:If X6Z then IðX;YÞP IðZ;YÞ(first place antitonicity);I2:If Y6Z then IðX;YÞ6IðX;ZÞ(second place isotonicity);1378 B.C.Bedregal et al./Information Sciences180(2010)1373–1389I 3:I ð½1;1 ;X Þ¼X (left neutrality principle);I 4:I ðX ;I ðY ;Z ÞÞ¼I ðY ;I ðX ;Z ÞÞ(exchange principle);I 5:I ðX ;Y Þ¼I ðX ;I ðX ;Y ÞÞ(iterative boolean-like law);I 6:N ðX Þ¼I ðX ;N ðX ÞÞ,where N is a strong interval fuzzy negation;I 7:N ðX Þ¼I ðX ;½0;0 Þis a strong interval fuzzy negation;I 8:I ðX ;½1;1 Þ¼½1;1 (dominance of truth of consequent);I 9:I ðX ;Y ÞP Y ;I 10:I ðX ;Y Þ¼I ðN ðY Þ;N ðX ÞÞ,where N is a strong interval fuzzy negation (contra-positive);I 11:I ð½0;0 ;X Þ¼½1;1 (dominance falsity).It is always possible to canonically obtain an interval fuzzy implication from any fuzzy implication.The interval fuzzy implication satisfies the optimality property and preserves the properties satisfied by the corresponding fuzzy implication.Proposition 16.If I is a fuzzy implication then b I is an interval fuzzy implication.Proof.It is straightforward.hIn the next results,we adopt a canonical way to construct,under some conditions,interval fuzzy implication from fuzzy implication and vice-versa.The properties of fuzzy implications presented in Section 5are related with the respective prop-erties of interval fuzzy implications enrolled above.Theorem 17.Let I 1and I 2be fuzzy implications satisfying the Properties I1and I2and such that I 16I 2.If I 1and I 2satisfy a Property Ik ,for k ¼1;...;6;8;...;11,then I :U 2!U ,defined byI ðX ;Y Þ¼½I 1ðX ;Y Þ;I 2ðX ;Y Þ ;ð20Þsatisfies the Property I k .Proof.Since I 16I 2and by the Properties I1and I2,one has that I 1ð;Y Þ6I 1ðX ;Y Þ6I 2ðX ;Y Þ6I 2ðX ;,and,therefore,I is well defined.It follows that:I 1:Let X ;Y ;Z 2U such that X 6Z .Since X 6Z ;X 6Z ,and I 1and I 2satisfy Property I1,then it holds that I 1ðZ ;Y Þ6I 1ðX ;Y Þand I 2ðZ ;6I 2ðX ;Þ.So,by Eq.(20),it follows that I ðZ ;Y Þ6I ðX ;Y Þ.I 2:Let X ;Y ;Z 2U such that Y 6Z .Since Y 6Z ;Y 6Z ,and I 1and I 2satisfy Property I2,then it holds that I 1ðX ;Y Þ6I 1ðX ;Z Þand I 2ðX ;Y Þ6I 2ðX ;Z Þ.Then,by Eq.(20),it follows that I ðX ;Y Þ6I ðX ;Z Þ.I 3:It holds that I ð½1;1 ;X Þ¼½I 1ð1;X Þ;I 2ð1;¼½X ; ¼X .I 4:By Property I4,it follows that:I ðX ;I ðY ;Z ÞÞ¼I ðX ;½I 1ð;Z Þ;I 2ðY ;Þ Þ¼½I 1ðI 1ðZ ÞÞ;I 2ðX ;I 2ðY ;¼½I 1ð;I 1ð;Z ÞÞ;I 2ðY ;I 2ðX ;ÞÞ ¼I ðY ;I ðX ;Z ÞÞ:I 5:By Property I5,it follows that:I ðX ;Y Þ¼½I 1ðX ;Y Þ;I 2ðX ;Y Þ ¼½I 1ðX ;I 1ðX ;Y ÞÞ;I 2ðX ;I 2ðX ;Y ÞÞ ¼I ðX ;½I 1ðX ;Y Þ;I 2ðX ;Y Þ Þ¼I ðX ;I ðX ;Y ÞÞ:I 6:Let N be a strong interval fuzzy negation.By Proposition 13,there exists a strong fuzzy negation N such thatN ðX Þ¼½N ðX Þ;N ðX Þ .It follows that:I ðX ;N ðX ÞÞ¼I ðX ;½N ð;N ðX Þ Þ¼½I 1ðN ðÞÞ;I 2ðX ;N ðX ÞÞ ¼½N ð;N ðX Þ ðby Property I6Þ¼N ðX Þ:I 8:One has that I ðX ;½1;1 Þ¼½I 1ðX ;1Þ;I 2ðX ;1Þ ¼½1;1 .I 9:By Property I9,it holds that I 1ðX ;Y ÞP Y and I 2ðX ;Y ÞP Y .Then,it follows that I ðX ;Y Þ¼½I 1ðX ;Y Þ;I 2ðX ;Y Þ P Y .I 10:Let N be a strong interval fuzzy negation.By Proposition 13,there exists a strong fuzzy negation N such thatN ðX Þ¼½N ðX Þ;N ðX Þ .So,by Property I10,it follows that:I ðX ;Y Þ¼½I 1ðY Þ;I 2ðX ;Þ ¼½I 1ðN ðY Þ;N ðÞÞ;I 2ðN ð;N ðX ÞÞ ¼I ð½N ð;N ðY Þ ;½N ðÞ;N ðX Þ Þ¼I ðN ðY Þ;N ðX ÞÞ:I 11:One has that I ð½0;0 ;X Þ¼½I 1ð0;X Þ;I 2ð0;Þ ¼½1;1 .hRemark 18.According to the conditions stated by Theorem 17,the Property I 7does not hold even if both I 1and I 2satisfy the Property I7.For example,considering I 1ðx ;y Þ¼min f 1Àx þy ;1g and I 2ðx ;y Þ¼min f ffiffiffiffiffiffiffiffiffiffiffiffiffiffiffiffiffiffiffiffiffiffi1Àx 2þy p ;1g ,it is immediate that I 1and I 2are fuzzy implications,as they satisfy Eq.(16),and it holds that I 16I 2.Moreover,considering N I 1ðx Þ¼I 1ðx ;0Þ¼1Àx and N I 2ðx Þ¼I 2ðx ;0Þ¼ffiffiffiffiffiffiffiffiffiffiffiffiffiffi1Àx 2p ,it is immediate that N I 1is a strong fuzzy negation,and,sinceB.C.Bedregal et al./Information Sciences 180(2010)1373–13891379。
“大象无形”——意大利卡斯格兰德建筑师事务所
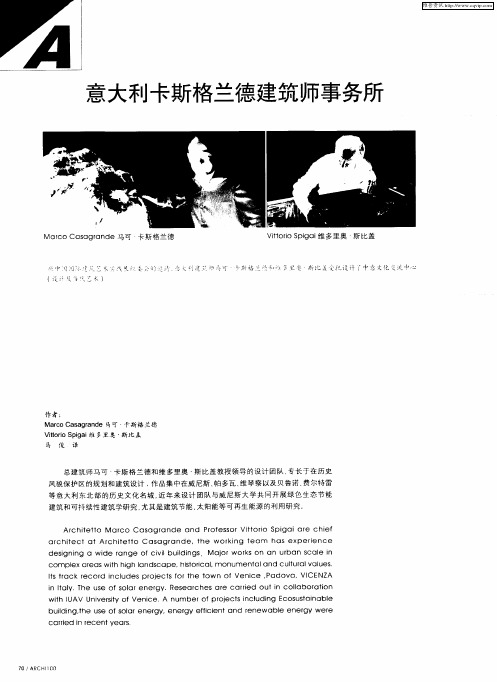
意大利卡斯格兰德建筑师事务所
Mar Cas de马 可 co agr an
卡斯格 兰 德
Vi o i pgai 多里奥 t Si 维 tr a
斯 比盖
幔 中 : I 己= 替 鼻 曼 冬 , . 、 : : j j童: 喜 f 5 芝置 马可
等 意大 利东 北 部 的历 史 文化 名城 。 年 来设 计 团 队与 威尼 斯 大 学共 同 开展 绿色 生 态 节能 近 建筑 和可 持续 性建 筑学研 究 . 尤其 是建 筑节 能 、 阳能 等可 再生 能源 的利用 研 究。 太
A r chiet o t t M a r co as C ag nde nd Pr es o Vit i r a a of s r t or a Spi r gaio e Chi ef
i Ial n t y.T he e ol rener us ofs a gy.Res ea r ches a ca i ou n col r e r ed r t i l abo i r on at
wi U nv i ni t IAV U ie s yofVe ce. m b ofpr e sicu n c s saiabl h rt A nu er ct n ldig E o u t n oj e
,
墼 固 “ .
