L. Multi Agent System for Negotiation and Decision Support
全球2℃温升目标与应对气候变化长期目标的演进——从联合国气候变化框架公约到巴黎协定
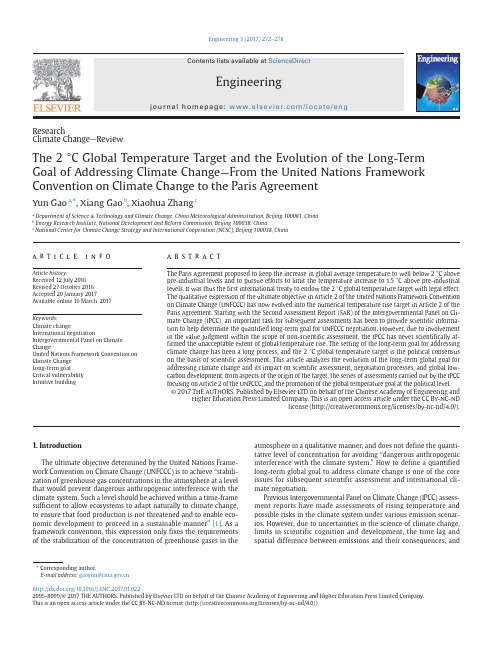
Engineering 3 (2017) 272–278ResearchClimate Change—ReviewThe 2 °C Global Temperature Target and the Evolution of the Long-Term Goal of Addressing Climate Change—From the United Nations Framework Convention on Climate Change to the Paris AgreementYun Gao a ,*, Xiang Gao b , Xiaohua Zhang caDepartment of Science & Technology and Climate Change, China Meteorological Administration, Beijing 100081, China bEnergy Research Institute, National Development and Reform Commission, Beijing 100038, China cNational Center for Climate Change Strategy and International Cooperation (NCSC), Beijing 100038, Chinaa r t i c l e i n f oa b s t r a c tArticle history:Received 12 July 2016Revised 27 October 2016Accepted 20 January 2017Available online 16 March 2017The Paris Agreement proposed to keep the increase in global average temperature to well below 2 °C above pre-industrial levels and to pursue efforts to limit the temperature increase to 1.5 °C above pre-industrial levels. It was thus the first international treaty to endow the 2 °C global temperature target with legal effect. The qualitative expression of the ultimate objective in Article 2 of the United Nations Framework Convention on Climate Change (UNFCCC) has now evolved into the numerical temperature rise target in Article 2 of the Paris Agreement. Starting with the Second Assessment Report (SAR) of the Intergovernmental Panel on Cli-mate Change (IPCC), an important task for subsequent assessments has been to provide scientific informa-tion to help determine the quantified long-term goal for UNFCCC negotiation. However, due to involvement in the value judgment within the scope of non-scientific assessment, the IPCC has never scientifically af-firmed the unacceptable extent of global temperature rise. The setting of the long-term goal for addressing climate change has been a long process, and the 2 °C global temperature target is the political consensus on the basis of scientific assessment. This article analyzes the evolution of the long-term global goal for addressing climate change and its impact on scientific assessment, negotiation processes, and global low- carbon development, from aspects of the origin of the target, the series of assessments carried out by the IPCC focusing on Article 2 of the UNFCCC, and the promotion of the global temperature goal at the political level.© 2017 THE AUTHORS. Published by Elsevier LTD on behalf of the Chinese Academy of Engineering andHigher Education Press Limited Company. This is an open access article under the CC BY-NC-NDlicense (/licenses/by-nc-nd/4.0/).Keywords:Climate changeInternational negotiationIntergovernmental Panel on Climate ChangeUnited Nations Framework Convention on Climate Change Long-term goalCritical vulnerability Intuitive building1. IntroductionThe ultimate objective determined by the United Nations Frame-work Convention on Climate Change (UNFCCC) is to achieve “stabili-zation of greenhouse gas concentrations in the atmosphere at a level that would prevent dangerous anthropogenic interference with the climate system. Such a level should be achieved within a time-frame sufficient to allow ecosystems to adapt naturally to climate change, to ensure that food production is not threatened and to enable eco-nomic development to proceed in a sustainable manner” [1]. As a framework convention, this expression only fixes the requirements of the stabilization of the concentration of greenhouse gases in the atmosphere in a qualitative manner, and does not define the quanti-tative level of concentration for avoiding “dangerous anthropogenic interference with the climate system.” How to define a quantified long-term global goal to address climate change is one of the core issues for subsequent scientific assessment and international cli-mate negotiation.Previous Intergovernmental Panel on Climate Change (IPCC) assess-ment reports have made assessments of rising temperature and possible risks in the climate system under various emission scenar-ios. However, due to uncertainties in the science of climate change, limits in scientific cognition and development, the time lag and spatial difference between emissions and their consequences, and* Corresponding author.E-mail address: gaoyun@/10.1016/J.ENG.2017.01.0222095-8099/© 2017 THE AUTHORS. Published by Elsevier LTD on behalf of the Chinese Academy of Engineering and Higher Education Press Limited Company.This is an open access article under the CC BY-NC-ND license (/licenses/by-nc-nd/4.0/).Contents lists available at ScienceDirectjo ur n al h om e pag e: w w /locate/engEngineering273 Y. Gao et al. / Engineering 3 (2017) 272–278necessary value judgment other than scientific assessment for de-fining danger levels, the IPCC has never scientifically affirmed the indices that indicate “dangerous anthropogenic interference with the climate system,” and thus cannot define the unacceptable extent of global temperature rise based purely on science.Scientific research into the 2 °C temperature rise started a long time ago; however, the 2 °C global temperature target was not con-sidered as the action goal until the decision of the Council of the European Union (EU) conference in 1996 [2]. After the Copenhagen Climate Change Conference in 2009 and the Cancún Climate Change Conference in 2010, limiting the global temperature rise to below 2 °C above pre-industrial levels became the consensus of the in-ternational community. In 2008–2014, the IPCC’s Fifth Assessment Report (AR5) made a comprehensive assessment of the climate system change, risks, emission budget, and mitigation pathway choice of 2 °C global warming on the basis of the research results available. After scientific assessment and a series of political push-es, one of the three goals reached at the 2015 Paris Climate Change Conference was stated as “Holding the increase in the global average temperature to well below 2 °C above pre-industrial levels and pur-suing efforts to limit the temperature increase to 1.5 °C above pre- industrial levels” [3]. Thus, the long-term goal of addressing climate change has evolved from a qualitative expression of stabilizing the greenhouse gas concentration in the atmosphere, in Article 2 of the UNFCCC, to a global temperature target with specific value, in Article 2of the Paris Agreement.This article analyzes the evolution of the long-term goal for ad-dressing climate change, and the related impact on future scientific assessments, negotiation processes, and global low-carbon develop-ment, from the aspects of the origin of the 2 °C global temperature target, the related IPCC conclusion for Article 2 of the UNFCCC, and the promotion of the global temperature goal at the political level. 2. Early scientific research related to the 2 °C targetStudies regarding the 2 °C temperature rise can be traced back to the 1970s, when an explorative study was carried out in the European natural and social sciences to push decisions related to climate change. According to the overview given by Randalls [4] on the history of the EU’s temperature control goal, the proposal for the global temperature control goal was very strongly related to the scientific study of climate sensitivity. Equilibrium climate sensitivity (ECS) quantifies the response of the climate system to constant radiative forces on multi-century timescales. It is defined as the change in the global mean near-surface air temperature at equilibrium that is caused by a doubling of the atmospheric carbon dioxide (CO2) concentration [5]. If the ECS is 2 °C, then the doubling of the CO2 concentration (generally taken as 550 ppm) will result in a global average temperature rise of 2 °C [6]. In 1967, Manabe and Wetherald [7] used a heat balance model and estimated a tempera-ture response of approximately 2 °C to doubling CO2 concentrations; in subsequent climate change science, and particularly in the esti-mation of the climate system model, the doubling of CO2 has been taken as the core scenario for calculation. Initially, the ECS value was estimated by experts, and in the subsequent IPCC’s First to Third As-sessment Reports, it was taken as 1.5–4.5 °C. In the IPCC’s Fourth As-sessment Report, ECS was determined as 2.0–4.5 °C [8]. However, on the basis of many subsequent studies, the IPCC’s Fifth Assessment Report (AR5) made an elaborate analysis of this issue, considering it to be 1.5–4.5 °C, that is, extremely unlikely to be less than 1 °C and very unlikely to be greater than 6 °C [5]. With respect to mitigation, countermeasures and actions to address climate change involve a series of estimations and policy analyses on social and economic costs. In 1977, Nordhaus [9] made an explorative cost-benefit analy-sis of climate change using the CO2 concentration-doubling scenario; subsequent cost-benefit analyses of addressing climate change be-gan to take the doubling of CO2 or the 2 °C scenario as the starting point of exploration, reaching many research conclusions [10].In the 1980s, before the IPCC’s First Assessment Report (FAR) was released, climate change studies mostly focused on the relationship between increased anthropogenic greenhouse gas emission and greenhouse gas concentration in the atmosphere, and the global average temperature, calling attention to possible threats from an-thropogenic factors. However, there was insufficient basis to deter-mine the indices that should be chosen and the specific figure that would be used as the global ultimate objective in addressing climate change. In addition, since addressing climate change involves com-plex fields, discussion at the political or policy level tends to give a relatively prudent expression of proposed reductions of greenhouse gas emissions, and to wait for further scientific research results [4]. At that time, some scholars proposed a study of the threshold value of climate change from wider perspectives, in order to determine the level at which climate change can be accepted or avoided; that is, they hoped to make a systematic assessment of various risks that may result from climate change, instead of paying attention only to carbon emission [11].3. IPCC’s First and Second Assessment Reports and decision of the European CouncilIn 1990, the IPCC released its FAR. Based on the progress of study in that period, the FAR pointed out that the emissions from human activities resulted in an obvious increase of the concentration of greenhouse gases in the atmosphere, aggravated the greenhouse effect, and caused the global near-surface air temperature to rise, thus inciting the international community to immediately effect political progress and discuss how to take action to deal with global climate change. In this report, the assessment was made under the “business-as-usual” emissions scenario (Scenario A), along with other scenarios with progressively increasing levels of the controls (Scenarios B, C, and D); these scenarios held that in around 2025, 2040, 2050, and 2100, respectively, the equivalent CO2 would be two times that of pre-industrial-revolution levels, and the global average temperature would rise by 0.1–0.3 °C per decade. In order for the concentration to remain stable at the level of that period (1990), it would be necessary to immediately reduce the anthropogenic emis-sion of greenhouse gases (mainly CO2) by 60%, and reduce methane by 15%–20% [12]. The IPCC’s FAR placed emphasis on the rising tem-perature effect due to the anthropogenic emission of greenhouse gases; the scientific basis was insufficient at that time to formulate suggestions for a specific goal. Considering that addressing climate change involves wide and complex fields, the UNFCCC was formed under the encouragement of the FAR, and established the qualitative expression of the ultimate objective.As an important scientific support for the UNFCCC’s negotiation process, the IPCC included an examination of approaches to the realization of Article 2 of the UNFCCC in the Second Assessment Report following a resolution of the Executive Council of the World Meteorological Organization. In addition, the