Improved Prefix Based Format-Preserving Encryption
Improvements in MLLRtransform-based speaker recognition
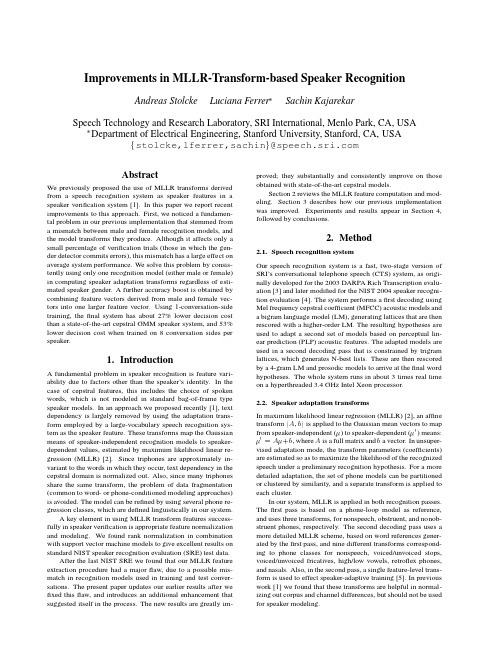
Improvements in MLLR-Transform-based Speaker Recognition Andreas Stolcke Luciana Ferrer Sachin KajarekarSpeech Technology and Research Laboratory,SRI International,Menlo Park,CA,USA Department of Electrical Engineering,Stanford University,Stanford,CA,USA stolcke,lferrer,sachin@AbstractWe previously proposed the use of MLLR transforms derived from a speech recognition system as speaker features in a speaker verification system[1].In this paper we report recent improvements to this approach.First,we noticed a fundamen-tal problem in our previous implementation that stemmed from a mismatch between male and female recognition models,and the model transforms they produce.Although it affects only a small percentage of verification trials(those in which the gen-der detector commits errors),this mismatch has a large effect on average system performance.We solve this problem by consis-tently using only one recognition model(either male or female) in computing speaker adaptation transforms regardless of esti-mated speaker gender.A further accuracy boost is obtained by combining feature vectors derived from male and female vec-tors into one larger feature ing1-conversation-side training,thefinal system has about27%lower decision cost than a state-of-the-art cepstral GMM speaker system,and53% lower decision cost when trained on8conversation sides per speaker.1.IntroductionA fundamental problem in speaker recognition is feature vari-ability due to factors other than the speaker’s identity.In the case of cepstral features,this includes the choice of spoken words,which is not modeled in standard bag-of-frame type speaker models.In an approach we proposed recently[1],text dependency is largely removed by using the adaptation trans-form employed by a large-vocabulary speech recognition sys-tem as the speaker feature.These transforms map the Gaussian means of speaker-independent recognition models to speaker-dependent values,estimated by maximum likelihood linear re-gression(MLLR)[2].Since triphones are approximately in-variant to the words in which they occur,text dependency in the cepstral domain is normalized out.Also,since many triphones share the same transform,the problem of data fragmentation (common to word-or phone-conditioned modeling approaches) is avoided.The model can be refined by using several phone re-gression classes,which are defined linguistically in our system.A key element in using MLLR transform features success-fully in speaker verification is appropriate feature normalization and modeling.We found rank normalization in combination with support vector machine models to give excellent results on standard NIST speaker recognition evaluation(SRE)test data.After the last NIST SRE we found that our MLLR feature extraction procedure had a majorflaw,due to a possible mis-match in recognition models used in training and test conver-sations.The present paper updates our earlier results after we fixed thisflaw,and introduces an additional enhancement that suggested itself in the process.The new results are greatly im-proved;they substantially and consistently improve on those obtained with state-of-the-art cepstral models.Section2reviews the MLLR feature computation and mod-eling.Section3describes how our previous implementation was improved.Experiments and results appear in Section4, followed by conclusions.2.Method2.1.Speech recognition systemOur speech recognition system is a fast,two-stage version of SRI’s conversational telephone speech(CTS)system,as origi-nally developed for the2003DARPA Rich Transcription evalu-ation[3]and later modified for the NIST2004speaker recogni-tion evaluation[4].The system performs afirst decoding using Mel frequency cepstral coefficient(MFCC)acoustic models and a bigram language model(LM),generating lattices