— —
:
口
哪 詈 I : 蝴 『 {甭 _ l l 籀 啊
册 删
维普资讯
N . N A NF E C T.RLH P L O 2 RO T I BToo l E V I OT A E SO o S A O HNN T
曰
■
,- ຫໍສະໝຸດ :{ ■ j — ■ 。 ●■ ●
L-fuzzy拓扑空间的F紧性理论研究

5E M( L ) .则 ( 1 ) 称 为 的 一远域 族 , 记作 ^ <A ( 5 ) , 如 中 的每 个 高 度 为 的分 子 ( ≤A ),有
别是 F紧陛的性质如何 , 是后来学者们关注的研究课
钔
.
果 对
PE , 使P c r / ( x D( 这里 r l ( x o ) 表示分子 的全体闭
它是 三 一 好 的推 广 .
关
键
词 :L —f u z z y拓扑空间 ;F紧 性; 一远域族 ;复盖 ;L - 好的推广
中图分 类号 :O 1 8 9 . 1 1
文献标 志码 :A 文章 编号 : 1 0 0 6 。 6 8 5 3 ( 2 0 1 3 ) 0 1 . 0 0 6 2 . 0 5
作者简介 :万诗敏 ( 1 9 7 7 一) ,男 ,安徽潜山人 ,天津城 市建设学 院副教授 ,硕士
天 津城 市建 设 学院学 报 万诗敏 : L — f u z z y 拓扑空间的F紧陛理论研究
天津城市建设学院学报 第 1 9 卷 第1 期 2 0 1 3 年 3月
J o u r n a l o f T i a n j i n I n s t i t u t e o f U r b a n C o n s t r u c t i o n V o 1 . 1 9 No . 1 Ma r . 2 0 1 3
说 明 ,本 文 中其 它所 使 用 的 概 念 和符 号 均 取 自文
献[ 3 ] . 定义 1 设 ( , 是L F拓 扑空 间 , A∈ , c
,
强紧f 生 和超 紧 … ; 并指出 F紧』 生 不具有闭遗传性
质 .因此 ,F紧性 的定义 就有改 进 的必要 . 1 9 8 3 年,
fuzzy control 外文翻译
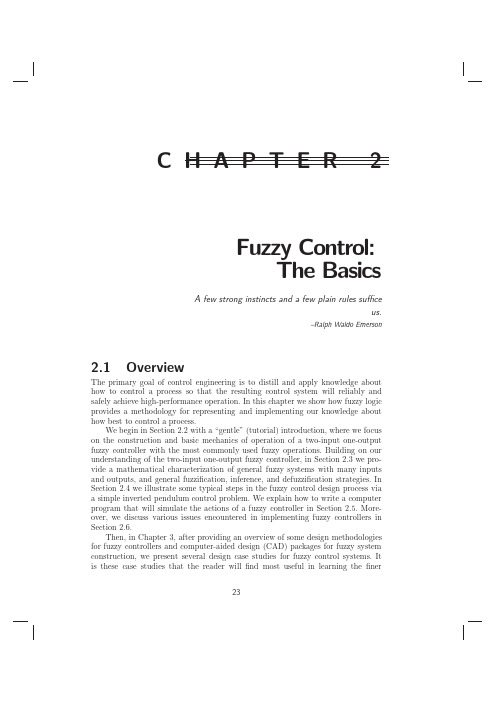
C H A P T E R2Fuzzy Control:The BasicsA few strong instincts and a few plain rules sufficeus.–Ralph Waldo Emerson2.1OverviewThe primary goal of control engineering is to distill and apply knowledge about how to control a process so that the resulting control system will reliably and safely achieve high-performance operation.In this chapter we show how fuzzy logic provides a methodology for representing and implementing our knowledge about how best to control a process.We begin in Section2.2with a“gentle”(tutorial)introduction,where we focus on the construction and basic mechanics of operation of a two-input one-output fuzzy controller with the most commonly used fuzzy operations.Building on our understanding of the two-input one-output fuzzy controller,in Section2.3we pro-vide a mathematical characterization of general fuzzy systems with many inputs and outputs,and general fuzzification,inference,and defuzzification strategies.In Section2.4we illustrate some typical steps in the fuzzy control design process via a simple inverted pendulum control problem.We explain how to write a computer program that will simulate the actions of a fuzzy controller in Section2.5.More-over,we discuss various issues encountered in implementing fuzzy controllers in Section2.6.Then,in Chapter3,after providing an overview of some design methodologies for fuzzy controllers and computer-aided design(CAD)packages for fuzzy system construction,we present several design case studies for fuzzy control systems.It is these case studies that the reader willfind most useful in learning thefiner2324Chapter2/Fuzzy Control:The Basicspoints about the fuzzy controller’s operation and design.Indeed,the best way toreally learn fuzzy control is to design your own fuzzy controller for one of theplants studied in this or the next chapter,and simulate the fuzzy control system toevaluate its performance.Initially,we recommend coding this fuzzy controller in ahigh-level language such as C,Matlab,or ter,after you have acquiredafirm understanding of the fuzzy controller’s operation,you can take shortcuts byusing a(or designing your own)CAD package for fuzzy control systems.After completing this chapter,the reader should be able to design and simulatea fuzzy control system.This will move the reader a long way toward implementationof fuzzy controllers since we provide pointers on how to overcome certain practicalproblems encountered in fuzzy control system design and implementation(e.g.,coding the fuzzy controller to operate in real-time,even with large rule-bases).This chapter provides a foundation on which the remainder of the book rests.After our case studies in direct fuzzy controller design in Chapter3,we will usethe basic definition of the fuzzy control system and study its fundamental dynamicproperties,including stability,in Chapter 4.We will use the same plants,andothers,to illustrate the techniques for fuzzy identification,fuzzy adaptive control,and fuzzy supervisory control in Chapters5,6,and7,respectively.It is thereforeimportant for the reader to have afirm grasp of the concepts in this and the nextchapter before moving on to these more advanced chapters.Before skipping any sections or chapters of this book,we recommend that the reader study the chapter summaries at the end of each chapter.In these summarieswe will highlight all the major concepts,approaches,and techniques that are coveredin the chapter.These summaries also serve to remind the reader what should belearned in each chapter.2.2Fuzzy Control:A Tutorial IntroductionA block diagram of a fuzzy control system is shown in Figure2.1.The fuzzy con-troller1is composed of the following four elements:1.A rule-base(a set of If-Then rules),which contains a fuzzy logic quantificationof the expert’s linguistic description of how to achieve good control.2.An inference mechanism(also called an“inference engine”or“fuzzy inference”module),which emulates the expert’s decision making in interpreting and ap-plying knowledge about how best to control the plant.3.A fuzzification interface,which converts controller inputs into information thatthe inference mechanism can easily use to activate and apply rules.4.A defuzzification interface,which converts the conclusions of the inferencemechanism into actual inputs for the process.1.Sometimes a fuzzy controller is called a“fuzzy logic controller”(FLC)or even a“fuzzylinguistic controller”since,as we will see,it uses fuzzy logic in the quantification of linguisticdescriptions.In this book we will avoid these phrases and simply use“fuzzy controller.”2.2Fuzzy Control:A Tutorial Introduction 25FIGURE 2.1Fuzzy controller.We introduce each of the components of the fuzzy controller for a simple prob-lem of balancing an inverted pendulum on a cart,as shown in Figure 2.2.Here,y denotes the angle that the pendulum makes with the vertical (in radians),l is the half-pendulum length (in meters),and u is the force input that moves the cart (in Newtons).We will use r to denote the desired angular position of the pendulum.The goal is to balance the pendulum in the upright position (i.e.,r =0)when it initially starts with some nonzero angle offthe vertical (i.e.,y =0).This is a very simple and academic nonlinear control problem,and many good techniques already existfor its solution.Indeed,for this standard configuration,a simple PID controller works well even in implementation.In the remainder of this section,we will use the inverted pendulum as a con-venient problem to illustrate the design and basic mechanics of the operation of a fuzzy control system.We will also use this problem in Section 2.4to discuss much more general issues in fuzzy control system design that the reader will find useful for more challenging applications (e.g.,the ones in the next chapter).FIGURE 2.2Inverted pendulumon a cart.26Chapter2/Fuzzy Control:The Basics2.2.1Choosing Fuzzy Controller Inputs and OutputsConsider a human-in-the-loop whose responsibility is to control the pendulum,asshown in Figure2.3.The fuzzy controller is to be designed to automate how ahuman expert who is successful at this task would control the system.First,theexpert tells us(the designers of the fuzzy controller)what information she or hewill use as inputs to the decision-making process.Suppose that for the invertedpendulum,the expert(this could be you!)says that she or he will usee(t)=r(t)−y(t)andde(t)dtas the variables on which to base decisions.Certainly,there are many other choices(e.g.,the integral of the error e could also be used)but this choice makes goodintuitive sense.Next,we must identify the controlled variable.For the invertedpendulum,we are allowed to control only the force that moves the cart,so thechoice here is simple.FIGURE2.3Human controlling aninverted pendulum on a cart.For more complex applications,the choice of the inputs to the controller and outputs of the controller(inputs to the plant)can be more difficult.Essentially,youwant to make sure that the controller will have the proper information availableto be able to make good