IPCC specifically for-mulated a synthesis report to present information on the scientific and technical issues related to interpreting Article 2 of the UNFCCC [13]. In fact, since the IPCC’s Second Assessment Report (SAR), providing scientific information to assist the quantification of the long-term goal for the UNFCCC’s negotiation has been an impor-tant task in the IPCC’s scientific assessments. According to the SAR published in 1996, the scientific, technical, economic, and social science literature does suggest ways to move toward the ultimate objective of the UNFCCC, but uncertainties remain for the judg-ment of what constitutes dangerous anthropogenic interference with the climate system and what needs to be done to prevent such274Y. Gao et al. / Engineering 3 (2017) 272–278and risk. Since both the magnitude and rate of climate change are very important, the basis for determining what constitutes “dan-gerous anthropogenic interference” would vary in different regions, depending on local characteristics, as would the consequences, ad-aptation, and mitigation capacity of the impact of climate change.Notably, the TAR introduced five “reasons for concern,” thus con-ceptually expressing the reasons for emphasizing the risks of climate change (Fig. 1) [14]. These five reasons include: ① risks to unique and threatened systems; ② risks from extreme climate events; ③ dis-t ribution of impact; ④ aggregate impact; and ⑤ risks from future large-scale discontinuities. In Fig. 1, the global average annual tem-perature represents the magnitude of climate change; however, it is pointed out that future impacts will be a function of the magnitude and rate of global and regional changes in mean climate, climate variability, extreme climate phenomena, socioeconomic conditions, and adaptation, depending on multiple aspects. The TAR also stat-ed that in the setting of the strategy, goal, and timetable to avoid “dangerous anthropogenic interference with the climate system,” it is necessary to consider inertia and uncertainty in the climate, eco-logical, and socioeconomic systems; assess possible risks of climate change from more comprehensive and intuitive perspectives; and relate the risks with global mean near-surface air temperature. As shown in Fig. 1, although the TAR did not define which kind of tem-perature rise should become the index of “dangerous anthropogenic interference with the climate system,” it showed that global warm-ing above 4 °C would pose extremely large risks. These five reasons for concern have been used consistently in subsequent IPCC assess-ments.In 2007, the IPCC released its Fourth Assessment Report (AR4), stating that the five “reasons for concern” identified in the TAR re-main a feasible framework for considering critical vulnerabilities. In the AR4, many risks are affirmed with higher confidence. For example, the report stated that regarding “unique and threatened systems,” observed climate change has had an impact on the polar and high mountain communities and ecosystems; if the global aver-age temperature becomes 1.5–2.5 °C higher than that in 1980–1999, approximately 20%–30% of the plant and animal species assessed so far are likely to face increased risks of extinction; and an increase of about 1–3 °C in the sea surface temperature would result in moreinterference. In the SAR, the conclusions related to the 2 °C temper-ature rise are that for the mid-range IPCC emission scenario (IS92), assuming the “best estimate” value of climate sensitivity and includ-ing the effects of future increases in aerosol concentrations, models project an increase in the global mean near-surface air tempera-ture relative to 1990 of about 2 °C, an increase in sea level of about 50 cm, a greater risk for small islands and low-lying coastal areas, and extensions of the geographical range and season for vector or-ganisms by 2100.The clear proposition that the global average temperature should not exceed 2 °C above the pre-industrial level was first raised in the decision of the European Council’s conference in 1996 [2]. Based on the IPCC’s SAR and the IS92, and after considering the possible risks of climate change, the European Council held that global greenhouse gas emissions should be halved from the 1990 figure, and that CO 2 concentration in the atmosphere should be stabilized at approxi-mately 550 ppm (twice the pre-industrial level), so as to realize the target of limiting the global average temperature rise to 2 °C above pre-industrial levels (the goal for global mitigation cooperation). The EU did not give a reason for this decision, and its definitive ex-pression did not find support in the IPCC’s SAR. The proposition does not currently receive wide international recognition.4. IPCC’s Third and Fourth Assessment Reports and promotion at the G8 SummitIn 2001, the IPCC released its Third Assessment Report (TAR), which provided newer and stronger evidence to prove that most of the warming observed over the last 50 years is attributable to human activities (probability > 66% but < 90%). The impacts of cli-mate change include both advantages and disadvantages, but the larger and quicker the change, the more obvious the leading posi-tion of adverse impacts. In the Synthesis Report of the IPCC’s TAR [14], Question 1 pointed out that the natural, technical, and social sciences can provide essential information and evidence needed for decisions on what constitutes “dangerous anthropogenic interfer-ence with the climate system.” However, such decisions are value judgments determined through sociopolitical processes that consid-er development, equity, and sustainability, as well as uncertainties Fig. 1. IPCC’s TAR: Corresponding figures for assessment and impact risks. SRES : Special Report on Emissions Scenarios ; A1Fl, A1B, A1T, A2, B1, and B2 are alternative development paths and related greenhouse gas emissions scenarios provide in SRES ; IS92a: Scenario a in IPCC emission scenario 1992. Source: Ref. [14], Fig. SPM-2 in Summary for Policymakers , Climate Change 2001: Impacts, Adaptation, and Vulnerability .275 Y. Gao et al. / Engineering 3 (2017) 272–278frequent coral bleaching and widespread mortality. Regarding ex-treme weather, the projected droughts, heat waves, floods, and their adverse impacts would increase. Regarding the distribution of im-pacts, regions with the weakest economies are often the most vul-nerable to climate change, and the low-latitude and less-developed regions face increasing risks. Regarding aggregate impacts, the net cost of impacts with increased warming would increase as time goes on. And finally, regarding the risks of large-scale singularities, global warming lasting for many centuries would result in a rise in the sea level, which would be much larger than that observed in the 20th century; melting of the Greenland and Antarctic ice sheets could oc-cur on century timescales.The IPCC’s reports should be neutral with respect to policy; thus, the IPCC only provides comprehensive, objective, open, and transparent assessment reports, and leaves readers to make their own judgments. It does not define “dangerous anthropogenic in-terference with the climate system.” Compared with the previous three assessment reports, the AR4 provided a clearer and more intuitive explanation and expression of risks, as shown in Fig. 2[15]. The important progress at this stage is that the scientific commu-nity has confirmed the importance of risk assessment and value judgment of climate change for the determination of the long-term goal, thus pushing the discussion of a global temperature goal into the political process.In February 2005, at the request of the European Council, the European Commission reported on a cost-benefit analysis of the medium- and long-term emission reduction strategy and goal [16]. This report assumed that, until 2100, the global average temperature would rise by 1.4–5.8 °C compared with that in 1990, and the Euro-pean temperature would rise by 2.0–6.3 °C. If the temperature rise could be controlled to within 2 °C, the benefits would be sufficient to offset the cost of the mitigation policy; if the temperature rose over 2 °C, it would probably result in quicker and more unpredict-able climate response, and even irreversible and disastrous conse-quences. Based on this report, European Parliament reiterated the 2 °C target in the same year, and held that the TAR’s conclusion had indicated the necessity to strengthen emission reduction and limit global risks [17]. In July 2005, the British Prime Minister Tony Blair asked the Gleneagles G8 Summit to list climate change as one of two priority themes, as the UK served as the rotating president of the G8 Summit. However, the G8 did not reach consensus on a global tem-perature goal.In 2006, Sir Nicholas Stern (the economic consultant for the Brit-ish Prime Minister) published The Economics of Climate Change: The Stern Review, in which he wrote that if no timely actions were tak-en in the following decades, climate change would result in the loss of 5%–20% of global GDP; however, if immediate and powerful global mitigation actions were taken to stabilize the concentration of greenhouse gases in the atmosphere at 500–550 ppm, the cost could be controlled at about 1% of global GDP per year [18]. After the publication of the IPCC’s AR4, climate change became one of the key topics of the G8 Summits in Heiligendamm (Germany) in 2007, in Toyako (Japan) in 2008, and in L’Aquila (Italy) in 2009. The final declaration made by the L’Aquila G8 Summit expressed willingness to limit the global temperature rise to within 2 °C of pre-industrial levels along with other countries, so that the global greenhouse gas emissions would be reduced by 50% before 2050, and so that devel-oped countries should reduce emissions by 80% or more by 2050. Although the scientific significance of taking a 2 °C temperature rise as the goal was unclear [19], a strong push from the EU at theFig. 2. IPCC’s AR4: Impact examples related to rising global average temperature. Source: Adapted from Ref. [15], Fig. 3.6 in Climate Change 2007: Synthesis Report.276Y. Gao et al. / Engineering 3 (2017) 272–278political level extended the discussion of the global temperature goal from the scientific level to the international political and diplo-matic levels.5. From the Copenhagen Climate Change Conference to the Paris Climate Change ConferenceThe 35th G8 Summit in July 2009 and the subsequent Major Economies Forum on Energy and Climate (MEF) were held just be-fore the Copenhagen Climate Change Conference, attracting world-wide attention. The leaders of the 17 participating members of the MEF delivered a joint declaration requiring that the results of the Copenhagen Climate Change Conference comply with the UNFCCC’s goal and scientific requirements, thus unanimously recognizing that the global average temperature rise shall not be over 2 °C higher than pre-industrial levels [20]. It was the first time that a consen-sus had been reached between the main developed and developing countries regarding the 2 °C target. This MEF Summit delivered a strong political signal to the Copenhagen Climate Change Conference at the end of the same year. Thanks to the efforts of major powers, the 2 °C global temperature target was written into the Copenhagen Accord. Since the Copenhagen Accord was not unanimously rec-ognized by all parties, it did not have legal effect. Part I—“A shared vision for long-term cooperative action”—of the Cancún Agreements formulated at the 2010 Cancún Climate Change Conference stated that nations should work together in “… reducing global greenhouse gas emissions so as to hold the increase in global average tempera-ture below 2 °C above pre-industrial levels, and that Parties should take urgent action to meet this long-term goal … strengthening the long-term global goal on the basis of the best available scientific knowledge, including in relation to a global average temperature rise of 1.5 °C” [21]. The 2 °C global temperature target was a global political consensus from then on.Since 2009, the political consensus of the 2 °C global tempera-ture target has had a major impact on the international scientific