Hypergraph-Regularized Sparse NMF
基于同质性降维和CMP算法的高光谱图像分类

基于同质性降维和CMP算法的高光谱图像分类晁拴社;楚恒【摘要】According to the fact that high-dimensionality and redundant information between bands of hyperspectral data, the paper proposes a new classification method based on homogeneity dimensionality reduction and Combined Matching Pursuit(CMP)algorithm. Firstly, the proposed method segments hyperspectral image by mean shift algorithm and obtains homogeneous blocks, and builds dimensionality reduction mapping function of the image block by manifold learning. And then the method trains sparse least squares SVM for low-dimensional data, but the method proposes a new sparse reconstruction method based on CMP algorithm for solving multiple iterations of Orthogonal MatchingPursuit(OMP) algorithm. By classification results of hyperspectral data can be drawn that the proposed method effectively improves the hyperspectral image classification accuracy.%针对高光谱数据维数高,波段间冗余信息大的问题,提出一种基于同质性降维和组合匹配追踪算法的高光谱图像分类方法.该方法首先利用均值漂移算法对高光谱图像进行分割得到同质性图像块,对同质性的图像块进行流行学习得到降维映射函数,然后由降维后的高光谱数据训练稀疏最小二乘支持向量机分类模型,为避免正交匹配追踪稀疏重构算法迭代次数多的缺点,提出一种基于组合匹配追踪的稀疏重构求解方法.通过高光谱数据的分类结果可以得出,该方法有效提高了高光谱图像的分类精度.【期刊名称】《计算机工程与应用》【年(卷),期】2017(053)013【总页数】5页(P206-210)【关键词】高光谱图像分类;同质性降维;稀疏最小二乘支持向量机;组合匹配追踪算法【作者】晁拴社;楚恒【作者单位】重庆邮电大学通信与信息工程学院,重庆 400065;重庆邮电大学通信与信息工程学院,重庆 400065;西南大学地理科学学院,重庆 400715【正文语种】中文【中图分类】TP391高光谱图像由成百上千个连续的窄波段形成,覆盖可见光到近红外区域,光谱分辨率高,可以进行地物间的精细分类,已经被应用在矿物勘测、精细农业、医学检验等民用领域和军事侦察、战场地图绘制等军事领域[1-2]。
改进阈值函数的指纹图像去噪方法
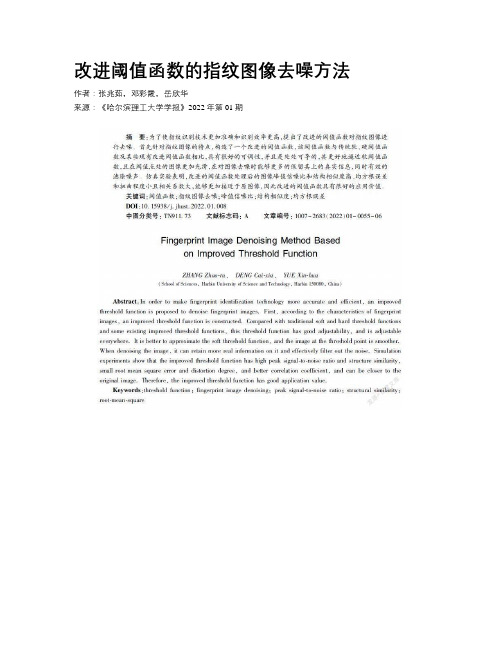
改进阈值函数的指纹图像去噪方法作者:张兆茹,邓彩霞,岳欣华来源:《哈尔滨理工大学学报》2022年第01期摘要:为了使指纹识别技术更加准确和识别效率更高,提出了改进的阈值函数对指纹图像进行去噪。
首先针对指纹图像的特点,构造了一个改进的阈值函数,该阈值函数与传统软、硬阈值函数及某些现有改进阈值函数相比,具有很好的可调性,并且是处处可导的,其更好地逼近软阈值函数,且在阈值点处的图像更加光滑,在对图像去噪时能够更多的保留其上的真实信息,同时有效的滤除噪声。
仿真实验表明,改进的阈值函數处理后的图像峰值信噪比和结构相似度高、均方根误差和扭曲程度小且相关系数大,能够更加接近于原图像,因此改进的阈值函数具有很好的应用价值。
关键词:阈值函数;指纹图像去噪;峰值信噪比;结构相似度;均方根误差DOI:10.15938/j.jhust.2022.01.008中图分类号: TN911.73 文献标志码: A 文章编号: 1007-2683(2022)01-0055-06Fingerprint Image Denoising Method Basedon Improved Threshold FunctionZHANG Zhaoru,DENG Caixia,YUE Xinhua(School of Sciences, Harbin University of Science and Technology, Harbin 150080,China)Abstract:In order to make fingerprint identification technology more accurate and efficient,an improved threshold function is proposed to denoise fingerprint images. First, according to the characteristics of fingerprint images, an improved threshold function is constructed. Compared with traditional soft and hard threshold functions and some existing improved threshold functions, this threshold function has good adjustability, and is adjustable everywhere. It is better to approximate the soft threshold function, and the image at the threshold point is smoother. When denoising the image, it can retain more real information on it and effectively filter out the noise. Simulation experiments show that the improved threshold function has high peak signaltonoise ratio and structure similarity, small root mean square error and distortion degree, and better correlation coefficient,and can be closer to the original image. Therefore, the improved threshold function has good application value.Keywords:threshold function; fingerprint image denoising; peak signaltonoise ratio; structural similarity; rootmeansquare0引言指纹图像以其具有唯一性、稳定性、可采集性、与主体永可分离性等优点[1-2],已成为身份识别的有效手段,被广泛应用于刑事侦查和安全验证中。
应用非负矩阵分解和RBPNN模型的掌纹识别方法
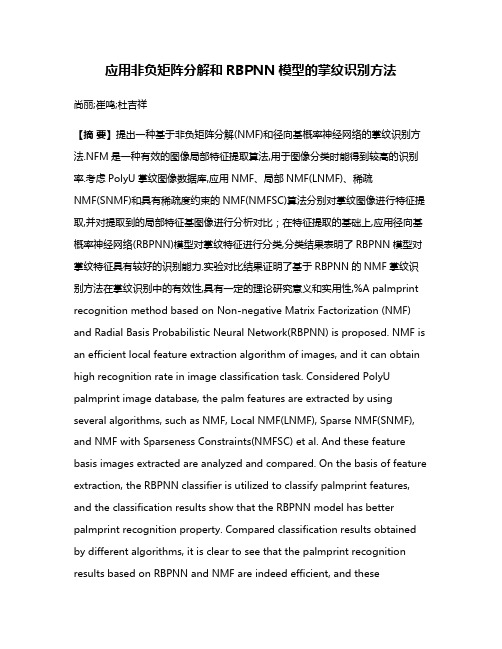
应用非负矩阵分解和RBPNN模型的掌纹识别方法尚丽;崔鸣;杜吉祥【摘要】提出一种基于非负矩阵分解(NMF)和径向基概率神经网络的掌纹识别方法.NFM是一种有效的图像局部特征提取算法,用于图像分类时能得到较高的识别率.考虑PolyU掌纹图像数据库,应用NMF、局部NMF(LNMF)、稀疏NMF(SNMF)和具有稀疏度约束的NMF(NMFSC)算法分别对掌纹图像进行特征提取,并对提取到的局部特征基图像进行分析对比;在特征提取的基础上,应用径向基概率神经网络(RBPNN)模型对掌纹特征进行分类,分类结果表明了RBPNN模型对掌纹特征具有较好的识别能力.实验对比结果证明了基于RBPNN的NMF掌纹识别方法在掌纹识别中的有效性,具有一定的理论研究意义和实用性,%A palmprint recognition method based on Non-negative Matrix Factorization (NMF) and Radial Basis Probabilistic Neural Network(RBPNN) is proposed. NMF is an efficient local feature extraction algorithm of images, and it can obtain high recognition rate in image classification task. Considered PolyU palmprint image database, the palm features are extracted by using several algorithms, such as NMF, Local NMF(LNMF), Sparse NMF(SNMF), and NMF with Sparseness Constraints(NMFSC) et al. And these feature basis images extracted are analyzed and compared. On the basis of feature extraction, the RBPNN classifier is utilized to classify palmprint features, and the classification results show that the RBPNN model has better palmprint recognition property. Compared classification results obtained by different algorithms, it is clear to see that the palmprint recognition results based on RBPNN and NMF are indeed efficient, and thesealgorithms behave certain theory research meaning and application in practice.【期刊名称】《计算机工程与应用》【年(卷),期】2012(048)004【总页数】5页(P199-203)【关键词】非负矩阵分解;局部特征提取;特征基图像;掌纹识别;径向基概率神经网络(RBPNN)分类器【作者】尚丽;崔鸣;杜吉祥【作者单位】苏州市职业大学电子信息工程系,江苏苏州215104;苏州市职业大学电子信息工程系,江苏苏州215104;中国科学技术大学自动化系,合肥230026;华侨大学计算机科学与技术系,福建泉州362021【正文语种】中文【中图分类】TN911.73图像特征的选择将左右图像识别的结果,能否在高维的原始数据空间内恰当有效地进行特征的提取和选择关系到下一步的分类决策操作,因此特征提取在模式识别中一直占据着重要的地位。
