A game-theoretic control approach for job shops in the
Job shop调度文化进化算法研究

R s a c n c t r l v u i a y a g i m s a pl d t ob s op p ob ems e e r h o ul a ol ton r l or h u e t p i oJ h r l e
p o l m r be
作业车 间调 度优化 问题 (S ) J P 在实 际生 产计划
中非 常普遍 ,也 是典型 的 N -ad问题 ,由于解空 P hr 间容 量大 、存在 工艺 约束限 制 ,其 研究一 直 是国际 上的 热点 。对于 求解 N -ad问题 ,现 在一般 采用 P hr
1 示。 所
智能 启发式 方法 ,如遗 传算法 … 、模拟 退火算 法f、 2 ]
微粒 群算法 [ 等 ,但是 启发式 搜 索方法都 面 临的 3 1 等 难题 是如何 提高全 局搜 索能力 , 何提 高收敛 速度 如 和稳 定性 。目前许 多研究都 设法吸 取 和融合 更多的 进化 思想 ,对算法 进行 改进 ,提 高其 搜索性 能 。
Ab t a t Mo t f c e u i g o t z t r bems i s rc: s h d l p i a i p o l os n mi on NP- a d p o l s h r r bems ma y h u i t lor h , n ei h e r h o e t es l . r e e o e t e s a c fb s u t Cut r l olt n r g i m, a e n t e n r s l a u Ev u i a y Alor h o t b s d o h mec a i m f u t r v l t n a d Cut r l g r h h n s o l al o u i n l a c u e o u Al o i ms gu d h i t n an t p o t , ie t e d r i d s e f ec o i d vd as i e s a c , y u i g t e k o e g f ut r l p c At h a n ii u l n t e r h b sn n wld e o l a a e. e s me t h h c u s t me t e t p i ,h o  ̄ ut r l p c v l ig a d u d t g. en CEA e p l d t o h r bems Th c l a a e i e ovn n p ai u s s n Th ar a p i J b s op p o l e o . e a g i m e t d wi a l i l t n. n h e uts o h t l or h i t s e t M t t S h ab s mu a i o a d t e r s l h ws t a A fe t e i CE i e c i n S v
大规模柔性作业车间调度问题分解建模和求解方法
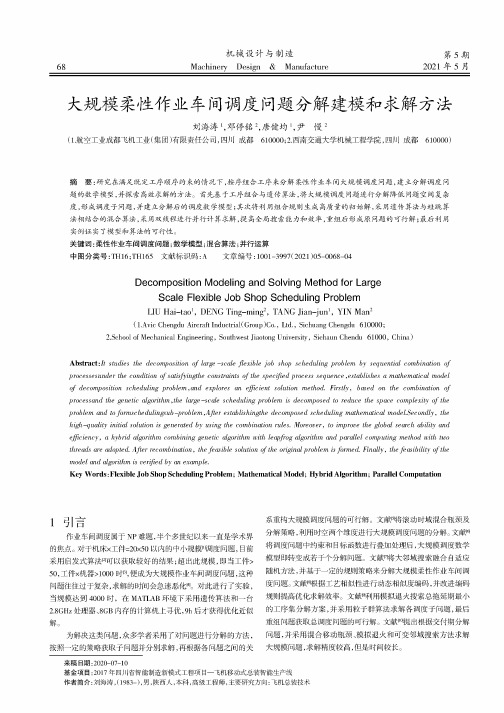
机械设计与制造68Machinery Design&Manufacture第5期2021年5月大规模柔性作业车间调度问题分解建模和求解方法刘海涛1,邓停铭2,唐健均1,尹慢2(1.航空工业成都飞机工业(集团)有限责任公司,四川成都610000;2.西南交通大学机械工程学院,四川成都610000)摘要:研究在满足既定工序顺序约束的情况下,按序组合工序来分解柔性作业车间大规模调度问题,建立分解调度问题的数学模型,并探索高效求解的方法。
首先基于工序组合与遗传算法,将大规模调度问题进行分解降低问题空间复杂度,形成调度子问题,并建立分解后的调度数学模型;其次将利用组合规则生成高质量的初始解,采用遗传算法与蛙跳算法相结合的混合算法,采用双线程进行并行计算求解,提高全局搜索能力和效率,重组后形成原问题的可行解;最后利用实例证实了模型和算法的可行性。
关键词:柔性作业车间调度问题;数学模型;混合算法;并行运算中图分类号:TH16;TH165文献标识码:A文章编号:1001-3997(2021)05-0068-04Decomposition Modeling and Solving Method for LargeScale Flexible Job Shop Scheduling ProblemLIU Hai-tao1,DENG Ting-ming2,TANGJian-jun1,YIN Man2(1.Avic Chengdu Aircraft Inductrial(Group)Co.,Ltd.,Sichuang Chengdu610000;2.School of Mechanical Engineering,Southwest Jiaotong University,Sichaun Chendu61000,China)Abstract:It studies the decomposition of large-scale flexible job shop scheduling problem by sequential combination of processesunder the condition of satisfyingthe constraints of the specified process sequence,e stablishes a mathematical model of decomposition scheduling problem,and explores an efficient solution method.Firstly,based on the combination of processand the genetic algorithm,the large-scale scheduling problem is decomposed to reduce the space complexity of the problem and to formschedulingsub-problem,fter establishingthe decomposed scheduling mathematical model.Secondly,the high-quality initial solution is generated by using the combination rules.Moreover,t o improve the global search ability and efficiency,a hybrid algorithm combining genetic algorithm with leapfrog algorithm and parallel computing method with two threads are adopted.After recombination,the feasible solution ofthe original problem is formed.Finally,t he feasibility ofthe model and algorithm is verified by an example.Key Words:Flexible Job Shop Scheduling Problem;Mathematical Model;Hybrid Algorithm;Parallel Computation1引言作业车间调度属于NP难题,半个多世纪以来一直是学术界的焦点。
半导体生产线动态实时智能调度方法研究【控制理论与控制工程专业优秀论文】
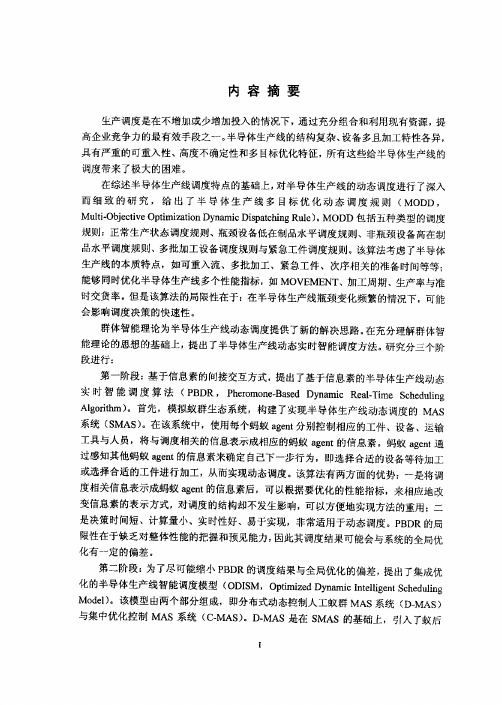
内容摘要生产调度是在不增加或少增加投入的情况下,通过充分组合和利用现有资源,提高企业竞争力的最有效手段之一。
半导体生产线的结构复杂、设备多且加工特性各异,具有严重的可重入性、高度不确定性和多目标优化特征,所有这些给半导体生产线的调度带来了极大的困难。
在综述半导体生产线调度特点的基础上,对半导体生产线的动态调度进行了深入而细致的研究,给出了半导体生产线多目标优化动态调度规则(MODD,Multi.objectiveOptimizationDynamicDispatc}lingRule)。
MODD包括五种类型的调度规则:正常生产状态调度规则、瓶颈设备低在制品水平调度规则、非瓶颈设备高在制品水平调度规则、多批加工设备调度规则与紧急工件调度规则。
该算法考虑了半导体生产线的本质特点,如可重入流、多批加工、紧急工件、次序相关的准备时间等等;能够同时优化半导体生产线多个性能指标。
如MOVEMENT、加工周期、生产率与准时交货率。
但是该算法的局限性在于:在半导体生产线瓶颈变化频繁的情况下,可能会影响调度决策的快速性。
群体智能理论为半导体生产线动态调度提供了新的解决思路。
在充分理解群体智能理论的思想的基础上,提出了半导体生产线动态实时智能调度方法。
研究分三个阶段进行:第一阶段:基于信息素的间接交互方式,提出了基于信息素的半导体生产线动态实时智能调度算法(PBDR,Pheromone.BasedDynamicReal.TimeSchedutingAlgorithm)。
首先,模拟蚁群生态系统,构建了实现半导体生产线动态调度的MAS系统(SMAS)。
在该系统中,使用每个蚂蚁agent分别控制相应的工件、设备、运输工具与人员,将与调度相关的信息表示成相应的蚂蚁agent的信息素。
蚂蚁agent通过感知其他蚂蚁agent的信息素来确定自己下一步行为,即选择合适的设备等待加工或选择合适的工件进行加工,从而实现动态调度。
该算法有两方面的优势:一是将调度相关信息表示成蚂蚁agent的信息素后,可以根据要优化的性能指标,来相应地改变信息素的表示方式,对调度的结构却不发生影响,可以方便地实现方法的重用;二是决策时间短、计算量小、实时性好、易于实现,非常适用于动态调度。
求解柔性作业车间调度问题的遗传-蚁群算法
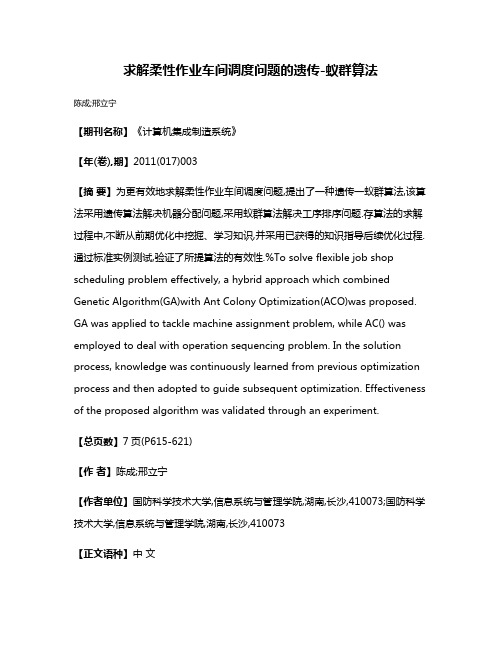