community. Corresponding climate change trend simulation, impact assessment, and emission reduction path studies have taken it as the object of scenario studies. Since the IPCC assessment is based on peer-reviewed scientific research, the AR5 of the IPCC fully assessed the scientific research related to the 2 °C global temperature target in 2014. A report from the AR5 Working Group I made the first quan-titative assessment on the cumulative emission space under the 2 °C global temperature target. The global average surface warming in the 21st century and subsequent period would mainly depend on the cumulative emission of CO2. In this report, transient climate response to cumulative carbon emissions (TCRE) is defined as the global mean near-surface air temperature change per 1000 GtC (the shorthand for gigatons of carbon) emitted into the atmosphere. It quantifies the transient response of the climate system to cumu-lative carbon emissions. According to the AR5, TCRE is likely to fall within the range of 0.8–2.5 °C per 1000 GtC; this applies for cumu-lative emissions up to about 2000 GtC, until the time temperatures peak. Limiting the warming caused by anthropogenic CO2 emissions alone with a probability of > 33%, > 50%, and > 66% to less than 2 °C since the period between 1861 and 1880 will require cumulative CO2 emissions from all anthropogenic sources to stay between 0 and about 1570 GtC (5760 GtCO2), 0 and about 1210 GtC (4440 GtCO2), and 0 and about 1000 GtC (3670 GtCO2) since that period, respec-tively; however, an amount of 515 (445–585) GtC (1890 (1630–2150) GtCO2), had already been emitted by 2011 [5].Based on the assessment of different sectors, regions, and key risks, the AR5 Working Group II pointed out that in case of a temperature rise of 1 °C or 2 °C compared with pre-industrial levels, the risks incurred by the world would be on the medium to high level, and in case of a temperature rise over 4 °C or even higher, the risks would be high or very high [22]. The AR5 Working Group III provided the most viable scenario to achieve the goal of keeping the global temperature rise until 2100 within 2 °C compared with the pre- industrial level: to limit the greenhouse gas concentration to 450 ppm CO2e (the shorthand for carbon dioxide equivalents). It will be necessary to reduce the global greenhouse gas emissions until 2030 to 50 Gt CO2e, to reduce global greenhouse gas emissions in 2050 by 40%–70% compared with the 2010 level, and to limit green-house gas emissions to net zero in 2100. The Report also assessed the development and technical choice in sectors such as energy, transport, building, human settlements, infrastructure, and spatial planning under this goal [23].In summary, the IPCC’s AR5 Synthesis Report defined the rela-tionship between cumulative anthropogenic emissions of CO2, glob-al average temperature change, and the potential risks to the climate system until 2050. As shown in Fig. 3[24], the risk level indicated by any given global temperature rise and the five major reasons corre-sponds to the cumulative anthropogenic emissions of CO2 within a certain range.The AR5 did not clearly define the indices and values constituting “dangerous anthropogenic interference with the climate system.” In fact, since it is highly sensitive for parties to consider the related information about Article 2 of the UNFCCC, when the AR5 Synthesis Report was reviewed and adopted at the IPCC’s 40th Session in Oc-tober 2014, the textbox providing information related to Article 2 of the UNFCCC was finally abandoned, and only the Preface of the Syn-thesis Report stated that the Report included information related to Article 2 of the UNFCCC. Nevertheless, the assessment conclusions drawn by the AR5 regarding the 2 °C global temperature target, as well as the scientific information required for decision-making (in-cluding emission budget, pathway, and technical choice) strength-ened the scientific basis for this political consensus.In 2011, the Durban Climate Change Conference established the Ad Hoc Working Group on the Durban Platform for Enhanced Action (hereinafter referred to as the Durban Platform). It launched the negotiation for an international mechanism applicable to all parties after 2020, and decided to strive for an ambitious global emission reduction goal with the timeframe for global greenhouse gas emis-sion peaking before 2050, taking into account reports such as the IPCC’s AR5 [25].From the launch of the Durban Platform to the conclusion of the Paris Agreement at the Paris Climate Change Conference, parties held different views about the expression of the UNFCCC’s prin-ciples, the scope of the agreement, and the legal form of the final results; however, the 2 °C global temperature target seemed indis-putable. The bilateral joint declarations delivered by China with the US, France, and the EU before the Paris Climate Change Conference had mentioned that it was required to “consider a global temper-ature goal within 2 °C.”† To a certain extent, this represented the consensus of China and the developed countries with respect to this issue. Based on scientific assessment and a series of political pushes, the Paris Agreement finally considered “holding the increase in the global average temperature to well below 2 °C above pre-industrial levels and pursuing efforts to limit the temperature increase to 1.5 °C above pre-industrial levels,” as one of three goals of the Agreement, and the 2 °C global temperature target was formally included in the international treaty with legal effect. During the 2016 Opening for the Signature of the Paris Agreement, held at the United Nations Headquarters in New York on 22 April, 175 parties (174 countries†US-China Joint Presidential Statement on Climate Change on November 12, 2014, in Beijing, China; EU-China Joint Declaration on Climate Change on June 29, 2015, in Brussels, Belgium; France-China Joint Presidential Statement on Climate Change on November 2, 2015, in Beijing, China.。
代理报关委托协议 英文
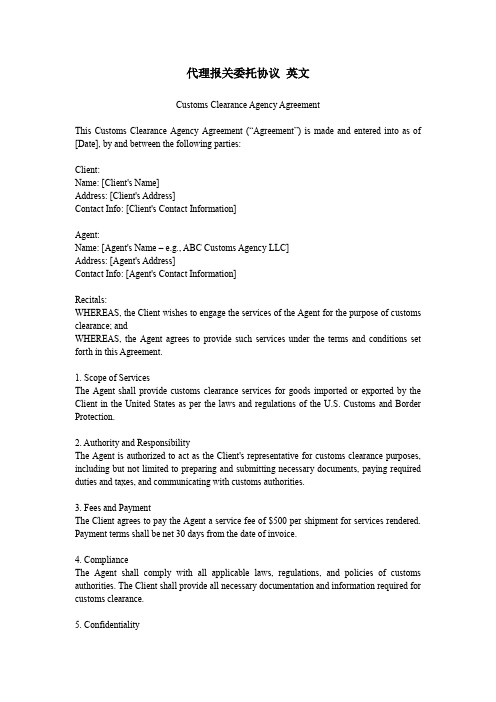
代理报关委托协议英文Customs Clearance Agency AgreementThis Customs Clearance Agency Agreement (“Agreement”) is made and entered into as of [Date], by and between the following parties:Client:Name: [Client's Name]Address: [Client's Address]Contact Info: [Client's Contact Information]Agent:Name: [Agent's Name – e.g., ABC Customs Agency LLC]Address: [Agent's Address]Contact Info: [Agent's Contact Information]Recitals:WHEREAS, the Client wishes to engage the services of the Agent for the purpose of customs clearance; andWHEREAS, the Agent agrees to provide such services under the terms and conditions set forth in this Agreement.1. Scope of ServicesThe Agent shall provide customs clearance services for goods imported or exported by the Client in the United States as per the laws and regulations of the U.S. Customs and Border Protection.2. Authority and ResponsibilityThe Agent is authorized to act as the Client's representative for customs clearance purposes, including but not limited to preparing and submitting necessary documents, paying required duties and taxes, and communicating with customs authorities.3. Fees and PaymentThe Client agrees to pay the Agent a service fee of $500 per shipment for services rendered. Payment terms shall be net 30 days from the date of invoice.4. ComplianceThe Agent shall comply with all applicable laws, regulations, and policies of customs authorities. The Client shall provide all necessary documentation and information required for customs clearance.5. ConfidentialityBoth parties agree to maintain the confidentiality of all information and documents related to the customs clearance process.6. LiabilityThe Agent shall not be liable for any delays, loss, or penalties due to inaccurate or incomplete information provided by the Client or due to reasons beyond the Agent's control.7. Term and TerminationThis Agreement shall be effective from the date of signing and remain in effect until December 31, 20XX. Either party may terminate this Agreement with 30 days' notice.8. Dispute ResolutionAny disputes arising out of or in connection with this Agreement shall be resolved through negotiation, or if necessary, by arbitration or legal action in the jurisdiction of [specified court/location].9. Entire AgreementThis Agreement constitutes the entire agreement between the parties regarding its subject matter and supersedes all prior communications, agreements, or understandings, both written and oral.IN WITNESS WHEREOF, the parties hereto have executed this Customs Clearance Agency Agreement as of the date first above written.Client Signature: __________Agent Signature: __________。
半导体生产线动态实时智能调度方法研究【控制理论与控制工程专业优秀论文】
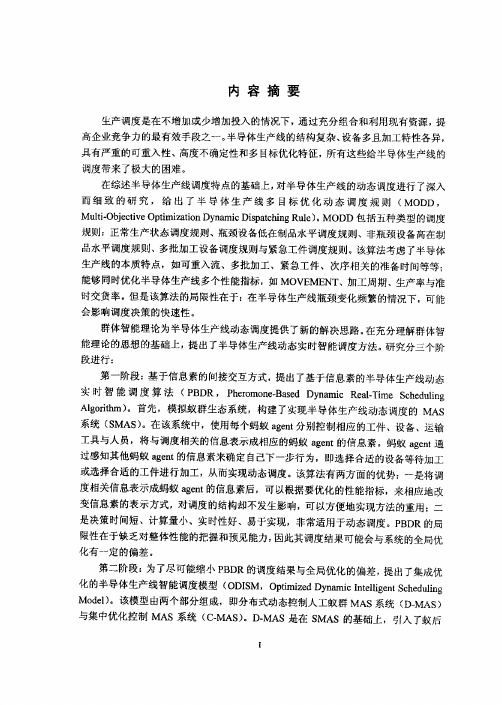
内容摘要生产调度是在不增加或少增加投入的情况下,通过充分组合和利用现有资源,提高企业竞争力的最有效手段之一。
半导体生产线的结构复杂、设备多且加工特性各异,具有严重的可重入性、高度不确定性和多目标优化特征,所有这些给半导体生产线的调度带来了极大的困难。
在综述半导体生产线调度特点的基础上,对半导体生产线的动态调度进行了深入而细致的研究,给出了半导体生产线多目标优化动态调度规则(MODD,Multi.objectiveOptimizationDynamicDispatc}lingRule)。
MODD包括五种类型的调度规则:正常生产状态调度规则、瓶颈设备低在制品水平调度规则、非瓶颈设备高在制品水平调度规则、多批加工设备调度规则与紧急工件调度规则。
该算法考虑了半导体生产线的本质特点,如可重入流、多批加工、紧急工件、次序相关的准备时间等等;能够同时优化半导体生产线多个性能指标。
如MOVEMENT、加工周期、生产率与准时交货率。
但是该算法的局限性在于:在半导体生产线瓶颈变化频繁的情况下,可能会影响调度决策的快速性。
群体智能理论为半导体生产线动态调度提供了新的解决思路。
在充分理解群体智能理论的思想的基础上,提出了半导体生产线动态实时智能调度方法。
研究分三个阶段进行:第一阶段:基于信息素的间接交互方式,提出了基于信息素的半导体生产线动态实时智能调度算法(PBDR,Pheromone.BasedDynamicReal.TimeSchedutingAlgorithm)。
首先,模拟蚁群生态系统,构建了实现半导体生产线动态调度的MAS系统(SMAS)。
在该系统中,使用每个蚂蚁agent分别控制相应的工件、设备、运输工具与人员,将与调度相关的信息表示成相应的蚂蚁agent的信息素。
蚂蚁agent通过感知其他蚂蚁agent的信息素来确定自己下一步行为,即选择合适的设备等待加工或选择合适的工件进行加工,从而实现动态调度。
该算法有两方面的优势:一是将调度相关信息表示成蚂蚁agent的信息素后,可以根据要优化的性能指标,来相应地改变信息素的表示方式,对调度的结构却不发生影响,可以方便地实现方法的重用;二是决策时间短、计算量小、实时性好、易于实现,非常适用于动态调度。
外贸函电

复习题外贸英文函电l D/D WPA L/C CFR D/Al FOB D/P EMP FPA B/Ll感谢10月1日的电子邮件。
你们增长的销售额,是你们成功分销我产品的明证。
l我们认识到价格是维持、拓展市场的一个重要因素。
在不降低质量的前提下,我们一直努力降低成本。
实际上,我们的利润一直很低。
这就是我们不能再降低价格的原因。
但我们不会停止降低成本的努力。
l作为一个选择方案,可行的方法也许是提供一个低档的但是质量仍可接受的低价产品。
一些品种的说明书在附件中。
请告知你们对哪些产品有兴趣。
l你方通过Chartered银行开出的信用证已收到。
经仔细审核,我们发现其中有些条款与销售确认书不符,不符点如下:l 1.我们同意接受的价格术语是CFR而不是FOB。
l 2.应允许转运和分批装运。
l 3.数量前需加上“大约”的字样。
l请修改信用证并把装运期和信用证的有效期分别延展到九月底和十月十五号。
感谢你们的合作。
1l For the past five years we have been selling your Electronic Lighters to wholesalers and large dealers in all parts of Pakistan and our clients are very much satisfied with your products.The styles and colours are very much to the taste of our market.We have built up a considerable number of well-established connections with excellent business results.l As we understand that you have no agent in Pakistan,we would like to offer our services.l For your reference,we would propose a sole agency agreement for a duration of three years with annual turnover of50,000,60,000and70,000pieces for the first,second and third year respectively.On all sales,we are entitled to receive a commission of15%.During the validity of the agency agreement,we shall not handle any other foreign products of the same line and competitive types.The area to be covered by the agency agreement is confined to Pakistan.l If you agree to grant us the sole agency we will devote full attention to the development of your products on the Pakistan market and will go into the matter further.l We look forward to receiving your favourable reply.2l Thank you for your letter of the31st October in connection with sole agency.l In reply,we wish to state that we appreciate your efforts in pushing the sales of our Electronic Lighters in Pakistan and we are satisfied with your work in the past.However,after serious consideration,we do not think conditions are ripe to entrust you with the agency at the present stage as the sales volume mentioned in your letter under reply is too small for us to grant you the agency.To tell you frankly,the average annual quantity we sold to your country in the past few years is larger than what you mentioned.l In spite of this,please do not misinterpret our above remark,which in no way implies dissatisfaction.If a bigger turnover can be realized to justify establishing the agency we would like you to represent us.l We hope you will agree with on this point and continue placing orders with us as you have done so far.l 1.Such color deviation existing between the products and the