hypermesh生成柔性体mnf文件[新版]
![hypermesh生成柔性体mnf文件[新版]](https://img.taocdn.com/s3/m/3449c9362e60ddccda38376baf1ffc4ffe47e27f.png)
Hypernesh生成柔性体MNF文件这里有详细的教程。
本文旨在step by step演示操作。
1选择optistruct模板2导入几何3划分网格4定义材料材料定义非常关键,以steel为例,弹性模量输入2.1e5,泊松比取0.3,密度为7.9e-6.(如果按照t mm s单位制,密度应为7.9e-9,或者按照kg mm s单位制,弹性模量应为2.1e8,但是此处必须这样定义,否则将出现后文总结的错误。
5定义单元属性6属性赋予单元7创建rigid单元8创建load collector1Card选cmsmeth(模态综合法)卡片属性method选CB(约束模态)nmodes自己定。
附9创建load collector210约束两个中心节点注意loadtype选ASET11创建loadstepsType选generic(针对hm10版本),其他选项不需要定义。
12 control cards(1) DTI UNITS(2) GLOBAL_CASE_CONTROL点击黄色区域选择第8步建立的cms。
(3)GPSTRESS(4) OUTPUT选择adamsmnf。
其他文件按照需要也可输出。
13 提交optistruct或者radioss计算。
得到mnf文件14用adams打开第7阶频率54.035Hz(去掉前6阶刚体模态)。
用单纯的模态分析对比可知模态基本一致。
查看质量信息等。
质量为0.52kg,与实际相符。
总结:材料属性的定义非常关键,否则会出现质量正确,但频率相差1000开根号倍(31.62倍);或者频率正确但质量相差1000倍的情况。
NSST域改进NMF的红外与可见光图像融合
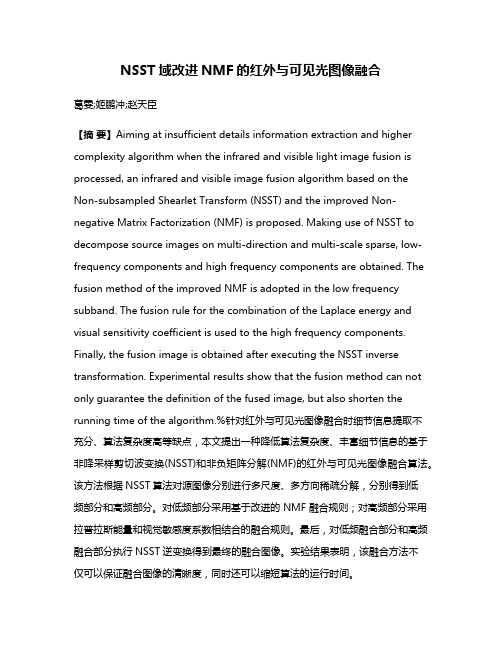
NSST域改进NMF的红外与可见光图像融合葛雯;姬鹏冲;赵天臣【摘要】Aiming at insufficient details information extraction and higher complexity algorithm when the infrared and visible light image fusion is processed, an infrared and visible image fusion algorithm based on the Non-subsampled Shearlet Transform (NSST) and the improved Non-negative Matrix Factorization (NMF) is proposed. Making use of NSST to decompose source images on multi-direction and multi-scale sparse, low-frequency components and high frequency components are obtained. The fusion method of the improved NMF is adopted in the low frequency subband. The fusion rule for the combination of the Laplace energy and visual sensitivity coefficient is used to the high frequency components. Finally, the fusion image is obtained after executing the NSST inverse transformation. Experimental results show that the fusion method can not only guarantee the definition of the fused image, but also shorten the running time of the algorithm.%针对红外与可见光图像融合时细节信息提取不充分、算法复杂度高等缺点,本文提出一种降低算法复杂度、丰富细节信息的基于非降采样剪切波变换(NSST)和非负矩阵分解(NMF)的红外与可见光图像融合算法。
基于多流形正则化非负矩阵分解的多视图聚类的开题报告
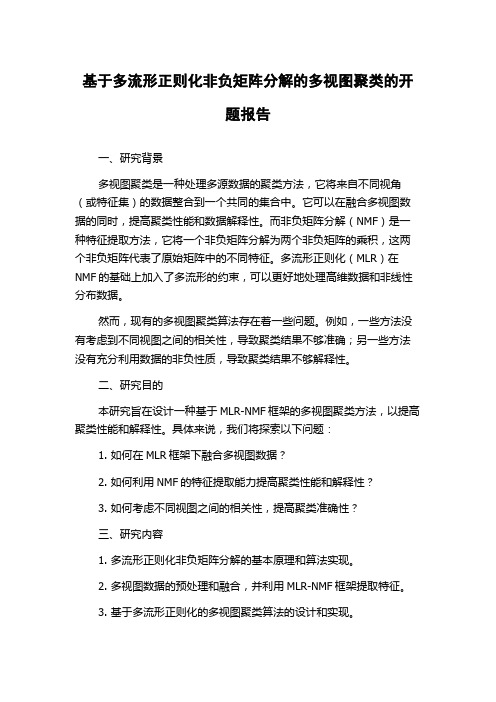
基于多流形正则化非负矩阵分解的多视图聚类的开题报告一、研究背景多视图聚类是一种处理多源数据的聚类方法,它将来自不同视角(或特征集)的数据整合到一个共同的集合中。
它可以在融合多视图数据的同时,提高聚类性能和数据解释性。
而非负矩阵分解(NMF)是一种特征提取方法,它将一个非负矩阵分解为两个非负矩阵的乘积,这两个非负矩阵代表了原始矩阵中的不同特征。
多流形正则化(MLR)在NMF的基础上加入了多流形的约束,可以更好地处理高维数据和非线性分布数据。
然而,现有的多视图聚类算法存在着一些问题。
例如,一些方法没有考虑到不同视图之间的相关性,导致聚类结果不够准确;另一些方法没有充分利用数据的非负性质,导致聚类结果不够解释性。
二、研究目的本研究旨在设计一种基于MLR-NMF框架的多视图聚类方法,以提高聚类性能和解释性。
具体来说,我们将探索以下问题:1. 如何在MLR框架下融合多视图数据?2. 如何利用NMF的特征提取能力提高聚类性能和解释性?3. 如何考虑不同视图之间的相关性,提高聚类准确性?三、研究内容1. 多流形正则化非负矩阵分解的基本原理和算法实现。
2. 多视图数据的预处理和融合,并利用MLR-NMF框架提取特征。
3. 基于多流形正则化的多视图聚类算法的设计和实现。
4. 实验验证和性能评估,与现有方法进行比较。
四、研究意义本研究将探索一种新的多视图聚类算法,具有以下优点:1. 充分利用NMF的特征提取能力,提高聚类性能和解释性。
2. 利用MLR框架,在保留数据非线性分布的同时,考虑不同视图之间的相关性,提高聚类准确性。
3. 通过实验证明,本方法在多个数据集上取得了较好的聚类结果,说明其具有很好的应用前景。
五、研究方法1. 文献综述,并分析现有多视图聚类方法的优缺点。
2. 提出基于MLR-NMF框架的多视图聚类方法,并设计实验验证其性能。
3. 实现算法,并使用多个公共数据集进行性能测试。
4. 分析实验结果,与现有方法进行比较,验证本方法的有效性。
nmfe算法 rna折叠最小自由能 -回复

nmfe算法rna折叠最小自由能-回复【NMFE算法RNA折叠最小自由能】RNA(核糖核酸)是一类生物分子,其序列中的碱基决定了其结构和功能。
折叠是指RNA分子通过碱基之间的互补配对形成特定的结构,进而发挥特定的功能。
RNA分子的折叠状态对于生物体的正常生理过程起着关键作用。
RNA折叠的问题可以形式化为在给定RNA序列的条件下,如何找到能量最小的折叠构象。
这是一个计算复杂度很高的问题,需要应用数学和计算机科学的技术。
近年来,科学家们提出了多种算法用于求解RNA折叠最小自由能的问题。
其中一种重要的算法是NMFE(Nucleotide Mutational Folding Evaluation)算法。
以下是NMFE算法的一步一步介绍:第一步:选择RNA序列作为输入,我们需要选择一个特定的RNA序列作为算法的初始输入。
RNA 序列是由四种不同的核苷酸(腺嘌呤A、尿嘧啶U、鸟嘌呤G和胞嘧啶C)组成的。
第二步:建立目标函数在NMFE算法中,我们需要定义一个目标函数来刻画给定的折叠结构的自由能。
自由能是描述了系统处于某个状态的稳定程度的物理量,其数值越小,表示该状态越稳定。
第三步:构建动态规划模型NMFE算法通过动态规划的方式来求解RNA折叠最小自由能问题。
动态规划是一种将复杂问题分解为相对简单的子问题并重复利用子问题解的方法。
在NMFE算法中,我们采用了自底向上的策略来构建动态规划模型。
具体地,我们从RNA序列的末端开始,逐渐向前计算,直到获得整个序列的最优折叠结构。
第四步:计算最小自由能在动态规划模型中,我们需要定义一系列的状态和转移方程来计算每一步的最小自由能。
具体来说,我们可以定义一个二维数组,每个元素代表了从第i个碱基到第j个碱基之间的最小自由能。
通过填充这个数组,我们可以递推地计算每一个子问题的最小自由能。
第五步:回溯获得最优解在计算得到最小自由能的同时,我们还需要记录每一步的选择,以便于最后回溯获得最优解的折叠结构。
肉骨粉显微近红外标准光谱库的快速构建方法
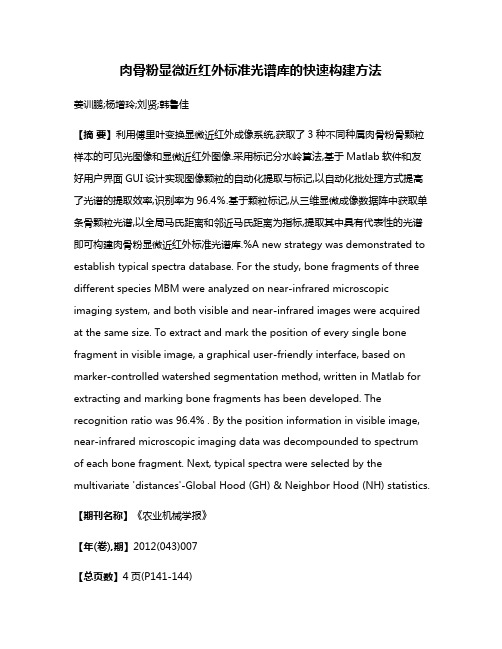