that are then rescored with a higher-order LM.The resulting hypotheses are used to adapt a second set of models based on perceptual lin-ear prediction(PLP)acoustic features.The adapted models are used in a second decoding pass that is constrained by trigram lattices,which generates N-best lists.These are then rescored by a4-gram LM and prosodic models to arrive at thefinal word hypotheses.The whole system runs in about3times real time on a hyperthreaded3.4GHz Intel Xeon processor.2.2.Speaker adaptation transformsIn maximum likelihood linear regression(MLLR)[2],an affine transform is applied to the Gaussian mean vectors to map from speaker-independent()to speaker-dependent()means: ,where is a full matrix and a vector.In unsuper-vised adaptation mode,the transform parameters(coefficients) are estimated so as to maximize the likelihood of the recognized speech under a preliminary recognition hypothesis.For a more detailed adaptation,the set of phone models can be partitioned or clustered by similarity,and a separate transform is applied to each cluster.In our system,MLLR is applied in both recognition passes. Thefirst pass is based on a phone-loop model as reference, and uses three transforms,for nonspeech,obstruent,and nonob-struent phones,respectively.The second decoding pass uses a more detailed MLLR scheme,based on word references gener-ated by thefirst pass,and nine different transforms correspond-ing to phone classes for nonspeech,voiced/unvoiced stops, voiced/unvoiced fricatives,high/low vowels,retroflex phones, and nasals.Also,in the second pass,a single feature-level trans-form is used to effect speaker-adaptive training[5].In previous work[1]we found that these transforms are helpful in normal-izing out corpus and channel differences,but should not be used for speaker modeling.2.3.Feature extraction and SVM modelingThe coefficients from one or more adaptation transforms are concatenated into a single feature vector and modeled using support vector machines.The data used is from conversational telephone speech,and each conversation side is processed as a unit by the speech recognition system.Consequently,each conversation side produces a single set of adaptation transforms pertaining to the same speaker,and hence a single feature vec-tor.Since our acoustic features(after dimensionality reduction) contain39components,the number of SVM feature compo-nents will equal the number of transforms.The trans-form for nonspeech(pause)models is left out of the feature vec-tor,since it cannot be expected to help in speaker recognition.An SVM is trained for each target speaker using the feature vectors from a background training set as negative examples(of which there are many,typically in the thousands),and the tar-get speaker training data as positive examples(of which there are few,typically1or8).To compensate for the severe imbal-ance between the target and background data,we adopted a cost model[6]to weight the positive examples500-fold with respect to the negative examples.Throughout,a linear inner-product kernel function was used for SVM training.Prior to SVM training or testing,features need to be nor-malized to equate their dynamic ranges.To this end,we ap-ply rank normalization,replacing each feature value by its rank among all the background data samples on a given dimension, and then scaling ranks to a value between0and1.Rank normal-ization not only scales the feature distribution to afixed interval, it also warps the distribution to be approximately uniform.This has the intuitive effect that the distance between two datapoints (along a single dimension)becomes proportional to the percent-age of the population that lies between them.An alternative to feature normalization is to optimize the kernel function explicitly for minimal classification error.This can be done in a number of ways,for example,by applying scal-ing factors to subfeature-vectors[7],or by introducing a scaling matrix