samples is normal and permissible,so the compensation claimed is impracticable.l 2.Many banks in Hong Kong can issue Letters of Credit and effect payment in Renminbi.l 3.We would like to place an order for500sets“Lenova”brand Model262notebook computers at price of US$550each,CIF EMP,for shipment during July/August.l 4.It is in view of our long-standing business relationship that we accept your counter offer.l 5.Owing to the high expense for the opening of L/C here,we suggest that after shipment,you draw on us a sight draft for collection through your bank.l 6.I don’t think I can cut our price to that extent you required.Shall I suggest that we meet each other half way?l7.It will be appreciated if you will immediately let us have your firm offer for TV sets.l8.You should lodge a claim against either the carrier or the insurance company as the goods was damaged during transit.l9.Taking into consideration of our friendly business relations,we are of the opinion that if you could increase your order to8,000sets we would allow a5%discount.l10.They can not accept any fresh orders now because of heavy commitments.l 1.Marine insurance policy is required for110%of the invoice value against FPA and War Risk as per the People’s Insurance Co.of China.l 2.If a party does not pay a sum of money when it falls due the other party is entitled to interest upon that sum.l 3.The new packaging of this article is exquisitely designed and we are confident that it will appeal strongly to customers.l 4.In the absence of your definite instructions we will cover your goods against W.P.A and War Risk according to usual practice.l 5.We are responsible to replace the defective products.l 6.Considering the small amount involved,we are prepared,as an exception to make payment by D/P at sight for your first trial order.l7.If the goods are to be transshipped from one means of transportation to another during the course of the entire voyage,it’s called“combined transport”.l8.Because of the high cost of air transportation,we prefer sea transportation.l9.The cartons used for packing our sewing machines are light but strong,which save shipping space and facilitate the storage and distribution of the goods.l10.As your cargos are shipped all year around,we make it a rule to cover insurance under open cover.l很抱歉,我方不能接受你方的还盘,因为我方报给你方的价格是很实际的。
中科大管理学院主要教授论文专著一览

方兆本个人简介:方兆本, 1945年生,浙江金华人。
教授,博士生导师。
1986年获美国匹兹堡大学统计学博士。
1990年加拿大温哥华UBC大学访问讲学。
中国现场统计学会理事、中国概率统计学会理事,美国当代统计索引CIS通讯编辑,美国ASA、IMS会员。
曾任科大数学系主任,现任全国政协常委,安徽省政协副主席,民建安徽省主委,中国科技大学商学院院长。
在《概率纪事》、《统计纪事》、《多元分析》等杂志发表论文几十篇。
推广Hofffding引理被收入Cox著统计渐进技术。
对多维相依序、泊松过程刻画、非参数回归、金融工程等问题做出深入研究。
近年力主统计与经济、金融、保险的结合,创立了科大的保险精算教育,致力于金融工程人才培养,推进MBA、MPA教育。
专业方向:概率论与数理统计、概率统计所授课程:随机过程、概率论、数理统计、多元统计分析(专业课)、随机分析(专业课)科研项目:1.火灾风险评估方法学2.针对信用卡用户的信用评估与风险分级3.合肥市个人信用评分系统4.深圳市个人信用评分系统5.鹏华基金投资组合与风险管理控制系统6.香港恒生指数期权市场波动性之研究7.大数量普通股投资优化的Portfolio模型及其解8.不完全市场中有价证券组合的定价理论9.个人与企业信用评分模型设计10.信息技术在金融证券市场中的应用及高性能决策分析软件系统的研究论文著作:股市波动性预测模型改进研究(本文从参数估计准则和收益率波动性的定量表达这两方面来探讨股市收益的波动性预测改进方法,并由些提出了以收益率偏差绝对值为代替偏差平方并利用非线性最小二乘法来估计参数的(A)GARCH – NLS模型。
最后,我人以国泰君安指数、上证综指以及深证成指1998.1.5 – 2002.9.25的每日收盘价格为样本来考察各种合理替代所产生的模型对股市波动性的预测性能影响。
结果发现,(A)GARCH –NLS模型对股市波动性的预测精确度有了较显著的提高。
Multiagent systems
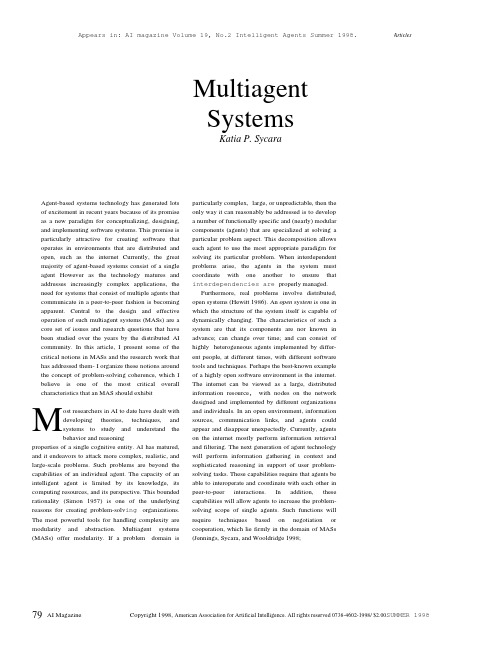
Appears in: AI magazine Volume 19, No.2 Intelligent Agents Summer 1998.ArticlesMultiagentystemsMThe characteristicsof MASs arethat(1) eachagent has incompleteinformation or capabilitiesfor solvingthe problemand, thus, has a limited viewpoint; (2)there is no system globalcontrol;(3) data are decentralized;and (4) computationis asynchronous. O’Hare and Jennings 1996; Bond and Gasser 1988).It is becoming increasingly clear that to be successful,increased research resources and attention should begiven to systems consisting of not one but multiple agents.The distributed AI (DAD community that started forming inthe early 1980s and was tiny compared to mainstream, single-agent AI is rapidly increasing. The growth of the MAS field isindisputable.Research in MASs is concerned with the study, behavior,and construction of a collection of possibly preexistingautonomous agents that interact with each other and theirenvironments. Study of such systems goes beyond the study ofindividual intelligence to consider, in addition, problemsolving that has social components. An MAS can be defined asa loosely coupled network of problem solvers that interact tosolve problems that are beyond the individual capabilities orknowledge of each problem solver (Durfee and Lesser 1989).These problem solvers, often ailed agent, are autonomous andcan be heterogeneous in nature.The characteristic of MASs are that (1) each agent hasincomplete information or capabilities for solving the problemand. thus, has a limited viewpoint; (2) there is no systemglobal control; (3) data are decentralized; and (4) computationis asynchronous. The motivations for the increasing interest inMAS research include the ability of MASs to do thefollowing:First is to solve problems that are too large for a centralizedagent to solve because of resource limitations or the sheer riskof having one centralized system that could be a performancebottleneck or could fail at critical times.Second is to allow for the interconnection andinteroperation of multiple existing legacy systems. To keeppace with changing businessneeds, legacy systems must periodically beupdated. Completely rewriting such software tends to beprohibitively expensive and is often simply impossible.Therefore, in the short to medium term, the only way that suchlegacy systems can remain useful is to incorporate them into awider cooperating agent community in which they can beexploited by other pieces of software. Incorporating legacysystems into an agent society can be done, for example, bybuilding an agent wrapper around the software to enable it tointeroperate with other systems (Genesereth and Ketchpel1994).Third is to provide solutions to problems that can naturally beregarded as a society of autonomous interacting components-agents.For example, in meeting scheduling a scheduling agent thatmanages the calendar of its user can be regarded asautonomous and interacting with other similar agentsthat manage calendars of different users (Garrido andSycara 1996; Dent et al. 1992). Such agents also canbe customized to reflect the preferences and constraintof their users. Other examples include air-trafficcontrol (Kinny et al 1992; Cammarata, McArthur, andSteeb 1983) and multiagent bargaining for buying andselling goods on the internetFourth is to provide solutions that efficiently useinformation sources that are spatially distributed.Examples of such domains include sensor networks(Corkill and Lesser 1983), seismic monitoring (Masonand Johnson 1989), and information gathering fromthe internet (Sycara et al. 1996).Fifth is to provide solutions in situations whereexpertise is distributed. Examples of such problemsinclude concurrent engineering (Lewis and Sycara1993), health care, and manufacturing.Sixth is to enhance performance along thedimensions of (1) computational efficiency becauseconcurrency of computation is exploited (as long ascommunication is kept minimal, for example, bytransmitting high-level information and results ratherthan low-level data); (2) reliability, that is, gracefulrecovery of component failures, because agents withredundant capabilities or appropriate interagentcoordination are found dynamically (for example,taking up responsibilities of agents that fail); (3)extensibility because the number and the capabilities ofagents working on a problem can be altered; (4)robustness, the system’s ability to tolerate uncertaintybecause suitable information is exchanged amongagents; (5) maintainability because a system composedof multiple components-agents is easier to maintainbecause of Its modularity; (6) responsiveness becausemodularity can handle anomalies locally, notpropagate them to the whole system; (7) flexibilitybecause agents with different abilities can adaptivelyorganize to solve the current problem; and(8) reuse because functionally specific agents can bereused in different agent teams to solve differentproblems.MASs are now a research reality and are rapidlyhaving a critical presence in many human-computerenvironments. My purpose in this article is not toprovide a detailed review of the field; I leave this taskto others (see, for example, Huhns and Singh [1997],O’Hare and Jennings [19961, Wooldridge andJennings [1995], Chaib-draa et al. [19921, and BondandAI MAGAZINEGasser [1988] for surveys). Rather than present an in-depth analysis and critique of the field, I instead briefly discuss some key topic and indicate how they are interrelated. Where appropriate, references to more detailed treatments are provided.Multiagent SystemIssues and ChallengesAlthough MASs provide many potential advantages, they also present many difficult challenges. Here, I present problems inherent in the design and implementation of MASs. The list of challenges includes problems first posed in Bond and Gasser (1988), but I have added some:First, how do we formulate, describe, decompose, and allocate problems and synthesize results among a group of intelligent agents?Second, how do we enable agents to communicate and interact? What communication languages and protocols do we use? How can heterogeneous agents interoperate? What and when can they communicate? How can we find useful agents in an open environment?Third, how do we ensure that agents act coherently in making decisions or taking action, accommodating the non-local effects of local decisions and avoiding harmful interactions? How do we ensure the MAS does not become resource bounded? How do we avoid unstable system behavior?Fourth, how do we enable individual agents to represent and reason about the actions, plans, and knowledge of other agents to coordinate with them; how do we reason about the state of their coordinated process (for example, initiation and completion)? Fifth, how do we recognize and reconcile disparate viewpoints and conflicting intentions among a collection of agents trying to coordinate their actions?Sixth, how do we engineer and constrain practical DAI systems? How do we design technology platforms and development methodologies for MASs?Solutions to these problems are intertwined (Gasser 1991). For example, different modeling schemes of an individual agent can constrain the range of effective coordination regimes; different procedures for communication and interaction have implications for behavioral coherence. Different problem and task decompositions can yield different interactions. It is arguable whether one can find a unique most important dimension along which a treatment of MASs can cogently be organized. Here, I attempt to use the dimension of effective overall problem-solving coherence of an MAS as the organizing theme.Ensuring that an MAS exhibits coherent collectivebehavior while it avoids unpredictable or harmfulbehavior (for example, chaos, oscillation) is indeed amajor challenge: By its very nature, an MAS lacksglobal perspective, global control, or global data.Coherence is a global (or regional) property of theMAS that could be measured by the efficiency, qualityand consistency of a global solution (system behavior)as well as the ability of the system to degradegracefully in the presence of local failures. Severalmethods for increasing coherence have been studied.These methods, along with issues of single-agentstructuring in an MAS, cover the topic I want tosurvey here.Individual Agent ReasoningSophisticated individual agent reasoning can increaseMAS coherence because each individual agent canreason about nonlocal effects of local actions, formexpectations of the behavior of others, or explain andpossibly repair conflicts and harmful interactions.Numerous works in AI research try to formalize alogical axiomatization for rational agents (seeWooldridge and Jennings (19951) for a survey). Thisaxiomatization is accomplished by formalizing amodel for agent behavior in terms of beliefs, desires,goals, and so on- These works are known as belief-desire-intention (BDI) systems (see Rao and Georgeff[1991] and Shoham (19931). An agent that has a BDI-type architecture has