肉骨粉显微近红外标准光谱库的快速构建方法姜训鹏;杨增玲;刘贤;韩鲁佳【摘要】利用傅里叶变换显微近红外成像系统,获取了3种不同种属肉骨粉骨颗粒样本的可见光图像和显微近红外图像.采用标记分水岭算法,基于Matlab软件和友好用户界面GUI设计实现图像颗粒的自动化提取与标记,以自动化批处理方式提高了光谱的提取效率,识别率为96.4%.基于颗粒标记,从三维显微成像数据阵中获取单条骨颗粒光谱,以全局马氏距离和邻近马氏距离为指标,提取其中具有代表性的光谱即可构建肉骨粉显微近红外标准光谱库.%A new strategy was demonstrated to establish typical spectra database. For the study, bone fragments of three different species MBM were analyzed on near-infrared microscopic imaging system, and both visible and near-infrared images were acquired at the same size. To extract and mark the position of every single bone fragment in visible image, a graphical user-friendly interface, based on marker-controlled watershed segmentation method, written in Matlab for extracting and marking bone fragments has been developed. The recognition ratio was 96.4% . By the position information in visible image, near-infrared microscopic imaging data was decompounded to spectrum of each bone fragment. Next, typical spectra were selected by the multivariate 'distances'-Global Hood (GH) & Neighbor Hood (NH) statistics.【期刊名称】《农业机械学报》【年(卷),期】2012(043)007【总页数】4页(P141-144)【关键词】肉骨粉;快速检测;显微近红外成像;标准光谱库;图像分割【作者】姜训鹏;杨增玲;刘贤;韩鲁佳【作者单位】中国农业大学工学院,北京100083;中国农业大学工学院,北京100083;中国农业大学工学院,北京100083;中国农业大学工学院,北京100083;中国农业大学动物营养学国家重点实验室,北京100083【正文语种】中文【中图分类】O657.33;TP391.41引言显微近红外成像技术是随着阵列检测器技术发展而产生的一种微区分析技术,具有非破坏性、干扰性小、光谱分辨率高、信噪比高等特点[1]。
- 1、下载文档前请自行甄别文档内容的完整性,平台不提供额外的编辑、内容补充、找答案等附加服务。
- 2、"仅部分预览"的文档,不可在线预览部分如存在完整性等问题,可反馈申请退款(可完整预览的文档不适用该条件!)。
- 3、如文档侵犯您的权益,请联系客服反馈,我们会尽快为您处理(人工客服工作时间:9:00-18:30)。
Hypergraph-Regularized Sparse NMF for Hyperspectral Unmixing Wenhong Wang,Yuntao Qian,Member,IEEE,and Yuan Yan Tang,Fellow,IEEEAbstract—Hyperspectral image(HSI)unmixing has attracted increasing research interests in recent decades.The major diffi-culty of it lies in that the endmembers and the associated abun-dances need to be separated from highly mixed observation data with few a priori information.Recently,sparsity-constrained non-negative matrix factorization(NMF)algorithms have been proved effective for hyperspectral unmixing(HU)since they can suffi-ciently utilize the sparsity property of HSIs.In order to improve the performance of NMF-based unmixing approaches,spectral and spatial constrains have been added into the unmixing model, but spectral–spatial joint structure is required to be more accu-rately estimated.To exploit the property that similar pixels within a small spatial neighborhood have higher possibility to share sim-ilar abundances,hypergraph structure is employed to capture the similarity relationship among the spatial nearby pixels.In the con-struction of a hypergraph,each pixel is taken as a vertex of the hypergraph,and each vertex with its k nearest spatial neighbor-ing pixels form a ing the hypergraph,the pixels with similar abundances can be accurately found,which enables the unmixing algorithm to obtain promising results.Experiments on synthetic data and real HSIs are conducted to investigate the performance of the proposed algorithm.The superiority of the proposed algorithm is demonstrated by comparing it with some state-of-the-art methods.Index Terms—Hypergraph learning,hyperspectral unmixing (HU),nonnegative matrix factorization(NMF),sparse coding.I.I NTRODUCTIONH YPERSPECTRAL remote sensing image generally con-tains hundreds of spectral bands about the scene,which is effective for detecting and recognizing the land-cover materials. Due to the limited spatial resolution of hyperspectral sensors, different types of materials may simultaneously exist in a sin-gle pixel,called“mixed pixel,”and the corresponding spectrum Manuscript received August12,2015;revised October24,2015;accepted December01,2015.Date of publication January05,2016;date of current version February09,2016.This work was supported in part by the National Natural Science Foundation of China under Grant61571393and Grant 61273244,in part by the Research Grants of the University of Macau under Grant MYRG2015-00049-FST and Grant MYRG2015-00050-FST,in part by the Science and Technology Development Fund(FDCT)of Macau under Grant 100-2012-A3and Grant026-2013-A,and in part by the Macau-China join Project008-2014-AMJ.(Corresponding author:Yuntao Qian.)W.Wang is with the Institute of Artificial Intelligence,College of Computer Science,Zhejiang University,Hangzhou310027,China,and also with the College of Computer Science,Liaocheng University,Liaocheng252059, China.Y.Qian is with the Institute of Artificial Intelligence,College of Computer Science,Zhejiang University,Hangzhou310027,China(e-mail: ytqian@).Y.Y.Tang is with the Faculty of Science and Technology,University of Macau,Macau999078,China.Color versions of one or more of thefigures in this paper are available online at .Digital Object Identifier10.1109/JSTARS.2015.2508448is a mixture of several pure spectrums(i.e.,endmembers).Theoccurrence of mixed pixels has become a challenging problemfor analyzing hyperspectral data quantitatively[1].Currently,hyperspectral unmixing(HU)is an effective technology totackle this problem[2],which aims to identify a collection ofendmembers corresponding to the pure materials present in thescene,and estimate the proportions(i.e.,abundances)of theendmembers in each pixel.Under the linear mixture model(LMM)of hyperspectralimage(HSI)[1],a number of HU methods have been proposedin recent decades.Among them,nonnegative matrix factoriza-tion(NMF)[3]and