derived from the within-speaker variances[8].However, neither of these methods was used here.2.4.Baseline systemsIn evaluating MLLR-feature-based speaker recognition sys-tems,we compared results to two state-of-the-art cepstral fea-ture systems.Thefirst baseline system is a Gaussian mixture model(GMM)with universal background model(UBM)[9], based on13MFCCs(without C0)andfirst-,second-,and third-order difference features.The features are mean-subtracted and modeled by2048mixture components.Gender-handset models are adapted from this model and used for feature transforma-tion[10].Thefinal features are mean and variance normalized at the utterance level.The detection score is the target/UBM likelihood ratio after TNORM[11].The second baseline system is also based on MFCCs(with first-and second-order differences),followed by the same fea-ture transformation and normalization steps.Thefinal features are then modeled with SVMs utilizing the polynomial sequence kernel proposed by[12],with some recently developed en-hancements[13].Principal component analysis is performed on the polynomial feature space,and the features are projected onto the subspace spanned by the background speaker set,as well as its orthogonal complement(there are more feature di-mensions than background speakers).This process is then car-ried out twice,for two different feature normalization variants, and four separate SVM models are trained.The overall system score is the sum of the four SVM scores,after TNORM.This enhanced cepstral SVM system was the single best-performing component of SRI’s NIST2005speaker recognition system [14].3.Improvements3.1.Gender issuesThe adaptation transforms are dependent on the recognition models relative to which they are computed.Features derived from the transforms can be compared meaningfully only if they were computed relative to the same recognition models.This becomes an issue if a recognition system uses multiple mod-els,for example,if recognition models are gender dependent, as is the case for our recognizer.Still,in principle gender de-pendency should not be a problem because of the special way in which trials in the NIST SRE data sets(as well as our own development data)are constructed.NIST SRE trials always have the same speaker gender(either all male or all female) in target training and test conversations.Presumably,gender mismatches between training and test data are considered un-interesting,since they would be too easy to classify as speaker mismatches.Unfortunately,the automatic gender classification per-formed by our recognizer makes a nonnegligible number of er-rors.After the2005NIST SRE,we realized that this led to a significant number of trials(on the order of20%)in which at least one of the conversation sides had incorrect gender,and hence mismatched MLLR transform features.In the following, we refer to such trials as“gender mismatched”,even though this refers only to the automatically detected gender according to our speech recognition system.1Table1shows the average target and impostor trial scores, as well as equal error rates(EERs)for trials in which a gender mismatch occurs,and compares them to the statistics for trials overall.These statistics are given for a standard cepstral GMM system as well as the MLLR SVM system described in[1].The data is drawn from the Fisher collection,and has one training conversation side per target speaker.The table shows that the MLLR system scores drop dramatically for trials with gender mismatch,unlike for the cepstral GMM.As a result,the MLLR system performs almost at chance level(46%equal error)for gender-mismatched cases.3.2.Fixing MLLR gender mismatchOne solution to the problem of gender-mismatched trials is to compute MLLR transforms with gender-independent mod-els.However,in our case this would have meant retraining the recognition system from scratch,a rather involved process.In-stead,we decided to simply use one of the