also been called deliberative.In my own work on the RETSINA multiagentinfrastructure, agents coordinate to gather informationin the context of user problem-solving tasks. EachRETSINA agent is a BDI-type agent that integratesplanning, scheduling, execution, informationgathering, and coordination with other agents (Decker,Pannu, et al. 1997; Sycara et at. 1996). Each agent hasa sophisticated reasoning architecture that consists ofdifferent modules that operate asynchronously.The planning module takes as input a set of goals andproduces a plan that satisfies the goals. The agentplanning process is based on a hierarchical tasknetwork (HTN) planning formalism. It takes as inputthe agents current set of goals, the current set of taskstructures, and a library of task-reduction schemas. Atask-reduction schema presents a way of carrying out atask by specifying a set of subtasks-actions anddescribing the information-flow relationships betweenthem. (See Williamson, Decker, and Sycara [19961]).The communication and coordination module acceptsand interprets messagesSophisticatedindividualagentreasoning canincrease MAScoherencebecause eachindividualagent canreason aboutnonlocal effectsof localactions,formexpectations ofthe behavior ofothers, orexplain andpossiblyrepair conflictsand harmfulinteractions.from other agents in KQML. Messages can contain requests for services. These requests become goals of the recipient agent. The scheduling module schedules each of the plan steps. The agent scheduling process takes as input, in general, the agents current set of plan instances and, in particular, the set of all executable actions and decides which action, if any, is to be executed next This action is then identified as a fixed intention until it is actually carried out (by the execution component). Agent-reactivity considerations are handled by the execution-monitoring process. Execution monitoring takes as input the agents next intended action and prepares, monitors, and completes its execution. The execution monitor prepares an action for execution by setting up a context (including the results of previous actions) for the action. It monitors the action by optionally providing the associated computation-limited resources--for example, the action might be allowed only a certain amount of time, and if the action does not complete before the time is up, the computation is interrupted, and the action is marked as having failed. Failed actions are handled by the exception-handling process. The agent has a domain-independent library of plan fragments (task structures) that are indexed by goals as well as a domain-specific library of plan fragments that can be retrieved and incrementally instantiated according to the current input parameters.Reactive agents have also been developed. Reactive agents have their roots in Brooks’s (1991) criticism of deliberative agents and his assertions that (1) intelligence is the product of the interaction of an agent and its environment and (2) intelligent behavior emerges from the interaction of various simpler behaviors organized in a layered way through a master-slave relationship of inhibition (subsumption archi-tecture). A different reactive architecture is based on considering the behavior of an agent as the result of competing entities trying to get control over the actions of the agent This idea has its root in the society of the mind (Minsky 1986) and has been employed in different ways by Maes (1990), Travers (1988), and Drogul and Ferber (Ferber 1996; Drogul and Ferber 1992). An agent is defined as a set of conflicting tasks where only one can be active simultaneously. A task is a high-level behavioral sequence as opposed to the low-level actions performed directly by actuators. A reinforcement mechanism is used as a basic learning tool to allow the agents to learn to be more efficient in tasks that are often used. This architecture has been used in the MANTA system to simulate the behavior of ants (Ferber 1996).Reactive agents do not have representations of their environment and act using a stimulus response type of behavior they respond to the present state of the environment in which they are situated. They do not take history into account or plan for the future. Through simple interactions with other agents, complex global behavior can emerge. This characteristic is a strength of the approach because theagents do not need to revise their world model as itchanges. Thus, robustness and fault tolerance are twoof the main properties of reactive systems. A group ofagents can complete a task even if one of them fails.However, purely reactive systems suffer from twomain limitations:First, because purely reactive agents make decisionsbased on local information, they cannot take intoconsideration non-local information or predict theeffect of their decisions on global behavior Suchmyopic behavior could lead to unpredictable andunstable system behavior (Thomas and Sycara 1998;Huberman and Hogg 1988). In reactive systems, therelationship between individual behaviors, environ-ment, and overall behavior is not understandable,which necessarily makes it hard to engineer agents tofulfill specific tasks: One must use a laborious processof experimentation, trial, and error to engineer an agentor an MAS. Despite these disadvantages, reactive sys-tems have advantages of speed (the sophisticatedreasoning of deliberative agents can slow them; hence,they are useful in rapidly changing environments).In fact, for most problems, neither a purely deliberativenor a purely reactive architecture is appropriate, buthybrid architectures can combine aspects of both.Typically, these architectures are realized as a numberof software layers, each dealing with a different levelof abstraction. Most architectures find three layerssufficient. Thus, at the lowest level in the hierarchy,there is typically a reactive layer, which makesdecisions about what to do based on raw sensor inputThe middle layer typically abstracts away from rawsensor input and deals with a knowledge-level view ofthe agents environment, typically making use ofsymbolic representations. The uppermost level of thearchitecture tends to deal with the social aspects of theenvironment. Coordination with other agents istypically represented in the uppermost layer .The waythat the layers interact with one another to produce theglobal behavior of the agent differs from architectureto architecture (Bonasso et at. 1996; Ferguson 1995,1992; Muller and Pischel 1994).The area of agent architectures, particularly layeredarchitectures, continues to be an areaReactive agentsdo not haverepresentationsof theirenvironmentand act using astimulus-response typeof behavior;they respond tothe presentstate of theenvironment inwhich they aresituated.Articlesof considerable research effort within the multiagent field. For example, there is ongoing work to investigate the appropriateness of var-ious architectures for different environment types. It turns out to be hard to evaluate one agent architecture against another, especially within the context of an MAS.OrganizationsAn organization provides a framework for agent interactions through the definition of roles, behavior expectations, and authority relations. Organizations are, in general, conceptualized in terms of their structure, that is, the pattern of information and control relations that exist among agents and the distribution of problem -solving capabilities among them. In cooperative problem solving, for example (Corkill and Lesser 1983), a structure gives each agent a high level view of how the group solves problems. The structure should also indicate the connec-tivity information to the agents so they can distribute subproblems to competent agents.In open-world environments, agents in the system are not statically predefined but can dynamically enter and exit an organization, which necessitates mechanisms for agent locat-ing. This task is challenging, especially in envi-ronments that include large numbers of agents and that have information sources, communi-cation links, and/or agents that might be appearing and disappearing. Researchers have identified different kinds of middle agent (Decker, Sycara, and Williamson 1997) that help agents find others. When an agent is instantiated, it advertises its capabilities to a middle agent An agent that is looking to find another that possesses a particular capability (for example, can supply particular information or achieve a problem-solving goal) can query a middle agent In the RETSINA infrastructure, there could be multiple middle agents, not only in type but also in number For example, protocols have been developed for distributed matchmaking (Jha et at. 1998). Another perspective in DAI defines organiza-tion less in terms of structure and more interms of current organization theory. Forexample, Gasser (1986) views an organizationas a "particular set of settled and unsettledquestions about beliefs and actions throughwhich agents view other agents." In this view,an organization is defined as a set of agentswith mutual commitments, global commit-ments, and mutual beliefs (Bond and Gasser1988). An organization consists of a group ofagents, a set of activities performed by theagents, a set of connections among agents, anda set of goals or evaluation criteria by whichthe combined activities of the agents are eval-uated. The organizational structure imposesconstraints on the ways the agentscommunicate and coordinate. Examples oforganizations that have been explored in theMAS literature include the following:Hierarchy: The authority for decision-making and control is concentrated in a singleproblem solver (or specialized group) at eachlevel in the hierarchy. Interaction is throughvertical communication from superior to sub-ordinate agent, and vice versa. Superior agentsexercise control over resources and decision-making.Community of experts: This organization isflat, where each problem solver is a specialistin some particular area. The agents interact byrules of order and behavior (Lewis and Sycara1993; Lander, Lesser, and Connell 1991).Agents coordinate though mutual adjustmentof their solutions so that overall coherence canbe achieved.Market: Control is distributed to the agentsthat compete for tasks or resources throughbidding and contractual mechanisms. Agentsinteract through one variable, price, which isused to value services (Mullen and Wellman1996; Davis and Smith 1983; Sandholm 1993).Agents coordinate through mutual adjustmentof prices.Scientific community: This is a model ofhow a pluralistic community could operate(Kornfeld and Hewitt 1981). Solutions toproblems are locally constructed, then they arecommunicated to other problem solvers thatcan test, challenge, and refine the solution(Lesser 1991).In open, dynamic environments, the issueof organizational adaptivity is crucial.Organizations that can adapt to changingcircumstances by altering the pattern ofinteractions among the different constituentagents have the potential to achieve coherencein changing and open environments. RETSINAexhibits organizational adaptivity throughcooperation mediated by middle agents.RETSINA agents find their collaboratorsdynamically based on the requirements of thetask and on which agents are part of thesociety at any given time, thus adaptivelyforming teams on demand (Decker,Williamson, and Sycara 1996a).Organizations are, in general,conceptualized in terms oftheir structure, that is, thepattern of informationand control relationsthat exist among agents andthe distribution of problem-solving capabilities amongthem.Task AllocationTask allocation is the problem of assigningresponsibility and problem-solving resourcesto an agent. Minimizing task interdependen-cies has two general benefits regarding coher-ence: First, it improves problem-solving effi-ciency by decreasing communication overheadSUMMER 1998among the problem-solving agents. Second, it improves the chances for solution consistency by minimizing potential conflicts. In the second case, it also improves efficiency because resolving conflicts can be a time-consuming process.The issue of task allocation was one of the earliest problems to be worked on in DAI