H Background-foreground segmentation based on dominant motion estimation and static segment
【IT专家】背景减除算法之K

背景减除算法之K2017/12/16 446 Python版本:3.5.2,Opencv版本:3.2.0,网上安装教程很多,在此不再赘述MOG2算法,即高斯混合模型分离算法,是MOG的改进算法。
它基于Z.Zivkovic发布的两篇论文,即2004年发布的“Improved adaptive Gausian mixture model for background subtraction”和2006年发布的“Efficient Adaptive Density Estimation per Image Pixel for the Task of Background Subtraction”中提出。
KNN算法,即K-nearest neigbours - based Background/Foreground Segmentation Algorithm。
2006年,由Zoran Zivkovic 和Ferdinand van der Heijden在论文”Efficient adaptive density estimation per image pixel for the task of background subtraction.”中提出。
下面介绍两种算法的具体实现,实验中所用到的视频为CASIA步态数据库,用以上两种算法分别提取步态轮廓图像步态视频已上传到百度云,步态视频,提取密码:9mt0 (一)MOG2算法实现import numpy as npimport cv2cap=cv2.VideoCapture(‘D:\gait-vedio\gait.avi’)#混合高斯,对每一帧的环境进行学习,常用来对不同帧进行比较,并存储以前的帧,可按时间推移的方法提高运动分析的结果。
fgbg=cv2.createBackgroundSubtractorMOG2() while(1): #默认第一帧为背景图片ret,frame=cap.read() fgmask=fgbg.apply(frame) cv2.imshow(‘frame’,fgmask) k=cv2.waitKey(30) 0xff#按’q’键退出循环if k== ord(‘q’): breakcap.release()cv2.destroyAllWindows() 实验结果,(一)KNN算法实现采用KNN实现视频图像的背景分割算法,并且保存每一帧图像(与视频文件在相同的文件夹) import cv2#视频文件路径datapath = “D:/test1gait/”bs = cv2.createBackgroundSubtractorKNN(detectShadows = False)#背景减除器,设置阴影检测#训练帧数history=20 bs.setHistory(history)frames=0camera = cv2.VideoCapture(datapath + “gait2.avi”)count = 0#对原始帧进行膨胀去噪,#前景区域二值化,将非白色(0-244)的非前景区域(包含背景以及阴影)均设为0,前景。
Multicamera People Tracking with a Probabilistic Occupancy Map
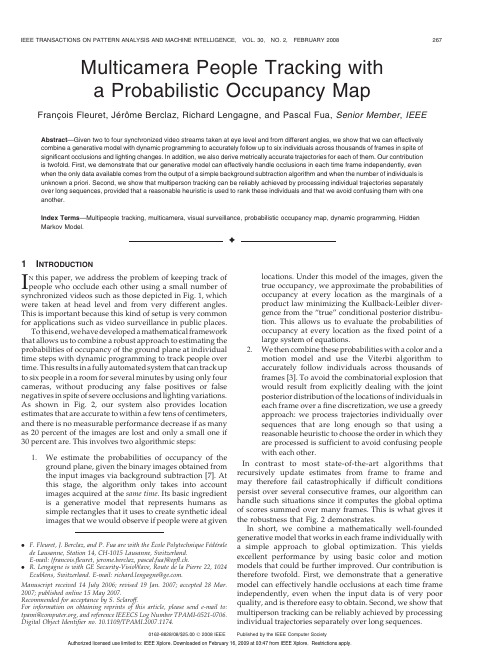
Multicamera People Tracking witha Probabilistic Occupancy MapFranc¸ois Fleuret,Je´roˆme Berclaz,Richard Lengagne,and Pascal Fua,Senior Member,IEEE Abstract—Given two to four synchronized video streams taken at eye level and from different angles,we show that we can effectively combine a generative model with dynamic programming to accurately follow up to six individuals across thousands of frames in spite of significant occlusions and lighting changes.In addition,we also derive metrically accurate trajectories for each of them.Our contribution is twofold.First,we demonstrate that our generative model can effectively handle occlusions in each time frame independently,even when the only data available comes from the output of a simple background subtraction algorithm and when the number of individuals is unknown a priori.Second,we show that multiperson tracking can be reliably achieved by processing individual trajectories separately over long sequences,provided that a reasonable heuristic is used to rank these individuals and that we avoid confusing them with one another.Index Terms—Multipeople tracking,multicamera,visual surveillance,probabilistic occupancy map,dynamic programming,Hidden Markov Model.Ç1I NTRODUCTIONI N this paper,we address the problem of keeping track of people who occlude each other using a small number of synchronized videos such as those depicted in Fig.1,which were taken at head level and from very different angles. This is important because this kind of setup is very common for applications such as video surveillance in public places.To this end,we have developed a mathematical framework that allows us to combine a robust approach to estimating the probabilities of occupancy of the ground plane at individual time steps with dynamic programming to track people over time.This results in a fully automated system that can track up to six people in a room for several minutes by using only four cameras,without producing any false positives or false negatives in spite of severe occlusions and lighting variations. As shown in Fig.2,our system also provides location estimates that are accurate to within a few tens of centimeters, and there is no measurable performance decrease if as many as20percent of the images are lost and only a small one if 30percent are.This involves two algorithmic steps:1.We estimate the probabilities of occupancy of theground plane,given the binary images obtained fromthe input images via background subtraction[7].Atthis stage,the algorithm only takes into accountimages acquired at the same time.Its basic ingredientis a generative model that represents humans assimple rectangles that it uses to create synthetic idealimages that we would observe if people were at givenlocations.Under this model of the images,given thetrue occupancy,we approximate the probabilities ofoccupancy at every location as the marginals of aproduct law minimizing the Kullback-Leibler diver-gence from the“true”conditional posterior distribu-tion.This allows us to evaluate the probabilities ofoccupancy at every location as the fixed point of alarge system of equations.2.We then combine these probabilities with a color and amotion model and use the Viterbi algorithm toaccurately follow individuals across thousands offrames[3].To avoid the combinatorial explosion thatwould result from explicitly dealing with the jointposterior distribution of the locations of individuals ineach frame over a fine discretization,we use a greedyapproach:we process trajectories individually oversequences that are long enough so that using areasonable heuristic to choose the order in which theyare processed is sufficient to avoid confusing peoplewith each other.In contrast to most state-of-the-art algorithms that recursively update estimates from frame to frame and may therefore fail catastrophically if difficult conditions persist over several consecutive frames,our algorithm can handle such situations since it computes the global optima of scores summed over many frames.This is what gives it the robustness that Fig.2demonstrates.In short,we combine a mathematically well-founded generative model that works in each frame individually with a simple approach to global optimization.This yields excellent performance by using basic color and motion models that could be further improved.Our contribution is therefore twofold.First,we demonstrate that a generative model can effectively handle occlusions at each time frame independently,even when the input data is of very poor quality,and is therefore easy to obtain.Second,we show that multiperson tracking can be reliably achieved by processing individual trajectories separately over long sequences.. F.Fleuret,J.Berclaz,and P.Fua are with the Ecole Polytechnique Fe´de´ralede Lausanne,Station14,CH-1015Lausanne,Switzerland.E-mail:{francois.fleuret,jerome.berclaz,pascal.fua}@epfl.ch..R.Lengagne is with GE Security-VisioWave,Route de la Pierre22,1024Ecublens,Switzerland.E-mail:richard.lengagne@.Manuscript received14July2006;revised19Jan.2007;accepted28Mar.2007;published online15May2007.Recommended for acceptance by S.Sclaroff.For information on obtaining reprints of this article,please send e-mail to:tpami@,and reference IEEECS Log Number TPAMI-0521-0706.Digital Object Identifier no.10.1109/TPAMI.2007.1174.0162-8828/08/$25.00ß2008IEEE Published by the IEEE Computer SocietyIn the remainder of the paper,we first briefly review related works.We then formulate our problem as estimat-ing the most probable state of a hidden Markov process and propose a model of the visible signal based on an estimate of an occupancy map in every time frame.Finally,we present our results on several long sequences.2R ELATED W ORKState-of-the-art methods can be divided into monocular and multiview approaches that we briefly review in this section.2.1Monocular ApproachesMonocular approaches rely on the input of a single camera to perform tracking.These methods provide a simple and easy-to-deploy setup but must compensate for the lack of 3D information in a single camera view.2.1.1Blob-Based MethodsMany algorithms rely on binary blobs extracted from single video[10],[5],[11].They combine shape analysis and tracking to locate people and maintain appearance models in order to track them,even in the presence of occlusions.The Bayesian Multiple-BLob tracker(BraMBLe)system[12],for example,is a multiblob tracker that generates a blob-likelihood based on a known background model and appearance models of the tracked people.It then uses a particle filter to implement the tracking for an unknown number of people.Approaches that track in a single view prior to computing correspondences across views extend this approach to multi camera setups.However,we view them as falling into the same category because they do not simultaneously exploit the information from multiple views.In[15],the limits of the field of view of each camera are computed in every other camera from motion information.When a person becomes visible in one camera,the system automatically searches for him in other views where he should be visible.In[4],a background/foreground segmentation is performed on calibrated images,followed by human shape extraction from foreground objects and feature point selection extraction. Feature points are tracked in a single view,and the system switches to another view when the current camera no longer has a good view of the person.2.1.2Color-Based MethodsTracking performance can be significantly increased by taking color into account.As shown in[6],the mean-shift pursuit technique based on a dissimilarity measure of color distributions can accurately track deformable objects in real time and in a monocular context.In[16],the images are segmented pixelwise into different classes,thus modeling people by continuously updated Gaussian mixtures.A standard tracking process is then performed using a Bayesian framework,which helps keep track of people,even when there are occlusions.In such a case,models of persons in front keep being updated, whereas the system stops updating occluded ones,which may cause trouble if their appearances have changed noticeably when they re-emerge.More recently,multiple humans have been simulta-neously detected and tracked in crowded scenes[20]by using Monte-Carlo-based methods to estimate their number and positions.In[23],multiple people are also detected and tracked in front of complex backgrounds by using mixture particle filters guided by people models learned by boosting.In[9],multicue3D object tracking is addressed by combining particle-filter-based Bayesian tracking and detection using learned spatiotemporal shapes.This ap-proach leads to impressive results but requires shape, texture,and image depth information as input.Finally, Smith et al.