求解柔性作业车间调度问题的遗传-蚁群算法陈成;邢立宁【期刊名称】《计算机集成制造系统》【年(卷),期】2011(017)003【摘要】为更有效地求解柔性作业车间调度问题,提出了一种遗传一蚁群算法,该算法采用遗传算法解决机器分配问题,采用蚁群算法解决工序排序问题.存算法的求解过程中,不断从前期优化中挖掘、学习知识,并采用已获得的知识指导后续优化过程.通过标准实例测试,验证了所提算法的有效性.%To solve flexible job shop scheduling problem effectively, a hybrid approach which combined Genetic Algorithm(GA)with Ant Colony Optimization(ACO)was proposed. GA was applied to tackle machine assignment problem, while AC() was employed to deal with operation sequencing problem. In the solution process, knowledge was continuously learned from previous optimization process and then adopted to guide subsequent optimization. Effectiveness of the proposed algorithm was validated through an experiment.【总页数】7页(P615-621)【作者】陈成;邢立宁【作者单位】国防科学技术大学,信息系统与管理学院,湖南,长沙,410073;国防科学技术大学,信息系统与管理学院,湖南,长沙,410073【正文语种】中文【中图分类】TP312;TPL8【相关文献】1.求解多目标柔性作业车间调度问题的两阶段混合Pareto蚁群算法 [J], 赵博选;高建民;陈琨2.求解柔性作业车间调度问题的两阶段参数自适应蚁群算法 [J], 凌海峰;王西山3.一种异步蚁群算法求解柔性作业车间调度问题 [J], 田松龄;陈东祥;王太勇;刘晓敏4.改进遗传蜂群算法求解分布式柔性作业车间调度问题 [J], 李佳路;王雷;王静云5.应用改进蚁群算法求解柔性作业车间调度问题 [J], 刘志勇;吕文阁;谢庆华;何明玉;杨杰;刘雄辉因版权原因,仅展示原文概要,查看原文内容请购买。
Intermittent_Control_for_Fixed-Time_Synchronizatio
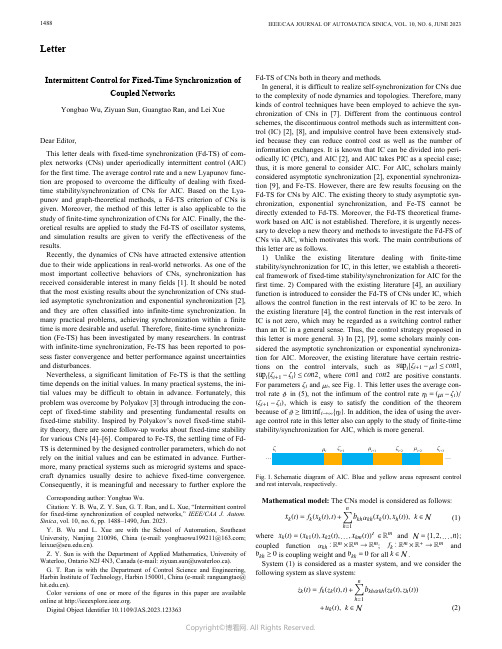
LetterIntermittent Control for Fixed-Time Synchronization ofCoupled NetworksYongbao Wu, Ziyuan Sun, Guangtao Ran, and Lei XueDear Editor,This letter deals with fixed-time synchronization (Fd-TS) of com-plex networks (CNs) under aperiodically intermittent control (AIC) for the first time. The average control rate and a new Lyapunov func-tion are proposed to overcome the difficulty of dealing with fixed-time stability/synchronization of CNs for AIC. Based on the Lya-punov and graph-theoretical methods, a Fd-TS criterion of CNs is given. Moreover, the method of this letter is also applicable to the study of finite-time synchronization of CNs for AIC. Finally, the the-oretical results are applied to study the Fd-TS of oscillator systems, and simulation results are given to verify the effectiveness of the results.Recently, the dynamics of CNs have attracted extensive attention due to their wide applications in real-world networks. As one of the most important collective behaviors of CNs, synchronization has received considerable interest in many fields [1]. It should be noted that the most existing results about the synchronization of CNs stud-ied asymptotic synchronization and exponential synchronization [2], and they are often classified into infinite-time synchronization. In many practical problems, achieving synchronization within a finite time is more desirable and useful. Therefore, finite-time synchroniza-tion (Fe-TS) has been investigated by many researchers. In contrast with infinite-time synchronization, Fe-TS has been reported to pos-sess faster convergence and better performance against uncertainties and disturbances.Nevertheless, a significant limitation of Fe-TS is that the settling time depends on the initial values. In many practical systems, the ini-tial values may be difficult to obtain in advance. Fortunately, this problem was overcome by Polyakov [3] through introducing the con-cept of fixed-time stability and presenting fundamental results on fixed-time stability. Inspired by Polyakov’s novel fixed-time stabil-ity theory, there are some follow-up works about fixed-time stability for various CNs [4]–[6]. Compared to Fe-TS, the settling time of Fd-TS is determined by the designed controller parameters, which do not rely on the initial values and can be estimated in advance. Further-more, many practical systems such as microgrid systems and space-craft dynamics usually desire to achieve fixed-time convergence. Consequently, it is meaningful and necessary to further explore the Fd-TS of CNs both in theory and methods.In general, it is difficult to realize self-synchronization for CNs due to the complexity of node dynamics and topologies. Therefore, many kinds of control techniques have been employed to achieve the syn-chronization of CNs in [7]. Different from the continuous control schemes, the discontinuous control methods such as intermittent con-trol (IC) [2], [8], and impulsive control have been extensively stud-ied because they can reduce control cost as well as the number of information exchanges. It is known that IC can be divided into peri-odically IC (PIC), and AIC [2], and AIC takes PIC as a special case; thus, it is more general to consider AIC. For AIC, scholars mainly considered asymptotic synchronization [2], exponential synchroniza-tion [9], and Fe-TS. However, there are few results focusing on the Fd-TS for CNs by AIC. The existing theory to study asymptotic syn-chronization, exponential synchronization, and Fe-TS cannot be directly extended to Fd-TS. Moreover, the Fd-TS theoretical frame-work based on AIC is not established. Therefore, it is urgently neces-sary to develop a new theory and methods to investigate the Fd-FS of CNs via AIC, which motivates this work. The main contributions of this letter are as follows.sup i{ζi+1−µi}≤con1 sup i{ζi+1−ζi}≤con2con1con2ζiµiϑηi=(µi−ζi)/ (ζi+1−ζi)ϑ≥liminf i→∞{ηi}1) Unlike the existing literature dealing with finite-time stability/synchronization for IC, in this letter, we establish a theoreti-cal framework of fixed-time stability/synchronization for AIC for the first time. 2) Compared with the existing literature [4], an auxiliary function is introduced to consider the Fd-TS of CNs under IC, which allows the control function in the rest intervals of IC to be zero. In the existing literature [4], the control function in the rest intervals of IC is not zero, which may be regarded as a switching control rather than an IC in a general sense. Thus, the control strategy proposed in this letter is more general. 