gender-dependent models(male or female)for all speaker samples.This means that gender-dependent processing steps(vocal tract length nor-malization and adaptation)are rerun with the opposite gender for about half the conversation sides.The recognition hypothe-ses used to compute the second,more detailed MLLR trans-forms,were kept unchanged from the original system,so no additional decoding was necessary relative to the original sys-tem.Table1:System output(scores and EER)comparison depending on whether the target training speaker and the test speaker are mismatched in automatic gender detection.Impostor trial scoresGender outputs mismatched mismatched mismatched7.000.133 3.99%6.350.065 5.19%SWB-II SRE-04English-onlyTraining8-side1-side1-sideConv.sides30581384578734225384Trials49111531720907FisherMLLR gender1-side5.57 4.96(old system).33182Male 6.25.06095.120532.983.21.29092Male+Female 5.34.05493.11767Systems were optimized using the Fisher and SRE-04datasets,and we give results on these to illustrate certain contraststhat guided our development.Final systems are then tested onall data sets.4.2.MLLR system resultsMLLR systems based on the more detailed(8)transforms fromthe second adaptation step give the best results,based on ourprevious experiments.Table3summarizes results on the datasets used for development,in terms of both minimum detectioncost function(DCF)2and EER.The four rows of results corre-spond to the original MLLR system that is affected by the gen-der mismatch problem,a system based on“male”transforms,a system based on“female”transforms,and one in which bothkinds of features are concatenated.It is clear thatfixing the gender mismatch problem reducesoverall equal error rates substantially,by31%to35%for theSRE-04data sets.(The mismatch problem is more severe onMixer data than on Fisher,due to higher gender classificationerror rates.)DCFs are reduced by12%to33%relative on SRE-04.Male and female transforms give approximately equal re-bining the two sets of transforms yields an additionalgain.EERs on SRE-04are reduced by an additional12%toTable5:Speaker verification results using baseline,MLLR,and combined systems.The MLLR SVM systems uses8+8transforms (same as last row in Table3)or2+2transforms(last row in Table4).The last row represents a three-way combined system.Fisher SRE-051-side1-side8-side8-sideMFCC GMM 1.927.777.17.17857.10259.21146.168864.38 4.31 3.33 3.05.04470.31339.26839MLLR(2+2)SVM 1.128.227.91.18130.05756.16283.157743.00 2.85 2.62 2.45.02419.25640.179504.01+MLLR(2+2)SVM.229313.11+MFCC SVM.21485MFCC GMM 4.84.070952.15+MLLR(8+8)SVM.1578MFCC(GMM+SVM) 4.61.06332Table4:Speaker verification results using MLLR features from1st adaptation stage(2transforms).The top number(in italics)in each table cell is the EER(%).The bottom number is theminimum DCF value.SRE-041-side8-sideMixed12.38.09934.199343.454.66.37794Female9.07.06153.171582.98 4.37.33962False Alarm probability (in %)M i s s p r o b a b i l i t y (i n %)Figure 1:Detection error tradeoff (DET)curves for baseline,8+8-transform MLLR,and combined systems,SRE-051-conversation-side condition.decoding pass.5.Conclusions and Future WorkWe have discovered and corrected a major problem in our previ-ous implementation of MLLR-transform-based speaker recog-nition,which was the result of gender classification errors in the speech recognition system.We fixed the resulting gender mis-match in speaker trials,and came upon a further improvement by combining transforms relative to multiple recognition mod-els (male and female,in our case).Our improved MLLR-based SVM speaker verification system now gives markedly better re-sults than our current cepstral GMM and SVM -bining the MLLR systems with these baseline systems gives a significant further error reduction.We plan to investigate MLLR for speech recognition in other languages,including the case where a trial involves a mix of several languages.6.AcknowledgmentsWe thank the anonymous reviewers for valuable suggestions,Ramana Gadde for clarifications of the MLLR implementation,and our colleagues Liz Shriberg,Kemal Sonmez,and Andy Hatch for fruitful discussions.This work was funded by a DoD KDD award via NSF IRI-9619921.The views herein are those of the authors and do not reflect the views of the funding agen-cies.7.References[1] A.Stolcke,L.Ferrer,S.Kajarekar, E.Shriberg,andA.Venkataraman,“MLLR transforms as features in speaker recognition”,in Proc.Interspeech ,pp.2425–2428,Lisbon,Sep.2005.