research. On the one extreme, the designer can make all the task assignments in advance, thus creating a nonadaptive problem-solving organization. This approach is limiting and inflexible for environments with a high degree of dynamism, openness, and uncertainty. However, one can do task allocation dynamically and flexibly. The issue of flexible allocation of tasks to multiple agents received attention early on (Davis and Smith 1983). Davis and Smith’s work resulted in the well-known CONTRACT NET PROTOCOL (CNP). In this protocol, agents can dynamically take two roles: (1) manager or (2) contractor. Given a task to perform, an agent first deter-mines whether it can break it into sub-tasks that can be performed concurrently. It utilizes the protocol to announce the tasks that could be transferred and requests bids from agents that could perform any of these tasks. An agent that receives a task-announcement message replies with a bid for the task, indicating how well it thinks it can perform the task. The contractor collects the bids and awards the task to the best bidder. The CNP allows nodes to broadcast bid requests to all others. The CNP was sub-sequently used to control factory floor operations (Parunak 1987). AIthough CNP was considered by Smith and Davis and many DAI researchers to be a negotiation principle, it is a coordination method for task allocation, CNP enables dynamic task allocation, allows agents to bid for multiple tasks at a time, and provides natural load balancing (busy agents need not bid). Its limitations are that It does not detect or resolve conflicts, the manager does not inform nodes whose bids have been refused, agents cannot refuse bids, there is no preemption in task execution (time-critical tasks might not be attended to), and it is communication intensive. Extensionsto CNP have been made by Sandholm and Lesser (1995), where decommitmentpenalties were introduced, and by Sycara(1997), where the theory of financial optionpricing has been used to achieve flexiblecontracting schemes in uncertainenvironment.Multiagent PlanningAgents can improve coherence by planningtheir actions. Planning for a single agent is aprocess of constructing a sequence of actionsconsidering only goals, capabilities, andenvironmental constraints. However, plan-ning in an MAS environment also considersthe constraints that the other agents’ activitiesplace on an agent’s choice of actions, theconstraints that an agent’s commitments toothers place on its own choice of actions,and the unpredictable evolution of the worldcaused by other unmodeled agents.Most early work in DAI has dealt withgroups of agents pursuing common goals(for example, Lesser [1991); Lesser, Durfee,and Corkill [1989]; Durfee [19881; Conry,Meyer and Lesser [19881; Cammaarata,McArthur, and Steeb [19831). Agentinteractions are guided by cooperationstrategies meant to improve their collectiveperformance. Most work on multiagentplanning assumes an individual sophisticatedagent architecture that enables them to dorather complex reasoning. Early work ondistributed planning took the approach ofcomplete planning before action. To producea coherent plan, the agents must be able torecognize subgoal interactions and avoidthem or resolve them. Early work byGeorgeff (Rao and Georgeff 1991) includeda static agent to recognize and resolve suchinteractions. The agents sent this agent theirplan; the agent examined them for criticalregions where, for example, contention forresources could cause them to fail. The agentthen inserted synchronization messages (akinto operating system semaphores) so that oneagent would wait till the resource wasreleased by another agent In the work byCammarata on air-traffic control (Steeb et al.1988; Cammarata, McArthur, and Steeb1983), the synchronizing agent wasdynamically assigned according to differentcriteria, and it could alter its p1w to removethe interaction (avoid collision). Anotherapproach to resolving subprobleminterdependencies that result from anincomplete set of data for each agent thatcould allow it to solve a subproblem terncompletely is theFUNCTIONALLY ACCURATE MODEL (FA/C)(Lesser 1991). In the PA/C model, agents donot need to have all the necessary informationlocally to solve their subproblems but insteadinteract through the asynchronous, coroutineexchange of partial results. With the FA/Cmodel, a series of sophisticated distributedcontrol schemes for agent coordination weredeveloped, such as the use of static metalevelinformation specified by an organizationalstructure and the use of dynamic metalevelinformation developed in partial globalplanning (PGP) (Durfee 1987).POP is a flexible approach to coordinationthat does not assume any particulardistribution of subproblems, expertise, orother resources but, instead, lets nodescoordinate in response to current situations(Durfee 1987)- Agent interactions take theform of communicating plans and goals at anappropriate level of abstraction. Thesecommunications enable a receiving agent toform expectations about the future behaviorof a sending agent, thus improving agentpredictability and network coherence (Durfee1988). Because agents are cooperative, therecipient agent uses the information in theplan to adjust its own local planning appro-priately, so that the common planning goals(and planning effectiveness criteria) are metBesides their common POPs, agents alsohave some common knowledge about howand when to use POPs. Decker and Lesser(1995) addressed some of the limitations ofthe POP by creating a generic POP-basedframework called TAEMS to handle issues ofreal time (for example, scheduling todeadlines) and metacontrol (for example, toobviate the need to do detailed planning at allpossible node interactions). TAUV45 has beenused as a framework for evaluation ofcoordination algorithms.Another direction of research incooperative multiagent planning has。
常用国际贸易术语
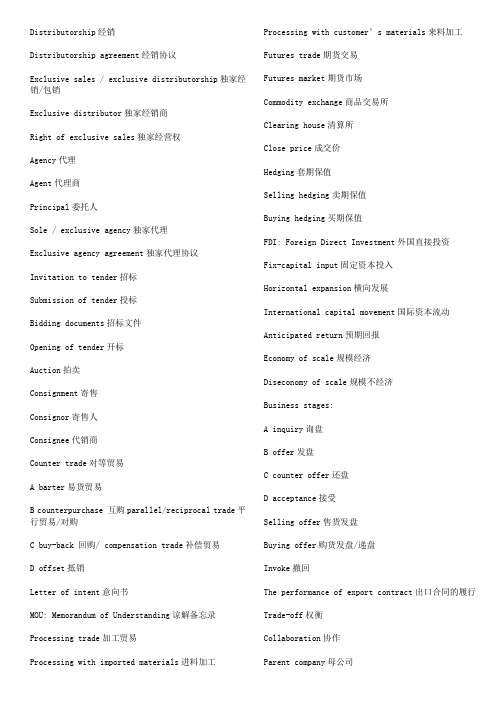
Distributorship经销Distributorship agreement经销协议Exclusive sales / exclusive distributorship独家经销/包销Exclusive distributor独家经销商Right of exclusive sales独家经营权Agency代理Agent代理商Principal委托人Sole / exclusive agency独家代理Exclusive agency agreement独家代理协议Invitation to tender招标Submission of tender投标Bidding documents招标文件Opening of tender开标Auction拍卖Consignment寄售Consignor寄售人Consignee代销商Counter trade对等贸易A barter易货贸易B counterpurchase 互购parallel/reciprocal trade平行贸易/对购C buy-back 回购/ compensation trade补偿贸易D offset抵销Letter of intent意向书MOU: Memorandum of Understanding谅解备忘录Processing trade加工贸易Processing with imported materials进料加工Processing with customer’s materials来料加工Futures trade期货交易Futures market期货市场Commodity exchange商品交易所Clearing house清算所Close price成交价Hedging套期保值Selling hedging卖期保值Buying hedging买期保值FDI: Foreign Direct Investment外国直接投资Fix-capital input固定资本投入Horizontal expansion横向发展International capital movement国际资本流动Anticipated return预期回报Economy of scale规模经济Diseconomy of scale规模不经济Business stages:A inquiry询盘B offer发盘C counter offer还盘D acceptance接受Selling offer售货发盘Buying offer购货发盘/递盘Invoke撤回The performance of export contract出口合同的履行Trade-off权衡Collaboration协作Parent company母公司Subsidiary子公司Licensor许可方Licensee被许可方Corporate family公司群Proprietary assets专利资产Operational form经营形式Line/scope of business经营范围Royalty许可使用费Master franchise特约总经销Cross-licensing相互特约经销Franchisee特约经销接受方Franchisor特约经销授权方Vertically integrated company纵向一体化公司Intangible property无形资产Licensing agreement许可协议Sales representative销售代表End user直接用户Overseas agent海外代理Credit investigation资信调查Potential pitfall潜在危险Global concentration面向世界Freight forwarder货运代理Sales record销售记录Piggyback export挂靠才出口Direct selling直销Production capacity生产能力Entry mode切入方式Export revenue出口收入Promotional campaign促销活动Essence of export出口本质Commission agent佣金代理商Export-import strategy进出口策略Commercial invoice商业发票B/L: Bill of Lading提单Consular invoice领事发票Certificate of origin原产地证书Export packing list出口装箱单Settlement of the payment 货款结算Payment currency支付货币Depreciate / Appreciate贬/升值Evaluation currency估价货币Convertible currency可转化货币Hard / Soft currency硬币/软币Operative Instruments:有效票据A. Bill of Exchange; Bill / Draft汇票B. Promissory note本票C. Check支票Banker’s draft银行汇票Commercial draft商业汇票Clean draft光票汇票Documentary draft跟单汇票Sight / Demand draft即期汇票Time / Usance draft远期汇票Commercial acceptance draft商业承兑汇票Banker’s acceptance draft银行承兑汇票Processes to handle a draft:A. to draw出票B. to present提示C. to accept承兑D. to pay支付Drawer出票人Drawee受票人Parties当事人Tenor期限Days of grace宽限期Prior party前手Subsequent party后手Dishonour拒付Recourse追索Face value票面价值Debtor债权人Bona fide holder善意持有人Notary public公证人General promissory note一般本票Banker’s promissory note or cashier’s order银行本票Depositor储户Falsely claim冒领Initial / Counter signature初/复签Crossed check划线支票Payment Methods:A. remittance汇付B. collection托收C letter of credit信用证Favourable exchange顺汇Adverse exchange逆汇Bank / Commercial credit银行/商业信用Remittance:A. T / T : telegraphic transfer电汇B. M / T: mail transfer信汇C. D / D: ( remittance by banker’s ) demand draft 票汇Remitter汇款人Remitting bank汇出行Receiving / Paying bank汇入行/解付行Type of remittance汇款方式Remittance fee汇费Cable confirmation电汇证实书Debit advice 付讫借记通知Payment order支付通知书C.W.O.: cash with order随订单付现O / A trade: open account trade赊帐贸易C.O.D.: Cash on delivery交货后付款Payment in advance预付货款Remittance against documents凭单付汇Draw a bill of exchange on向…开汇票Financial documents金融单据Commercial documents商业单据Documentary collection跟单托收Clean collection光票托收Payment balance货款尾数/余额Remitting bank托收银行Collecting bank代收行Presenting bank提示行Documentary Collection:跟单托收A. D / P: documents against payment付款交单B. D / A: documents against acceptance丞兑交单D / P at sight即期付款交单D / P after sight远期付款交单T/R: trust receipt信托收据Factoring保理(国际保付代理业务)Applicant / Opener开证申请人/开证人Opening / Issuing bank开证行Advising / Notifying bank通知行Beneficiary受益人Negotiating bank议付行Paying bank付款行Confirming bank保兑行Paying bank agent代付行Reimbursing bank偿付行Clearing bank信用证清算银行Transferring bank转让行L/G: banker’s letter of gurantee银行保函/银行保证书Varieties of L/C:A. documentary L/C跟单信用证B. clean L/C光票信用证C. revocable L/C可撤销信用证D. irrevocable L/C不可撤销信用证E. confirmed L/C保兑信用证F. unconfirmed L/C非保兑信用证G. sight payment L/C即期付款信用证H. deferred payment L/C延期付款信用证I. acceptance L/C承兑信用证K. negotiation L/C议付信用证L. sight L/C即期信用证M. time/usance L/C远期信用证N. transferable L/C可转让信用证O. untransferable L/C不可转让信用证Q. revolving L/C循环信用证R. reciprocal L/C对开信用证S. back-to-back L/C对背信用证T. anticipatory L/C (Red clause L/C)预支信用证/红条款信用证U. local L/C本地信用证V.standby L/C备用信用证Insurer保险人Insured投保人Insurance policy保险单Subject matter insured保险标的Insured amount保险金额Premium保险费Basic principles of insurance:A. insurable interest保险利益B. utmost good faith最大诚信C. principle of indemnity补偿原则D. proximate cause近因原则Risks:A. perils of the sea海上风险B. extraneous risks外来风险Losses损失:A. Total Loss全部损失/全损B Partial Loss部分损失General average contribution共同海损分摊Expenses:A sue and labour expenses施救费用B salvage charges救助费用PICC: People’s Insurance Company of China中国人民保险公司C.I.C.: China Insurance Clause中国保险条款I.C.C.: Institute Cargo Clause协会货物条款Basic Risks Coverage:A F.P.A: Free From Particular Average平安险B W.P.A.: With Particular Average水渍险C A.R.: All Risks一切险W/W clause: Warehouse to Warehouse Clause仓至仓条款General Additional Risks:A. clash and breakage碰损、破碎险B. taint of odour串味险C. fresh water and / or rain damage淡水雨淋险D. theft, pilferage and non-delivery: T.P.N.D.偷窃、提货不着险E. shortage短量险F. leakage渗漏险G. intermixture and contamination混杂、玷污险H. hook damage钩损险I. sweat and heating受潮收热险K. rust锈损险L. breakage of packing包装破裂险Special Additional Risks:A. war risk战争险B. strike risk罢工险C. aflatoxin黄曲霉素险D. failure to deliver交货不到险E. on deck risk舱面险F. import duty risk进口关税险G. rejection拒收险H fire risk extension clause (F.R.E.C.)—for storage of cargo at destination of Hong Kong including Kowloon or Macao.货物出口到香港(包括九龙)或澳门存仓火险责任扩展条款Insurance Documents;A. insurance policy保险单/大保单B. insurance certificate保险凭证C. combined certificate联合凭证D. open policy预约保单E. endorsement批单SRCC: strike, riots and civil commotions罢工、暴动、民变险I.O.P.: irrespective of percentage不论损失程度Claim settlement理赔Insurable value保险价值Franchise免赔率Insurance: To be covered by the Seller for 110% of the total invoice value against All Risks, as per and subject to the relevant ocean marine cargo clauses of the People’s Insurance Company of China, dated January1, 1981.保险由卖方按发票金额的110%投保一切险,以中国人民保险公司1981年1月1日有关海洋运输货物保险条款为准。
跟外国人谈生意你一定要懂得重点词组!