[25]propose a particle-filtering scheme that relies on Markov chain Monte Carlo(MCMC)optimization to handle entrances and departures.It also introduces a finer modeling of interactions between individuals as a product of pairwise potentials.2.2Multiview ApproachesDespite the effectiveness of such methods,the use of multiple cameras soon becomes necessary when one wishes to accurately detect and track multiple people and compute their precise3D locations in a complex environment. Occlusion handling is facilitated by using two sets of stereo color cameras[14].However,in most approaches that only take a set of2D views as input,occlusion is mainly handled by imposing temporal consistency in terms of a motion model,be it Kalman filtering or more general Markov models.As a result,these approaches may not always be able to recover if the process starts diverging.2.2.1Blob-Based MethodsIn[19],Kalman filtering is applied on3D points obtained by fusing in a least squares sense the image-to-world projections of points belonging to binary blobs.Similarly,in[1],a Kalman filter is used to simultaneously track in2D and3D,and objectFig.1.Images from two indoor and two outdoor multicamera video sequences that we use for our experiments.At each time step,we draw a box around people that we detect and assign to them an ID number that follows them throughout thesequence.Fig.2.Cumulative distributions of the position estimate error on a3,800-frame sequence(see Section6.4.1for details).locations are estimated through trajectory prediction during occlusion.In[8],a best hypothesis and a multiple-hypotheses approaches are compared to find people tracks from 3D locations obtained from foreground binary blobs ex-tracted from multiple calibrated views.In[21],a recursive Bayesian estimation approach is used to deal with occlusions while tracking multiple people in multiview.The algorithm tracks objects located in the intersections of2D visual angles,which are extracted from silhouettes obtained from different fixed views.When occlusion ambiguities occur,multiple occlusion hypotheses are generated,given predicted object states and previous hypotheses,and tested using a branch-and-merge strategy. The proposed framework is implemented using a customized particle filter to represent the distribution of object states.Recently,Morariu and Camps[17]proposed a method based on dimensionality reduction to learn a correspondence between the appearance of pedestrians across several views. This approach is able to cope with the severe occlusion in one view by exploiting the appearance of the same pedestrian on another view and the consistence across views.2.2.2Color-Based MethodsMittal and Davis[18]propose a system that segments,detects, and tracks multiple people in a scene by using a wide-baseline setup of up to16synchronized cameras.Intensity informa-tion is directly used to perform single-view pixel classifica-tion and match similarly labeled regions across views to derive3D people locations.Occlusion analysis is performed in two ways:First,during pixel classification,the computa-tion of prior probabilities takes occlusion into account. Second,evidence is gathered across cameras to compute a presence likelihood map on the ground plane that accounts for the visibility of each ground plane point in each view. Ground plane locations are then tracked over time by using a Kalman filter.In[13],individuals are tracked both in image planes and top view.The2D and3D positions of each individual are computed so as to maximize a joint probability defined as the product of a color-based appearance model and2D and 3D motion models derived from a Kalman filter.2.2.3Occupancy Map MethodsRecent techniques explicitly use a discretized occupancy map into which the objects detected in the camera images are back-projected.In[2],the authors rely on a standard detection of stereo disparities,which increase counters associated to square areas on the ground.A mixture of Gaussians is fitted to the resulting score map to estimate the likely location of individuals.This estimate is combined with a Kallman filter to model the motion.In[26],the occupancy map is computed with a standard visual hull procedure.One originality of the approach is to keep for each resulting connex component an upper and lower bound on the number of objects that it can contain. Based on motion consistency,the bounds on the various components are estimated at a certain time frame based on the bounds of the components at the previous time frame that spatially intersect with it.Although our own method shares many features with these techniques,it differs in two important respects that we will highlight:First,we combine the usual color and motion models with a sophisticated approach based on a generative model to estimating the probabilities of occu-pancy,which explicitly handles complex occlusion interac-tions between detected individuals,as will be discussed in Section5.Second,we rely on dynamic programming to ensure greater stability in challenging situations by simul-taneously handling multiple frames.3P ROBLEM F ORMULATIONOur goal is to track an a priori unknown number of people from a few synchronized video streams taken at head level. In this section,we formulate this problem as one of finding the most probable state of a hidden Markov process,given the set of images acquired at each time step,which we will refer to as a temporal frame.We then briefly outline the computation of the relevant probabilities by using the notations summarized in Tables1and2,which we also use in the following two sections to discuss in more details the actual computation of those probabilities.3.1Computing the Optimal TrajectoriesWe process the video sequences by batches of T¼100frames, each of which includes C images,and we compute the most likely trajectory for each individual.To achieve consistency over successive batches,we only keep the result on the first 10frames and slide our temporal window.This is illustrated in Fig.3.We discretize the visible part of the ground plane into a finite number G of regularly spaced2D locations and we introduce a virtual hidden location H that will be used to model entrances and departures from and into the visible area.For a given batch,let L t¼ðL1t;...;L NÃtÞbe the hidden stochastic processes standing for the locations of individuals, whether visible or not.The number NÃstands for the maximum allowable number of individuals in our world.It is large enough so that conditioning on the number of visible ones does not change the probability of a new individual entering the scene.The L n t variables therefore take values in f1;...;G;Hg.Given I t¼ðI1t;...;I C tÞ,the images acquired at time t for 1t T,our task is to find the values of L1;...;L T that maximizePðL1;...;L T j I1;...;I TÞ:ð1ÞAs will be discussed in Section 4.1,we compute this maximum a posteriori in a greedy way,processing one individual at a time,including the hidden ones who can move into the visible scene or not.For each one,the algorithm performs the computation,under the constraint that no individual can be at a visible location occupied by an individual already processed.In theory,this approach could lead to undesirable local minima,for example,by connecting the trajectories of two separate people.However,this does not happen often because our batches are sufficiently long.To further reduce the chances of this,we process individual trajectories in an order that depends on a reliability score so that the most reliable ones are computed first,thereby reducing the potential for confusion when processing the remaining ones. This order also ensures that if an individual remains in the hidden location,then all the other people present in the hidden location will also stay there and,therefore,do not need to be processed.FLEURET ET AL.:MULTICAMERA PEOPLE TRACKING WITH A PROBABILISTIC OCCUPANCY MAP269Our experimental results show that our method does not suffer from the usual weaknesses of greedy algorithms such as a tendency to get caught in bad local minima.We thereforebelieve that it compares very favorably to stochastic optimization techniques in general and more specifically particle filtering,which usually requires careful tuning of metaparameters.3.2Stochastic ModelingWe will show in Section 4.2that since we process individual trajectories,the whole approach only requires us to define avalid motion model P ðL n t þ1j L nt ¼k Þand a sound appearance model P ðI t j L n t ¼k Þ.The motion model P ðL n t þ1j L nt ¼k Þ,which will be intro-duced in Section 4.3,is a distribution into a disc of limited radiusandcenter k ,whichcorresponds toalooseboundonthe maximum speed of a walking human.Entrance into