[2] C.Leggetter and P.Woodland,“Maximum likelihood lin-ear regression for speaker adaptation of HMMs”,Com-puter Speech and Language ,vol.9,pp.171–186,1995.[3] A.Stolcke,H.Franco,R.Gadde,M.Graciarena,K.Pre-coda,A.Venkataraman,D.Vergyri,W.Wang,J.Zheng,Y .Huang, B.Peskin,I.Bulyko,M.Ostendorf,and K.Kirchhoff,“Speech-to-text research at SRI-ICSI-UW”,in DARPA RT-03Workshop ,Boston,May 2003,/speech/tests/rt/rt2003/spring/presentations/sri+-rt03-stt.pdf.[4]S.S.Kajarekar,L.Ferrer,E.S.K.Sonmez,A.Stol-cke,A.Venkataraman,and J.Zheng,“SRI’s 2004NIST speaker recognition evaluation system”,in Proc.ICASSP ,vol.1,pp.173–176,Philadelphia,Mar.2005.[5]H.Jin,S.Matsoukas,R.Schwartz,and F.Kubala,“Fastrobust inverse transform SAT and multi-stage adaptation”,in Proceedings DARPA Broadcast News Transcription and Understanding Workshop ,pp.105–109,Lansdowne,V A,Feb.1998.Morgan Kaufmann.[6]K.Morik,P.Brockhausen,and T.Joachims,“Combiningstatistical learning with a knowledge-based approach—a case study in intensive care monitoring”,in I.Bratko and S.Dzeroski,editors,Proceedings of the 16th InternationalFalse Alarm probability (in %)M i s s p r o b a b i l i t y (i n %)Figure 2:Detection error tradeoff (DET)curves for baseline,8+8-transform MLLR,and combined systems,SRE-058-conversation side condition.Conference on Machine Learning ,pp.268–277,San Fran-cisco,CA,1999.Morgan Kaufmann.[7]A.O.Hatch,A.Stolcke,andB.Peskin,“Combining fea-ture sets with support vector machines:Application to speaker recognition”,in Proceedings IEEE Workshop on Speech Recognition and Understanding ,pp.75–79,San Juan,Puerto Rico,Nov.2005.[8]A.O.Hatch and A.Stolcke,“Generalized linear kernels for one-versus-all classification:Application to speaker recognition”,in Proc.ICASSP ,Toulouse,May 2006.[9]D.A.Reynolds,T.F.Quatieri,and R.B.Dunn,“Speaker verification using adapted Gaussian mixture models”,Digital Signal Processing ,vol.10,pp.19–41,2000.[10]D.A.Reynolds,“Channel robust speaker verification via channel mapping”,in Proc.ICASSP ,vol.2,pp.53–56,Hong Kong,Apr.2003.[11]R.Auckenthaler,M.Carey,and H.Lloyd-Thomas,“Score normalization for text-independent speaker verification systems”,Digital Signal Processing ,vol.10,Jan.2000.[12]W.M.Campbell,“Generalized linear discriminant se-quence kernels for speaker recognition”,in Proc.ICASSP ,vol.1,pp.161–164,Orlando,FL,May 2002.[13]S.S.Kajarekar,“Four weightings and a fusion:A cepstral-SVM system for speaker recognition”,in Pro-ceedings IEEE Workshop on Speech Recognition and Un-derstanding ,pp.17–22,San Juan,Puerto Rico,Nov.2005.[14]L.Ferrer, E.Shriberg,S.S.Kajarekar, A.Stolcke,K.S¨o nmez, A.Venkataraman,and H.Bratt,“Thecontribution of cepstral and stylistic features to SRI’s2005NIST speaker recognition evaluation system”,in Proc.ICASSP ,Toulouse,May 2006.[15] A.Martin,ler,M.Przybocki,J.Campbell,andH.Nakasone,“Conversational telephone speech corpus collection for the NIST speaker recognition evaluation 2004”,in Proceedings 4th International Conference on Language Resources and Evaluation ,pp.587–590,Lis-bon,May 2004.。
用后验信噪比修正小波包自适应阈值的语音增强算法
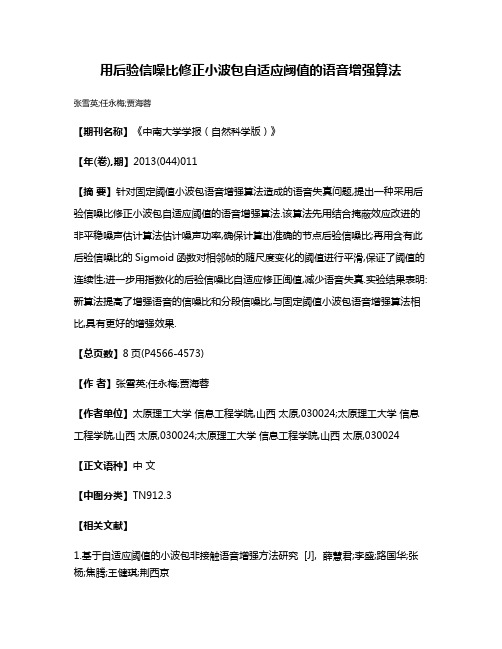
用后验信噪比修正小波包自适应阈值的语音增强算法
张雪英;任永梅;贾海蓉
【期刊名称】《中南大学学报(自然科学版)》
【年(卷),期】2013(044)011
【摘要】针对固定阈值小波包语音增强算法造成的语音失真问题,提出一种采用后验信噪比修正小波包自适应阈值的语音增强算法.该算法先用结合掩蔽效应改进的非平稳噪声估计算法估计噪声功率,确保计算出准确的节点后验信噪比;再用含有此后验信噪比的Sigmoid函数对相邻帧的随尺度变化的阈值进行平滑,保证了阈值的连续性;进一步用指数化的后验信噪比自适应修正闽值,减少语音失真.实验结果表明:新算法提高了增强语音的信噪比和分段信噪比,与固定阈值小波包语音增强算法相比,具有更好的增强效果.