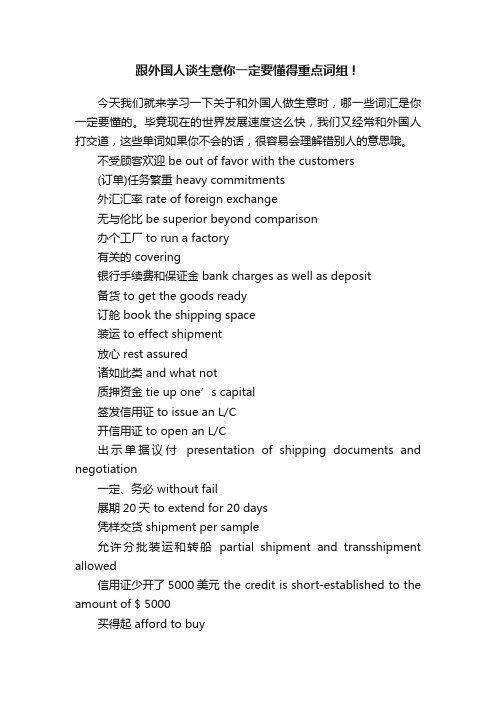
跟外国人谈生意你一定要懂得重点词组!今天我们就来学习一下关于和外国人做生意时,哪一些词汇是你一定要懂的。
毕竟现在的世界发展速度这么快,我们又经常和外国人打交道,这些单词如果你不会的话,很容易会理解错别人的意思哦。
不受顾客欢迎 be out of favor with the customers(订单)任务繁重 heavy commitments外汇汇率 rate of foreign exchange无与伦比 be superior beyond comparison办个工厂 to run a factory有关的 covering银行手续费和保证金 bank charges as well as deposit备货 to get the goods ready订舱 book the shipping space装运 to effect shipment放心 rest assured诸如此类 and what not质押资金 tie up one’s capital签发信用证 to issue an L/C开信用证 to open an L/C出示单据议付presentation of shipping documents and negotiation一定、务必 without fail展期20天 to extend for 20 days凭样交货 shipment per sample允许分批装运和转船partial shipment and transshipment allowed信用证少开了5000美元 the credit is short-established to the amount of $ 5000买得起 afford to buy装船延期 to postpone the shipmen t尽力 to exert oneself及时交货 timely delivery着手发运货物 set about making delivery在有效期内 be within validity撤盘 to withdraw the offer金融状况 financial status装船通知 shipping advice装船须知 shipping instruction超过船舷 pass over the rail一劳永逸地告诉你 to tell you once and for all 活到老学到老 one lives to learn租船 to charter a ship承担费用 to bear charges履行责任 to discharge/fulfill responsibilities 装运港 loading port关税 custom duty合同号 contract number外行 layman或者说 or rather货物吊上船 the goods on the hook拍卖这一库存 to sell off the stock海关手续 custom formalities亏本 lose out按严格程序 be in strict rotation提前装运 to advance shipment确保准时装运 to ensure punctual shipment 交易量 volume of trade(工人)上三班 work three shifts在手的过期未交货订单 back orders打退堂鼓 to back out忙季 busy season旺季 high season萧条季节 slack season销售季节 shopping season上市、旺季 be in season下市、淡季 be out of season木箱 wooden case纸盒 cardboard box关税配额 custom quota海关放行 custom clearance海关官员 custom officer安排装船 arrange shipment加速装船 expedite shipment延期装船 extend shipment提前装船 advance shipment延迟装船 postpone shipment期货 future舱位订满 the shipping space has been booked up直达轮 direct steamer增加货物被盗或损坏的风险add to the risks of pilferage and damage修改信用证 to amend L/C整批装运 ship the whole lot at one time提出建议 to put forth a proposal急需这批货 be in urgent need of the goods在途中 be in transit信用证条款与合同条款严格一致 the stipulations in the L/C are in strict conformity with that of the contract目的港 port of destination聚乙烯包装物 polythene wrapper橱窗展览 window show立足市场 find a footing in the market赶时髦 to keep up with fashion式样新奇、时尚 novel and fashionable design颜色鲜亮 bright color迎合顾客口味to meet customers’ taste按照 as per携带轻便 light to handle保证安全 to ensure safety为……而感谢 be obliged for分公司 branch office小圆桶 keg麻袋 gunny bag袋sack听(容器)tin铁皮圆桶 drum茶叶箱 chest捆 bundle琵琶桶 barrel包装费用 packing charges装箱单 packing list包装指示 packing instructions出口包装 export packing内包装 inner packing外包装 outer packing中性包装 neutral packing适合海运的包装seaworthy packing/packing suitable for sea voyage远洋运输 ocean transportation瓦楞硬纸板箱 three-layer cardboard boxes抢眼又抢钱 catch the eyes as well as the purse平安险 From Particular Average水渍险 With Particular Average一切险 All Risks额外保险 extra premium偷窃、提货不着险risk of Theft, Pilferage and Non-Delivery (TPND)破碎险 risk of Breakage油渍险 risk of Oil渗漏险 risk of Leakage玷污险 risk of Contamination罢工暴动、民变险 risk of Strike, Riot and Civil Commotion淡水、雨淋险 risk of Fresh Water Damage提出索赔 to lodge a claim由某人付款for one’s account商赞处Commercial Counselor’s Office按发票金额 at the invoice value检验报告 a survey report独家代理 sole agency短交 short delivery猪鬃 bristle有广泛的业务联系have a wide connection with/be closely connected with/ do considerable business with/ have close contact with/ be in close contact with销售代理人 sales agent/selling agent采购代理人 purchasing agent/buying agent独家代理人 exclusive agent/sole agent佣金代理人 commission agent广告代理人 advertising agent代理协议 agency agreement年成交量 annual turnover不遗余力 spare no effort生意兴隆 a prosperous business在这一方面 in this connection红豆 Red Bean销售条款 sales terms销售合同 sales contract销售额 sales turnover销售代表 sales representative市场活跃 market active市场呆滞market dull市价下跌 market declining行市坚挺 market firm行市疲软 market easy市况平稳 market stable市况坚挺 market strong市况不明 market uncertain市场疲软 market weak控制市场 to control the market稳定物价 to stabilize the price加倍努力 double your efforts看透心事read one’s mind国内需求会急剧上涨 the domestic demand will go up sharply 定个时间 fix the time纺织品 textile电器产品 electricity products化工产品 chemicals达成协议 come to terms with增添和删除 addition and deletion市场下跌(熊市) with the market being bear会议纪要 meeting minutes附件 accessory中国国际贸易促进委员会 CCPIT仲裁委员会 Arbitration Commission求助于仲裁 resort to arbitration享有很高声誉 enjoy a high prestige仲裁裁决 arbitral award完好无损 sound and intact中国商检局 China Commodity of Inspection符合出口标准 be up to the standard of export优质干蘑菇 well-dried mushrooms of fine quality向…...要求赔偿to approach …. For compensation当场 on the spot证明 certify/verify/testify在途中 during transit/en route/on the way提出索赔to lodge/make/raise/issue/file/register/put in/ bring up a claim短重 short weight就货进行索赔 claim on goods就损坏索赔 claim for damage就数量索赔 claim for amount向某人索赔 claim with/against somebody接受索赔 to accept a claim受理索赔 to entertain a claim同意索赔 to admit a claim驳回索赔 to dismiss a claim拒绝索赔 to reject/decline/turn down a claim解决索赔 to settle a claim放弃索赔 to waive a claim撤回索赔 to withdraw a claim提单清洁的 Bill of Lading is clean剪样 cutting权威部门authorized department合同中写明的 specified in the contract接受索赔 to entertain a claim不可挽回的损失 get retrievably lost检验费 inspection fee理赔方面公平合理 be prompt and equitable in settling claims 澄清问题 get the matter cleared up货物发霉不能食用the contents are moldy and unfit for consumption密封 airtight塑料薄膜口袋 plastic bag迅速发货 immediate/rush dispatch of the goods董事会 board of directors为……铺平道路 pave the way for友善的洽谈 amiable negotiation留下深刻鲜明的印象leave a deep and vivid impression on somebody主持会议 to preside over a meeting扩音器 public address system再见,一路顺风(法)Bon Voyage检票 check in与国际接轨 bring in line with the international practice登舱 go on board世界贸易组织 World Trade Organization (WTO)。
- 1、下载文档前请自行甄别文档内容的完整性,平台不提供额外的编辑、内容补充、找答案等附加服务。
- 2、"仅部分预览"的文档,不可在线预览部分如存在完整性等问题,可反馈申请退款(可完整预览的文档不适用该条件!)。
- 3、如文档侵犯您的权益,请联系客服反馈,我们会尽快为您处理(人工客服工作时间:9:00-18:30)。
Multi Agent System for Negotiation and Decision Support Zoltán BALOGH, Michal LACLAVÍK, Ladislav HLUCHÝInstitute of Informatics, Slovak Academy of Sciences, Bratislavabalogh.ui@savba.sk, laclavik.ui@savba.sk, hluchy.ui@savba.skhttp://ups.savba.sk/AbstractIn this paper we analyse multi-agent systems. Recommendation of techniques for negotiation and decision support of mobile agents are presented. We support our theory by providing a simple example of an intelligent multi-agent negotiation and decision making system. Example is developed by Java IBM Aglet multi-agent system.1IntroductionAgent-based systems technology has become a new paradigm for conceptualizing, designing, and implementing software systems. Agents are sophisticated computer programs that act autonomously on behalf of their users, across open and distributed environments, to solve a growing number of complex problems. Increasingly, however, applications require multiple agents that can work together. A Multi-Agent System (MAS) is a loosely coupled network of software agents that interact to solve problems that are beyond the individual capacities or knowledge of each problem solver [11]. Mobile agents are a beneficial technology for the creation of distributed systems. The reasons supporting this statement, are as follows [1]:Agents reduce the network load. Mobile agents allow to package a conversationand dispatch it to a destination host,where the interaction can take placelocally.Agents overcome network latency.Mobile agents offer a solution to problem of unacceptable latencies incritical real-time systems, because theycan be dispatched from a central controller to act locally and directlyexecute the controller’s directions. Agents encapsulate protocols.Communication protocols often becomea legacy problem. Mobile agents canmove to remote hosts to establish channels based on proprietary protocols.Agents execute asynchronously and autonomously. Mobile devices must often rely on expensive or fragile network connections. Embedding tasksinto mobile agents, which can then bedispatched into the network, solves thisproblem.Agents adopt dynamically, by the ability to sense their environment and reactautonomously to changes.Agents are naturally heterogenous.Because mobile agents are generally dependent only on their execution environment, they provide optimal conditions for system integration.Agents are robust and fault-tolerant, because of their ability to react dynamically to unfavorable situations and events.2Research Infrastructure for the ModelThere are many infrastructures for developing more or less complex distributed multiagent systems. The Aglets Software Development Kit (SDK) is an environmentfor programming mobile autonomous Internet agents in Java. An Aglet (Light Weight Agent) is a Java object that can move from one host on the network to another. That is, an aglet that executes on one host can suddenly halt execution, dispatch to a remote host, and resume execution there. When the aglet moves, it takes along its program code as well as its state (data). A built-in security mechanism makes it safe for a computer to host untrusted aglets [14].For implementing the model proposed in this article we chose IBM’s Aglets SDK. The ASDK uses Java to implement agents. The following facts suspected Java as an ideal programming language suitable for our purposes:Java supports secure migration ofclasses.Interfaces to systems “speaking”KQML are available for Java.Java is platform independent.SQL interfaces to databases areimplemented.There are developmentenvironments available for Java.3Negotiation and Decision Support 3.1Present Stage of NegotiationNegotiation theory was first used in game theory. Negotiation in game theory is different from real life. The game software does not have to learn to make good decisions. The game has already all needed information for decision making. Still in game theory, we can find two good ideas: In game theory, agents have knowledge of other agent negotiationand decision making algorithm.Game agents have all needed information for negotiating on acertain place and they can usecommon knowledge instead of itsown.Auctioning is most popular and mostly used negotiation strategy. Usually agents are auctioning on goods or services. User creates agent with certain conditions, values, and bidding strategies and agent is bidding in auction instead of the user.In this paper, we present Cut cake algorithm that can be also used as Auctioning algorithm with some modifications. Nomad Auctioning System or site can be quoted as good examples of these systems.Negotiation Agents can be also usedto improve regular person-to-person negotiation. InterNeg project is good example. [16]. These way agents can be usedto search, sort, choose, or recommend some information, solution, but final decision isleft to the users. Negotiation does not haveto end-up with rejecting or accepting. The negotiation result can be data for next negotiation. In this case, agents work as a good secretary.Communication is always negotiating. All of multi-agent systems haveto use kind of communication-negotiation protocol. Negotiation needs some communication protocol for agents to understand each other. There are many communications languages and protocols. Many agents systems are building its own ones. KQML standard is promissing technology in this area.Agents are promising technology for Market Places. The most important role is role of negotiation, which is taking place of people negotiation when buying and selling goods. Several projects of Market Places have been developed. One of them KABASH, [15] is a multi-agent electronic marketplace that proposes to transform the online shopping experience by leveragingthe unique features of the Internet and software agent technologies. Aglets MarketPlace is newer market place[14]. It is a based on Aglets mobile agent technology.Possible negotiation problems and solutions:Decision MakingLearningCoordination – cooperation, movingfrom place to placeNegotiation – cooperation,competitionSimilarity to human negotiationFair and envy-freeAgent Negotiation is always based on conditions (price > offer, time = time of session etc.). Real life negotiation is not always like this. For negotiating strategies non-condition decision making is required. We could possibly use neural networks or some genetic based algorithms. Here we cansee the problem of storing and sorting theinformation. It is very hard for an agent tolearn as neural networks if it has to carry theinformation with itself. It is better to createsome negotiation-decision centers. The agentthen passes only its conditions and states andthe negotiation center will provide thenegotiation. Fair negotiation is also a problem. Itcan be solved