3) In [2], [9], some scholars mainly con-sidered the asymptotic synchronization or exponential synchroniza-tion for AIC. Moreover, the existing literature have certain restric-tions on the control intervals, such as ,, where and are positive constants. For parameters and , see Fig. 1. This letter uses the average con-trol rate in (5), not the infimum of the control rate, which is easy to satisfy the condition of the theorem because of . In addition, the idea of using the aver-age control rate in this letter also can apply to the study of finite-time stability/synchronization for AIC, which is more general.x k(t)=(x k1(t),x k2(t),...,x km(t))αkh:R m×R m→R m f k:R m×R+→R mb kh≥0b kk=0k∈Nwhere and ; coupled function ; and is coupling weight and for all .System (1) is considered as a master system, and we consider theCorresponding author: Yongbao Wu.Citation: Y. B. Wu, Z. Y. Sun, G. T. Ran, and L. Xue, “Intermittent control for fixed-time synchronization of coupled networks,” IEEE/CAA J. Autom. Sinica, vol. 10, no. 6, pp. 1488–1490, Jun. 2023.Y. B. Wu and L. Xue are with the School of Automation, Southeast University, Nanjing 210096, China (e-mail:Z. Y. Sun is with the Department of Applied Mathematics, University of G. T. Ran is with the Department of Control Science and Engineering, Harbin Institute of Technology, Harbin 150001, China (e-mail: ranguangtao@ ).Color versions of one or more of the figures in this paper are available online at .Digital Object Identifier 10.1109/JAS.2023.123363ζiζi+1μi+1μi+2ζi+2ζi+3μi……Fig. 1. Schematic diagram of AIC. Blue and yellow areas represent control and rest intervals, respectively.1488 IEEE/CAA JOURNAL OF AUTOMATICA SINICA, VOL. 10, NO. 6, JUNE 2023z k (t )∈R m u k (t )in which and is an AIC strategy.y k (t )=z k (t )−x k (t )Let be error vector. Based on systems (1) andf k (x k k k (z k k (x k kh (x k k h h )=in which and sign(y k )=diag(sign(y k 1),sign(y k 2),...,sign(y km ))N ={0,1,2,...}[ζi ,µi )[µi ,ζi +1)ζ0=0[y k ]p =(|y k 1|p ,|y k 2|p ,...,|y km |p )T ;ϱs>0(s =1,2,3)q >10<p <1where and ; and stand for the i th control interval and rest interval, respectively; , , , and .f k αkh βk >0φkh >0Assumption 1: Functions and satisfy the Lipschitz condi-tions with Lipschitz constants and , respectively.ϑ∈(0,1)Definition 1 [8]: For AIC strategy (4), there are and con 12[t 2,t 1)ϑT ϑin which stands for the total control interval length on , represents the average control rate, and is called the elasticity number.T (y (0))>0lim t →T (y (0))y (t )=0y (t )≡0t ≥T (y (0))y (t )=(y T 1(t ),y T 2(t ),...,y T n (t ))T T (y (0))y (0)T ∗>0y(0)Definition 2: The master system (1) and slave system (2) are said to achieve Fe-TS, if there is a settling time such that and for , where . The time is called the settling time of syn-chronization, which is dependent of . Especially, if there is a fixed time which is independent of , then systems (1) and (2) achieve the Fd-TS.Analysis of Fd-TS: This section gives two lemmas to study the Fd-TS under AIC (4). Then, a Fd-TS criterion of CNs is given.a 12∗∗in which , , , and Then, whenϑProof: See Section II in the Supplementary material.■iin which , , and If there existsˆa11exp {(1−q )˘εT ϑϑwhere , and are defined in Definition 1.Proof: See Section III in the Supplementary material.■Now, a main result is state as follows.(G ,A )A =(b kh φkh )n ×n ˘ε>0Theorem 1: If the digraph with is stronglyconnected, and there exists satisfying the following inequali-a 33k 44k 3k 1k )−4∑n h =1b kh φkh >0a 4k =2βk +4∑n h =1b kh φkh T ∗in which , , , and , then systems (1)and (2) achieve the Fd-TS, and settling time satisfiesˆa 1=a 1exp {(1−q )˘εT ϑ}a 2=2σmin ϱ2a 1=2σmin ϱ3(mn )1−q2σ1min =min k ∈N {c 1−p2k }σ2min =min k ∈N {c 1−q 2k }c k >0with , , ,, , and can be found in [10].Proof: See Section IV in the Supplementary material.