the scene and departure from it are naturally modeled,thanks to the270IEEE TRANSACTIONS ON PATTERN ANALYSIS AND MACHINE INTELLIGENCE,VOL.30,NO.2,FEBRUARY 2008TABLE 2Notations (RandomQuantities)Fig.3.Video sequences are processed by batch of 100frames.Only the first 10percent of the optimization result is kept and the rest is discarded.The temporal window is then slid forward and the optimiza-tion is repeated on the new window.TABLE 1Notations (DeterministicQuantities)hiddenlocation H,forwhichweextendthemotionmodel.The probabilities to enter and to leave are similar to the transition probabilities between different ground plane locations.In Section4.4,we will show that the appearance model PðI t j L n t¼kÞcan be decomposed into two terms.The first, described in Section4.5,is a very generic color-histogram-based model for each individual.The second,described in Section5,approximates the marginal conditional probabil-ities of occupancy of the ground plane,given the results of a background subtractionalgorithm,in allviewsacquired atthe same time.This approximation is obtained by minimizing the Kullback-Leibler divergence between a product law and the true posterior.We show that this is equivalent to computing the marginal probabilities of occupancy so that under the product law,the images obtained by putting rectangles of human sizes at occupied locations are likely to be similar to the images actually produced by the background subtraction.This represents a departure from more classical ap-proaches to estimating probabilities of occupancy that rely on computing a visual hull[26].Such approaches tend to be pessimistic and do not exploit trade-offs between the presence of people at different locations.For instance,if due to noise in one camera,a person is not seen in a particular view,then he would be discarded,even if he were seen in all others.By contrast,in our probabilistic framework,sufficient evidence might be present to detect him.Similarly,the presence of someone at a specific location creates an occlusion that hides the presence behind,which is not accounted for by the hull techniques but is by our approach.Since these marginal probabilities are computed indepen-dently at each time step,they say nothing about identity or correspondence with past frames.The appearance similarity is entirely conveyed by the color histograms,which has experimentally proved sufficient for our purposes.4C OMPUTATION OF THE T RAJECTORIESIn Section4.1,we break the global optimization of several people’s trajectories into the estimation of optimal individual trajectories.In Section 4.2,we show how this can be performed using the classical Viterbi’s algorithm based on dynamic programming.This requires a motion model given in Section 4.3and an appearance model described in Section4.4,which combines a color model given in Section4.5 and a sophisticated estimation of the ground plane occu-pancy detailed in Section5.We partition the visible area into a regular grid of G locations,as shown in Figs.5c and6,and from the camera calibration,we define for each camera c a family of rectangular shapes A c1;...;A c G,which correspond to crude human silhouettes of height175cm and width50cm located at every position on the grid.4.1Multiple TrajectoriesRecall that we denote by L n¼ðL n1;...;L n TÞthe trajectory of individual n.Given a batch of T temporal frames I¼ðI1;...;I TÞ,we want to maximize the posterior conditional probability:PðL1¼l1;...;L Nül NÃj IÞ¼PðL1¼l1j IÞY NÃn¼2P L n¼l n j I;L1¼l1;...;L nÀ1¼l nÀ1ÀÁ:ð2ÞSimultaneous optimization of all the L i s would beintractable.Instead,we optimize one trajectory after theother,which amounts to looking for^l1¼arg maxlPðL1¼l j IÞ;ð3Þ^l2¼arg maxlPðL2¼l j I;L1¼^l1Þ;ð4Þ...^l Nüarg maxlPðL Nül j I;L1¼^l1;L2¼^l2;...Þ:ð5ÞNote that under our model,conditioning one trajectory,given other ones,simply means that it will go through noalready occupied location.In other words,PðL n¼l j I;L1¼^l1;...;L nÀ1¼^l nÀ1Þ¼PðL n¼l j I;8k<n;8t;L n t¼^l k tÞ;ð6Þwhich is PðL n¼l j IÞwith a reduced set of the admissiblegrid locations.Such a procedure is recursively correct:If all trajectoriesestimated up to step n are correct,then the conditioning onlyimproves the estimate of the optimal remaining trajectories.This would suffice if the image data were informative enoughso that locations could be unambiguously associated toindividuals.In practice,this is obviously rarely the case.Therefore,this greedy approach to optimization has un-desired side effects.For example,due to partly missinglocalization information for a given trajectory,the algorithmmight mistakenly start following another person’s trajectory.This is especially likely to happen if the tracked individualsare located close to each other.To avoid this kind of failure,we process the images bybatches of T¼100and first extend the trajectories that havebeen found with high confidence,as defined below,in theprevious batches.We then process the lower confidenceones.As a result,a trajectory that was problematic in thepast and is likely to be problematic in the current batch willbe optimized last and,thus,prevented from“stealing”somebody else’s location.Furthermore,this approachincreases the spatial constraints on such a trajectory whenwe finally get around to estimating it.We use as a confidence score the concordance of theestimated trajectories in the previous batches and thelocalization cue provided by the estimation of the probabil-istic occupancy map(POM)described in Section5.Moreprecisely,the score is the number of time frames where theestimated trajectory passes through a local maximum of theestimated probability of occupancy.When the POM does notdetect a person on a few frames,the score will naturallydecrease,indicating a deterioration of the localizationinformation.Since there is a high degree of overlappingbetween successive batches,the challenging segment of atrajectory,which is due to the failure of the backgroundsubtraction or change in illumination,for instance,is met inseveral batches before it actually happens during the10keptframes.Thus,the heuristic would have ranked the corre-sponding individual in the last ones to be processed whensuch problem occurs.FLEURET ET AL.:MULTICAMERA PEOPLE TRACKING WITH A PROBABILISTIC OCCUPANCY MAP2714.2Single TrajectoryLet us now consider only the trajectory L n ¼ðL n 1;...;L nT Þof individual n over T temporal frames.We are looking for thevalues ðl n 1;...;l nT Þin the subset of free locations of f 1;...;G;Hg .The initial location l n 1is either a known visible location if the individual is visible in the first frame of the batch or H if he is not.We therefore seek to maximizeP ðL n 1¼l n 1;...;L n T ¼l nt j I 1;...;I T Þ¼P ðI 1;L n 1¼l n 1;...;I T ;L n T ¼l nT ÞP ðI 1;...;I T Þ:ð7ÞSince the denominator is constant with respect to l n ,we simply maximize the numerator,that is,the probability of both the trajectories and the images.Let us introduce the maximum of the probability of both the observations and the trajectory ending up at location k at time t :Èt ðk Þ¼max l n 1;...;l nt À1P ðI 1;L n 1¼l n 1;...;I t ;L nt ¼k Þ:ð8ÞWe model jointly the processes L n t and I t with a hidden Markov model,that isP ðL n t þ1j L n t ;L n t À1;...Þ¼P ðL n t þ1j L nt Þð9ÞandP ðI t ;I t À1;...j L n t ;L nt À1;...Þ¼YtP ðI t j L n t Þ:ð10ÞUnder such a model,we have the classical recursive expressionÈt ðk Þ¼P ðI t j L n t ¼k Þ|fflfflfflfflfflfflfflfflffl{zfflfflfflfflfflfflfflfflffl}Appearance modelmax P ðL n t ¼k j L nt À1¼ Þ|fflfflfflfflfflfflfflfflfflfflfflfflfflfflfflfflffl{zfflfflfflfflfflfflfflfflfflfflfflfflfflfflfflfflffl}Motion modelÈt À1ð Þð11Þto perform a global search with dynamic programming,which yields the classic Viterbi algorithm.This is straight-forward,since the L n t s are in a finite set of cardinality G þ1.4.3Motion ModelWe chose a very simple and unconstrained motion model:P ðL n t ¼k j L nt À1¼ Þ¼1=Z Áe À k k À k if k k À k c 0otherwise ;&ð12Þwhere the constant tunes the average human walkingspeed,and c limits the maximum allowable speed.This probability is isotropic,decreases with the distance from location k ,and is zero for k k À k greater than a constantmaximum distance.We use a very loose maximum distance cof one square of the grid per frame,which corresponds to a speed of almost 12mph.We also define explicitly the probabilities of transitions to the parts of the scene that are connected to the hidden location H .This is a single door in the indoor sequences and all the contours of the visible area in the outdoor sequences in Fig.1.Thus,entrance and departure of individuals are taken care of naturally by the estimation of the maximum a posteriori trajectories.If there are enough evidence from the images that somebody enters or leaves the room,then this procedure will estimate that the optimal trajectory does so,and a person will be added to or removed from the visible area.4.4Appearance ModelFrom the input images I t ,we use background subtraction to produce binary masks B t such as those in Fig.4.We denote as T t the colors of the pixels inside the blobs and treat the rest of the images as background,which is ignored.Let X tk be a Boolean random variable standing for the presence of an individual at location k of the grid at time t .In Appendix B,we show thatP ðI t j L n t ¼k Þzfflfflfflfflfflfflfflfflffl}|fflfflfflfflfflfflfflfflffl{Appearance model/P ðL n t ¼k j X kt ¼1;T t Þ|fflfflfflfflfflfflfflfflfflfflfflfflfflfflfflfflfflffl{zfflfflfflfflfflfflfflfflfflfflfflfflfflfflfflfflfflffl}Color modelP ðX kt ¼1j B t Þ|fflfflfflfflfflfflfflfflfflfflffl{zfflfflfflfflfflfflfflfflfflfflffl}Ground plane occupancy:ð13ÞThe ground plane occupancy term will be discussed in Section 5,and the color model term is computed as follows.4.5Color ModelWe assume that if someone is present at a certain location k ,then his presence influences the color of the pixels located at the intersection of the moving blobs and the rectangle A c k corresponding to the location k .We model that dependency as if the pixels were independent and identically distributed and followed a density in the red,green,and blue (RGB)space associated to the individual.This is far simpler than the color models used in either [18]or [13],which split the body area in several subparts with dedicated color distributions,but has proved sufficient in practice.If an individual n was present in the frames preceding the current batch,then we have an estimation for any camera c of his color distribution c n ,since we have previously collected the pixels in all frames at the locations272IEEE TRANSACTIONS ON PATTERN ANALYSIS AND MACHINE INTELLIGENCE,VOL.30,NO.2,FEBRUARY2008Fig.4.The color model relies on a stochastic modeling of the color of the pixels T c t ðk Þsampled in the intersection of the binary image B c t produced bythe background subtraction and the rectangle A ck corresponding to the location k .。