decisions and have proper control inputs to be able tosteer the system in the directions needed to be able to achieve high-performanceoperation.Practically speaking,access to information and the ability to effectivelycontrol the system often cost money.If the designer believes that proper informationis not available for making control decisions,he or she may have to invest in anothersensor that can provide a measurement of another system variable.Alternatively,the designer may implement somefiltering or other processing of the plant outputs.In addition,if the designer determines that the current actuators will not allowfor the precise control of the process,he or she may need to invest in designingand implementing an actuator that can properly affect the process.Hence,while insome academic problems you may be given the plant inputs and outputs,in manypractical situations you may have someflexibility in their choice.These choices2.2Fuzzy Control:A Tutorial Introduction27 affect what information is available for making on-line decisions about the controlof a process and hence affect how we design a fuzzy controller.Once the fuzzy controller inputs and outputs are chosen,you must determinewhat the reference inputs are.For the inverted pendulum,the choice of the referenceinput r=0is clear.In some situations,however,you may want to choose r assome nonzero constant to balance the pendulum in the off-vertical position.To dothis,the controller must maintain the cart at a constant acceleration so that the pendulum will not fall.After all the inputs and outputs are defined for the fuzzy controller,we canspecify the fuzzy control system.The fuzzy control system for the inverted pendu-lum,with our choice of inputs and outputs,is shown in Figure2.4.Now,within this framework we seek to obtain a description of how to control the process.We see thenthat the choice of the inputs and outputs of the controller places certain constraintson the remainder of the fuzzy control design process.If the proper information isnot provided to the fuzzy controller,there will be little hope for being able to designa good rule-base or inference mechanism.Moreover,even if the proper informationis available to make control decisions,this will be of little use if the controller isnot able to properly affect the process variables via the process inputs.It must be understood that the choice of the controller inputs and outputs is a fundamentally important part of the control design process.We will revisit this issue several times throughout the remainder of this chapter(and book).FIGURE2.4Fuzzy controller for an inverted pendulum on a cart.2.2.2Putting Control Knowledge into Rule-BasesSuppose that the human expert shown in Figure2.3provides a description of howbest to control the plant in some natural language(e.g.,English).We seek to takethis“linguistic”description and load it into the fuzzy controller,as indicated bythe arrow in Figure2.4.28Chapter2/Fuzzy Control:The BasicsLinguistic DescriptionsThe linguistic description provided by the expert can generally be broken intoseveral parts.There will be“linguistic variables”that describe each of the time-varying fuzzy controller inputs and outputs.For the inverted pendulum,“error”describes e(t)“change-in-error”describes ddt e(t)“force”describes u(t)Note that we use quotes to emphasize that certain words or phrases are linguistic descriptions,and that we have added the time index to,for example,e(t),to em-phasize that generally e varies with time.There are many possible choices for the linguistic descriptions for variables.Some designers like to choose them so that they are quite descriptive for documentation purposes.However,this can sometimes lead to long descriptions.Others seek to keep the linguistic descriptions as short as pos-sible(e.g.,using“e(t)”as the linguistic variable for e(t)),yet accurate enough so that they adequately represent the variables.Regardless,the choice of the linguistic variable has no impact on the way that the fuzzy controller operates;it is simply a notation that helps to facilitate the construction of the fuzzy controller via fuzzy logic.Just as e(t)takes on a value of,for example,0.1at t=2(e(2)=0.1),linguistic variables assume“linguistic values.”That is,the values that linguistic variables take on over time change dynamically.Suppose for the pendulum example that “error,”“change-in-error,”and“force”take on the following values:“neglarge”“negsmall”“zero”“possmall”“poslarge”Note that we are using“negsmall”as an abbreviation for“negative small in size”and so on for the other variables.Such abbreviations help keep the linguistic de-scriptions short yet precise.For an even shorter description we could use integers:“−2”to represent“neglarge”“−1”to represent“negsmall”“0”to represent“zero”“1”to represent“possmall”“2”to represent“poslarge”This is a particularly appealing choice for the linguistic values since the descriptions are short and nicely represent that the variable we are concerned with has a numeric quality.We are not,for example,associating“−1”with any particular number of radians of error;the use of the numbers for linguistic descriptions simply quantifies the sign of the error(in the usual way)and indicates the size in relation to the2.2Fuzzy Control:A Tutorial Introduction29 other linguistic values.We shallfind the use of this type of linguistic value quite convenient and hence will give it the special name,“linguistic-numeric value.”The linguistic variables and values provide a language for the expert to expressher or his ideas about the control decision-making process in the context of the framework established by our choice of fuzzy controller inputs and outputs.Recallthat for the inverted pendulum r=0and e=r−y so thate=−yandd dt e=−ddtysince ddt r=0.First,we will study how we can quantify certain dynamic behaviorswith linguistics.In the next subsection we will study how to quantify knowledge about how to control the pendulum using linguistic descriptions.For the inverted pendulum each of the following statements quantifies a different configuration of the pendulum(refer back to Figure2.2on page25):•The statement“error is poslarge”can represent the situation where the pendulum is at a significant angle to the left of the vertical.•The statement“error is negsmall”can represent the situation where the pendulum is just slightly to the right of the vertical,but not too close to the vertical to justify quantifying it as“zero”and not too far away to justify quantifying it as “neglarge.”•The statement“error is zero”can represent the situation where the pendulum is very near the vertical position(a linguistic quantification is not precise,hence we are willing to accept any value of the error around e(t)=0as being quantified linguistically by“zero”since this can be considered a better quantification than “possmall”or“negsmall”).•The statement“error is poslarge and change-in-error is possmall”can representthe situation where the pendulum is to the left of the vertical and,since ddt y<0,the pendulum is moving away from the upright position(note that in this case the pendulum is moving counterclockwise).