■ϑRemark 1: Theorem 1 requires two inequalities in (8) to be true.We can get that if the average control rate is greater, the condi-tions are easier to meet when other parameters are fixed, which shows that the design of IC has an essential impact on the Fd-TS of CNs.U (t )=exp {Θ(t )}Ψ(t )Θ(t )t ∈[µi ,ζi +1),Remark 2: In the proof of Lemma 2, we use an auxiliary function , where function is defined in Section III-1of the Supplementary material. Moreover, when we can U(t )≤−(˘εϑ−a 4)U (t )[µi ,ζi +1)which enables to be established in the rest intervals . Similar ideas were discussed in semilinear sys-tems [8]. This letter uses the technique to overcome the difficulty of studying Fd-TS of CNs under AIC.sup i {ζi +1−µi }=con 1sup i {ζi +1−ζi }=con 2con 1con 2ηi =(µi −ζi )/(ζi +1−ζi )ϑ≥inf i ∈N {ηi }Remark 3: In Lemma 2, we give a synchronization criterion to achieve the Fd-TS of the systems under AIC for the first time. In the existing results [2], [9], some scholars mainly considered the asymp-totic synchronization or exponential synchronization for IC. More-over, the existing results have certain restrictions on the control and rest intervals, such as , ,where and are positive constants. In this letter, we use the average control rate instead of the infimum of the control rate , which is easier to satisfy the conditions of the theorem because of . In addition, the idea of using an average control rate in this letter also applies to the study of Fe-TS, which is more general. However, as far as we know, no author has considered the general case for Fe-TS under AIC by using the technique of this letter.T ∗ϑT ϑa 1a 2T ∗ϑT ∗Remark 4: We give an important differential inequality (7) in Lemma 2, which can deal with Fd-TS of CNs for AIC. Moreover, the fixed time depends on the average control rate and elasticity number . In addition, we find that the larger parameters and in (7), the smaller the fixed time . And when the average control rate is greater, the settling time will be smaller.Remark 5: Recently, some scholars have considered the Fd-TS for CNs under AIC [4]. For the IC, this letter allows the control function in the rest intervals of the IC to be zero. In the existing results [4], the control function in the rest intervals of IC is not zero, which may be regarded as a switching control. In addition, the average control rate of AIC is considered, which provides less conservative results.Application and numerical simulations: See Section IV in the Supplementary material.Conclusions: We considered the Fd-TS of CNs under AIC. The average control rate and a new Lyapunov function were proposed to overcome the difficulty of dealing with fixed-time stability/synchro-nization of CNs for AIC. Meanwhile, a Fd-TS criterion of CNs was given. Finally, we applied the theoretical results to study the Fd-TS of oscillator systems, and simulation results were given to verify the effectiveness of the results. Considering the influence of the delay factor, the Fd-TS of delayed CNs under AIC will be studied in the future.Acknowledgments: This work was supported in part by the Natu-ral Science Foundation of Jiangsu Province of China (BK20220811,BK20202006); the National Natural Science Foundation of China (62203114, 62273094); the Fundamental Research Funds for the Central Universities, and the “Zhishan” Scholars Programs of South-WU et al .: INTERMITTENT CONTROL FOR FD-TS OF CNS 1489east University; China Postdoctoral Science Foundation (2022M 710684); and Excellent Postdoctoral Foundation of Jiangsu Provinceof China (2022ZB116).Supplementary material: The supplementary material of this let-ter can be found in links https:///est/d3296793221015/pdf.ReferencesF. Dörfler and F. Bullo, “Synchronization in complex networks of phaseoscillators: A survey,” Automatica , vol. 50, no. 6, pp. 1539–1564, 2014.[1]X. Liu and T. Chen, “Synchronization of complex networks viaaperiodically intermittent pinning control,” IEEE Trans. Automatic Control , vol. 60, no. 12, pp. 3316–3321, 2015.[2]A. Polyakov, “Nonlinear feedback design for fixed-time stabilization oflinear control systems,” IEEE Trans. Automatic Control , vol. 57, no. 8,pp. 2106–2110, 2011.[3]Q. Gan, F. Xiao, and H. Sheng, “Fixed-time outer synchronization ofhybrid-coupled delayed complex networks via periodically semi-intermittent control,” J. Franklin Institute , vol. 356, no. 12, pp. 6656–6677, 2019.[4]J. Liu, Y. Wu, M. Sun, and C. Sun, “Fixed-time cooperative tracking fordelayed disturbed multi-agent systems under dynamic event-triggered control,” IEEE/CAA J. Autom. Sinica , vol. 9, no. 5, pp. 930–933, 2022.[5]Z. Zuo, B. Tian, M. Defoort, and Z. Ding, “Fixed-time consensustracking for multiagent systems with high-order integrator dynamics,”IEEE Trans. Autom. Control , vol. 63, no. 2, pp. 563–570, 2018.[6]W. Yu, P. DeLellis, G. Chen, M. Di Bernardo, and J. Kurths,“Distributed adaptive control of synchronization in complex networks,”IEEE Trans. Autom. Control , vol. 57, no. 8, pp. 2153–2158, 2012.[7]Y. Guo, M. Duan, and P. Wang, “Input-to-state stabilization ofsemilinear systems via aperiodically intermittent event-triggered control,” IEEE Trans. Control Network Syst., vol. 9, no. 2, pp. 731–741, 2022.[8]Y. Wu, S. Zhuang, and W. Li, “Periodically intermittent discreteobservation control for synchronization of the general stochastic complex network,” Automatica , vol. 110, p. 108591, 2019.[9]M. Y. Li and Z. Shuai, “Global-stability problem for coupled systems ofdifferential equations on networks,” J. Differential Equations , vol. 248,no. 1, pp. 1–20, 2010.[10] 1490IEEE/CAA JOURNAL OF AUTOMATICA SINICA, VOL. 10, NO. 6, JUNE 2023。