surveillance

Human Detection in Surveillance ApplicationsAshish DesaiAbstractTo attack the problem of detecting humans in a surveillance video, there are a few techniques that can be used. In this project, both a color based algorithm and a motion based algorithm were implemented, eventually leading to a combined approach. While each method had advantages and disadvantages, the combined approach led to an algorithm that worked exceptionally well with lateral movement relative to the camera, as well as with objects that were sufficiently far from the camera. While movement towards and away from the camera, especially very near to the camera caused some problems, I believe extensions to the project can improve upon the algorithm to make it very robust. IntroductionWhen trying to determine a good project to pursue regarding digital video processing, I decided to look around in my everyday life and look for a problem to solve. During this time, the parking garage I used often had petty vandalism problems, where criminals were breaking into the garage and vandalizing cars. To prevent this, our landlord had installed cameras, but this did not deter criminals, because they knew that the viewing of the tapes would happen later when they were long gone. So, I began to wonder: what if we had a real-time count of all the people in the garage, along with their pictures?Problem StatementReally this problem can be easily expanded to any surveillance application. In terms of our class, this breaks down to a foreground/background segmentation problem, which can then be coupled with segmenting the resulting foreground into separate objects. Formally, given a video sequence, I would like to determine the number of people present at every frame, as well as save a picture of them.MethodologyTo attack the segmentation problem, I decided to attempt two separate approaches (and eventually I decided to combine them). The first approach was to use a color based segmentation algorithm that will look at each pixel in a frame and determine whether it is in the foreground or background based on its color. The second approach was to use a segmentation algorithm based on motion estimation to determine whether each block in a particular frame was in the foreground or background based on the next frame in the sequence. Each of these methods is described in detail below.A 25-second video sequence was captured at 10 frames per second in my apartment where each frame contained between zero and three people. The algorithms described below were used to estimate the number of people in each frame and the results will be discussed in the subsequent section.Color Based SegmentationThe color-based segmentation that I used was based on the Staufer and Grimson paper given in class (see references). I do not want to restate the entire algorithm, but the basis for this method is that every pixel in a frame has a probability of being a particular color based on a mixture of multiple Gaussian probability distributions. If a particular pixel is considered a part of the background, it should remain constant (within a small variance due to noise sources) for long periods of time. However, as objects move in front of a background, a particular pixel will change color briefly and then return to the original color. Therefore, if we keep track of multiple Gaussian distributions for each pixel, and determine which of these Gaussians is in the foreground and which are in the background, we can make a good estimation for our current pixel, given the various probabilities. This is done by updating the mean and variance of each Gaussian based on the current values, determining the most probable Gaussian and determining whether this Gaussian is in the foreground or background. For more details, please refer to the Staufer and Grimson paper given in the reference section.For the most part, the algorithm was used as described in the paper, using a learning constant of α = 0.7 and K = 5 Gaussians. Some notable differences between my algorithm and that of the paper is that I determined that a pixel value must be closer than 1.5 standard deviations from the mean to be considered a sample from a particular Gaussian, versus Staufer and Grimson using 2.5 standard deviations. Also, I initialized the mean and variances of the Gaussians to random values with relatively large variances, which leads to the initial frames of the sequence to be considered complete background.Without regurgitating the Staufer and Grimson paper, each pixel is considered background if it is assigned to the Gaussian with the highest ratio of weight/variance (this effectively means it is the most recently present Gaussian with the highest probability). When simply implementing this algorithm, I found that in addition to the desired targets, many other pixels were still considered foreground. However, often they were isolated pixels, so I combined the above algorithm with a connectivity requirement that led to the elimination of any foreground pixel that was not immediately adjacent to four other classified foreground pixels.Finally, I took advantage of the fact that I knew I was looking for fairly large objects. After the connectivity requirement, there were clusters of pixels that were very close to each other but not touching. Therefore, I connected groups that were within a4x4 block of each other. To take advantage of the large target size, I finally eliminated any group that contained fewer than 100 pixels.As a note, I purposely wanted to set up my color segmentation algorithm to prevent any false detection of foreground. This was done so that when I later combined this with the motion estimation (described later to have high false detection) I could weight the confidence of the color segmentation very high (this will be addressed more in the combination section). Therefore, I chose a fairly high value for the learning constant so objects will quickly be determined as background. Also, because I only had 10 fps, and I wanted to converge very quickly, the high learning rate was essential.Motion Based SegmentationThe method for motion based segmentation was very simplistic, both because more time was spent to improve the color based segmentation, and because of the desireto reduce complexity for the long-term goal of a real-time algorithm. Basically, for each frame in the sequence, a simple block-matching algorithm was implemented between the current frame and the next frame. For the block matching, I used 16x16 blocks with full search over a +16/-15 search area with a SAD criterion. Then, a simple threshold criterion was chosen, in which any block that was determined to move more than 8 pixels (in any direction) was considered a foreground object. While I did look at other means to do segmentation, such as K-means clustering, I was trying to reduce complexity for the reasons stated above. Additionally, I wanted the output for the motion-based segmentation to have the property that it would not eliminate any foreground blocks by classifying them as background. This was imperative for the combination approach described below. Again, to reduce the effects of noise, I also put size constraints on any foreground objects such that any object that contained fewer than four blocks was no longer considered part of the foreground.Combination of Color and Motion Based SegmentationAs both of the previously described methods had their benefits and disadvantages (described in the Results section), I decided to combine the two methods to provide the best detection throughout the video sequence. As described above, the parameters for the color-based segmentation were chosen such that there was a very high confidence that its output was indeed foreground pixels, but perhaps not all of them were captured. Additionally, the motion-based segmentation was developed such that all the foreground pixels were captured, but perhaps many background pixels as well. Therefore, a weighting was done with each of the outputs where more weight was given to higher connected foreground pixels, and those in the color