【总页数】8页(P4566-4573)
【作者】张雪英;任永梅;贾海蓉
【作者单位】太原理工大学信息工程学院,山西太原,030024;太原理工大学信息工程学院,山西太原,030024;太原理工大学信息工程学院,山西太原,030024
【正文语种】中文
【中图分类】TN912.3
【相关文献】
1.基于自适应阈值的小波包非接触语音增强方法研究 [J], 薛慧君;李盛;路国华;张杨;焦腾;王健琪;荆西京
2.一种新的自适应阈值小波包语音增强算法 [J], 任永梅;肖冬瑞
3.基于新阈值函数和自适应阈值的小波包语音增强研究 [J], 刘冲冲;邹翔;周正仙
4.联合改进子空间的自适应小波包阈值语音增强算法 [J], 任永梅;张雪英;贾海蓉;贾雅琼
5.基于双正交小波包分解的自适应阈值语音增强 [J], 李如玮;鲍长春;窦慧晶
因版权原因,仅展示原文概要,查看原文内容请购买。
211232484_基于改进FA_算法与不完全Beta_函数的图像增强技术

第40卷第2期Vol.40㊀No.2重庆工商大学学报(自然科学版)J Chongqing Technol &Business Univ(Nat Sci Ed)2023年4月Apr.2023基于改进FA 算法与不完全Beta 函数的图像增强技术沈汝涵,周孟然,凌㊀胜安徽理工大学力学与光电物理学院,安徽淮南232001摘㊀要:针对传统计算机在复杂图像信息分析以及后期处理不达预期的问题,提出了利用改进原始的萤火虫算法(FA )在不完全Beta 函数上动态寻优调整灰度曲线的光电图像增强新策略㊂新策略主要从算法角度出发改进传统FA 算法,针对原有的吸引度容易造成局部最优等问题引入新吸引度公式㊁针对算法陷入局部震荡添加自扰动和克服陷入局部最优的迭代检测环节,改进完成的新算法(Firefly Algorithm Growth ,FAG )结合非完全Beta 函数动态寻找最优值下的图像灰度曲线㊂将改进的FAG 与FA 新老算法在四种常见基准函数上进行对比实验测试他们的性能,结果显示改良FAG 算法在性能上更优;在改良FAG 结合非完全Beta 与FA 结合非完全Beta 增强同一图像的实验中加入直方图算法增强图像作为对照组,综合结果显示改进后的新策略更胜一筹㊂综合结果显示群智能算法在结合图像处理手段来达到图像增强的目的上具有很好的应用价值,新策略在低对比度条件下的光电图像实现了有效的增强㊂关键词:萤火虫算法;不完全Beta 函数;图像增强;融合算法中图分类号:TP301.6㊀㊀文献标识码:A ㊀㊀doi:10.16055/j.issn.1672-058X.2023.0002.009㊀㊀㊀㊀㊀㊀㊀㊀㊀㊀㊀㊀㊀㊀㊀㊀㊀㊀㊀㊀㊀㊀㊀㊀㊀㊀㊀㊀㊀㊀㊀㊀㊀㊀㊀㊀㊀㊀㊀㊀㊀㊀㊀㊀㊀㊀㊀㊀㊀㊀㊀㊀㊀㊀㊀㊀㊀㊀㊀㊀㊀㊀㊀㊀㊀㊀㊀㊀㊀㊀㊀㊀㊀收稿日期:2022-01-10㊀修回日期:2022-02-25㊀文章编号:1672-058X(2023)02-0057-07作者简介:沈汝涵(1997 ),男,安徽淮南人,硕士研究生,从事智能控制与图像处理研究.引用格式:沈汝涵,周孟然,凌胜.基于改进FA 算法与不完全Beta 函数的图像增强技术[J].重庆工商大学学报(自然科学版),2023,40(2):57 63.SHEN Ruhan,ZHOU Mengran,LING Sheng.Image enhancement technology based on improved FA algorithm and incompleteBeta function[J].Journal of Chongqing Technology and Business University (Natural Science Edition),2023,40(2):57 63.Image Enhancement Technology Based on Improved FA Algorithm and Incomplete Beta Function SHEN Ruhan,ZHOU Mengran,LING ShengSchool of Mechanics and Optoelectronic Physics,Anhui University of Science and Technology,Anhui Huainan 232001,ChinaAbstract :Aiming at the problem that the traditional computer cannot meet the expectation in the information analysis and post -processing of complex images,a new photoelectric image enhancement strategy was proposed,which used the improved original firefly algorithm (FA)to dynamically optimize and adjust the gray curve on the