its variants attract increasing attentionsdue to the good unmixing performance for highly mixed HSIs[4]–[8].NMF can learn a parts-based representation of thedata by factorizing a nonnegative matrix into two nonnegativefactor matrices.When it is applied to HU,NMF can esti-mate the endmembers and abundances simultaneously withoutthe pure-pixel assumption.However,NMF has a large num-ber of local minima owning to the nonconvexity of its costfunction,which leads to unstable unmixing results.To dealwith this problem,an effective scheme is to introduce addi-tional structure constraints into NMF model.For example,theconstraint of sparsity has been introduced into NMF-basedunmixing methods,so that the inherent sparseness property ofHSI is sufficiently exploited[4],[5],[9],[10].Recently,a novelsparsity-constrained NMF(L1/2-NMF)unmixing method[4]has been widely used in HU[2],[8],[11].In L1/2-NMF,L1/2regularizer,instead of L1regularizer,is imposed on the abundances to enforce the sparse estimations.L1/2-NMF hasbeen demonstrated to be superior compared with some ofthe state-of-the-art approaches.However,like other sparsity-constrained NMF,the unmixing performance of L1/2-NMF isstill susceptible to the initial setting and the noise disturbance.Besides the constraint of sparsity,other constrains based onthe characteristics of HSI have been proposed to improve theNMF-based unmixing methods.Some constraints are depen-dent on spectral similarity between pixels,which aim toexploit the similarity relations of pixels in the spectral space.For example,a graph-regularized L1/2-NMF unmixing algo-rithm(GLNMF)[8]is proposed by incorporating graph-basedregularization into sparse NMF,which tries to make moreaccurate abundance estimations by capturing the intrinsic man-ifold structure of the data.Different from the constraint ofspectral similarity,some methods adopt the spatial similar-ity constraints,by which the structure consistency of HSIamong the spatially nearby pixels are considered.In[6],avariant of NMF,named abundance separation and smoothness-constrained NMF(ASSNMF),is proposed by integrating an1939-1404©2015IEEE.Personal use is permitted,but republication/redistribution requires IEEE permission.See /publications_standards/publications/rights/index.html for more information.abundance separation constraint with a spatial similarity con-straint,so that the abundances of a pixel are promoted to be consistent with the average abundances of its surrounding blocks.In[12],a weighted NMF unmixing algorithm(WNMF) is presented,where a local neighborhood weight is designed to enforce the abundance similarity among spatial neighbor-ing pixels.To utilize both spectral and spatial information,a few NMF variants incorporate the spectral–spatial similarity constrains.In[13],a neighborhood-preserving regularization, which assumes that each pixel can be linearly reconstructed by its spatial nearby pixels,is added into NMF to keep the local geometric structure of the data.In[11],a dual graph-regularized sparse NMF(DGNMF)is proposed by integrating the data consistency constraint in the spatial space with the intrinsic manifold constraint in the spectral space.Among these constraints,spectral–spatial joint constraint has shown con-siderable potentials for HU applications because it can make use of the spatial and spectral information simultaneously. Nevertheless,how to effectively model the spectral–spatial joint relationship remains a challenging problem.In order to make full use of the spatial–spectral joint infor-mation in the sparse unmixing model,in this paper,a novel unmixing algorithm,named hypergraph-regularized L1/2-NMF (HG L1/2-NMF),is proposed.According to the basic spatial–spectral correlation property of HSI,the similar pixels within a small spatial neighborhood have higher possibility to share similar abundances.Thus,the spectral similarity of the spa-tial nearby pixels can be used to promote the consistency of the abundances.In our method,this spectral–spatial joint struc-ture is modeled by a hypergraph rather than a simple graph because hypergraph learning has some attractive advantages. Currently,hypergraph learning has been successfully applied in manyfields such as image classification[14]and cluster-ing[15],information retrieval[16],sparse coding[17],and HSI analysis[18]–[20].Compared with the simple graph that just represents the pairwise relation between two pixels,the hypergraph can model the high-order relation among multiple pixels by including more than two vertices in its hyperedges. This enables us to model the spatial and spectral similarity relationships more accurately.In a hypergraph model,each vertex represents a pixel of HSI,and each hyperedge rep-resents