output were weighted heavier than those in the motion-based output. Finally, a threshold was heuristically determined to provide a nice balance between the two methods.The block diagram of the overall system is shown below in Figure 1.ResultsThroughout the methodology section, I alluded to some of the results of the various methods, as they led to modifications of some of the algorithms. Overall, the color based segmentation performed very well (I spent the most time adjusting the parameters of this method), while the motion based segmentation had an adequate performance.More specifically, the color based segmentation mainly had problems when there were large occlusions for a number frames, and then they disappeared. This is probably because the learning constant was chosen to be fairly high, so these large occlusions caused the previous background to be lost from memory. Also, the color based segmentation had problems with shadows being considered a foreground object. Overall, however, most of the output of the color based method was in fact foreground (but not necessarily all of the foreground).On the other hand, the motion based segmentation was designed to identify all foreground objects, with the trade-off of falsely identifying background as foreground. This method successfully did this, where the falsely detected background occurred mostly from reflective surfaces (possibly detected moving objects out of camera view) and the walls near the camera (possibly from automatic gain control of the camera when foreground objects moved towards or away from camera). Additionally, the motion estimation had some problems with aspect ratio changes, because this was based on block matching, rather than affine parameters. Finally, there were a few frames that underwent global motion by a person bumping the camera. This was not handled well by the motion based segmentation, because of the use of a simple threshold versus clustering of motion vectors.Finally, the combination of these methods actually worked quite well. For lateral movement only, the detection worked exceptionally well, whereas with large occlusions or aspect ratio changes still posed problems. Again, the combination performed better than the individual algorithms. Specifically, the problems with shadows and global motion were completely eliminated.Figure 2. Comparison of MethodsFigure 2 shows the output of the various methods, where a black pixel value means that it was considered background. Here we see that the color based segmentation was pretty accurate, the motion based identified the object, as well as some of the wall, and the combined method did an excellent job. The picture with the box around the foreground object is the final output given by the algorithm to provide the goal of counting the number of people and providing a picture. Note that this example frame involved only lateral motion.Figure 3. Number of People per FrameFigure 3 shows the actual number of people in each frame, as well as the estimated number given from the combined algorithm. From frames 0 through 100, there was either no motion, or only lateral motion. As was mentioned earlier, the algorithm works exceptionally well (mean error of 0.13 people per frame) with this type of motion. Frames 100 through 175, as well as Frames 195 through 251 include very large occlusions, as well as large changes in the aspect ratio of objects. Both because of lack of affine parameters and automatic gain control of the camera, the algorithm contains many errors during this period of time (mean error of 0.99 people per frame).Figure 4. Lateral Motion FrameFigure 4 shows a frame where only lateral motion occurs, and the algorithm works very well.Figure 5. Frame with Occlusion/Aspect Ratio ChangesFigure 5 shows a frame with changes in aspect ratio, occlusion, and possible automatic gain control changes (object suddenly appears immediately in front of camera). Notice the large number of false detections, as well as the missing detection of the large foreground object that just appeared in front of the camera (on the left).Figure 6. Aspect Ratio Change Far from CameraFigure 6 shows that the change in aspect ratio was detected properly as long as the object remained further away from the camera. Again, this may be due to the fact that the automatic gain control of the camera is not initiated, and the color based segmentation is weighted more heavily than the motion based segmentation.Figure 7. Connected Foreground ObjectsFigure 7 shows an instance where the algorithm tries to identify two connected people (on the left), but cannot accurately separate them. This is due to the fact that the only connectivity requirements and thresholds were used. A possible improvement upon this method would be to use the motion vectors to segment two foreground objects that may be connected based on other algorithms.ConclusionOverall, I believe that this project was very successful in achieving the goal of identifying the number of people in a frame as well as providing a picture of them. While there is always room for improvement, the algorithm works exceptionally well for lateral movement relative to the camera, and for objects that are not close enough to the camera to cause large occlusions of the background.To improve upon this algorithm, many other methods could be considered. For example, the use of affine parameters for motion-based segmentation would improve the problems with large aspect ratio changes. The use of a log-based search for block matching could provide an opportunity to make the algorithm truly real-time (I expect that this could have been achieved for the color based segmentation). If K-means clustering was used for motion-based segmentation, this would better handle global motion, as well as perhaps segment multiple people that are connected from the color algorithm. Finally, the implementation of a temporal constraint that would utilize the knowledge of detected humans from previous and/or future frames could definitely improve upon this algorithm.References1. Chris Stauffer and W.E.L. Grimson. “Adaptive background mixture models for real-time tracking”, CVPR99, Fort Colins, CO, (June 1999).。
对码本模型中码字结构的改进

对码本模型中码字结构的改进李文辉;李慧春;王莹;姜园媛;孙明玉【摘要】针对码本结构,提出一种简化算法.该算法通过将码字元组中判断该码字是否冗余的元素——最大未使用时间改为由元组的其他变量直接计算而不存储在码字中,去除了该变量所占用的空间,将6元组替换为5元组.实验结果表明,该改进不会对运动目标检测增加额外计算,准确性和实时性不受影响,并可减少码本模型占用的内存.%The codeword space was reduced according to calculating the longest interval so that codeword is never recurred by other variable in tuple, and the interval is not stored in codeword, thus 6-tuple based codeword is replaced by 5-tuple. The experimental result shows that the new codebook model is as fast and accurate as the original model. Moreover, the memory space demanded is reduced.【期刊名称】《吉林大学学报(理学版)》【年(卷),期】2012(050)003【总页数】6页(P517-522)【关键词】运动目标检测;码本模型;码字结构;5元组码字【作者】李文辉;李慧春;王莹;姜园媛;孙明玉【作者单位】吉林大学计算机科学与技术学院,长春130012;吉林大学计算机科学与技术学院,长春130012;吉林大学计算机科学与技术学院,长春130012;吉林大学计算机科学与技术学院,长春130012;吉林大学计算机科学与技术学院,长春130012【正文语种】中文【中图分类】TP391.4视频图像的运动目标检测是智能视频监控系统中最基本、最重要的技术. 提取运动目标较普遍的方法是背景相减法. 该方法的原理是将当前帧与背景模型做比较, 如果同位置的像素特征、像素区域特征或其他特征存在一定程度的相似性, 则当前帧这些位置的像素点或区域是背景, 其他区域构成前景运动目标区域[1].码本算法是Chalidabhongse和Kim等[2-3]提出的建立背景模型的方法. 码本的思想是:根据每个像素点连续采样值的颜色距离和亮度范围将背景像素值量化后用码本表示, 然后利用背景相减法的思想把新输入像素值与该点对应的码本做比较判断, 从而提取出前景运动目标.由于码本方法具有对复杂环境适应性强, 实时性好的优点, 因此在智能视频监控中作为运动目标检测方法得到广泛应用. 进一步, Kim等[4]又在码本算法中加入了两个重要改进----层次建模和自适应码本的更新, 增强了码本模型适应光线缓慢变化、场景物体运动等动态变化环境的能力. 在改善检测性能方面, 引入Markov随机场的码本模型在动态背景中能更有效地提取前景[5]. 把码本方法和HSV阴影去除方法相结合的“锥体-柱体混合”码本模型, 能消除阴影和强光对前景提取的影响[6]. 文献[7]提出的块均值码本模型(BMCB)和文献[8]提出的块和像素级连的码本模型都考虑了像素与其邻近像素的关系, 在复杂环境中可获得更准确的运动目标. 在提高码本算法的实时性方面, 文献[9]根据经验值设置每个码字长度的上限, 可减小码本算法对内存的需求; 文献[10]提出基于“盒子”的码本模型, 比Kim等[3]的码本算法计算量更少, 实时性更好.目前, 多数对码本算法的改进都关注于改善码本模型的检测效果和提高算法实时性两方面, 对于码字结构的改进却很少关注. 本文在不改变Kim等所提出约束条件的前提下, 对码字结构进行改进, 去除了码字中表示最大未使用时间的元素. 对码字结构的简化可减少码本模型的内存开销, 且不影响运动目标检测的准确性与实时性.1 码本背景模型描述1.1 构建像素码本假设训练阶段单个像素的采样值序列为X={x1,x2,…,xN}, X中的每个元素都是RGB向量, 训练帧数为N. 设C={c1,c2,…,cL}为该像素的码本, 码本中含有L个码字. 每个像素码本中的码字个数由采样值的变化情况决定. Kim等[3]提出的码字ci(i=1,2,…,L)包括两部分: RGB向量和6元组其中:和分别表示码字中的最小和最大亮度值; fi表示码字出现的频率;λi表示该码字没有出现的最大时间间隔;pi和qi分别表示码字第一次出现和最后一次出现的时间.训练阶段每个采样值xt(1≤t≤N)都和已有的码字进行比较. 找到(如果存在)最匹配的码字cm, 并对该码字进行更新;如果找不到匹配码字, 则为其创建一个新的码字存入码本中. 码本提取过程如下.算法1 构建像素码本.1) C ← Ø, L ← 0;在集合C={ci,1≤i≤L}中根据以下条件找到与xt匹配的码字cm:为采样阈值;如果C=Ø或无匹配, L ← L+1, 产生一个新的码字cL:vL←(R,G,B), auxL←〈I,I,1,t-1,t,t〉;(1)否则更新匹配的码字cm:end for;3) 消除冗余的码字. 对于ci(i=1,2,…,L):temp λi←max{λi,N-qi+pi-1};(4)初始码本为:M←{ckck∈C∧temp λk<Tλ}, k为码字的索引 //阈值Tλ常取训练帧数的一半, 即Tλ=N/2.1.2 颜色和亮度计算颜色距离和亮度范围的公式如下:其中α(α<1)和β(β>1)是限定亮度变化范围的因子, 通常取0.4≤α≤0.7, 1.1≤β≤1.5.1.3 用码本检测运动目标码本背景模型建立后, 可直接使用背景相减法获得运动目标. 利用码本方法检测x是否属于运动目标的算法过程BGS(x)如下.算法2 运动目标提取.2) 在M中根据以下条件寻找与x匹配的码字:colordist(x,vi)≤ε2,算法2中, ε2是检测阈值, 通常ε2>ε1.1.4 码本模型的更新初始训练后, 场景可能会发生变化. 如在街道上, 交通工具会进入或离开停车场. 此外, 光照变化也会导致背景的变化. 为了码本模型的更新, Kim等[3]引入了缓存码本, 缓存码本中的码字和背景码本中的码字结构相同. 码本的动态更新过程如下.算法3 码本模型更新.1) 训练结束后, 获得背景码本M, 建立缓存码本M′;2) 对于新像素, 在M中寻找匹配码字, 如果找到, 更新该码字;3) 如果没有找到, 在M′中寻找匹配码字并更新. 如果M′中没有匹配, 则建立新码字h, 并插入到M′中;4) 根据TM′精简M′, 即M′←M′-{hk′hk′∈M′, λk′>TM′};(7)5) 将在M′中停留足够时间的码字移到M中, 即M←M+{hk′hk′∈M′, fk′>Tadd};(8)6) 从M中删除超过一定时间未被匹配的码字, 即M←M-{ckck∈M, λk>TM}.(9)2 对码字结构的改进2.1 理论分析元素λi的作用是在训练结束和码本更新时作为删除冗余码字的依据. 训练过程中, λi的更新公式如下:λi=max{λi,t-qi}.(10)令λ′=t-qi,(11)则λ′表示码字再出现时未使用的时间, 由式(10)可见, λi是训练过程中最大的λ′. 精简码本时, 如果码字最后的λi≥Tλ, 则为冗余码字, 需要删除. 事实上, 并不需要找到λ′的最大值. 如果码字在t时刻, 已有λ′≥Tλ, 即可认为该码字为冗余的.同理, 在码本模型的更新中, 也不需要根据码字的最大未使用时间删除冗余码字. 如果背景码本M中码字的未使用时间超过TM, 或缓存码本M′中码字的未使用时间超过TM′, 则认为该码字可被删除.2.2 算法实现在去除表示码字最大未使用时间所占用的空间后, 还可以进一步减少训练过程所用时间:背景码本中的码字一定是在前Tλ帧中第一次出现的, 在后Tλ帧中才出现的码字一定不会是背景码本中的码字. 