incomplete Beta function.The new strategy mainly improved the traditional FA algorithm from the perspective of the algorithm,introduced a new attraction formula for the original attraction degree that was prone to local optimality,added self -perturbation to solve the problem that the algorithm fell into local oscillation,and avoided the iterative detection link that fell into local optimality.The improved new Firefly Algorithm Growth (FAG)was combined with an incomplete Beta function to dynamically search for image gray curve under optimal value.The improved FAG was compared with the old and new FA algorithms on four common benchmark functions to test their performance,and the results showed that the improved FAG algorithm was superior in terms of performance.In experiments where the same image was enhanced by the improved FAG combined with incomplete Beta and FA combined with incomplete Beta,the histogram algorithm was added to enhance the image as the control group,and the comprehensive results showed that the improved new strategy was superior.The results show that the swarm intelligence algorithm has a good application value in the combination of image processing means to achieve the purpose of image enhancement.The new strategy can effectively enhance photoelectric images under the condition of low contrast.Keywords :firefly algorithm;incomplete Beta function;image enhancement;fusion algorithm重庆工商大学学报(自然科学版)第40卷1㊀引㊀言人们的日常生活中缺少不了光电图像增强,不仅实用且应用广泛㊂针对不同的场合,增强后的图像不仅能够提升感兴趣部分,压抑不感兴趣的部分来提升整体对比度以获取更多的信息㊂常见的处理方法包括两种,一是直接对图像灰度级进行处理的算法,通常认为这是在空域操作,另外一种则是基于频域改变图像的变换系数值㊂不同非线性函数应用在不同灰度特性图像上,快速寻找出适合的非线性函数是待解决的主要问题㊂古良玲[1]对非完全beta函数进行改进,很好地解决了因确定Beta函数参数具有主观性㊁盲目性从而导致的图像增强效果不显著的问题,利用模拟退火算法提升了寻找参数的速度㊂王小芳[2]提出一种模糊蚁群算法,将图像经过灰度线性变换后将边缘点进行聚类,再利用蚁群搜索之后的多张边界图进行叠加得到增强的图片㊂两者的研究都是利用了群算法在快速搜索区域内最优解的基础上改良图像处理方法,前者关注点为图像的灰度曲线的自适应获取,后者则是图片的混合增强来增强图像边缘,为改进策略开拓了两种不同的思路㊂但古良玲采用的模拟退火算法在性能上仍然欠优,采用新的群算法会有更好的提升空间;王小芳混合图片只是对于前者的变化后的图片进行多次叠加来获取最优,容易引入人为误差㊂FATEENSEK[3]提出了IFA(Intelligent Firefly Algori