•The statement“error is negsmall and change-in-error is possmall”can represent the situation where the pendulum is slightly to the right of the vertical and,sinced dt y<0,the pendulum is moving toward the upright position(note that in thiscase the pendulum is also moving counterclockwise).It is important for the reader to study each of the cases above to understand how the expert’s linguistics quantify the dynamics of the pendulum(actually,each partially quantifies the pendulum’s state).30Chapter2/Fuzzy Control:The BasicsOverall,we see that to quantify the dynamics of the process we need to have a good understanding of the physics of the underlying process we are trying to control.While for the pendulum problem,the task of coming to a good understanding ofthe dynamics is relatively easy,this is not the case for many physical processes.Quantifying the process dynamics with linguistics is not always easy,and certainlya better understanding of the process dynamics generally leads to a better linguisticquantification.Often,this will naturally lead to a better fuzzy controller providedthat you can adequately measure the system dynamics so that the fuzzy controllercan make the right decisions at the proper time.RulesNext,we will use the above linguistic quantification to specify a set of rules(arule-base)that captures the expert’s knowledge about how to control the plant.Inparticular,for the inverted pendulum in the three positions shown in Figure2.5,we have the following rules(notice that we drop the quotes since the whole rule islinguistic):1.If error is neglarge and change-in-error is neglarge Then force is poslargeThis rule quantifies the situation in Figure2.5(a)where the pendulum has alarge positive angle and is moving clockwise;hence it is clear that we shouldapply a strong positive force(to the right)so that we can try to start thependulum moving in the proper direction.2.If error is zero and change-in-error is possmall Then force is negsmallThis rule quantifies the situation in Figure2.5(b)where the pendulum hasnearly a zero angle with the vertical(a linguistic quantification of zero does notimply that e(t)=0exactly)and is moving counterclockwise;hence we shouldapply a small negative force(to the left)to counteract the movement so that itmoves toward zero(a positive force could result in the pendulum overshootingthe desired position).3.If error is poslarge and change-in-error is negsmall Then force is negsmallThis rule quantifies the situation in Figure2.5(c)where the pendulum is far tothe left of the vertical and is moving clockwise;hence we should apply a smallnegative force(to the left)to assist the movement,but not a big one since thependulum is already moving in the proper direction.Each of the three rules listed above is a“linguistic rule”since it is formed solely from linguistic variables and values.Since linguistic values are not preciserepresentations of the underlying quantities that they describe,linguistic rules arenot precise either.They are simply abstract ideas about how to achieve good controlthat could mean somewhat different things to different people.They are,however,at2.2Fuzzy Control:A Tutorial Introduction31(a)(b)(c)FIGURE2.5Inverted pendulum in various positions.a level of abstraction that humans are often comfortable with in terms of specifyinghow to control a process.The general form of the linguistic rules listed above isIf premise Then consequentAs you can see from the three rules listed above,the premises(which are sometimescalled“antecedents”)are associated with the fuzzy controller inputs and are onthe left-hand-side of the rules.The consequents(sometimes called“actions”)are associated with the fuzzy controller outputs and are on the right-hand-side of therules.Notice that each premise(or consequent)can be composed of the conjunctionof several“terms”(e.g.,in rule3above“error is poslarge and change-in-error is negsmall”is a premise that is the conjunction of two terms).The number of fuzzy controller inputs and outputs places an upper limit on the number of elementsin the premises and consequents.Note that there does not need to be a premise (consequent)term for each input(output)in each rule,although often there is.Rule-BasesUsing the above approach,we could continue to write down rules for the pendulumproblem for all possible cases(the reader should do this for practice,at least fora few more rules).Note that since we only specify afinite number of linguisticvariables and linguistic values,there is only afinite number of possible rules.Forthe pendulum problem,with two inputs andfive linguistic values for each of these,there are at most52=25possible rules(all possible combinations of premiselinguistic values for two inputs).A convenient way to list all possible rules for the case where there are not toomany inputs to the fuzzy controller(less than or equal to two or three)is to use atabular representation.A tabular representation of one possible set of rules for theinverted pendulum is shown in Table2.1.Notice that the body of the table lists thelinguistic-numeric consequents of the rules,and the left column and top row of thetable contain the linguistic-numeric premise terms.Then,for instance,the(2,−1)position(where the“2”represents the row having“2”for a numeric-linguistic valueand the“−1”represents the column having“−1”for a numeric-linguistic value)has a−1(“negsmall”)in the body of the table and represents the rule32Chapter2/Fuzzy Control:The BasicsIf error is poslarge and change-in-error is negsmall Then force is negsmall which is rule3above.Table2.1represents abstract knowledge that the expert hasabout how to control the pendulum given the error and its derivative as inputs.TABLE2.1Rule Table for the Inverted Pendulum“force”“change-in-error”˙eu−2−1012−222210“error”−12210−1e0210−1−2110−1−2−220−1−2−2−2The reader should convince him-or herself that the other rules are also valid and take special note of the pattern of rule consequents that appears in the body of thetable:Notice the diagonal of zeros and viewing the body of the table as a matrixwe see that it has a certain symmetry to it.This symmetry that emerges whenthe rules are tabulated is no accident and is actually a representation of abstractknowledge about how to control the pendulum;it arises due to a symmetry in thesystem’s dynamics.We will actually see later that similar patterns will be foundwhen constructing rule-bases for more challenging applications,and we will showhow to exploit this symmetry in implementing fuzzy controllers.2.2.3Fuzzy Quantification of KnowledgeUp to this point we have only quantified,in an abstract way,the knowledge thatthe human expert has about how to control the plant.Next,we will show how touse fuzzy logic to fully quantify the meaning of linguistic descriptions so that wemay automate,in the fuzzy controller,the control rules specified by the expert.Membership FunctionsFirst,we quantify the meaning of the linguistic values using“membership func-tions.”Consider,for example,Figure2.6.This is a plot of a functionμversus e(t)that takes on special meaning.The functionμquantifies the certainty2that e(t)can be classified linguistically as“possmall.”To understand the way that a mem-bership function works,it is best to perform a case analysis where we show how tointerpret it for various values of e(t):2.The reader should not confuse the term“certainty”with“probability”or“likelihood.”Themembership function is not a probability density function,and there is no underlying probabilityspace.By“certainty”we mean“degree of truth.”The membership function does not quantifyrandom behavior;it simply makes more accurate(less fuzzy)the meaning of linguisticdescriptions.2.2Fuzzy Control:A Tutorial Introduction33•If e(t)=−π/2thenμ(−π/2)=0,indicating that we are certain that e(t)=−π/2is not“possmall.”•If e(t)=π/8thenμ(π/8)=0.5,indicating that we are halfway certain thate(t)=π/8is“possmall”(we are only halfway certain since it could also be“zero”with some degree of certainty—this value is in a“gray area”in terms oflinguistic interpretation).•If e(t)=π/4thenμ(π/4)=1.0,indicating that we are absolutely certain thate(t)=π/4is what we mean by“possmall.”