这是因为新码字建立时, 按式(1), λ=t-1, t为当前的时间, 即码字最大未使用时间λ的初值为码字第一次出现的时间减1, 在以后的训练过程中, λ的值不会小于该初值. 如果λ≥Tλ, 则训练结束后, 该码字也会被当作冗余码字去除.因此, 设码字结构中auxi为五元组:算法步骤如下.算法4 改进后的算法过程.1) for t=1 to Tλdo寻找和xt匹配的码字, 如果存在更新该码字;如果不存在建立新的码字;end for;2) for t=Tλ+1 to N do寻找与xt匹配的码字cm, 如果t-qm≥Tλ, 删除该码字;否则更新该码字;不为新出现的像素建立码字;end for;3) 训练结束后, 精简码本M←{ckck∈C∧(N-qk+pk-1)<Tλ},(12)k为码字的索引;4) for t>N to end do检测运动目标, 更新匹配的码字;更新码本:M′←M′{hk′hk′∈M′, t-qk′>TM′},M←M+{hk′hk′∈M′, fk′>Tadd},M←M-{ckck∈M, t-qk>TM}.算法4中k和k′为码字的索引. 为了提高码本算法的效率, 步骤4)中更新码本时可以隔一定帧数进行一次码本的更新, 如10帧, 即不必每帧都更新码本.3 实验结果与分析为了验证应用本文方法所建的模型占用内存空间少、并能有效地检测运动目标、实时性较Kim等[3]提出的方法好, 本文在微软公司及IBM公司提供的测试视频库上进行了测试, 所用机器配置为:双核CPU, 频率2.8 GHz, 1 G内存, 环境为VC++. 实验分为三部分:检测精度、处理时间及存储空间的对比. 实验中使用的相关数据如下:α=0.6, β=1.3, ε1=20, ε2=23.图1 运动目标检测实验效果Fig.1 Experimental results of motion detection 3.1 检测精度的对比图1为从两个视频中捕获的帧图像检测实验结果, 分别为人物视频和车辆视频.由图1可见, 本文方法和Kim等[3]提出的码本算法检测结果基本一致. 为了定量比较本文算法和码本算法的性能差异, 分别计算了图1中两帧图像的错误前景点率(FP rate)、正确前景点率(TP rate)和精度(Precision)[11-13], 各项指标计算方法如下:FP rate=, TP rate =, Precision=,(13)其中: fp表示错误前景点数; tp表示正确前景点数; fn表示错误背景点数; tn表示正确背景点数; (fp+tn)表示真实前景图像中的背景点总数; (tp+fn)表示真实前景图像中的前景点总数. 计算结果列于表1.表1 性能参数对比Table 1 Performance parameters comparison视频FP rate 码本算法本文算法TP rate码本算法本文算法Precision码本算法本文算法人物视频0.079 20.072 70.990 80.990 60.846 00.856 7车辆视频0.006 20.003 70.849 10.849 10.566 80.689 3由表1可见, 本文方法和Kim等[3]码本算法的检测结果存在一定的差异, 这是因为在码本算法中, 新像素与码本中各个码字进行匹配时, 只需找到第一个满足条件的码字即可, 并不需要遍历整个码本链表后找到最佳匹配的码字, 而各个码字之间存在交集是可能的. 排在前面的码字被匹配的机会大, 精简码本时, 留在码本背景模型中的机会也大;排在后面的码字被匹配的机会小, 所以更容易被当成冗余码字从码本中删除. 此外, 对匹配上的码字更新过程也会使码字表示的范围发生改变. 因为本文方法不为训练后半阶段出现的新像素建立码字, 并及时删除冗余码字, 所以“准冗余码字”在训练阶段不会参与匹配, 给码本中其他码字更多匹配和更新的机会.3.2 处理时间的对比针对样本视频分别计算应用本文方法和码本方法平均每帧的处理时间, 结果列于表2.表2 处理时间的对比(ms)Table 2 Processing time comparison(ms)视频训练阶段码本算法本文算法检测阶段码本算法本文算法人物视频22.752 921.521 525.883 225.189 9车辆视频18.578 317.695 921.585 319.622 0由表2可见, 本文方法的处理时间较少.3.3 存储空间的对比因为本文对码字结构改进的目的是减少码本模型所占用的内存空间, 所以分别测试了本文算法和码本算法应用在所选视频上时, 模型所占用内存的情况, 结果列于表3. 表3 内存的对比(Kb)Table 3 Memory comparison(Kb)视频码本算法本文算法人物视频4 6424 180车辆视频3 1242 812由表3可见, 改进后码本模型所占用的内存空间约减少了1/9. 实验中按浮点型占用4个字节, 整型占用2个字节计算, 导致内存使用量改变的原因是:码本算法每个码字包括5个浮点型数据和4个整型数据(f,λ,p,q), 平均每个像素处的码本包括4个码字, 所以模型所占用的空间是112个字节[4]. 本文算法的码字结构相比于Kim等[3]提出的码本算法节省了一个整型数据的空间, 每个码字所占用的空间是104个字节.综上所述, 本文改进了码本结构, 提出了一种减小码本模型所需要内存开销的方法. 该方法具有广泛的实用性, 可作为有关码本模型各种算法的补充, 在不影响其背景建模结果的前提下, 减少了内存需求.参考文献【相关文献】[1] ZHANG Jun, DAI Ke-xue, LI Guo-hui. HSV Color-Space and Codebook Model Based Moving Objects Detection [J]. Systems Engineering and Electronics, 2008, 30(3): 423-427. (张军, 代科学, 李国辉. 基于HSV颜色空间和码本模型的运动目标检测 [J]. 系统工程与电子技术, 2008, 30(3): 423-427.)[2] Chalidabhongse T H, Kim K, Harwood D, et al. A Perturbation Method for Evaluating Background Subtraction Algorithms [C]//Joint IEEE International Workshop on Visual Surveillance and Performance Evaluation of Tracking and Surveillance. Nice, France: [s.n.], 2003, 10: 11-12.[3] Kim K, Chalidabhongse T H, Harwood D, et al. Background Modeling and Subtraction by Codebook Construction [C]//2004 International Conference on Image Processing. New York: IEEE Press, 2004: 3061-3064.[4] Kim K, Chalidabhongse T H, Harwood D, et al. Real-Time Foreground-Background Segmentation Using Codebook Model [J]. Real-Time Imaging, 2005, 11(3): 172-185. [5] WU Ming-jun, PENG Xian-rong. Spatio-Temporal Context for Codebook-Based Dynamic Background Subtraction [J]. AEU-International Journal of Electronics and Communications, 2010, 64(8): 739-747.[6] Doshi A, Trivedi M. “Hybrid Cone-Cylinder” Codebook Model for Foreground Detection with Shadow and Highlight Suppression [C]//Proc IEEE International Conference on Video and Signal Based Surveillance. Washington DC: IEEE Computer Society, 2006: 19.[7] LI Qi, SHAO Chun-fu, YUE Hao, et al. Real-Time Foreground-Background Segmentation Based on Improved Codebook Model [C]//2010 3rd International Congress on Image and Signal Processing. Yantai: IEEE Xplore, 2010: 269-273.[8] GUO Jing-ming, HSO Chih-sheng. Cascaded Background Subtraction Using Block-Based and Pixel-Based Codebooks [C]//2010 International Conference on Pattern Recognition. Washington DC: IEEE Computer Society, 2010: 1373-1376.[9] ZHANG Zhao-hui, CHEN Rui-qing, LU Han-qing, et al. Moving Foreground Detection Based on Modified Codebook [C]//2009 2nd International Congress on Image and Signal Processing. Washington DC: IEEE Computer Society, 2009: 1-5.[10] TU Qiu, XU Yi-ping, ZHOU Man-li. Box-Based Codebook Model for Real-Time Objects Detection [C]//7th World Congress on Intelligent Control and Automation. Washington DC: IEEE Computer Society, 2008: 7621-7625.[11] Maddalena L, Petrosino A. A Self-organizing Approach to Background Subtraction for Visual Surveillance Applications [J]. IEEE Transaction on Image Processing, 2008, 17(7): 1168-1177.[12] LIU Yang-yang, SHEN Xuan-jing, WANG Yi-qi, et al. Design and Implementation of Embedded Intelligent Monitor System Based on ARM [J]. Journal of Jilin University: Information Science Edition, 2011, 29(2): 158-163. (刘阳阳, 申铉京, 王一棋, 等. 基于ARM的智能监控系统的设计与实现 [J]. 吉林大学学报: 信息科学版, 2011, 29(2): 158-163.)[13] DING Ying, LI Wen-hui, FAN Jing-tao, et al. Fuzzy Integral Feature Based Algorithm for Moving Infrared Object Detection [J]. Journal of Jilin University: Engineering and Technology Edition, 2010, 40(5): 1330-1335. (丁莹, 李文辉, 范静涛, 等. 基于模糊积分特征的红外图像运动目标检测算法 [J]. 吉林大学学报: 工学版, 2010, 40(5): 1330-1335.)。
车牌识别外文翻译

中英文翻译A configurable method for multi-style license platerecognitionAutomatic license plate recognition (LPR) has been a practical technique in the past decades. Numerous applications, such as automatic toll collection, criminal pursuit and traffic law enforcement , have been benefited from it . Although some novel techniques, for example RFID (radio frequency identification), WSN (wireless sensor network), etc., have been proposed for car ID identification, LPR on image data is still an indispensable technique in current intelligent transportation systems for its convenience and low cost. LPR is generally divided into three steps: license plate detection, character segmentation and character recognition. The detection step roughly classifies LP and non-LP regions, the segmentation step separates the symbols/characters from each other in one LP so that only accurate outline of each image block of characters is left for the recognition, and the recognition step finally converts greylevel image block into characters/symbols by predefined recognition models. Although LPR technique has a long research history, it is still driven forward by various arising demands, the most frequent one of which is the variation of LP styles, for example:(1) Appearance variation caused by the change of image capturingconditions.(2)Style variation from one nation to another.(3)Style variation when the government releases new LP format. Wesummed them up into four factors, namely rotation angle,line number, character type and format, after comprehensive analyses of multi-style LP characteristics on real data. Generally speaking, any change of the above four factors can result in the change of LP style or appearance and then affect the detection, segmentation or recognition algorithms. If one LP has a large rotation angle, the segmentation and recognition algorithms for horizontal LP may not work. If there are more than one character lines in one LP, additional line separation algorithm is needed before a segmentation process. With the variation of character types when we apply the method from one nation to another, the ability to re-define the recognition models is needed. What is more, the change of LP styles requires the method to adjust by itself so that the segmented and recognized character candidates can match best with an LP format.Several methods have been proposed for multi-national LPs or multiformat LPs in the past years while few of them comprehensively address the style adaptation problem in terms of the abovementioned factors. Some of them only claim the ability of processing multinational LPs by redefining the detection and segmentation rules or recognition models.In this paper, we propose a configurable LPR method which is adaptable from one style to another, particularly from one nation to another, by defining the four factors as parameters.1Users can constrain the scope of a parameter and at the same time the method will adjust itself so that the recognition can be faster and more accurate. Similar to existing LPR techniques, we also provide details of detection, segmentation and recognition algorithms. The difference is that we emphasize on the configurable framework for LPR and the extensibility of the proposed method for multistyle LPs instead of the performance of each algorithm.In the past decades, many methods have been proposed for LPR that contains detection, segmentation and recognition algorithms. In the following paragraphs, these algorithms and LPR methods based on them are briefly reviewed.LP detection algorithms can be mainly classified into three classes according to the features used, namely edgebased algorithms, colorbased algorithms and texture-based algorithms. The most commonly used method for LP detection is certainly the combinations of edge detection and mathematical morphology .In these methods, gradient (edges) is first extracted from the image and then a spatial analysis by morphology is applied to connect the edges into LP regions. Another way is counting edges on the image rows to find out regions of dense edges or to describe the dense edges in LP regions by a Hough