the similarity relation among a group of pixels with higher similarity in a spatial block.By hypergraph learning, the spatial–spectral joint structure as a regularization term is incorporated into the sparse unmixing model to enforce the consistency of abundances among the pixels in the same hyper-edge.Moreover,with its hyperedge structure and the associated weights,the hypergraph can provide a robust representation of the similarity relationship among the spatial neighboring pixels,which alleviates the noise disturbance to the unmixing results.The performance of the proposed unmixing method is investigated by conducting a series of experiments on synthetic data sets and real-world HSIs.Our method is compared with some of the state-of-the-art unmixing algorithms to validate its effectiveness.This paper is organized as follows.In Section II,we give a brief review of LMM and NMF.In Section III,we present the HG L1/2-NMF unmixing algorithm,its update rules,and some implementation issues.Extensive experimental results on syn-thetic data and real HSIs are given in Section IV.We conclude this paper in Section V.II.P RELIMINARYA.Linear Spectrum Mixture ModelAccording to the LMM,an observed pixel spectrum is assumed to be a linear combination of a set of endmember signatures weighted by the associated abundance fractions[1]. Given that a hypespectral image with L bands and N pixels is denoted by Y∈R L×N,each pixel of Y can be formalized asy=As+e(1) where y∈R L×1is a vector denoting the target pixel,A∈R L×P is the endmember matrix consisting of P endmembersignatures a j,for j=1,...,P,s∈R P×1is the abundance vector including the proportions of corresponding endmem-bers in the pixel y,and e∈R L×1represents a measurement error vector.In general,the abundance vector s always satisfies the abundance nonnegativity constraint(ANC)and the abun-dance sum-to-one constraint(ASC),which are respectively represented byANC:s j≥0,j=1,...,P(2)ASC:Pj=1s j=1.(3)Using the matrix operations,(1)can be re-expressed by a compact formY=AS(4)where S=[s1,s2,...,s N]∈R P×N denotes the abundance matrix,and the measure error term is removed from the model by merging it into the endmember matrix[7].Under LMM,HU can be regarded as a blind source sep-aration problem,in which the endmember matrix A and the abundance matrix S need to be estimated simultaneously.B.Nonnegative Matrix FactorizationNMF is a powerful tool for data analysis,which provides a parts-based representation for nonnegative data.At present, NMF has been demonstrated effective for HU analysis owning to its inherent advantages.Given a nonnegative matrix Y∈R L×N and a prespecified positive integer P<min(L,N),NMF aims to factorize Y as a product of two nonnegative matrix factors A∈R L×P and S∈R P×N such that the error between Y and its reconstruction AS is minimized.Generally,NMF is formalized as the following optimization problem:minA,SF(A,S)= Y−AS 2F;s.t.A,S 0(5)where“ ”represents the element-wise great-or-equal relation, and · F denotes the Frobenious norm.W ANG et al.:HYPERGRAPH-REGULARIZED SPARSE NMF FOR HU683 NMF wasfirst introduced by Paatero and Tapper underthe name of positive matrix factorization[21].It became tobe widely used since Lee and Seung proposed the followingmultiplicative update rules(MURs)[22]:A←A.∗(Y S T./ASS T)(6)S←S.∗(A T Y./A T AS)(7)where(·)T is the matrix transpose operator,and“.∗”and“./”stand for element-wise matrix multiplication and division,respectively.Thereafter,many effective optimization methodsfor NMF have been proposed,such as active set method[23],projected gradient method[24],projected nonnegative leastsquares[25],and optimal gradient method[26].Among them,MUR is the most used approach due to its simplicity andeasiness to implement.Although(5)is convex with respect to either A or S,it is non-convex for both of them simultaneously.As a result,there area large amount of local minima in the solution space of NMF.This will lead to instable unmixing results when NMF is appliedto HU.To alleviate this problem,an effective method is tointroduce additional constraints into NMF,so that the feasiblesolutions of the algorithm are confined.Recently,sparsity-constrained NMF has received increasingattentions because sparseness is an important property of HSI[4],[5],[9],[10].In L1/2-NMF unmixing method[4],L1/2regularizer as sparseness constraint is incorporated into NMFto pursue sparser abundances.Since L1/2-NMF can effectivelyexploit the inherent sparseness of the data,it shows com-parative superiority compared with the basic NMF and othersparse NMF approaches.The objective function of L1/2-NMFis formulated asF(A,S)=12Y−AS 2F+λ S 1/2(8)where S 1/2= P,Np,n=1(s pn)1/2is the L1/2regularizationandλ∈R+is the regularization