•If e(t)=πthenμ(π)=0,indicating that we are certain that e(t)=πis not “possmall”(actually,it is“poslarge”).FIGURE2.6Membership function forlinguistic value“possmall.”The membership function quantifies,in a continuous manner,whether values ofe(t)belong to(are members of)the set of values that are“possmall,”and hence itquantifies the meaning of the linguistic statement“error is possmall.”This is why itis called a membership function.It is important to recognize that the membershipfunction in Figure2.6is only one possible definition of the meaning of“error is possmall”;you could use a bell-shaped function,a trapezoid,or many others.For instance,consider the membership functions shown in Figure2.7.For some application someone may be able to argue that we are absolutely certain that anyvalue of e(t)nearπ4is still“possmall”and only when you get sufficiently far fromπ4do we lose our confidence that it is“possmall.”One way to characterize this un-derstanding of the meaning of“possmall”is via the trapezoid-shaped membership function in Figure2.7(a).For other applications you may think of membership in the set of“possmall”values as being dictated by the Gaussian-shaped member-ship function(not to be confused with the Gaussian probability density function) shown in Figure2.7(b).For still other applications you may not readily acceptvalues far away fromπ4as being“possmall,”so you may use the membership func-tion in Figure2.7(c)to represent this.Finally,while we often think of symmetric characterizations of the meaning of linguistic values,we are not restricted to these34Chapter 2/Fuzzy Control:The Basicssymmetric representations.For instance,in Figure 2.7(d)we represent that we be-lieve that as e (t )moves to the left of π4we are very quick to reduce our confidencethat it is “possmall,”but if we move to the right of π4our confidence that e (t )is“possmall,”diminishes at a slower rate.(a) Trapezoid.(b) Gaussian.(c) Sharp peak.(d) Skewed triangle.FIGURE 2.7A few membership function choices for representing “error ispossmall.”In summary,we see that depending on the application and the designer (ex-pert),many different choices of membership functions are possible.We will further discuss other ways to define membership functions in Section 2.3.2on page 55.It is important to note here,however,that for the most part the definition of a member-ship function is subjective rather than objective.That is,we simply quantify it in a manner that makes sense to us,but others may quantify it in a different manner.The set of values that is described by μas being “positive small”is called a “fuzzy set.”Let A denote this fuzzy set.Notice that from Figure 2.6we are absolutely certain that e (t )=π4is an element of A ,but we are less certain thate (t )=π16is an element of A .Membership in the set,as specified by the membership function,is fuzzy;hence we use the term “fuzzy set.”We will give a more precise description of a fuzzy set in Section 2.3.2on page 55.A “crisp”(as contrasted to “fuzzy”)quantification of “possmall”can also be specified,but via the membership function shown in Figure 2.8.This membership function is simply an alternative representation for the interval on the real line π/8≤e (t )≤3π/8,and it indicates that this interval of numbers represents “poss-mall.”Clearly,this characterization of crisp sets is simply another way to represent a normal interval (set)of real numbers.While the vertical axis in Figure 2.6represents certainty,the horizontal axis is also given a special name.It is called the “universe of discourse”for the input e (t )since it provides the range of values of e (t )that can be quantified with linguistics2.2Fuzzy Control:A Tutorial Introduction35FIGURE2.8Membership function for acrisp set.and fuzzy sets.In conventional terminology,a universe of discourse for an input oroutput of a fuzzy system is simply the range of values the inputs and outputs cantake on.Now that we know how to specify the meaning of a linguistic value via a mem-bership function(and hence a fuzzy set),we can easily specify the membershipfunctions for all15linguistic values(five for each input andfive for the output)of our inverted pendulum example.See Figure2.9for one choice of membership functions.Notice that(for our later convenience)we list both the linguistic values andthe linguistic-numeric values associated with each membership function.Hence,we see that the membership function in Figure2.6for“possmall”is embeddedamong several others that describe other sizes of values(so that,for instance,the membership function to the right of the one for“possmall”is the one that represents“error is poslarge”).Note that other similarly shaped membership functions makesense(e.g.,bell-shaped membership functions).We will discuss the multitude ofchoices that are possible for membership functions in Section2.3.2on page55.The membership functions at the outer edges in Figure2.9deserve specialattention.For the inputs e(t)and ddt e(t)we see that the outermost membershipfunctions“saturate”at a value of one.This makes intuitive sense as at some point the human expert would just group all large values together in a linguistic de-scription such as“poslarge.”The membership functions at the outermost edges appropriately characterize this phenomenon since they characterize“greater than”(for the right side)and“less than”(for the left side).Study Figure2.9and convince yourself of this.For the output u,the membership functions at the outermost edges cannot be saturated for the fuzzy system to be properly defined(more details on this point will be provided in Section2.2.6on page44and Section2.3.5on page65).The basic reason for this is that in decision-making processes of the type we study,we seek to take actions that specify an exact value for the process input.We do not generally indicate to a process actuator,“any value bigger than,say,10,is acceptable.”It is important to have a clear picture in your mind of how the values of the membership functions change as,for example,e(t)changes its value over time. For instance,as e(t)changes from−π/2toπ/2we see that various membership。
- 1、下载文档前请自行甄别文档内容的完整性,平台不提供额外的编辑、内容补充、找答案等附加服务。
- 2、"仅部分预览"的文档,不可在线预览部分如存在完整性等问题,可反馈申请退款(可完整预览的文档不适用该条件!)。
- 3、如文档侵犯您的权益,请联系客服反馈,我们会尽快为您处理(人工客服工作时间:9:00-18:30)。
Fuzzy Sets and Systems160(2009)1218–1232/locate/fss L-fuzzy interiors and L-fuzzy closuresFu-Gui Shi∗Department of Mathematics,School of Science,Beijing Institute of Technology,Beijing100081,PR ChinaReceived26October2007;received in revised form8September2008;accepted12September2008Available online26September2008AbstractIn this paper,we introduce new definitions of L-fuzzy neighborhood systems,L-fuzzy interior operators and L-fuzzy closure operators.Three characterizations of the category L-FTOP of L-fuzzy topological spaces and their L-fuzzy continuous mappings are presented by means of the category L-FNS of L-fuzzy neighborhood spaces and their continuous mappings,the category L-FIS of L-fuzzy interior spaces and their L-fuzzy continuous mappings,and the category L-FCS of L-fuzzy closure spaces and their L-fuzzy continuous mappings.©2008Elsevier B.V.All rights reserved.Keywords:L-fuzzy topology;L-fuzzy neighborhood system;L-fuzzy interior;L-fuzzy closure1.IntroductionSince Chang[1]introduced[0,1]-topology in fuzzy set theory,many researchers have tried to generalize the theory of general topology to the fuzzy setting.A Chang topology is a crisp subset of I X.However,in a completely different direction,Höhle[5]presented the notion of a fuzzy topology being viewed as an I-subset of a powerset2X.Then Kubiak [9]and˘Sostak[14]independently extended Höhle’s fuzzy topology to L-subsets of L X,which is called L-fuzzy topology (see[6,7,12]).It is well known that neighborhood systems,interior operators and closure operators play an important role in topology and they are very good ways to characterize topology.Many authors[2,4,7,8]have studied(quasi-)neighborhood systems,interior operators and closure operators in L-fuzzy topological spaces.The notions of quasi-neighborhood systems and remote-neighborhood systems play an important role in L-topology [10].In[4],Fang generalized the quasi-neighborhood systems from L-topological spaces to L-fuzzy topological spaces and proved that the category of L-fuzzy quasi-neighborhood spaces and their continuous mappings is isomorphic to the category of L-fuzzy topological spaces and their continuous mappings.However,the notion of neighborhoods is also important in L-topology.In some case,we can use neighborhoods and quasi-neighborhoods at the same time.For example,in[13]we defined a T2axiom by means of neighborhoods and remote-neighborhoods.Therefore it is necessary to generalize neighborhood systems to L-fuzzy topology.