不对称约束多人非零和博弈的自适应评判控制

第40卷第9期2023年9月控制理论与应用Control Theory&ApplicationsV ol.40No.9Sep.2023不对称约束多人非零和博弈的自适应评判控制李梦花,王鼎,乔俊飞†(北京工业大学信息学部,北京100124;计算智能与智能系统北京市重点实验室,北京100124;智慧环保北京实验室,北京100124;北京人工智能研究院,北京100124)摘要:本文针对连续时间非线性系统的不对称约束多人非零和博弈问题,建立了一种基于神经网络的自适应评判控制方法.首先,本文提出了一种新颖的非二次型函数来处理不对称约束问题,并且推导出最优控制律和耦合Hamilton-Jacobi方程.值得注意的是,当系统状态为零时,最优控制策略是不为零的,这与以往不同.然后,通过构建单一评判网络来近似每个玩家的最优代价函数,从而获得相关的近似最优控制策略.同时,在评判学习期间发展了一种新的权值更新规则.此外,通过利用Lyapunov理论证明了评判网络权值近似误差和闭环系统状态的稳定性.最后,仿真结果验证了本文所提方法的有效性.关键词:神经网络;自适应评判控制;自适应动态规划;非线性系统;不对称约束;多人非零和博弈引用格式:李梦花,王鼎,乔俊飞.不对称约束多人非零和博弈的自适应评判控制.控制理论与应用,2023,40(9): 1562–1568DOI:10.7641/CTA.2022.20063Adaptive critic control for multi-player non-zero-sum games withasymmetric constraintsLI Meng-hua,WANG Ding,QIAO Jun-fei†(Faculty of Information Technology,Beijing University of Technology,Beijing100124,China;Beijing Key Laboratory of Computational Intelligence and Intelligent System,Beijing100124,China;Beijing Laboratory of Smart Environmental Protection,Beijing100124,China;Beijing Institute of Artificial Intelligence,Beijing100124,China)Abstract:In this paper,an adaptive critic control method based on the neural networks is established for multi-player non-zero-sum games with asymmetric constraints of continuous-time nonlinear systems.First,a novel nonquadratic func-tion is proposed to deal with asymmetric constraints,and then the optimal control laws and the coupled Hamilton-Jacobi equations are derived.It is worth noting that the optimal control strategies do not stay at zero when the system state is zero, which is different from the past.After that,only a critic network is constructed to approximate the optimal cost function for each player,so as to obtain the associated approximate optimal control strategies.Meanwhile,a new weight updating rule is developed during critic learning.In addition,the stability of the weight estimation errors of critic networks and the closed-loop system state is proved by utilizing the Lyapunov method.Finally,simulation results verify the effectiveness of the method proposed in this paper.Key words:neural networks;adaptive critic control;adaptive dynamic programming;nonlinear systems;asymmetric constraints;multi-player non-zero-sum gamesCitation:LI Menghua,WANG Ding,QIAO Junfei.Adaptive critic control for multi-player non-zero-sum games with asymmetric constraints.Control Theory&Applications,2023,40(9):1562–15681引言自适应动态规划(adaptive dynamic programming, ADP)方法由Werbos[1]首先提出,该方法结合了动态规划、神经网络和强化学习,其核心思想是利用函数近似结构来估计最优代价函数,从而获得被控系统的近似最优解.在ADP方法体系中,动态规划蕴含最优收稿日期:2022−01−21;录用日期:2022−11−10.†通信作者.E-mail:***************.cn.本文责任编委:王龙.科技创新2030–“新一代人工智能”重大项目(2021ZD0112302,2021ZD0112301),国家重点研发计划项目(2018YFC1900800–5),北京市自然科学基金项目(JQ19013),国家自然科学基金项目(62222301,61890930–5,62021003)资助.Supported by the National Key Research and Development Program of China(2021ZD0112302,2021ZD0112301,2018YFC1900800–5),the Beijing Natural Science Foundation(JQ19013)and the National Natural Science Foundation of China(62222301,61890930–5,62021003).第9期李梦花等:不对称约束多人非零和博弈的自适应评判控制1563性原理提供理论基础,神经网络作为函数近似结构提供实现手段,强化学习提供学习机制.值得注意的是, ADP方法具有强大的自学习能力,在处理非线性复杂系统的最优控制问题上具有很大的潜力[2–7].此外, ADP作为一种近似求解最优控制问题的新方法,已经成为智能控制与计算智能领域的研究热点.关于ADP的详细理论研究以及相关应用,读者可以参考文献[8–9].本文将基于ADP的动态系统优化控制统称为自适应评判控制.近年来,微分博弈问题在控制领域受到了越来越多的关注.微分博弈为研究多玩家系统的协作、竞争与控制提供了一个标准的数学框架,包括二人零和博弈、多人零和博弈以及多人非零和博弈等.在零和博弈问题中,控制输入试图最小化代价函数而干扰输入试图最大化代价函数.在非零和博弈问题中,每个玩家都独立地选择一个最优控制策略来最小化自己的代价函数.值得注意的是,零和博弈问题已经被广泛研究.在文献[10]中,作者提出了一种改进的ADP方法来求解多输入非线性连续系统的二人零和博弈问题.An等人[11]提出了两种基于积分强化学习的算法来求解连续时间系统的多人零和博弈问题.Ren等人[12]提出了一种新颖的同步脱策方法来处理多人零和博弈问题.然而,关于非零和博弈[13–14]的研究还很少.此外,控制约束在实际应用中也广泛存在.这些约束通常是由执行器的固有物理特性引起的,如气压、电压和温度.因此,为了确保被控系统的性能,受约束的系统需要被考虑.Zhang等人[15]发展了一种新颖的事件采样ADP方法来求解非线性连续约束系统的鲁棒最优控制问题.Huo等人[16]研究了一类非线性约束互联系统的分散事件触发控制问题.Yang和He[17]研究了一类具有不匹配扰动和输入约束的非线性系统事件触发鲁棒镇定问题.这些文献考虑的都是对称约束,而实际应用中,被控系统受到的约束也可能是不对称的[18–20],例如在污水处理过程中,需要通过氧传递系数和内回流量对溶解氧浓度和硝态氮浓度进行控制,而根据实际的运行条件,这两个控制变量就需要被限制在一个不对称约束范围内[20].因此,在控制器设计过程中,不对称约束问题将是笔者研究的一个方向.到目前为止,关于具有控制约束的微分博弈问题,有一些学者取得了相应的研究成果[12,21–23].但可以发现,具有不对称约束的多人非零和博弈问题还没有学者研究.同时,在多人非零和博弈问题中,相关的耦合Hamilton-Jacobi(HJ)方程是很难求解的.因此,本文针对一类连续时间非线性系统的不对称约束多人非零和博弈问题,提出了一种自适应评判控制方法来近似求解耦合HJ方程,从而获得被控系统的近似最优解.本文的主要贡献如下:1)首次将不对称约束应用到连续时间非线性系统的多人非零和博弈问题中;2)提出了一种新颖的非二次型函数来处理不对称约束问题,并且当系统状态为零时,最优控制策略是不为零的,这与以往不同;3)在学习期间,用单一评判网络结构代替了传统的执行–评判网络结构,并且提出了一种新的权值更新规则;4)利用Lyapunov方法证明了评判网络权值近似误差和系统状态的一致最终有界(uniformly ultimately bounded,UUB)稳定性.2问题描述考虑以下具有不对称约束的N–玩家连续时间非线性系统:˙x(t)=f(x(t))+N∑j=1g j(x(t))u j(t),(1)其中:x(t)∈Ω⊂R n是状态向量且x(0)=x0为初始状态,R n代表由所有n-维实向量组成的欧氏空间,Ω是R n的一个紧集;u j(t)∈T j⊂R m为玩家j在时刻t所选择的策略,且T j为T j={[u j1u j2···u jm]T∈R m:u j min u jl u j max, |u j min|=|u j max|,l=1,2,···,m},(2)其中:u jmin∈R和u j max∈R分别代表控制输入分量的最小界和最大界,R表示所有实数集.