transformation .Edge analysis is the most straightforward method with low computation complexity and good extensibility. Compared with edgebased algorithms, colorbased algorithms depend more on the application conditions. Since LPs in a nation often have several2predefined colors, researchers have defined color models to segment region of interests as the LP regions .This kind of method can be affected a lot by lighting conditions. To win both high recall and low false positive rates, texture classification has been used for LP detection. In Ref.Kim et al. used an SVM to train texture classifiers to detect image block that contains LP pixels.In Ref. the authors used Gabor filters to extract texture features in multiscales and multiorientations to describe the texture properties of LP regions. In Ref. Zhang used X and Y derivative features,grey-value variance and Adaboost classifier to classify LP and non-LP regions in an image.In Refs. wavelet feature analysis is applied to identify LP regions. Despite the good performance of these methods the computation complexity will limit their usability. In addition, texture-based algorithms may be affected by multi-lingual factors.Multi-line LP segmentation algorithms can also be classified into three classes, namely algorithms based on projection,binarization and global optimization. In the projection algorithms, gradient or color projection on vertical orientation will be calculated at first. The “valleys”on the projection result are regarded as the space between characters and used to segment characters from each other.Segmented regions are further processed by vertical projection to obtain precise bounding boxes of the LP characters. Since simple segmentation methods are easily affected by the rotation of LP, segmenting the skewed LP becomes a key issue to be solved. In the binarization algorithms, global or local methods are often used3to obtain foreground from background and then region connection operation is used to obtain character regions. In the most recent work, local threshold determination and slide window technique are developed to improve the segmentation performance. In the global optimization algorithms, the goal is not to obtain good segmentation result for independent characters but to obtain a compromise of character spatial arrangement and single character recognition result. Hidden Markov chain has been used to formulate the dynamic segmentation of characters in LP. The advantage of the algorithm is that the global optimization will improve the robustness to noise. And the disadvantage is that precise format definition is necessary before a segmentation process.Character and symbol recognition algorithms in LPR can be categorized into learning-based ones and template matching ones. For the former one, artificial neural network (ANN) is the mostly used method since it is proved to be able to obtain very good recognition result given a large training set. An important factor in training an ANN recognition model for LP is to build reasonable network structure with good features. SVM-based method is also adopted in LPR to obtain good recognition performance with even few training samples. Recently, cascade classifier method is also used for LP recognition. Template matching is another widely used algorithm. Generally, researchers need to build template images by hand for the LP characters and symbols. They can assign larger weights for the important points, for example, the corner points, in the4template to emphasize the different characteristics of the characters. Invariance of feature points is also considered in the template matching method to improve the robustness. The disadvantage is that it is difficult to define new template by the users who have no professional knowledge on pattern recognition, which will restrict the application of the algorithm.Based on the abovementioned algorithms, lots of LPR methods have been developed. However, these methods aremainly developed for specific nation or special LP formats. In Ref. the authors focus on recognizing Greek LPs by proposing new segmentation and recognition algorithms. The characters on LPs are alphanumerics with several fixed formats. In Ref. Zhang et al. developed a learning-based method for LP detection and character recognition. Their method is mainly for LPs of Korean styles. In Ref. optical character recognition (OCR) technique are integrated into LPR to develop general LPR method, while the performance of OCR may drop when facing LPs of poor image quality since it is difficult to discriminate real character from candidates without format supervision. This method can only select candidates of best recognition results as LP characters without recovery process. Wang et al. developed a method to recognize LPR with various viewing angles. Skew factor is considered in their method. In Ref. the authors proposed an automatic LPR method which can treat the cases of changes of illumination, vehicle speed, routes and backgrounds, which was realized by developing new detection and segmentation algorithms with robustness to the5illumination and image blurring. The performance of the method is encouraging while the authors do not present the recognition result in multination or multistyle conditions. In Ref. the authors propose an LPR method in multinational environment with character segmentation and format independent recognition. Since no recognition information is used in character segmentation, false segmented characters from background noise may be produced. What is more, the recognition method is not a learning-based method, which will limit its extensibility. In Ref. Mecocci et al. propose a generative recognition method. Generative models (GM) are proposed to produce many synthetic characters whose statistical variability is equivalent (for each class) to that showed by real samples. Thus a suitable statistical description of a large set of characters can be obtained by using only a limited set of images. As a result, the extension ability of character recognition is improved. This method mainly concerns the character recognition extensibility instead of whole LPR method.From the review we can see that LPR method in multistyle LPR with multinational application is not fully considered. Lots of existing LPR methods can work very well in a special application condition while the performance will drop sharply when they are extended from one condition to another, or from several styles to others.多类型车牌识别配置的方法自动车牌识别(LPR)在过去的几十年中的实用技术。
足球比赛视频中的目标检测与跟踪算法研究
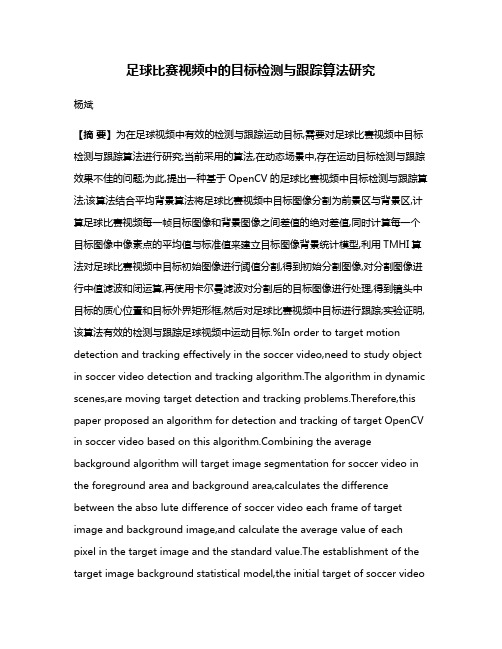
足球比赛视频中的目标检测与跟踪算法研究杨斌【摘要】为在足球视频中有效的检测与跟踪运动目标,需要对足球比赛视频中目标检测与跟踪算法进行研究;当前采用的算法,在动态场景中,存在运动目标检测与跟踪效果不佳的问题;为此,提出一种基于OpenCV的足球比赛视频中目标检测与跟踪算法;该算法结合平均背景算法将足球比赛视频中目标图像分割为前景区与背景区,计算足球比赛视频每一帧目标图像和背景图像之间差值的绝对差值,同时计算每一个目标图像中像素点的平均值与标准值来建立目标图像背景统计模型,利用TMHI算法对足球比赛视频中目标初始图像进行阈值分割,得到初始分割图像,对分割图像进行中值滤波和闭运算,再使用卡尔曼滤波对分割后的目标图像进行处理,得到镜头中目标的质心位置和目标外界矩形框,然后对足球比赛视频中目标进行跟踪;实验证明,该算法有效的检测与跟踪足球视频中运动目标.%In order to target motion detection and tracking effectively in the soccer video,need to study object in soccer video detection and tracking algorithm.The algorithm in dynamic scenes,are moving target detection and tracking problems.Therefore,this paper proposed an algorithm for detection and tracking of target OpenCV in soccer video based on this bining the average background algorithm will target image segmentation for soccer video in the foreground area and background area,calculates the difference between the abso lute difference of soccer video each frame of target image and background image,and calculate the average value of each pixel in the target image and the standard value.The establishment of the target image background statistical model,the initial target of soccer videoimage segmentation using TMHI algorithm to obtain the initial image segmentation,median filtering and closed operation of image segmentation,and then use the Calman filter to process the target image after segmentation,target centroid position and get outside rectangular shots target in the frame.Then the goal in soccer video tracking.The experimental results show that the moving target detection and tracking algorithm for soccer video effectively.【期刊名称】《计算机测量与控制》【年(卷),期】2017(025)009【总页数】4页(P266-268,306)【关键词】足球比赛;视频;目标检测;目标跟踪【作者】杨斌【作者单位】商洛学院,陕西商洛726000【正文语种】中文【中图分类】TP391近几年,随着科技的发展及人们对生活质量要求的提高,对视频目标检测与跟踪技术的需求越来越广泛[1]。
一种自适应运动目标检测算法及其应用