parameter.However,the unmixing performance of L1/2-NMF is still not robust to the initial setting of the endmember and abundance matrices. In addition,the separation results yielded by L1/2-NMF are often influenced by noise interference.In order to get superior unmixing solutions,more constraints concerning other a priori structures of HSI should be added into NMF model.III.HG NMF U NMIXING A LGORITHMIn this section,wefirst introduce the concepts about hyper-graph learning.Then,the proposed HG L1/2-NMF algorithm is described.In addition,the MURs for the optimization proce-dure and the implementation issues are addressed.A.Hypergraph LearningHypergraph learning[15],[27]is extended from the learn-ing technologies based on simple graph.A simple graph model only considers the pair-wise relationship between data samples. This will lead to the loss of information about some com-plex relationships that are crucial to particular applications.For Fig.1.Illustration of the hypergraph˜G with its incidence matrix. example,it is easy tofind two close data samples by the pair-wise similarity,while it is hard to infer whether there is a group of(more than two)similar samples.Unlike the simple graph, a hypergraph can capture the high-order relationships among multiple data samples,which is expected to effectively model the spectral–spatial joint structure of HSI.Now,some concepts about the hypergraph are introduced.Definition1:Let G=(V,E),where V={v1,v2,...,v N} is afinite set of elements,and E={e1,e2,...,e M}is a collection of subsets of V.G is a hypergraph defined on V if:1)e j=∅,for j=1,2,...,M;2)e1∪e2,...,∪e M=V.An element of V is called a vertex,and each e j∈E is known as a hyperedge of G.Each hyperedge e can be assigned with a positive value w(e),which is referred to a hyperedge weight. Commonly,an incidence matrix H∈R|V|×|E|is used to rep-resent the incidence relation between a vertex and a hyperedge, which is formalized asH(v,e)=1,if v∈e0,otherwise.(9)Fig.1gives an illustration of a hypergraph˜G=(˜V,˜E)with its incidence matrix˜H,where˜V={v1,v2,v3,v4,v5,v6,v7,v8} and˜E={e1={v1,v2,v3},e2={v3,v4,v5,v6},e3={v6, v7,v8}}.In Fig.1,the solid nodes stand for the vertices and the node sets marked by the ellipses denote the hyperedges. The corresponding incidence matrix˜H is shown in the right of the hypergraph˜G.The degree of each vertex v∈V is defined as the sum of weights associated with the hyperedges that v belongs to,i.e., d(v)={e∈E|v∈e}w(e).The hyperedge degree of each e∈E is defined as the number of vertices included in e,i.e.,δ(e)= |e|.Specifically,d(v)andδ(e)can be calculated,respectively, byd(v)=Mj=1w(e j)H(v i,e j)(10)δ(e)=Nj=1H(v j,e).(11)Let D v stands for the diagonal matrix of vertex degree whose entry(D v)ij=d(v i)if i=j,and(D v)ij=0other-wise.Similarly,D e and W individually denote the matrices of684IEEE JOURNAL OF SELECTED TOPICS IN APPLIED EARTH OBSERV ATIONS AND REMOTE SENSING,VOL.9,NO.2,FEBRUARY2016 Fig.2.Generation procedure of the hypergraph.Atfirst,each pixel of the given HSI is regarded as a centroid node to determine a spatial neighborhood.Next,the KNNs of the centroid pixel(marked by dashed line square),which are chosen within the corresponding spatial neighborhood,are used to generate a hyperedge. At last,all the obtained hyperedges form a hypergraph as a whole.hyperedge degree and hyperedge weight,which consist ofδ(e j) and w(e j),for j=1,2,...,M,respectively,in their diagonal entries.Then,the unnormalized hypergraph Laplacian matrix can be formalized asL Hyper=D v−B(12) where B=HW(D e)−1H T.Generally,a hyperedge is allowed to include an arbitrary number of vertices according to its definition.For the sake of simplicity,in this paper,the uniform hypergraph which contains the same number of nodes in each hyperedge is employed.B.HG L1/2-NMFAs aforementioned,NMF-based unmixing methods should benefit from both spatial and spectral information of HSI. Therefore,to improve the unmixing performance of the sparse NMF,spatial and spectral joint information available in the data needs to be fully exploited in the unmixing process.According to the basic property of HSI,the spatial-nearby pixels(but they are not requested to be spatial-adjacent)generally have highly consistent mixture structure such that they have great probability to share similar abundances.At the same time,the similarity between two pixels in the spectral space is also help-ful to identify the pixels with similar abundances.Therefore, the similarity relationships between pixels in the spectral space and in spatial neighborhood both play important roles in HU. In order to accuratelyfind the similar pixels within local spatial region,in this paper,a hypergraph G s=(V s,E s,W s) is constructed