假设1非线性系统(1)是可控的,并且x=0是被控系统(1)的一个平衡点.此外,∀j∈N,f(x)和g j(x)是未知的Lipschitz函数且f(0)=0,其中集合N={1,2,···,N},N 2是一个正整数.假设2∀j∈N,g j(0)=0,且存在一个正常数b gj使∥g j(x)∥ b gj,其中∥·∥表示在R n上的向量范数或者在R n×m上的矩阵范数,R n×m代表由所有n×m维实矩阵组成的空间.注1假设1–3是自适应评判领域的常用假设,例如文献[6,13,19],是为了保证系统的稳定性以及方便后文中的稳定性证明,其中假设3出现在后文中的第3.2节.定义与每个玩家相关的效用函数为U i(x,U)=x T Q i x+N∑j=1S j(u j),i∈N,(3)其中U={u1,u2,···,u N}并且Q i是一个对称正定矩阵.此外,为了处理不对称约束问题,令S j(u j)为S j(u j)=2αj m∑l=1ujlβjtanh−1(z−βjαj)d z,(4)其中αj和βj分别为αj=u jmax−u j min2,βj=u jmax+u jmin2.(5)因此,与每个玩家相关的代价函数可以表示为J i(x0,U)=∞U i(x,U)dτ,i∈N,(6)1564控制理论与应用第40卷本文希望构建一个Nash均衡U∗={u∗1,u∗2,···,u∗N},来使以下不等式被满足:J i(u∗1,···,u∗i,···,u∗N)J i(u∗1,···,u i,···,u∗N),(7)其中i∈N.为了方便,将J i(x0,U)简写为J i(x0).于是,每个玩家的最优代价函数为J∗i (x0)=minu iJ i(x0,U),i∈N.(8)在本文中,如果一个控制策略集的所有元素都是可容许的,那么这个集合是可容许的.定义1(容许控制[24])如果控制策略u i(x)是连续的,u i(x)可以镇定系统(1),并且J i(x0)是有限的,那么它是集合Ω上关于代价函数(6)的可容许控制律,即u i(x)∈Ψ(Ω),i∈N,其中,Ψ(Ω)是Ω上所有容许控制律的集合.对于任意一个可容许控制律u i(x)∈Ψ(Ω),如果相关代价函数(6)是连续可微的,那么非线性Lyapu-nov方程为0=U i(x,U)+(∇J i(x))T(f(x)+N∑j=1g j(x)u j),(9)其中:i∈N,J i(0)=0,并且∇(·) ∂(·)∂x.根据最优控制理论,耦合HJ方程为0=minU H i(x,U,∇J∗i(x)),i∈N,(10)其中,Hamiltonian函数H i(x,U,∇J∗i(x))为H i(x,U,∇J∗i(x))=U i(x,U)+(∇J∗i (x))T(f(x)+N∑j=1g j(x)u j),(11)进而,由∂H i(x,U,∇J∗i(x))∂u i=0可得出最优控制律为u∗i (x)=−αi tanh(12αig Ti(x)∇J∗i(x))+¯βi,i∈N,(12)其中¯βi=[βiβi···βi]T∈R m.注2根据式(2)和式(5),能推导出βi=0,即¯βi=0,又根据式(12)可知u∗i(0)=0,i∈N.因此,为了保证x=0是系统(1)的平衡点,在假设2中提出了条件∀j∈N,g j(0)=0.将式(12)代入式(10),耦合HJ方程又能表示为(∇J∗i (x))T f(x)+N∑j=1((∇J∗i(x))T g j(x)¯βj)+x T Q i x−N∑j=1((∇J∗i(x))Tαj g j(x)tanh(A j(x)))+N∑j=1S j(−αj tanh(A j(x))+¯βj)=0,i∈N,(13)其中J∗i(0)=0并且A j(x)=12αjg Tj(x)∇J∗j(x).如果已知每个玩家的最优代价函数值,那么相关的最优状态反馈控制律就可以直接获得,也就是说式(13)是可解的.可是,式(13)这种非线性偏微分方程的求解是十分困难的.同时,随着系统维数的增加,存储量和计算量也随之以指数形式增加,也就是平常所说的“维数灾”问题.因此,为了克服这些弱点,在第3部分提出了一种基于神经网络的自适应评判机制,来近似每个玩家的最优代价函数,从而获得相关的近似最优状态反馈控制策略.3自适应评判控制设计3.1神经网络实现本节的核心是构建并训练评判神经网络,以得到训练后的权值,从而获得每个玩家的近似最优代价函数值.首先,根据神经网络的逼近性质[25],可将每个玩家的最优代价函数J∗i(x)在紧集Ω上表示为J∗i(x)=W Tiσi(x)+ξi(x),i∈N,(14)其中:W i∈Rδ是理想权值向量,σi(x)∈Rδ是激活函数,δ是隐含层神经元个数,ξi(x)∈R是重构误差.同时,可得出每个玩家的最优代价函数梯度为∇J∗i(x)=(∇σi(x))T W i+∇ξi(x),i∈N,(15)将式(15)代入式(12),有u∗i(x)=−αi tanh(B i(x)+C i(x))+¯βi,i∈N,(16)其中:B i(x)=12αig Ti(x)(∇σi(x))T W i∈R m,C i(x)=12αig Ti(x)∇ξi(x)∈R m.然后,将式(15)代入式(13),耦合HJ方程变为W Ti∇σi(x)f(x)+(∇ξi(x))T f(x)+x T Q i x+N∑j=1((W Ti∇σi(x)+(∇ξi(x))T)g j(x)¯βj)−N∑j=1(αj W Ti∇σi(x)g j(x)tanh(B j(x)+C j(x)))−N∑j=1(αj(∇ξi(x))T g j(x)tanh(B j(x)+C j(x)))+N∑j=1S j(−αj tanh(B j(x)+C j(x))+¯βj)=0,i∈N.(17)值得注意的是,式(14)中的理想权值向量W i是未知的,也就是说式(16)中的u∗i(x)是不可解的.因此,第9期李梦花等:不对称约束多人非零和博弈的自适应评判控制1565构建如下的评判神经网络:ˆJ∗i (x)=ˆW Tiσi(x),i∈N,(18)来近似每个玩家的最优代价函数,其中ˆW i∈Rδ是估计的权值向量.同时,其梯度为∇ˆJ∗i(x)=(∇σi(x))TˆW i,i∈N.(19)考虑式(19),近似的最优控制律为ˆu∗i(x)=−αi tanh(D i(x))+¯βi,i∈N,(20)其中D i(x)=12αig Ti(x)(∇σi(x))TˆW i.同理,近似的Hamiltonian可以写为ˆHi(x,ˆW i)=ˆW T i ϕi+x T Q i x+N∑j=1(ˆW Ti∇σi(x)g j(x)¯βj)−N ∑j=1(αjˆW Ti∇σi(x)g j(x)tanh(D j(x)))+N∑j=1S j(−αj tanh(D j(x))+¯βj),i∈N,(21)其中ϕi=∇σi(x)f(x).此外,定义误差量e i=ˆH i(x,ˆW i )−H i(x,U∗,∇J∗i(x))=ˆH i(x,ˆW i).为了使e i足够小,需要训练评判网络来使目标函数E i=12e Tie i最小化.在这里,本文采用的训练准则为˙ˆW i =−γi1(1+ϕTiϕi)2(∂E i∂ˆW i)=−γiϕi(1+ϕTiϕi)2e i,i∈N,(22)其中:γi>0是评判网络的学习率,(1+ϕT iϕi)2用于归一化操作.此外,定义评判网络的权值近似误差为˜Wi=W i−ˆW i.因此,有˙˜W i =γiφi1+ϕTiϕie Hi−γiφiφT i˜W i,i∈N,(23)其中:φi=ϕi(1+ϕTiϕi),e Hi=−(∇ξi(x))T f(x)是残差项.3.2稳定性分析本节的核心是通过利用Lyapunov方法讨论评判网络权值近似误差和闭环系统状态的UUB稳定性.这里,给出以下假设:假设3∥∇ξi(x)∥ b∇ξi ,∥∇σi(x)∥ b∇σi,∥e Hi∥ b e Hi,∥W i∥ b W i,其中:b∇ξi,b∇σi,b e Hi,b W i 都是正常数,i∈N.定理1考虑系统(1),如果假设1–3成立,状态反馈控制律由式(20)给出,且评判网络权值通过式(22)进行训练,则评判网络权值近似误差˜W i是UUB 稳定的.证选取如下的Lyapunov函数:L1(t)=N∑i=1(12˜W Ti˜Wi)=N∑i=1L1i(t),(24)计算L1i(t)沿着式(23)的时间导数,即˙L1i(t)=γi˜W Tiφi1+ϕTiϕie Hi−γi˜W TiφiφTi˜Wi,i∈N,(25)利用不等式¯X T¯Y12∥¯X∥2+12∥¯Y∥2(注:¯X和¯Y都是具有合适维数的向量),并且考虑1+ϕTiϕi 1,能得到˙L1i(t)γi2(∥φTi˜Wi∥2+∥e Hi∥2)−γi˜W TiφiφTi˜Wi=−γi2˜W TiφiφTi˜Wi+γi2∥e Hi∥2,i∈N.(26)根据假设3,有˙L1i(t) −γi2λmin(φiφTi)∥˜W i∥2+γi2b2e Hi,i∈N,(27)其中λmin(·)表示矩阵的最小特征值.因此,当不等式∥˜W i∥>√b2e Hiλmin(φiφTi),i∈N(28)成立时,有˙L1i(t)<0.根据标准的Lyapunov定理[26],可知评判网络权值近似误差˜W i是UUB稳定的.证毕.定理2考虑系统(1),如果假设1–3成立,状态反馈控制律由式(20)给出,且评判网络权值通过式(22)进行训练,则系统状态x(t)是UUB稳定的.证选取如下的Lyapunov函数:L2i(t)=J∗i(x),i∈N.(29)计算L2i(t)沿着系统˙x=f(x)+N∑j=1g j(x)ˆu∗j的时间导数,即˙L2i(t)=(∇J∗i(x))T(f(x)+N∑j=1g j(x)ˆu∗j)=(∇J∗i(x))T(f(x)+N∑j=1g j(x)u∗j)+N∑j=1((∇J∗i(x))T g j(x)(ˆu∗j−u∗j)),i∈N.(30)考虑式(13),有˙L2i(t)=−x T Q i x−N∑j=1S j(u∗j)+N∑j=1((∇J∗i(x))T g j(x)(ˆu∗j−u∗j))Σi,i∈N,(31)1566控制理论与应用第40卷利用不等式¯XT ¯Y 12∥¯X ∥2+12∥¯Y ∥2,并且考虑式(15)–(16)(20),可得Σi 12N ∑j =1∥−αj tanh (D j (x ))+αj tanh (F j (x ))∥2+12N ∑j =1∥g Tj (x )((∇σi (x ))T W i +∇ξi (x ))∥2,i ∈N ,(32)其中F j (x )=B j (x )+C j (x ).然后,利用不等式∥¯X+¯Y∥2 2∥¯X ∥2+2∥¯Y ∥2,有Σi N ∑j =1(∥αj tanh (D j (x ))∥2+∥αj tanh (F j (x ))∥2)+N ∑j =1∥g Tj (x )(∇σi (x ))T W i ∥2+N ∑j =1∥g T j (x )∇ξi (x )∥2,i ∈N ,(33)其中D j (x )∈R m ,F j (x )∈R m 分别被表示为[D j 1(x )D j 2(x )···D jm (x )]T 和[F j 1(x )F j 2(x )···F jm (x )]T .易知,∀θ∈R ,tanh 2θ 1.因此,有∥tanh (D j (x ))∥2=m ∑l =1tanh 2(D jl (x )) m,(34)∥tanh (F j (x ))∥2=m ∑l =1tanh 2(F jl (x )) m.