2021年2月第2期Vol. 42 No. 2 2021小型微型计算机系统Journal of Chinese Computer Systems一种自适应运动目标检测算法及其应用李善超,车国霖,张果,杨晓洪(昆明理工大学信息工程与自动化学院,昆明650500)E-mail :991186428@ qq. com摘要:针对ViBe 算法在动态背景下存在鬼影消除时间长、算法适应性差、前景检测噪声多的问题,本文提出一种基于ViBe 算法框架的改进算法.该算法釆用鬼影检测法标记第1帧中的鬼影区域,并向位于鬼影区域的背景模型中强制引入背景样本,从而快速抑制鬼影;在像素分类过程中,引入自适应分类阈值,解决全局阈值易受动态噪声干扰的问题;在背景模型更新中,根 据像素分类的匹配值来动态决定更新因子,提高算法适应场景变化的能力.定性与定量的对比实验结果表明,本文算法相较于ViBe 算法能够有效地检测动态背景下的运动目标,应用于河流漂浮物检测场景中也有较好的效果.关键词:ViBe ;动态背景;运动目标检测;自适应方法;河流漂浮物检测中图分类号:TP391文献标识码:A 文章编号:1000-1220(2021)02-0381-06Adaptive Moving Target Detection Algorithm and Its ApplicationLI Shan-chao ,CHE Guo-lin ,ZHANG Guo,YANG Xiao-hong(Faculty of Information Engineering and Automation ,Kunming University of Science and Technology ,Kunming 650500,China)Abstract : Aiming at the problem that ViBe algorithm has long ghost elimination time , poor algorithm adaptability and high foreground detection noise in dynamic background , this paper proposes an improved algorithm based on ViBe algorithm framework. The algorithmuses the ghost detection method to mark the ghost region in the first frame , and forces the background sample into the background model in the ghost region to quickly suppress the ghost. In the pixel classification process , the adaptive classification threshold is intro ・ duced to solve the problem that the global threshold is susceptible by dynamic noise interference. In the background model update , theupdate factor is dynamically determined according to the matching number of the pixel classification to improve the algorithm's abilityto adapt to scene changes. The comparison experimental results of qualitative and quantitative shows that the algorithm in this paper can effectively detect moving targets in dynamic background compared to the ViBe algorithm , and it also has a better effect in the de tection of river floating objects.Key words : ViBe ; dynamic background ; moving target detection ; adaptive method ; river floating debris1引言运动目标检测在智能视频监控的应用中扮演着重要的角 色,是计算机视觉领域的一个研究热点⑴•运动目标检测的 实质是在视频序列中定位运动中的目标,而准确的前景检测 是目标分类、目标跟踪和行为识别研究的重要基石⑺叫运动目标检测算法按类别可分为帧差法⑴、光流法⑷、背景建模 法"向3种.帧差法原理简单且易于设计,然而其检测结果存 在空洞和鬼影的问题.光流法虽然精度高,但由于其计算量大,不适用于对实时性有较高要求的场景.背景建模法是在初 始化过程中构建出由背景样本组成的模型,并将当前帧与背 景模型进行差分,从而对像素进行分类,最后得到运动目标.其具有精度高实时性好的特点.背景模型的准确性决定了背景建模法的检测精度,主要影响检测精度的因素有鬼影问题、 动态背景、噪声干扰等⑴.高斯混合模型(GMM ,Gaussian mixture model)[8]是运动目标检测算法中最为经典的算法,其本质是基于像素样本统 计信息的背景建模方法,能够对复杂背景进行准确建模,然而 其计算复杂度较高GMG 算法切是统计背景模型的概率,采 用贝叶斯逐像素分割,但在动态场景中其检测精确度较低.核 密度估计算法(KDE,Kernel Density Estimation)[10]是一种非 参数背景建模方法,其通过大量的背景样本估算背景像素的概率密度函数,从而根据像素背景概率来分类像素,然而其内 存占用与计算复杂度都较高.Bamich 等人⑴•切于2009年提出一种非参数化视频背景提取算法(ViBe , Visual BackgroundExtractor),该算法是为每个像素设置一个样本集,并与新帧像素进行阈值比较,从而对像素进行分类,其具有实时性好、鲁棒性高和易于集成于嵌入式设备的特点.然而ViBe 算法仍 存在一些不足,限制了其在动态场景中的应用.例如:1)当初 始化图像中存在运动中的目标时,ViBe 算法会在后续帧中检 测到鬼影,降低了算法的检测精度且鬼影消除时间长;2)ViBe 算法在动态场景中检测精度低,容易受动态噪声干扰;3) ViBe 算法的背景模型更新策略无法适应背景动态的变化.针对ViBe 算法存在的问题,本文提出一种自适应运动目收稿日^:2020-03-06 收修改稿日期:202045-11基金项目:国家重点研发计划项目(2017YFB0306405)资助;国家自然科学基金项目 (61364008)资助.作者简介:李善超,男,1994年生,硕士研究生,研究方向为数字图像处理;车国霖,男,1975年生,硕士,副教授,研究方向为 智能控制;张 果,男,1976年生,博士,副教授,研究方向为智能測控;杨晓洪,女,1964年生,高级工程师,研究方向为综合自动化.382小型微型计算机系统2021年标检测算法.本文将从以下3个方面对ViBe算法进行改进.1)采用鬼影检测法标记鬼影区域并强制引入背景样本,加速鬼影的抑制;2)采用自适应匹配阈值的方法进行像素分类,提高算法抗干扰的能力;3)根据像素分类的匹配值动态调整更新因子,提高算法适应场景变化的能力.本文采用CDNET 数据集中dynamicBackground视频类中的5个视频序列和3组河流漂浮物的视频序列进行研究,以本文算法和其他5种算法为例,定性、定量对实验结果做出质量评价和分析.研究结果表明,本文算法相较于ViBe算法在召回率、精确率和F 度量值方面均有提高,错误分类比更低,达到了预期的目标.2ViBe算法原理ViBe算法是基于样本随机聚类的背景建模算法,具有运算效率高、易于设计、易于集成嵌入设备的特点,能够实现快速的背景建模和运动目标检测.算法的步骤包括背景模型初始化、像素分类过程和背景模型更新.2.1背景模型初始化1)背景模型定义:ViBe算法的背景模型是由N个背景样本组成的,v(x)是像素x的像素值,则背景模型M(x)定义如公式(1)所示:=|Vj(x),v2(x),v N(x)|(1)2)背景模型初始化:ViBe算法利用视频序列第1帧建立背景模型,从第2帧开始算法就可以有效地检测运动目标.背景模型初始化是在像素x的8邻域Nc(x)中选取一个像素值作为背景样本,重复N次,如公式(2)所示:(N g(x)=Ui,¾,--,¾IJ(2)〔M(x)=1v(ylyeN c(x))I3)随机选取策略:背景建模时,背景样本始终采用随机选取邻域像素的策略,以使背景模型更加稳定可靠.2.2像素分类过程ViBe算法采用计算欧氏距离来进行像素的分类. S”(v(x))是以像素值v(x)作中心,匹配阈值R为半径的二维欧氏空间,若v(x))与M(x)的交集H{•}中元素个数不小于最小匹配数则认为像素x是背景像素,如公式(3)所示:H{Sx(v(x))n I V,(x),v2(x),—,v w(x)I I(3) 2.3背景模型更新1)保守更新机制:ViBe算法通过保守更新机制进行背景模型更新,即如果像素被分类为背景像素,则以i/e(e是更新因子)的概率替代背景模型中的任一样本.假设时间是连续且选择过程是无记忆性的,在任一dt时间后,背景模型的样本随时间变化的概率如公式(4)所示:P(t,t+dt)=e-1"(^)d,(4)公式(4)表明,背景模型样本值的预期剩余寿命都呈指数衰减,背景模型的样本更新与时间无关.2)随机更新机制:ViBe算法通过随机更新机制进行样本替换,使得每个样本的存在时间成平滑指数衰减,提高了算法适应背景变化的能力,避免了旧像素长期不更新带来的模型劣化的问题.3)空间传播机制:ViBe算法也将背景像素引入邻域的背景模型中,保证了邻域像素空间的一致性.例如,用背景像素替换任一邻域(x)中的任一样本.ViBe算法首次将随机聚类技术应用于运动目标检测中,使得算法在背景模型初始化、像素分类过程、背景模型更新3个方面都比较简单,保证了算法的实时性,因此ViBe算法被广泛应用于现实生活中3提出的改进算法ViBe算法采用随机采样、非参数化和无记忆的更新策略,使得其具有较好的性能,但其在动态场景下仍然存在不能快速抑制鬼影、难以消除动态噪声以及无法适应场景动态变化的问题,本文将从以下3个方面对ViBe算法进行改进.3.1鬼影检测ViBe算法利用第1帧建立背景模型,但也不可避免的将第1帧中存在的运动目标前景像素引入到背景模型中,导致鬼影问题和彫响算法的检测精度.假设背景模型M(x)是由第1帧中的前景像素样本f(x)组成的,当运动目标离开时,ZU)不在背景像素值b(x)的S”(b(x))圆内,背景像素被错误的分类为前景,如公式(5)所示,则在第1帧中运动目标所在的区域就会出现虚拟的前景(鬼影).rM(x)=|/;(x)J2(x),―J N(x)}(s&(x))nM(x)=0本文针对这一问题,应用鬼影检测法标记出第1帧中的鬼影区域,并向位于鬼影区域的背景模型中强制引入背景样本,减少其中前景像素的数量,从而快速抑制鬼影.鬼影检测法借鉴了帧间差分法并对其进行改进,其原理是提取视频序列的前3帧图像,第1帧图像分别与后两帧图像做差分运算,设定差分阈值并对差分后的图像进行二值化分类,将二值化结果做逻辑或操作和形态学操作,即得到标记有第1帧运动目标的鬼影模板Ghost(x),在鬼影模板Ghost(x)中大于0的位置是第1帧中鬼影区域的.具体定义如公式(6)、公式(7)和公式(8)所示:if I厶(x)-厶+|(兀)I>Tif\IM一人+|(x)lwTM)={o-/“2(x)IWTGhost(x)=£>i(x)or D2(x)(6)(7)(8)式中:D(x)为二值化图像,人(x)为第R帧输入图像,一般A=1为图像差分阈值,。
codebook

Real-time foreground–background segmentationusing codebook model 2005Codebook是无参数背景检测算法的经典算法之一,本文虽然不是最新最前沿的论文,但是本文较为全面的介绍了codebook背景检测算法,之后的各种无参数的算法很多借鉴了codebook,在其之上做了很多的改进形成新的检测技术体系。
对于参数背景检测模型大多都是基于概率分布,如混合高斯模型(MOG)这里不再过多介绍,主要介绍一下codebook的背景建模及其分类条件。
背景模型建立:codebook算法是基于RGB彩色图像设计,对于灰度图像做一些细节修改同样适用。
对于一个视频序列,每个像素都有N个RGB分量:,我们定义代表codeword分量,每个像素都含有L个codeword分量,对于不同的训练样本,像素所包含的codeword分量不同。
每个codeword又包含如下变量:一个RGB变量容器,还有一个6元组的向量AUXi向量每个属性的定义如下::代表亮度所允许的最大和最小值,被分别分配给所有像素的codebook。
:代表每个codebook出现的频率(checked):在一段训练时间内,此codebook没有被重新checked的时间间隔。
:带便此codebook被第一次与最近一次被checked的时间。
背景模型的建立的算法:首先设,L为0,后面为空集Xt为当先时间的像素的RGB,当前光照强度定义为:从像素的codebook中选取合适的codeword,选取的codeword需要满足下面两个条件:a:当前时刻的颜色与codeword中的Vi之间的色差小于。
b:当前的光照强度在允许的光照强度范围内。
如果没有合适的codeword,那么在像素的codebook添加一个新的codeword,参数如下:然后更新被选择的codeword:。
背景检测条件:1-对于一些(K)个codeword。
- 1、下载文档前请自行甄别文档内容的完整性,平台不提供额外的编辑、内容补充、找答案等附加服务。
- 2、"仅部分预览"的文档,不可在线预览部分如存在完整性等问题,可反馈申请退款(可完整预览的文档不适用该条件!)。
- 3、如文档侵犯您的权益,请联系客服反馈,我们会尽快为您处理(人工客服工作时间:9:00-18:30)。
min E D =
( x , y )∈R
∑
ρ (uf x + vf y + f t , σ ) ,
(3)
here the ρ - function is chosen as the Geman-McClure function (Black, x2 x2 + σ 2
f (x, t + 1) = f ( x − u( x; a), t ), 1 where f(x, t) is the brightness function in time instant t, x = ( x, y ) is coordinate of the image pixel, and u( x; a) is the motion vector. We assume the affine flow model (6 parameters) for the dominant object u ( x, y ) a0 + a1 x + a 2 y u( x; a) = = v ( x , y ) a 3 + a 4 x + a5 y
,
4
with σ as the scale parameter, and f x , f y , f t as partial derivatives of brightness function with respect to x, y and t. The SOR iteration update equations are
2. Background
The dominant motion model-based method used for segmention, compared to the multiple model competition method, is more efficient because it does not need to consider how many objects occur in the scene and looks simpler from its algorithmic form. It is valid for some application fields, for example, background/foreground segmentation. In the use of dominant motion model, one of the key steps is determination of the dominant object. It is a region or object corresponding to the dominant motion. (Black, 1996) put forward a dominant motion estimation method in a simulated annealing framework, but it cannot give a clean region segmentation
Background-Foreground Segmentation Based on Dominant Motion Estimation and Static Segmentation
Yu Huang, Dietrich Paulus, Heinrich Niemann Chair for Pattern Recognition, Dept. of Computer Science University of Erlangen-Nürnberg, 91058, Erlangen, Germany E-mail: YuHuang@rmatik.uni-erlangen.de
Abstract: This paper addresses the problem of image segmentation using motion and luminance
information. We use the dominant motion model to calculate both the background and foreground motion in a robust estimation framework and then combine it with the result of static segmentation using the watershed algorithm to segment the foreground from the background. In this paper, the previous pixelbased (or over a small neighborhood) motion measure is replaced by the patch-based motion measure in motion segmentation. Experimental results are given to show the efficiency of our method. Key words: Dominant motion, robust estimation, static segmentation, watershed.
3. Patch-based dominant motion segmentation
In this paper we combine the static segmentation with the dominant motion model. Here oversegmentation is needed in the process of static segmentation in order to make pixels in each subregion having the similar motion. There are some current methods available for this task, for example, the watershed algorithm, the pixel-based region growing and the quadtree split-merge method etc. In this paper, we choose the watershed algorithm. Based on the static segmentation result, we replace the pixel-based motion measure with the proposed patch or region-based motion measure to make a clear segmentation of the dominant motion region. 3.1 The SOR method for dominant motion estimation Before we present our approach, the Simultaneous-Over-Relaxation (SOR) method (Black, 1996) for dominant motion estimation is described simply. First, the interframe motion is defined as
1. Introduction
The segmentation of image sequences into regions or `objects´ has received a large attention in recent years. Applications like object tracking, video coding and structure from motion can benefit from a meaningful segmentation. But it is by now not solved being a chicken-and-egg problem. The methods of motion segmentation can be grouped into two broad classes (Sawhney, 1996). One class solves the problem by letting multiple models simultaneously compete for the description of the individual motion measurements (Wang, 1994), and the another one excavates out the multiple models sequentially by solving for a dominant model (Irani, 1994). For the former method, its difficulties occur at determination of the number of models or uncertainty of mixture models. The latter one may confront puzzles in the case of absence of dominant motion, and it yet lacks competition amongst the motion models. In this paper, we discuss the dominant motion based method used for background and foreground segmentation. In Sect. 2, we present related works and background. In Sect. 3, the dominant motion estimation method described in (Black, 1996) is outlined, and its combination with static segmentation using the watershed algorithm is presented. Finally experimental results are reported in Sect. 4 and concluding remarks are given in Sect. 5.