based on the overlapped spatial neighborhoods of a given HSI.Fig.2gives an illustration of the generation procedure of the hypergraph.Atfirst,each pixel spectrum y i in the HSI Y=[y1,y2,...,y N]is taken as an element of the vertex set V s.Then,each vertex v i∈V s acts as the“centroid”node of the located spatial region,and the corresponding hyper-edge e i∈E s is constructed by including v i and its K-nearest neighbors(KNNs)that are chosen from the same spatial neigh-borhood as v i.Therefore,the hypergraph G s consists of N hyperedges that are constructed on different spatial regions. Based on these hyperedges,the incidence matrix H ∈R N×N is obtained by following(9).Next,each hyperedge e i∈E s is assigned a positive weight,which models the spectral similar-ity of the pixels included in the hyperedge e i.The weight can be calculated as follows[18]:w(e i)=y j∈e iexp−y j−y i 22(13)whereδ=1KNNi=1{y j∈e i}y i−y j denotes the mean distance among all the spatial neighboring ing this weight estimation method,a hyperedge that consists of pixels with higher similarity will be associated with a larger weight. According to the above steps,each vertex is connected with at least one hyperedge and each hyperedge is associated with a weight.Then,the diagonal weight matrix W s∈R N×N can be given by(W s)ij=w(e i),if i=j0,otherwise.(14)With the obtained weight matrix W s,we can enforce the consistency of abundances corresponding to the pixels in each hyperedge by minimizing the following function:C=e∈E s{i,j}∈ew(e)δ(e)s i−s j 2(15) which can be reexpressed asC=Tr(SL Hypers S)(16)W ANG et al.:HYPERGRAPH-REGULARIZED SPARSE NMF FOR HU685 where Tr(·)stands for the trace operation of a matrix andL Hypers is the hypergraph Laplacian matrix of G s and definedbyL Hypers=D v−H W s(D e)−1(H )T.(17)Note that D v∈R N×N and D e∈R N×N in(17),respectively,denote the matrices of vertex degree and hyperedge degree ofhypergraph G s.By introducing the hypergraph-based regular-ization term(16)into the objective function of the originalL1/2-NMF,we obtain the HG L1/2-NMF as follows:F(A,S)= Y−AS 2F+α S 12+βTr(SL Hypers ST)(18)whereα∈R+andβ∈R+are the regularization parameters.C.Update RulesThe objective function F of HG L1/2-NMF in(18)is noncon-vex for both A and S simultaneously.Therefore,it is unrealistic to expect an optimization procedure to produce the global opti-mal solution.In this section,we deduce the MURs using the method of Lagrange multipliers[28],[29]tofind the local minima via an alternating optimization procedure.The objective function F in(18)can be reexpressed asF=Tr((Y−AS)(Y−AS)T)+α S 12+βTr(SL Hypers ST)=Tr(Y Y T)−2Tr(Y S T A T)+Tr(ASS T A T)+α A 12+βTr(SL Hypers ST).(19)Considering that the objective function is subject to the con-straints A lp≥0and S pn≥0,the corresponding Lagrange multipliers are defined asΥlp≥0andΛpn≥0,respectively. Then,the Lagrange functionΨis given byΨ=Tr(Y Y T)−2Tr(Y S T A T)+Tr(ASS T A T)+α S 12+βTr(SL Hypers ST)+Tr(ΥA T)+Tr(ΛS T).(20)Taking the partial derivatives ofΨwith respect to A and S, respectively,we get∂Ψ∂A =−2Y S T+2ASS T+Υ(21)∂Ψ∂S =−2A T Y+2A T AS+α2S−12+2βSL Hypers+Λ.(22)Using the Karush–Kuhn–Tucker(KKT)conditionsΥlp A lp=0 andΛpn S pn=0,the following formulas for A lp and S pn are obtained(ASS T)lp A lp−(Y S T)lp A lp=0(23)(A T AS)pn S pn+(α4S−12)pn S pn+(βSD v)pn S pn =(A T Y)pn S pn+β(SH W s(D e)−1(H )T)pn S pn.(24)Fig.3.Six endmembers used to create synthetic data sets.From the above equations,we can deduce the following MURs:A lp←A lp(Y S T)lp(ASS T)lp(25)S pn←S pn(A T Y+βSH W s(D e)−1(H )T)pn(A T AS+(α4S−12)+βSD v)pn.(26)The convergences of these two update rules are guaranteed by the Theorem1.Theorem1:The objective function F in(18)is nonincreas-ing under the update rules in(25)and(26).The detail proof for Theorem1is presented in the Appendix. The proof procedure is similar to the convergence proof of the standard NMF[22].D.Algorithm ImplementationIn this section,several implementation issues of the pro-posed unmixing algorithm are addressed.Thefirst issue is about the initialization of the matrices A and S.Since the objective function in(18)is nonconvex with respect to A and S simul-taneously,we can only expect to achieve local minima with the aforementioned update rules.Thus,it will yield different unmixing outputs when different initial settings are provided for these two matrices.In this paper,we adopt two initialization methods:1)random initialization and2)VCA-FCLS initial-ization.VCA-FCLS initialization provides initial endmembers and abundances via vertex component analysis(VCA)[30] and fully constrained least squares(FCLSs)[31]algorithms, respectively.In the experiments on synthetic HSI data,ran-dom initialization is employed,which