(35)同时,根据假设2–3,有Σi N ∑j =1(2α2j m +b 2g j b 2∇σi b 2W i +b 2g j b 2∇ξi ),i ∈N ,(36)根据式(2)(4)–(5),可知S j (u ∗j ) 0.于是,有˙L2i (t ) −λmin (Q i )∥x ∥2+ϖi ,i ∈N ,(37)其中ϖi =N ∑j =1(2α2j m +b 2g j b 2∇σi b 2W i +b 2g j b 2∇ξi ).因此,根据式(37)可知,当不等式∥x ∥>√ϖiλmin (Q i )成立时,有˙L2i (t )<0.即,如果x (t )满足下列不等式:∥x ∥>max {√ϖ1λmin (Q 1),···,√ϖNλmin (Q N )},(38)则,∀i ∈N ,都有˙L 2i (t )<0.同理,可得闭环系统状态x (t )也是UUB 稳定的.证毕.4仿真结果考虑如下的3–玩家连续时间非线性系统:˙x =[−1.2x 1+1.5x 2sin x 20.5x 1−x 2]+[01.5sin x 1cos x 1]u 1(x )+[1.2sin x 1cos x 2]u 2(x )+[01.1sin x 2]u 3(x ),(39)其中:x (t )=[x 1x 2]T ∈R 2是状态向量,u 1(x )∈T 1={u 1∈R :−1 u 1 2},u 2(x )∈T 2={u 2∈R :−0.2 u 2 1}和u 3(x )∈T 3={u 3∈R :−0.4 u 3 0.8}是控制输入.令Q 1=2I 2,Q 2=1.8I 2,Q 3=0.3I 2,其中I 2代表2×2维单位矩阵.同时,根据式(5)可知,α1=1.5,β1=0.5,α2=0.6,β2=0.4,α3=0.6,β3=0.2.因此,与每个玩家相关的代价函数可以表示为J i (x 0)= ∞0(x TQ i x +3∑j =1S j (u j ))d τ,i =1,2,3,(40)其中S j (u j )=2αju jβj tanh −1(z −βjαj)d z =2αj (u j −βj )tanh −1(u j −βjαj)+α2j ln (1−(u j −βj )2α2j).(41)然后,本文针对系统(39)构建3个评判神经网络,每个玩家的评判神经网络权值分别为ˆW1=[ˆW 11ˆW 12ˆW13]T ,ˆW 2=[ˆW 21ˆW 22ˆW 23]T ,ˆW 3=[ˆW 31ˆW 32ˆW33]T ,激活函数被定义为σ1(x )=σ2(x )=σ3(x )=[x 21x 1x 2x 22]T,且隐含层神经元个数为δ=3.此外,系统初始状态取x 0=[0.5−0.5]T ,每个评判神经网络的学习率分别为γ1=1.5,γ2=0.8,γ3=0.2,且每个评判神经网络的初始权值都在0和2之间选取.最后,引入探测噪声η(t )=sin 2(−1.2t )cos(0.5t )+cos(2.4t )sin 3(2.4t )+sin 5t +sin 2(1.12t )+sin 2t ×cos t +sin 2(2t )cos(0.1t ),使得系统满足持续激励条件.执行学习过程,本文发现每个玩家的评判神经网络权值分别收敛于[6.90912.99046.6961]T ,[4.89012.23475.2062]T ,[1.79450.33212.4583]T .在60个时间步之后去掉探测噪声,每个玩家的评判网络权值收敛过程如图1–3所示.然后,将训练好的权值代入式(20),能得到每个玩家的近似最优控制律,将其应用到系统(39),经过10个时间步之后,得到的状态轨迹和控制轨迹分别如图4–5所示.由图4可知,系统状态最终收敛到了平衡点.由图5可知,每个玩家的控制轨迹都没有超出预定的边界,并且可以观察到u 1,u 2和u 3分别收敛于0.5,0.4和0.2.综上所述,仿真结果验证了所提方法的有效性.第9期李梦花等:不对称约束多人非零和博弈的自适应评判控制1567䇴 㖁㔌U / s图1玩家1的评判网络权值收敛过程Fig.1Convergence process of the critic network weights forplayer1䇴 㖁㔌U / s图2玩家2的评判网络权值收敛过程Fig.2Convergence process of the critic network weights forplayer2﹣䇴 㖁㔌U / s图3玩家3的评判网络权值收敛过程Fig.3Convergence process of the critic network weights forplayer 35结论本文首次将不对称约束应用到连续时间非线性系统的多人非零和博弈问题中.首先,获得了最优状态反馈控制律和耦合HJ 方程,并且为了解决不对称约束问题,建立了一种新的非二次型函数.值得注意的是,当系统状态为零时,最优控制策略是不为零的.其次,由于耦合HJ 方程不易求解,提出了一种基于神经网络的自适应评判算法来近似每个玩家的最优代价函数,从而获得相关的近似最优控制律.在实现过程中,用单一评判网络结构代替了经典的执行–评判结构,并且建立了一种新的权值更新规则.然后,利用Lyap-unov 理论讨论了评判网络权值近似误差和系统状态的UUB 稳定性.最后,仿真结果验证了所提算法的可行性.在未来的工作中,会考虑将事件驱动机制引入到连续时间非线性系统的不对称约束多人非零和博弈问题中,并且将该研究内容应用到污水处理系统中也是笔者的一个重点研究方向.﹣0.5﹣0.4﹣0.3﹣0.2﹣0.10.00.10.20.00.10.20.30.40.5(U )Y 1(U )Y 2图4系统(39)的状态轨迹Fig.4State trajectory of the system (39)0.00.51.01.52.00.00.20.40.60.81.01.200.012345678910﹣0.40.4﹣0.20.2(U )V 3(U )V 2(U )V 1U / s 012345678910U / s 012345678910U / s (c)(b)(a)(U )V 1(U )V 2(U )V 3图5系统(39)的控制轨迹Fig.5Control trajectories of the system (39)1568控制理论与应用第40卷参考文献:[1]WERBOS P J.Beyond regression:New tools for prediction andanalysis in the behavioral sciences.Cambridge:Harvard Universi-ty,1974.[2]HONG Chengwen,FU Yue.Nonlinear robust approximate optimaltracking control based on adaptive dynamic programming.Control Theory&Applications,2018,35(9):1285–1292.(洪成文,富月.基于自适应动态规划的非线性鲁棒近似最优跟踪控制.控制理论与应用,2018,35(9):1285–1292.)[3]CUI Lili,ZHANG Yong,ZHANG Xin.Event-triggered adaptive dy-namic programming algorithm for the nonlinear zero-sum differential games.Control Theory&Applications,2018,35(5):610–618.(崔黎黎,张勇,张欣.非线性零和微分对策的事件触发自适应动态规划算法.控制理论与应用,2018,35(5):610–618.)[4]WANG D,HA M,ZHAO M.The intelligent critic framework foradvanced optimal control.Artificial Intelligence Review,2022,55(1): 1–22.[5]WANG D,QIAO J,CHENG L.An approximate neuro-optimal solu-tion of discounted guaranteed cost control design.IEEE Transactions on Cybernetics,2022,52(1):77–86.[6]YANG X,HE H.Adaptive dynamic programming for decentralizedstabilization of uncertain nonlinear large-scale systems with mis-matched interconnections.IEEE Transactions on Systems,Man,and Cybernetics:Systems,2020,50(8):2870–2882.[7]ZHAO B,LIU D.Event-triggered decentralized tracking control ofmodular reconfigurable robots through adaptive dynamic program-ming.IEEE Transactions on Industrial Electronics,2020,67(4): 3054–3064.[8]WANG Ding.Research progress on learning-based robust adaptivecritic control.Acta Automatica Sinica,2019,45(6):1037–1049.(王鼎.基于学习的鲁棒自适应评判控制研究进展.自动化学报, 2019,45(6):1037–1049.)[9]ZHANG Huaguang,ZHANG Xin,LUO Yanhong,et al.An overviewof research on adaptive dynamic programming.Acta Automatica Sini-ca,2013,39(4):303–311.(张化光,张欣,罗艳红,等.自适应动态规划综述.自动化学报, 2013,39(4):303–311.)[10]L¨U Yongfeng,TIAN Jianyan,JIAN Long,et al.Approximate-dynamic-programming H∞controls for multi-input nonlinear sys-tem.Control Theory&Applications,2021,38(10):1662–1670.(吕永峰,田建艳,菅垄,等.非线性多输入系统的近似动态规划H∞控制.控制理论与应用,2021,38(10):1662–1670.)[11]AN P,LIU M,WAN Y,et al.Multi-player H∞differential gameusing on-policy and off-policy reinforcement learning.The16th In-ternational Conference on Control and Automation.Electr Network: IEEE,2020,10:1137–1142.