∗Tel.:+861088583137.E-mail addresses:fuguishi@,f.g.shi@(F.-G.Shi).0165-0114/$-see front matter©2008Elsevier B.V.All rights reserved.doi:10.1016/j.fss.2008.09.002F .-G.Shi /Fuzzy Sets and Systems 160(2009)1218–12321219In [7],a notion of L -fuzzy neighborhood systems was introduced as follows:Definition 1.1(Höhle and ˘Sostak [7]).Let X be a set.A map N :X ×L X ×L →L is called an L -fuzzy neighborhoodsystem iff N satisfies the following axioms:(N1)N (x , ,a )= ;(N2)u v,b a ⇒N (x ,u ,a ) N (x ,v,b );(N3)N (x ,u 1,a )∧N (x ,u 2,b ) N (x ,u 1∧u 2,a ∧b )(where ⊗is replaced by ∧);(N4)N (x ,u ,a ) u (x );(N5)N (x ,u ,a ){N (x ,v,b ):v (y ) N (y ,u ,a )∀y ∈X };(N6)N (x ,u ,⊥)=u (x );(N7)For any nonempty subset {a i :i ∈ }of L the implication holds:u (x ) N (x ,u ,a i )∀i ∈ ,∀x ∈X ⇒u (x ) Nx ,u ,i ∈a i ∀x ∈X .In [2,7,8],two notions of interior operators and closure operators were defined,respectively,as follows:Definition 1.2(Höhle and ˘Sostak [7]).A map I :L X ×L →L X is called an L -fuzzy interior operator on X iff itsatisfies the following conditions:(I1)I ( ,a )= ∀a ∈L ;(I2)I (v,b ) I (u ,a )whenever v u ,a b ;(I3)I (u ,a )∧I (v,b ) I (u ∧v,a ∧b );(I4)I (u ,a ) u ;(I5)I (u ,a ) I (I (u ,a ),a );(I6)I (u ,⊥)=u ;(I7)If ∅ K ⊆L ,I (u ,a )=u 0∀a ∈K ,then I (u ,∨K )=u 0.Definition 1.3(Chattopadhyay and Samanta [2],Kim [8]).A map C :L X ×L 0→L X is called an L -fuzzy closure operator on X iff it satisfies the following conditions:for all , ∈L X ,r ,s ∈L 0,(C1)C (⊥,a )=⊥;(C2)C ( ,r ) ;(C3) ⇒C ( ,r ) C ( ,r );(C4)C ( ∨ ,r )=C ( ,r )∨C ( ,r );(C5)r s ⇒C ( ,r ) C ( ,s );(C6)C ( ,r )=C (C ( ,r ),r );(C7)If r = {s ∈L :C ( ,s )= },then C ( ,r )= .Definitions 1.2and 1.3actually rely on the level L -topology T [r ]of the L -fuzzy topology T ,i.e.,I ( ,r )and C ( ,r )can be viewed as the interior and the closure of in L -topology T [r ][15].Moreover the conditions of Definitions 1.1–1.3are also more complicated.Therefore their applications are restricted to some degree.The aim of this paper is to introduce new definitions of L -fuzzy neighborhood systems,L -fuzzy interior operators and L -fuzzy closure operators in L -fuzzy topological spaces in different ways from [2,7,8].Our definitions do not rely on the level L -topology T [r ]of the L -fuzzy topology T .Three characterizations of the category L -FTOP of L -fuzzy topological spaces and their L -fuzzy continuous mappings are presented by means of the category L -FNS of L -fuzzy neighborhood spaces and their continuous mappings,the category L -FIS of L -fuzzy interior spaces and their L -fuzzy continuous mappings,and the category L -FCS of L -fuzzy closure spaces and their L -fuzzy continuous mappings.2.PreliminariesThroughout this paper,L denotes a completely distributive DeMorgan algebra.The smallest element and the largest element in L are denoted by ⊥and ,respectively.X is a nonempty set.The set of all nonzero co-prime elements of L1220F .-G.Shi /Fuzzy Sets and Systems 160(2009)1218–1232is denoted by J (L ).The set of all nonzero co-prime elements of L X is denoted by J (L X ).It is easy to see that J (L X )is exactly the set of all fuzzy points x ( ∈J (L )).We say that a is wedge below b in L ,denoted by a ≺b ,if for every subset D ⊆L , D b implies d a for some d ∈D [3].A complete lattice L is completely distributive if and only if b ={a ∈L :a ≺b }for each b ∈L .For any b ∈L ,define (b )={a ∈L :a ≺b }.It is easy to see that for all a ∈L , (a )= b ≺a (b ).Hence the wedge below relation in a completely distributive lattice has the interpolation property,this means a ≺b ⇒∃c ∈L such that a ≺c ≺b .Moreover we can see that a ≺ i ∈ b i ⇒∃b i such that a ≺b i .Some properties of the map can be found in [10].For any L -fuzzy set A ∈L X and any a ∈L ,we use the following notations:A [a ]={x ∈X :A (x ) a },A (a )={x ∈X :A (x ) a },a (x )=a ,∀x ∈X .Definition 2.1(Höhle and ˘Sostak [7],Kubiak [9],Rodabaugh [12],˘Sostak [14]).An L -fuzzy topology on a set X isa map T :L X →L such that(LFT1)T ( )=T (⊥)= ;(LFT2)∀U ,V ∈L X ,T (U ∧V ) T (U )∧T (V );(LFT3)∀U j ∈L X ,j ∈J ,T ( j ∈J U j ) j ∈J T (U j ).T (U )can be interpreted as the degree to which U is an open L -set.T ∗(U )=T (U )will be called the degree ofclosedness of U ,where U is the L -complement of U .The pair (X ,T )is called an L -fuzzy topological space.A mapping f :(X ,T 1)→(Y ,T 2)is said to be continuous with respect to L -fuzzy topologies T 1and T 2if T 1(f ←L (U )) T 2(U )holds for all U ∈L Y ,where f ←L is defined by f ←L(U )(x )=U (f (x ))[11].The category of L -fuzzy topological spaces and their continuous mappings is denoted by L -FTOP .In an L -topological space (X , ),an open L -set U is said to be a quasi-neighborhood of a fuzzy point x ,if x U or U (x )=(U (x )) .In [4],Fang generalized the concept of quasi-neighborhood systems to L -fuzzy topological spaces as follows:Definition 2.2(Fang [4]).An L -fuzzy quasi-neighborhood system on X is a set Q ={Q x :x ∈J (L X )}of maps {Q x :L X →L }satisfying the following conditions:(LFQ1)Q x ( )= and Q x (⊥)=⊥;(LFQ2)Q x (A ) ⊥⇒x A ;(LFQ3)Q x (A ∧B )=Q x (A )∧Q x (B );(LFQ4)Q x (A )=x D A y D Q y (D ).A set X equipped with an L -fuzzy quasi-neighborhood system Q ={Q x :x ∈J (L X )},denoted by (X ,Q ),is called an L -fuzzy quasi-neighborhood space.A mapping f :X →Y between two L -fuzzy quasi-neighborhood spaces (X ,Q 1)and (Y ,Q 2)is called continuous if ∀x ∈J (L X ),∀U ∈L Y ,(Q 2)f →L (x)(U ) (Q 1)x (f ←L (U )),where f →L (x )=f (x ).The category of L -fuzzy quasi-neighborhood spaces and their continuous mappings is denoted by L -FQN .3.L -fuzzy neighborhood systemsIn this section,we shall present a new definition of L -fuzzy neighborhood systems,and study the relation between L -fuzzy neighborhood systems and L -fuzzy topologies.In an L -topological space (X , ),an open L -set U is said to be a neighborhood of a fuzzy point x ,if x U or U (x ).It is easy to see that the neighborhood systems of a fuzzy point x is different from theF.-G.Shi/Fuzzy Sets and Systems160(2009)1218–12321221 quasi-neighborhood systems of x .Now we generalize the concept of neighborhood systems to L-fuzzy topological spaces as follows:Definition3.1.An L-fuzzy neighborhood system on X is defined to be a set N={N x:x ∈J(L X)}of maps{N x:L X→L}satisfying the following conditions:(LFN1)N x( )= and N x(⊥)=⊥;(LFN2)N x(U)=⊥for any x U;(LFN3)N x(U∧V)=N x(U)∧N x(V);(LFN4)N x(U)=x V Uy ≺VN y (V).N x(U)is called the degree to which U is a neighborhood of x .A set X equipped with an L-fuzzy neighborhood system N={N x:x ∈J(L X)},denoted by(X,N),is called anL-fuzzy neighborhood space.A mapping f:X→Y between two L-fuzzy neighborhood spaces(X,N1)and(Y,N2)is called continuous if ∀x ∈J(L X),∀U∈L Y,(N2)f→L (x )(U) (N1)x (f←L(U)).It is easy to check that L-fuzzy neighborhood spaces and their continuous mappings form a category,denoted by L-FNS.Theorem3.2.Let T:L X→L be an L-fuzzy topology on X.∀x ∈J(L X),define a mapping N T x:L X→L byN T x(U)=x V UT(V).Then N T={N T x:x ∈J(L X)}is an L-fuzzy neighborhood system,which is called the L-fuzzy neighborhood systeminduced by T.Proof.First we prove the following:(LFN0)N T x(U)=≺ NTx(U).It is obvious that N T x(U)≺ NTx(U).Now we prove N T x(U)≺ NTx(U).Let a≺≺ NTx(U).Thena≺N T x(U)for any ≺ .Hence there exists V x∈L X such that x V x U and a T(V x )for any ≺ .LetW=≺ V x .Then we have that x W U anda≺ T(V x ) T⎛⎝≺V x⎞⎠=T(W)x V UT(V)=N T x(U).This shows N T x(U)≺ NTx(U).(LFN1)and(LFN2)are true trivially.(LFN3)From the definition of N T x,it follows that∀U,V∈L X,U V⇒N T x(U) N T x(V).Hence∀U,V∈L X,we always haveN T x(U∧V) N T x(U)∧N T x(V).In order to prove N T x(U∧V) N T x(U)∧N T x(V),let a≺N T x(U)∧N T x(V).Then a≺N T x(U)and a≺N T x(V).This implies thata≺x W U T(W)and a≺x W VT(W).1222F.-G.Shi/Fuzzy Sets and Systems160(2009)1218–1232 Hence there are W1and W2such thatx W1 U,x W2 V,a T(W1)and a T(W2).Thus we have thatx W1∧W2 U∧V and a T(W1)∧T(W2) T(W1∧W2). Thereforea T(W1∧W2)x W V∧U T(W)=N T x(U∧V).This shows N T x(U∧V) N T x(U)∧N T x(V).