generates the initial value for each entry of A and S randomly in interval[0,1],whereas VCA-FCLS initialization is used in the experiments on real HSI data.The second issue involves the ASC constraint.As the method suggested in[4]and[31],the observed HSI data matrix Y and the endmember matrix A are,respectively,augmented as686IEEE JOURNAL OF SELECTED TOPICS IN APPLIED EARTH OBSERV ATIONS AND REMOTE SENSING,VOL.9,NO.2,FEBRUARY2016 Fig.4.Performance of HG L1/2-NMF with respect to parameters(a)βand(b)K.Fig.5.Results on synthetic data with different SNRs:(a)SAD and(b)RMSE.Y a←Yδ1T N(27)A a←Aδ1T P(28)whereδcontrols the impact of ASC on the cost function,whichis set to15in the experiments as suggested in[32].1T N and1T Pare one row vectors with the dimensions of P and N,respec-tively.Accordingly,in each update step of S,the matrices Yand A are replaced by Y a and A a,respectively,in the updaterule(26).The third issue concerns the L1/2sparsity operator in theupdate rule(26).Since the operation(s pn)−12is invalid if s pnequals zero,effective measures should be taken to keep therobustness of the algorithm.Here,we follow the method used in[4],in which those entries of S with the values less than a pre-defined threshold10−4will be neglected by the correspondingsparsity operator.The last issue is about the selection of the parameters ofHG L1/2-NMF algorithm.There are four critical parameters:the size of neighborhood,the regularization parametersα,β,and the number of neighbors K.In the experiments,the size ofneighborhood is defined as5×5.The regularization parameterαcan impact the effect of sparsity constraint,which has beeninvestigated in[4].A proper value forαis determined byα=1√LLl=1√N− [Y]l,: 1/ [Y]l,: 2√N−1(29)where[Y]l,:denotes the l th band of the observed HSI Y.As forhow to select the suitable values forβand K,it will be analyzedin the experiments.Based on these implementation techniques,the proposedHG L1/2-NMF algorithm for unmixing is given in Algorithm1.Its computational cost primarily concerns the update steps ofmatrices A and S included in the iteration body.For the pur-pose of accurately analyzing the computational complexity,we count thefloating-point arithmetic operations involved inthe algorithm.To be specific,the number offloating-pointoperations needed by the step6is2N(LP+P2)+P2(2L−1);whereas,in step8,it is N2(P2+2P)+N(2LP+P2+6P)+P2(2L−1).Given that the algorithm terminates afterm iterations,the overall computational cost of the proposedalgorithm is O(mN2(P2+2P)).Algorithm1.HG L1/2-NMF Algorithm For HyperspectralUnmixingInput:The hyperspectral image Y≡[y1,y2,...,y N]∈R L×N;The algorithm regularization parametersαandβ;The number of nearest neighbors K;Output:The abundance matrix S;The endmember matrix A;1:Estimate the number of endmember P;2:Initialize endmember matrix A and abundance matrix S;3:Augment Y to generate Y a by(27);4:Construct hypergraph G s and compute L Hypers by(17);5:repeat6:Update A according to(25);7:Augment A to obtain A a following(28);8:Update S by(26);9:until the stopping condition of the algorithm is satisfied.W ANG et al.:HYPERGRAPH-REGULARIZED SPARSE NMF FOR HU687 Fig.6.Results on synthetic data with different degrees of mixing:(a)SAD and(b)RMSE.Fig.7.Results on synthetic data with different numbers of pixels:(a)SAD;(b)RMSE;and(c)Runningtime.Fig.8.Convergence curves of all thealgorithms.Fig.9.False-color image of the HYDICE Washington DC Mall data.IV.E XPERIMENTS R ESULTSIn this section,a series of experiments are conducted on syn-thetic and real-world HSI data to investigate the performanceof our algorithm.In the experiments on synthetic data,wecompare the proposed HG L1/2-NMF algorithm with threerepresentative algorithms:1)L1/2-NMF;2)GLNMF;and3)VCA-FCLS.In the experiments on real data,the proposedHG L1/2-NMF algorithm is compared with six representativemethods:1)ASSNMF;2)L1/2-NMF;3)GLNMF;4)DGNMF;5)minimum-volume-constrained NMF(MVCNMF)[7];and6)VCA.In these algorithms,HG L1/2-NMF,ASSNMF,andDGNMF consider the spatial information in unmixing.A.Performance CriteriaTo measure the unmixing accuracy of the algorithms,twowidely used criteria named spectral angle distance(SAD)androot-mean-square error(RMSE)are adopted in our experi-ments.For the p th endmember,the SAD between the estimatedendmemberˆa p and the corresponding reference signature a p isdefined asSAD p=cos−1a T pˆa pa p ˆa p(30)where · stands for the Euclidean norm of vector.In order tocompare the similarity between the reference abundances andthe estimated ones,the RMSE criteria for the p th endmember isdefined asRMSE p=1NNj=1(s pj−ˆs pj)2(31)where s pj denotes the reference abundance for the p th endmem-ber at the j th pixel,andˆs pj represents the estimated one.The。