[12]REN H,ZHANG H,MU Y,et al.Off-policy synchronous iterationIRL method for multi-player zero-sum games with input constraints.Neurocomputing,2020,378:413–421.[13]LIU D,LI H,WANG D.Online synchronous approximate optimallearning algorithm for multiplayer nonzero-sum games with unknown dynamics.IEEE Transactions on Systems,Man,and Cybernetics: Systems,2014,44(8):1015–1027.[14]V AMVOUDAKIS K G,LEWIS F L.Non-zero sum games:Onlinelearning solution of coupled Hamilton-Jacobi and coupled Riccati equations.IEEE International Symposium on Intelligent Control.Denver,CO,USA:IEEE,2011,9:171–178.[15]ZHANG H,ZHANG K,XIAO G,et al.Robust optimal controlscheme for unknown constrained-input nonlinear systems via a plug-n-play event-sampled critic-only algorithm.IEEE Transactions on Systems,Man,and Cybernetics:Systems,2020,50(9):3169–3180.[16]HUO X,KARIMI H R,ZHAO X,et al.Adaptive-critic design fordecentralized event-triggered control of constrained nonlinear inter-connected systems within an identifier-critic framework.IEEE Trans-actions on Cybernetics,2022,52(8):7478–7491.[17]YANG X,HE H.Event-triggered robust stabilization of nonlin-ear input-constrained systems using single network adaptive critic designs.IEEE Transactions on Systems,Man,and Cybernetics:Sys-tems,2020,50(9):3145–3157.[18]WANG L,CHEN C L P.Reduced-order observer-based dynamicevent-triggered adaptive NN control for stochastic nonlinear systems subject to unknown input saturation.IEEE Transactions on Neural Networks and Learning Systems,2021,32(4):1678–1690.[19]YANG X,ZHU Y,DONG N,et al.Decentralized event-driven con-strained control using adaptive critic designs.IEEE Transactions on Neural Networks and Learning Systems,2022,33(10):5830–5844.[20]WANG D,ZHAO M,QIAO J.Intelligent optimal tracking withasymmetric constraints of a nonlinear wastewater treatment system.International Journal of Robust and Nonlinear Control,2021,31(14): 6773–6787.[21]LI M,WANG D,QIAO J,et al.Neural-network-based self-learningdisturbance rejection design for continuous-time nonlinear con-strained systems.Proceedings of the40th Chinese Control Confer-ence.Shanghai,China:IEEE,2021,7:2179–2184.[22]SU H,ZHANG H,JIANG H,et al.Decentralized event-triggeredadaptive control of discrete-time nonzero-sum games over wireless sensor-actuator networks with input constraints.IEEE Transactions on Neural Networks and Learning Systems,2020,31(10):4254–4266.[23]YANG X,HE H.Event-driven H∞-constrained control using adap-tive critic learning.IEEE Transactions on Cybernetics,2021,51(10): 4860–4872.[24]ABU-KHALAF M,LEWIS F L.Nearly optimal control laws for non-linear systems with saturating actuators using a neural network HJB approach.Automatica,2005,41(5):779–791.[25]HORNIK K,STINCHCOMBE M,WHITE H.Universal approxima-tion of an unknown mapping and its derivatives using multilayer feed-forward networks.Neural Networks,1990,3(5):551–560.[26]LEWIS F L,JAGANNATHAN S,YESILDIREK A.Neural NetworkControl of Robot Manipulators and Nonlinear Systems.London:Tay-lor&Francis,1999.作者简介:李梦花博士研究生,目前研究方向为自适应动态规划、智能控制,E-mail:*********************;王鼎教授,博士生导师,目前研究方向为智能控制、强化学习,E-mail:*****************.cn;乔俊飞教授,博士生导师,目前研究方向为智能计算、智能优化控制,E-mail:***************.cn.。
Teece(蒂斯)——动态能力与战略管理中译版
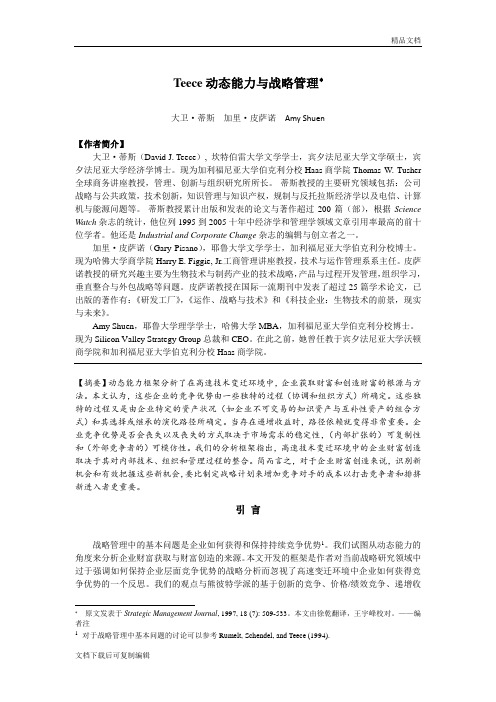
Teece动态能力与战略管理*大卫·蒂斯加里·皮萨诺Amy Shuen【作者简介】大卫·蒂斯(David J. Teece), 坎特伯雷大学文学学士,宾夕法尼亚大学文学硕士,宾夕法尼亚大学经济学博士。
现为加利福尼亚大学伯克利分校Haas商学院Thomas W. Tusher 全球商务讲座教授,管理、创新与组织研究所所长。
·蒂斯教授的主要研究领域包括:公司战略与公共政策,技术创新,知识管理与知识产权,规制与反托拉斯经济学以及电信、计算机与能源问题等。
·蒂斯教授累计出版和发表的论文与著作超过200篇(部),根据Science Watch杂志的统计,他位列1995到2005十年中经济学和管理学领域文章引用率最高的前十位学者。
他还是Industrial and Corporate Change杂志的编辑与创立者之一。
加里·皮萨诺(Gary Pisano),耶鲁大学文学学士,加利福尼亚大学伯克利分校博士。
现为哈佛大学商学院Harry E. Figgie, Jr.工商管理讲座教授,技术与运作管理系系主任。
皮萨诺教授的研究兴趣主要为生物技术与制药产业的技术战略,产品与过程开发管理,组织学习,垂直整合与外包战略等问题。
皮萨诺教授在国际一流期刊中发表了超过25篇学术论文,已出版的著作有:《研发工厂》,《运作、战略与技术》和《科技企业:生物技术的前景,现实与未来》。
Amy Shuen,耶鲁大学理学学士,哈佛大学MBA,加利福尼亚大学伯克利分校博士。
现为Silicon Valley Strategy Group总裁和CEO。
在此之前,她曾任教于宾夕法尼亚大学沃顿商学院和加利福尼亚大学伯克利分校Haas商学院。
【摘要】动态能力框架分析了在高速技术变迁环境中,企业获取财富和创造财富的根源与方法。
本文认为,这些企业的竞争优势由一些独特的过程(协调和组织方式)所确定。
一种求解Job-Shop调度问题的新型蚁群算法
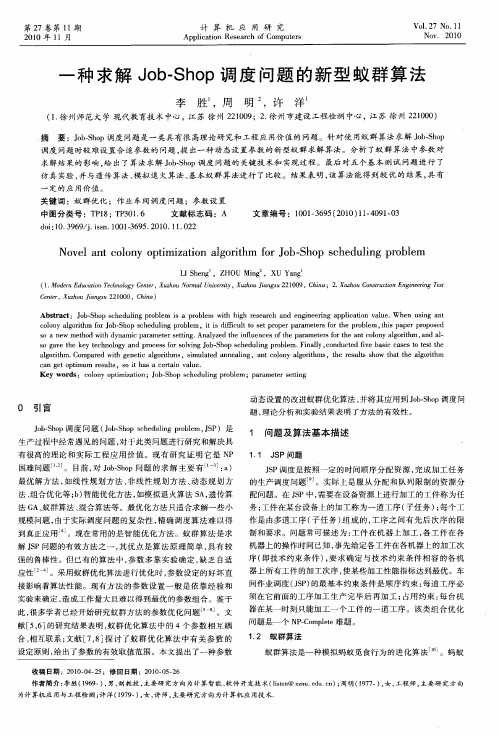
调度 问题 时较难 设置合 适参 数 的问题 , 出一种 动 态设 置参数 的新 型蚁 群 求解算 法。分析 了蚁 群 算 法 中参 数 对 提
求解结果 的影 响 , 出了算法 求解 JbS o 给 o .h p调度 问题 的 关键 技术 和 实现过程 。最后 对 五个基 本测试 问题进 行 了
S a e t e k y tc n lg n r c s rs l ig J b S o c e u i g p o l m. i al , o d c e v a i c s st e t h O g v h e e h oo y a d p o e sf o vn o — h p s h d l r b e F n l c n u td f e b s a e t s t e o n y i c o
Cne, uh uJagu2 10 C ia et X zo i s 20 0, hn ) r n
Ab t a t o — h p s h d l g p o lm sa p o l m wi ih r s a c n n i e r g a p ia in v le W h n u i g a t s r c :J b S o c e u i r b e i r b e t hg e e r h a d e g n e n p l t au . n h i c o e s n n c l n l o t m o o — h p s h d l g p o lm ,i i df c l t e r p rp rmee sfr t e p o l m,h sp p rp o o e o o y ag r h frJ b S o c e u i r be i n t s i ut o s tp o e a a tr o h r b e ti a e r p s d i