(LFN4)∀V∈L X with x V U and∀ ≺ ,obviously we haveT(V)y ≺V N T y(V) N T x(V) N T x(U).This impliesN T x(U)=x V UT(V)x V Uy ≺VN T y(V) N T x(U).Hence by(LFN0)we obtainN T x(U)x V Uy ≺VN T y(V)≺N T x(U)=N T x(U).ThereforeN T x(U)=x V Uy ≺VN T y(V).çThe following lemma shows that an L-fuzzy topology can be denoted by its L-fuzzy neighborhood system.Lemma3.3.For an L-fuzzy topology T on X and for any U∈L X,it follows that T(U)=x ≺UN T x(U).Proof.It is obvious that T(U)x ≺UN T x(U).Now we prove T(U)x ≺UN T x(U).Let a≺x ≺UN T x(U).Then for any x ≺U,there exists V x ∈L X such that x V x U and a T(V x ).Obviously U=x ≺U V x .Thuswe haveT(U)=T ⎛⎝x ≺UV x⎞⎠x ≺UT(V x) a.This shows T(U)x ≺UN T x(U).çTheorem3.4.If f:(X,T1)→(Y,T2)is continuous with respect to L-fuzzy topologies T1and T2,then f:(X,N T1)→(Y,N T2)is also continuous with respect to L-fuzzy neighborhood systems N T1and N T2.Proof.If f:(X,T1)→(Y,T2)is continuous with respect to L-fuzzy topologies T1and T2,then∀U∈L Y, T2(U) T1(f←L(U)),this implies that∀x ∈J(L X),N T2f→L (x )(U)=f→L(x ) V UT2(V)x f←L(V) f←L(U)T1(f←L(V)) N T1x(f←L(U)).Therefore f:(X,N T1)→(Y,N T2)is continuous.çF .-G.Shi /Fuzzy Sets and Systems 160(2009)1218–12321223Theorem 3.5.Let N ={N x :x ∈J (L X )}be an L-fuzzy neighborhood system on X.Define T N :L X →L byT N (U )=x ≺UN x (U ).Then T N is an L-fuzzy topology on X and N (TN )=N .Proof.Check that T N is an L -fuzzy topology on X as follows:(LFT1)is trivial from (LFN1).(LFT2)can be proved by the following fact:∀U ,V ∈L X ,T N(U ∧V )=x ≺U ∧VN x (U ∧V )=x ≺U ∧VN x (U )∧N x (V )⎛⎝ x ≺UN x (U )⎞⎠∧⎛⎝ x ≺VN x (V )⎞⎠=T N (U )∧T N (V ).(LFT3)holds by the following fact:∀{U i :i ∈ }⊆L X ,T N i ∈U i = x ≺i ∈U iN x i ∈U i = i ∈ x ≺U iN x i ∈U ii ∈x ≺U iN x (U i )= i ∈T N (U i ).By (LFN4)and Theorem 3.2we can obtain N (T N )=N .çBy Lemma 3.3we can prove the next result.Corollary 3.6.For an L-fuzzy topology T on X ,it follows that T =T (NT).Theorem 3.7.If f :(X ,N 1)→(Y ,N 2)is continuous with respect to L-fuzzy neighborhood systems N 1and N 2,then f :(X ,T N 1)→(Y ,T N 2)is continuous with respect to L-fuzzy topologies T N 1and T N 2.Proof.If f :(X ,N 1)→(Y ,N 2)is continuous with respect to L -fuzzy neighborhood systems N 1and N 2,then ∀U ∈L Y ,∀x ∈J (L X ),(N 2)f →L (x)(U ) (N 1)x (f ←L (U )),this impliesTN 2(U )=y ≺U(N 2)y (U )f →L (x)≺U (N 2)f →L(x)(U )=x ≺f ←L(U )(N 2)f →L(x)(U )x ≺f ←L(U )(N 1)x (f ←L (U ))=T N 1(f ←L (U )).Therefore f :(X ,T N 1)→(Y ,T N 2)is continuous.çThe next result follows from Theorems 3.4,3.5,3.7and Corollary 3.6.Theorem 3.8.L -FTOP is isomorphic to L -FNS .The above results show that L -fuzzy topologies and L -fuzzy neighborhood systems come to the same thing.It maybe a convenient way to investigate local properties of an L -fuzzy topology by means of L -fuzzy neighborhood systems or L -fuzzy quasi-neighborhood systems.1224F .-G.Shi /Fuzzy Sets and Systems 160(2009)1218–12324.L -fuzzy interior operatorsIn this section,we shall introduce a new definition of L -fuzzy interior operators,and study the relation between them and L -fuzzy topologies.For an L -topological space (X , ),its L -interior operator i :L X →L X satisfies the following conditions:(1)i ( )= ;(2)i (A ) A ;(3)i (A ∧B )=i (A )∧i (B );(4)i (A ) i (i (A )).Now we consider such a function I (A ):J (L X )→2defined byI (A )(x )=1,x i (A ),0,x i (A ).It is easy to check the following conditions:(LI1)I (A )(x )=1⇔∀ ≺ ,I (A )(x )=1,∀x ∈J (L X );(LI2)I ( )(x )=1for any x ∈J (L X );(LI3)I (A )(x )=0for any x A ;(LI4)I (A ∧B )=I (A )∧I (B );(LI5)(I (A ))(0)⊆(I ( (I (A ))(0)))(0).Based on the above fact,we can generalize L -interior operators to L -fuzzy topological spaces as follows:Definition 4.1.An L -fuzzy interior operator on X is a map Int :L X →L J (LX )satisfying the following conditions:(LFI1)Int(A )(x )=≺ Int(A )(x ),∀x ∈J (L X );(LFI2)Int( )(x )= for any x ∈J (L X );(LFI3)Int(A )(x )=⊥for any x A ;(LFI4)Int(A ∧B )=Int(A )∧Int(B );(LFI5)∀a ∈L \{ },(Int(A ))(a )⊆(Int((Int(A ))(a )))(a ).Int(A )(x )is called the degree to which x belongs to the interior of A .A set X equipped with an L -fuzzy interior operator Int (or Int X ),denoted by (X ,Int),is called an L -fuzzy interior space.A mapping f :X →Y between two L -fuzzy interior spaces (X ,Int X )and (Y ,Int Y )is called continuous if ∀x ∈J (L X ),∀U ∈L Y ,Int Y (U )(f →L (x )) Int X (f ←L (U ))(x ).It is easy to check that L -fuzzy interior spaces and their continuous mappings form a category,denoted by L -FIS .The following theorem explains the relation between the L -fuzzy neighborhood system and the L -fuzzy interior operator of an L -fuzzy topology.Theorem 4.2.Let T be an L-fuzzy topology on X and let N T ={N T x:x ∈J (L X )}be the L-fuzzy neighborhood system induced by T .Define a mapping Int T :L X →L J (L X )byInt T (A )(x )=N Tx(A ).Then Int T is an L-fuzzy interior operator on X ,which is called the L-fuzzy interior operator induced by T .Proof.(LFI1)–(LFI3)are obvious.(LFI4)can be obtained from (LFN3).In order to prove (LFI5),let x ∈(Int T (A ))(a ).ThenInt T (A )(x )=N Tx(A )= x U Ay ≺UN Ty(U ) a .F .-G.Shi /Fuzzy Sets and Systems 160(2009)1218–12321225Hence there exists U ∈L X such that x U A andy ≺UInt T (U )(y )=y ≺UN T y(U ) a .This implies that y ∈(Int T (U ))(a )holds for any y ≺U .By (LFI4)we havex U = {y :y ≺U } (Int T (U ))(a )(Int T (A ))(a ).ThereforeIntT(Int T(A ))(a )(x )=N Tx(Int T(A ))(a )=x U(Int T (A ))(a )y ≺UN T y(U ) a .This shows x ∈(Int T ((Int T (A ))(a )))(a ).The proof of (LFI5)is completed.çThe next corollary give a representation of the L -fuzzy interior operator by means of an L -fuzzy topology.It can be obtained from Theorems 3.2and 4.2.Corollary 4.3.Let T be an L-fuzzy topology on X and let Int T be the L-fuzzy interior operator induced by T .Then ∀x ∈J (L X ),∀A ∈L X ,Int T (A )(x )=x V AT (V ).Theorem 4.4.If f :(X ,T 1)→(Y ,T 2)is continuous with respect to L-fuzzy topologies T 1and T 2,then f :(X ,Int T 1)→(Y ,Int T 2)is continuous with respect to L-fuzzy interior operators Int T 1and Int T 2.Proof.If f :(X ,T 1)→(Y ,T 2)is continuous with respect to L -fuzzy topologies T 1and T 2,then ∀U ∈L Y ,T 2(U ) T 1(f ←L (U )),this implies that ∀x∈J (L X ),Int T 2(U )(f →L (x ))= f →L (x) V U T 2(V )x f ←L (V ) f ←L(U )T 1(f ←L (V )) Int T 1(f ←L (U ))(x ).Therefore f :(X ,Int T 1)→(Y ,Int T 2)is continuous.çTheorem 4.5.Let Int :L X →L J (LX )be an L-fuzzy interior operator on X .∀x ∈J (L X ),define N Int x:L X →L by N Int x(A )=Int(A )(x ).Then N Int ={N Int x:x ∈J (L X )}is an L-fuzzy neighborhood system on X .Proof.(LFN1)–(LFN3)holds trivially from (LFI2)–(LFI4).We need to prove (LFN4).First we check N Int x (U ) x V U y ≺V N Int y (V ).Suppose that b ∈L and N Int x(U ) b .Then there exists a ∈ (b )such that N Int x (U ) a .This implies Int(U )(x ) a .By (LFI5)we havex ∈(Int(U ))(a )⊆ Int (Int(U ))(a )(a ).Let V =(Int(U ))(a ).Then x V U and there exists y ∈(Int(U ))(a )such that y y for any y ≺V .Furtherwe havey ∈(Int(U ))(a )⊆ Int (Int(U ))(a )(a )=(Int(V ))(a ).1226F.-G.Shi/Fuzzy Sets and Systems160(2009)1218–1232 This implies Int(V)(y ) a.By(LFI1)we know Int(V)(y ) Int(V)(y ).Hence N Int y(V)=Int(V)(y ) a.Thereforey ≺VN Int y(V) b.This showsx V Uy ≺VN Int y(V) b.The proof of N Int x(U)x V Uy ≺V N Int y(V)is completed.Second we check N Int x(U)x V Uy ≺VN Int y(V).Suppose that b∈L andx V Uy ≺VN Int y(V) b.Then there exists a∈ (b)such thatx V Uy ≺VN Int y(V) a.Further there exists V∈L X such that x V U andy ≺VN Int y(V) a.Hence N Int x(V) a for any≺ .By(LFI1)we haveN Int x(U)=Int(U)(x )=≺Int(U)(x )≺Int(V)(x )=≺N Int x(V) b.This shows N Int x(U)x V Uy ≺VN Int y(V).