by knowledge negotiatingalgorithm of other agent. In the negotiatingcenter, this can be solved by increasingknowledge of center by each negotiating.Envy-free and also fair is the Cut Cakealgorithm, which is also described below.Cooperative negotiation – cooperation on projects, meetings, etc.Goals: - we know here that we can give our(e.g. Timetable data) data to other agent andhe will give ours. Agents will agree on some time if possible. If we have some profit function, agents can put profit function together – the best solution is found. Non-cooperative negotiation:Problems with same conditions of profit forall negotiators.3.2 Decision Making and RationalityAccording to Doyle [5] judgements of human rationality commonly involveseveral different conceptions of rationality,including a logical conception used to judgethoughts, and an economic one used to judgeactions or choices. Intelligence involves bothperception and action. In most cases, actionsare not determined by the agent‘s situation,but instead involve choices to do one thingrather than another. Thus, both thinking andchoice are central operations in thinking.The fundamental issue in the theory of economic rationality is choice among alternatives. Economic rationality means making …good“ choices, where goodness is determined by how well choices accord with the agent‘s preferences among alternatives. Preferences Preference is the fundamental concept of economic rationality. We write B A S to mean that agent prefers B to A , and A ~ B to mean that the agent is indifferent between the two alternatives. We also write B A S to mean that B A S does not hold and B A S that either A ~ B or B A S . The collection of all these comparisonsconstitutes the agent‘s set of preferences.These preferences may change over time.Rational agents choose maximally preferredalternatives. If },...,,{21n A A A =α is the set of alternatives, then A i is a rational choice from among these alternatives just in case ji A A S for every A j ∈ α.. There may beseveral rational choices, or none at all if the set of preferences is inconsistent or if the set of alternatives is empty or infinite. The theory does not require that a rational agent explicitly calculates or computes the maximality of its choices, only that the agent chooses alternatives that are infact maximal according to its preferences. The theory requires, as a minimum basis for rationality, that strict preference is a strict partial order, indifference is an equivalence~ ~relation (transitive and asymmetric), and any two alternatives are either indifferent or one is preferred to the other, but not both at the same time. These separate requirements on preference and indifference may be put formally, for all alternatives A , B and C :1. Either B A S or A B S , (completeness)2. If B A S , then B A S , (consistency)3. If B A S and C B S , then C A S (transitivity). The rationality constraints imply that we may represent the set of preferences by means of a numerical utility function u, which ranks the alternatives according to degrees of desirability, so that u(A) < u(B) whenever B A S and u(A) = u(B) whenever A ~ B . By working with utility functions instead of sets of preferences, we may speak of rational choice as choosing so as to maximize utility. The same set of preferences may be presented by many utility functions, as any strictly increasing transformation of a utility function will provide the same choices under maximalization. The distinction between the cost or values of something and its utility or disutility is one of the great strengths of the theory of economic rationality. The cost of some alternative says nothing about the value or benefits received from it, and neither cost nor benefit need be identical with the utility of the alternative if the risk posed by the action diminishes (or increases) its attractiveness to the agent. The utility of an action is usually some function of the cost, benefit, risk and other properties of the action.Decision TheoryCompared with the basic theory,decision theory adds probability measures p A which indicate the likelihood of each possible outcome for each alternative A . Decision theory supposes that the agent does not know the actual situation, but does havebeliefs or expectations about the consequences of choice in different states. Decision theory also strengthens the notion of utility from an ordinal utility function u to a cardinal utility function U , which inputs different values to each possible outcome. Ordinal utility functions use numeric values simply as ways of ranking the alternatives in a linear order. Amounts of cardinal utility can be added and subtracted to produce other amounts of utility. This makes it possible to combine the utilities foreseen in different possible outcomes of A into the expectedutility U(A), defined to be the utility of all possible outcomes weighted by their probability of occurrence, or formally,∑=SAdefS U S pA U ),()()(where the sum ranges over allpossible situations or states of nature under discussion. The decision-theoretic definition of preference isB A S if and only if U(A) ≤ U(B).Like the theory of preference, theassumptions of decision theory can be also formulated qualitatively [5].3.3 Negotiation Model of Goods andServicesThis model is fair and envy-free. In this model, we can see how real life solution would be useful in agent negotiation. One person divides the cake in two, and the other chooses the first piece. This is fair in that each believes he or she received at least a half, and envy-free in that neither would wish to trade. If a third person will come, each of those two will cut his/her portion into three parts and the third person will take one piece from each one. This protocol succeeds even when participants disagree about the portion’s value [7].Let’s bring this protocol into Agents world. First Agent can use the cake-cutting algorithm when negotiating for resources.~ ~~ ~ ~ ~ ~ ^^^^For example, two agents sharing CPU time might also wish to complete their computation as soon as possible. One agent might select the sizes of the processor’s time slices, while the other would have first choice of the resulting slices. Similar negotiation could occur over bandwidth, relative cache size, locks on databases, storage space in a file system, or task decomposition and distribution. This algorithm can be applied not only on two agents but on N agents as well. When n+1 agents come to negotiate, each of n agents will divide its sources into n+1 parts. Agent n+1 will choose one slice from each agent. Formal Description of Cut Cake Algorithm<A, S, F, D>A – A set of Agents for negotiation.S – A set of sources for negotiation.At the beginning, one agent holdsthis set. S i is the source held by A iagent.F – A set of f i functions f i ∈ F, and f iis cutting function or algorithm ofagent A i.D - A set of d i functions d i ∈ D, andd i is decision function or algorithmof agent A i. This function is used fordecision making.4Implementation of Goods andServices AlgorithmIn a classical multi-agent system, each autonomous agent must be able to decide how to behave in various situations. Coordination, negotiation, decision-making, and learning, like other agent‘s activities, create a huge demand on agent’s problem solving abilities. Creating greater demand means making agents more complicated. In such situations, mobile agents must carry bigger code segments, what is reflected in heavier network load and consequently inefficient resource exploitation. Therefore, we think that creating specialized agents is a good solution to lighten the demand on individual agents. Reasonable specialization would make it possible for an agent to facilitate services of other agents and therefore be more concerned with tasks delegated to him.Class DescriptionNegCenter Represents the negotiationcenter. It is a subclass of themain Aglet class. Agents canaccess it with KQML requestsfor negotiating. Class storesinformation about services intoMySQL database via a JDBCinterface. The followinginformation is stored: name,category, description, minimumquantity, maximum quantity,value (price). This informationis stored when agent sends a bidto negotiation center. It is usedwhen agent sends offer tocenter. It is also sorted by pricesand possibilities and passed toagent.AgentNeg Subclass of Aglets andJKQML.Supports KQMLrequests and NegCenter specificcommunication functions.AgentBidderSubclass of AgentNeg class.Can be used as agent which isbidding some services (seller). AgentOffer Subclass of AgentNeg class.Can be used as agent which isoffering some services (buyer).Tab. 1. Implemented classesOur main goal is to create an agent, nested into a multi-agent system, which would handle negotiation and support decision making of an agent domain. The main entity in our model, called negotiation center, collects all the information neededfor effective decision making (Fig. 1). Exchange of detailed specifications and information is done by means of negotiation. Concentration of all information about offered and demanded goods and services creates a framework for effective functioning of agent domains. In addition,benefits of adding new features and abilities to the negotiation center are shared by every member in a domain. Detailed description of each class of our model is given in Tab. 1. Negotiation center uses decision algorithms to rationally apportion goods and services into parts with equal utilities. In order to enable the system to compute utilities, negotiation center needs to know the utility functions of particular goods and services. Individual utilities are functions of elements such as price, amount, time, etc. On the other hand, client agents need to make the best choice among alternatives offered by the negotiation center. We suppose that each client agent aims at maximizing the total agent’s utility while choices must accord with the agent’s preferences.The relative disadvantage of our model is that each agent in the agent domain must truly believe that the specialized agent which facilitates negotiation and decision support for him is fair. To ensure fair behavior of our negotiation center, we utilize the Cut Cake algorithm described above. Negotiation and resource distribution by this algorithm is fast, fair and efficient.We base the inter-agent communication on KQML, which alsoensures standard message interchange withother multi-agent platforms. Using standard protocols makes the model suitable for verification in real commercial systems. 5 Conclusion and Future WorkIn the article, we provide formal description of algorithms for negotiation and decision support. We also propose a reference model of a multi-agent system. The main entity in our model is represented by a negotiation center, which assists other agents with negotiation and decision making inside an agent domain.By implementing our model we want to prove legitimation of specialized agents, as well as manifest the benefits the rational specialization of agents might bring. Our future work will be primarily concerned with improving the architecture of our model, aswell as accommodating algorithms for multi-agent environments.References[1] Bigus, J.: Constructing Intelligent Agents with Java. Willey Computer Publishing, Canada, 1997.[2] Chuang, T., Yadav, S., B.: An Agent-Based Architecture Of An AdaptiveDecision Support System, 1997. Fig. 1. Model[3] Cockayne, W., R., Zyda, M.: Mobile Agents, Manning Publication co., USA, 1997.[4] Cremonini, M., Omicini A., Zamboneli,F.: Ruling Agent Motion in Structured Enviroments, HPCN Europe, 2000.[5] DOYLE, J.: Rationality and its Roles in Reasoning. In Computational Intelligence, Vol. 8, No. 2 (May 1992), pp. 376-409. [6] Genesereth, M., R., Ketchpel, S. P.: Software Agents.[7] Huhns, M., N., Malhotra, A., K.: Negotiating for Goods and Services, IEEE Internet Computing, July-August 1999. [8] Labrou, Y., Finin, T.: A Proposal for a new KQML Specification, TR CS-97-03, available through the Stanford University Computer Science Department, 1997. [9] Lange, D., B., Oshima, M.: Programming and Deploying Java Mobile Agents with Aglets. Addison-Wesley, Canada, 1998. [10] Zeng, D., Sycara, K.: How Can Agent Learn to Negotiate? In: Intelligent agents III, ECAI ‘96 Workshop, pp.233-244, 1996. [11] Intelligent Software Agents.[/~softagents/] [12] Knowbot System Software[/home/koe/] [13] The Open Agent Architecture [/~oaa/][14] Agents Software Development Kit Home [http://www.trl.ibm.co.jp/aglets/] [15] KABASH[/kasbah/] [16] FOR AND ABOUT NEGOTIATIONS [/]。