çBy Theorems3.5,4.2and4.5we can obtain the following result.Corollary4.6.Let Int:L X→L J(L X)be an L-fuzzy interior operator on X.Define T Int:L X→L byT Int(U)=x ≺UInt(U)(x ).Then T Int is an L-fuzzy topology on X and Int(T Int)=Int.By Corollaries3.6,4.3and Theorem4.5we can obtain the following result.Corollary4.7.For an L-fuzzy topology T on X,T(Int T)=T.Theorem4.8.If f:(X,Int X)→(Y,Int Y)is continuous with respect to L-fuzzy interior operators Int X and Int Y,then f:(X,T Int X)→(Y,T Int Y)is continuous with respect to L-fuzzy topologies T Int X and T Int Y.Proof.If f:(X,Int X)→(Y,Int Y)is continuous with respect to L-fuzzy interior operators Int X and Int Y,then ∀U∈L Y,∀x ∈J(L X),Int Y(U)(f→(x )) Int X(f←(U))(x ),this impliesT Int Y(U)=y ≺U Int Y(U)(y )f→L(x )≺UInt Y(U)(f→L(x ))=x ≺f←L(U)Int Y(U)(f→L(x ))x ≺f←L(U)Int X(f←L(U))(x )=T Int X(f←L(U)).Therefore f:(X,T Int X)→(Y,T Int Y)is continuous.çThe next result follows from Theorems4.4,4.8and Corollaries4.6,4.7.Theorem4.9.L-FTOP is isomorphic to L-FIS.The above results show that L-fuzzy topologies and L-fuzzy interior operators come to the same thing.F .-G.Shi /Fuzzy Sets and Systems 160(2009)1218–123212275.L -fuzzy closure operatorsIn this section,we shall introduce a new definition of L -fuzzy closure operators,and study the relation between them and L -fuzzy topologies.For an L -topological space (X , ),its L -closure operator c :L X →L X satisfies the following conditions:(1)c (⊥)=⊥;(2)c (A ) A ;(3)i (A ∨B )=c (A )∨c (B );(4)c (A ) c (c (A )).Now we consider such a function C (A ):J (L X )→2defined byC (A )(x )=1,x c (A ),0,x c (A ).It is easy to check the following conditions:(LC1)C (A )(x )=1⇔∀ ≺ ,C (A )(x )=1,∀x ∈J (L X );(LC2)C (⊥)(x )=0for any x ∈J (L X );(LC3)C (A )(x )=1for any x A ;(LC4)C (A ∨B )=C (A )∨C (B );(LC5)(C ((C (A ))[1]))[1]⊆(C (A ))[1].Based on the above fact,we can generalize L -closure operators to L -fuzzy topological spaces as follows:Definition 5.1.An L -fuzzy closure operator on X is a mapping Cl :L X →L J (L X )satisfying the following conditions:(LFC1)Cl(A )(x )=≺ Cl(A )(x ),∀x ∈J (L X );(LFC2)Cl(⊥)(x )=⊥for any x ∈J (L X );(LFC3)Cl(A )(x )= for any x A ;(LFC4)Cl(A ∨B )=Cl(A )∨Cl(B );(LFC5)∀a ∈L \{⊥},(Cl((Cl(A ))[a ]))[a ]⊆(Cl(A ))[a ].Cl(A )(x )is called the degree to which x belongs to the closure of A .A set X equipped with an L -fuzzy closure operator Cl (or Cl X ),denoted by (X ,Cl),is called an L -fuzzy closure space.A mapping f :X →Y between two L -fuzzy closure spaces (X ,Cl X )and (Y ,Cl Y )is called continuous if ∀x ∈J (L X ),∀A ∈L X ,Cl X (A )(x ) Cl Y (f →L (A ))(f →L (x )).It is easy to check that L -fuzzy closure spaces and their continuous mappings form a category,denoted by L -FCS .The next theorem is obvious.Theorem 5.2.A mapping f :X →Y between two L-fuzzy closure spaces (X ,Cl X )and (Y ,Cl Y )is continuous if and only if ∀x ∈J (L X ),∀B ∈L Y ,Cl X (f ←L (B ))(x ) Cl Y (B )(f →L (x )).The following theorem explains the relation between the L -fuzzy quasi-neighborhood system and the L -fuzzy closure operator of an L -fuzzy topology.Theorem 5.3.Let T be an L-fuzzy topology on X and let Q ={Q x :x ∈J (L X )}be the quasi-neighborhood systeminduced by T .Define a mapping Cl T :L X →L J (L X)byCl T (A )(x )=(Q x (A )) .Then Cl T is an L-fuzzy closure operator on X ,which is called the L-fuzzy closure operator induced by T .Proof.(LFC1)–(LFC3)are obvious.(LFC4)holds from(LFQ3).Now we prove(LFC5). (LFC5)∀a∈L\{⊥},let x /∈(Cl T(A))[a].Then by(LFQ4)we knowx D Ay DQ y (D )=Q x(A )=(Cl T(A)(x )) a .Hence there exists D∈L X such that x D A andy D (Cl T(D)(y )) =y DQ y (D ) a .This implies that y /∈(Cl T(D))[a]holds for any y D.By(LFC4)we havex D(Cl T(D))[a](Cl T(A))[a].ThereforeCl T(Cl T(A))[a](x )=Q x(Cl T(A))[a]=x D(Cl T(A))[a]y DQ y (D ) a .This shows x /∈(Cl T((Cl T(A))[a]))[a].The proof of(LFC5)is completed.çThe next corollary give a representation of the L-fuzzy closure operator by means of an L-fuzzy topology. Corollary5.4.Let T be an L-fuzzy topology on X and let Cl T be the L-fuzzy closure operator induced by T.Then ∀x ∈J(L X),∀A∈L X,Cl T(A)(x )=x D A(T(D )) .Proof.By Theorem5.3and Q y(A )=x D AT(D )we can prove the corollary.çTheorem5.5.If f:(X,T1)→(Y,T2)is continuous with respect to L-fuzzy topologies T1and T2,then f:(X,Cl T1)→(Y,Cl T2)is continuous with respect to L-fuzzy closure operators Cl T1and Cl T2.Proof.If f:(X,T1)→(Y,T2)is continuous with respect to L-fuzzy topologies T1and T2,then∀B∈L Y, T2(B) T1(f←L(B)),this implies that∀x ∈J(L X),Cl T2(B)(f→L(x ))=f→L(x ) D B (T2(D ))x f←L(D) f←L(B)T1(f←L(D ))Cl T1(f←L(B))(x ).Therefore f:(X,Cl T1)→(Y,Cl T2)is continuous.çTheorem5.6.Let Cl:L X→L J(L X)be an L-fuzzy closure operator on X.∀x ∈J(L X),define Q x :L X→L by Q x(A)=(Cl(A )(x )) .Then Q={Q x:x ∈J(L X)}is an L-fuzzy quasi-neighborhood system on X.Proof.(LFQ1)–(LFQ3)hold trivially from(LFC2)–(LFC4).We need to prove(LFQ4).First we check Q x(A)x D Ay DQ y (D ).Suppose that b ∈L and Q x (A ) b .Then there exists a ∈ (b )such that Q x (A ) a .This implies Cl(A )(x ) a .By (LFC5)we havex /∈(Cl(A))[a ]⊇ Cl (Cl(A))[a ][a ].Let D =(Cl(A ))[a ].We shall check x D A .In fact,if x D ,then for any ≺ ,there exists x ∈(Cl(A ))[a ]such that x x .By (LFC1)we know Cl(A )(x ) Cl(A )(x ) a .Further Cl(A )(x )=≺ Cl(A )(x ) a ,this contradicts to x /∈(Cl(A ))[a ].There-fore x D A .By(Cl(A))[a ]⊇ Cl (Cl(A))[a ][a ]=(Cl(D ))[a ].we know Cl(D )(y ) a for any y D .HenceQ y (D )=(Cl(D )(y )) a .Therefore y D Q y (D ) b .This shows x D A y D Q y (D ) b .The proof of Q x(A ) x D A y D Q y (D)is completed.Second we check Q x (A ) x D Ay D Q y (D ).Suppose that b ∈L and x D Ay D Q y (D ) b .Then there exists D ∈L X such that x D A and y D Q y (D ) b .Hence Q x (D) b .Q x (A )=(Cl(A )(x )) (Cl(D )(x )) =Q x (D ) b .This shows Q x (A ) x D Ay D Q y (D ).çTheorem 5.7.Let Cl :L X →L J (LX )be an L-fuzzy closure operator on X.Define T Cl :L X →L byTCl(U )=x U(Cl(U )(x )) .Then T Cl is an L-fuzzy topology on X and Cl (TCl )=Cl.Proof.By Proposition 3.9in [4]and Theorem 5.6we know that T Cl is an L -fuzzy topology on X .Cl (T Cl )=Cl can beproved from Theorems 5.3and 5.6.çAnalogously we have the following result.Corollary 5.8.For an L-fuzzy topology T on X ,T (ClT)=T .Theorem 5.9.If f :(X ,Cl X )→(Y ,Cl Y )is continuous with respect to L-fuzzy closure operators Cl X and Cl Y ,then f :(X ,T Cl X )→(Y ,T Cl Y )is continuous with respect to L-fuzzy topologies T Cl X and T Cl Y .Proof.If f :(X ,Cl X )→(Y ,Cl Y )is continuous with respect to L -fuzzy closure operators Cl X and Cl Y ,then ∀B ∈L Y ,∀x ∈J (L X ),Cl Y (B )(f →(x )) Cl X (f ←(B ))(x ),this impliesT Cl Y (B )=y B(Cl Y (B )(y ))f →L (x) B (Cl Y (B )(f →L (x )))=x f ←L (B )(Cl Y (B )(f →L (x )))x f ←L(B )(Cl X (f ←L (B ))(x )) =T Cl X (f ←L (B )).Therefore f :(X ,T Cl X )→(Y ,T Cl Y )is continuous.çThe next result follows from Theorems 5.5,5.7and Corollary 5.8.Theorem 5.10.L -FTOP is isomorphic to L -FCS .The above results show that L -fuzzy topologies and L -fuzzy closure operators come to the same thing.6.The relation between Int T and Cl TIn this section,we give the relation between the L -interior operator Int T and the L -fuzzy closure operator Cl T .For an L -topological space (X , ),as we know,Cl (A )=(Int (A )) for any A ∈L X .But for an L -fuzzy topological space (X ,T ),Cl T (A )=(Int T (A )) is not true generally,i.e.,it is possible that there exists x ∈J (L X )such that (Cl T (A )(x )) Int T (A )(x ).This can be seen from the following example.Example 6.1.Let X ={x }be a single point set and L ={1,0.7,0.5,0.3,0},where 1 =0,0.7 =0.3,0.5 =0.5.Define an L -fuzzy topology on X byT (1)=T (0)=1,T (0.7)=0.7,T (0.5)=0.5,T (0.3)=0.3.ThenInt T (0.7)(x )=V 0.7T (V )=⎧⎪⎪⎨⎪⎪⎩0, =1,0.7, =0.7,0.7, =0.5,0.7, =0.3,(Cl T (0.7 )(x )) = <V 0.7T (V )=⎧⎪⎪⎨⎪⎪⎩0.7, =1,0.7, =0.7,0.7, =0.5,0, =0.3.Obviously (Cl T (A )) Int T (A ).However,we have the following relation theorem.Theorem 6.2.Let T be an L-fuzzy topology on X ,and let Int T and Cl T be ,respectively ,the L-fuzzy interior operatorand the L-fuzzy closure operator induced by T .Then ∀x ∈J (L X ),∀A ∈L X ,(1)Cl T (A )(x )=(Int T (A )(x )) ;(2)Int T (A )(x )=(Cl T (A )(x )) .Proof.(1)By Corollaries 4.3and 5.4we know that ∀x ∈J (L X ),∀A ∈L X ,Int T (A )(x )= x V AT (V ),(Cl T (A )(x )) =x D AT (D ).。