thm)㊂IFA能高效解决全局优化的问题㊂这种改进从算法的底层逻辑加以完善,将原有的萤火虫位置公式从有规律可循变成随机形式,增加了算法的随机性㊂张慧宁[4]针对早熟收敛现象在萤火虫算法后期影响寻优而改进局部扰动,防止陷入局部最优,提高了局部寻优的效率,很好地提升了田舍的边界的区分度㊂俞文静[5]针对萤火虫算法改进位置更新策略克服了陷入极值的问题,采用自适应变步长策略同时改进了荧光素α的挥发与增益效率β,算法收敛性与图片清晰度都有一定的提升㊂张慧宁㊁俞文静的贡献是针对萤火虫陷入局部震荡的缺陷进行改良,效果显著㊂郭红山[6]对红外热区进行快速确定标示,用萤火虫优化算法进行半径动态数据更新在其决策域内,结果表明FA算法在增强红外图像领域效果显著㊂另外还有很多学者在群算法以及创新改进图像处理手段方面做出自己的贡献㊂本文研究内容主要从改进算法角度出发,改进原始FA函数,下文简称新函数FAG,改进的算法包括了从算法的初始化阶段的更新,以及核心的吸引力公式的改良㊂此外参考古良玲所采用的非完全Beta函数,利用改进的新群智能算法拟合非完全beta函数寻找图像的灰度曲线的最优值来增强图像㊂2㊀算㊀法2.1㊀萤火虫算法萤火虫算法(Firefly Algorithm,FA):一种新型群智能优化算法㊂标准萤火虫提出基于三个理论:其一是萤火虫不分性别,这样所有的萤火虫都可不分性别吸引周围的萤火虫,这种观念区分于其他群居算法例如狮群算法的性别分工以及蚁群算法的蚁后;第二点是萤火虫之间的吸引力随着荧光的亮度增加而增加与随距离的增加而减少;第三点萤火虫亮度应由待优化的目标函数决定[7]㊂总结来说就是萤火虫自身因目标函数而存在光亮,带较暗荧光的萤火虫会被亮度较亮的萤火虫所吸引,也就是带有更好的目标函数的萤火虫,并且向之移动且更新自身的定位,而相同亮度的萤火虫不会互相吸引,而是随机运动㊂萤火虫的荧光相对亮度计算为l=l0ˑe-γr ij(1)笛卡尔距离r ij计算公式如下:r ij= x i-x j =ðd k=1x i,k-x j,k()2(2) l0为初始亮度㊂吸引力的定义为如下:β=β0ˑe-γr2ij(3)式(3)中的β0为最大吸引强度,萤火虫移动的距离与吸引度的值成正比,从多篇文献的实验结果来看β0通常可以取值为1[8]㊂γ的值代表了空气吸收光率,取值范围通常在(0.1,10)之间,不同的问题在取值的时候差异会较大㊂位置更新函数:x^i=x i+βˑx j-x i()+αεj(4) x i与x j是萤火虫的各自位置,x^i为更新后空间位置,步长α范围在(0,1)之间,εj是随机数向量㊂2.2㊀图像的非线性增强图像的线性增强主要通过线性函数,主要的思想是通过将图像的灰度范围压缩或者拓展变成一个新的灰度范围即增强原图里的两个灰度值间的动态范围来拉伸感兴趣的区间从而达到增强图像的目的㊂而非线性图像增强则是通过非线性映射算法,通常我们常用的方法是通过几种运算,指数㊁对数运算以及幂运算㊂非线性变换往往通过灰度压缩,来增强其他范围的图像信息来改善整张图片的信息㊂更常见的就是直方图85第2期沈汝涵,等:基于改进FA 算法与不完全Beta 函数的图像增强技术增强,直方图通过积分概率密度函数转化为概率密度为1的图像,从而达到增强图像的作用,直方图均衡化的实质也是一种特定区域的展宽,但是会导致图像曝光严重,造成一定失真㊂当原始图像给定时,对应的直方图增强的效果也是固定的,所以应用的场景较为简单,不能处理复杂的图像㊂丁生荣[9]提出一种基于非完全Beta 函数的图像增强算法,此文解决了遗传算法等自适应确定Beta 函数参数㊂其实也就是选择优化非线性函数的取值,最优参数的获得意味着最优的灰度变化曲线,即可以自适应并且增强图像㊂首先将不完全Beta 函数归一化:F (α,β,x )=B -1(α,β)x ʏx 0t α-1(1-t )β-1dt(0<α,β<10)(5)其中B(α,β)=Γ(α)Γ(β)/Γ(α+β),通过调整α,β参数可以得到四种非线性曲线㊂当α>β时对亮区进行拉升,α<β则是增大暗区的灰度差别,α=β=1时为直线㊂处理步骤如下:先判断图像的色彩如果是彩色则处理如下:f ᶄ(x ,y )=f (x ,y )-G minG max -G min(6)f ᶄ(x ,y )表示归一化后的灰度值,G max 和G min 是灰度的最大与最小值,f (x ,y )ɪ[G max ,G min ],所以f ᶄ(x ,y )ɪ[0,1]㊂F (u )为归一化后的非完全Beta 函数(0<0<1),公式如下:gᶄ(x ,y )=F f ᶄ(x ,y )()0ɤgᶄ(x ,y )ɤ1()(7)其中,G max =255,G min =0㊂将图像的优化函数作为图像的方差,对于M ˑN 大小的图像计算方法如下㊂f (I )=1MN ðM i =1ðN j =1I 2(x ,y )-∣1MN ðM i =1ðNj =1I (x ,y )2(8)2.3㊀经典萤火虫算法及改进算法介绍针对原始萤火虫的步长通常设置为固定值,不能在算法的前期后期很好地适应萤火虫的搜索需求的问题以及萤火虫步长周围并没有更优的荧光吸引导致保持原位置不更新等问题,众多学者采取了一些可行且有效的策略加以改进㊂FARAHANI 等[10]提出一种混合算法将GA 与FA融合将两个子群交换后再进行下一轮迭代,提高了萤火虫算法的前期搜索的效果的同时采用Gauss 函数随机设置步长㊂另外在前期的超级个体会大量地吸引其他大型个体导致了种群数数的大量下降,导致的收敛能力的下降甚至停滞㊂符强[11]在他的改进算法中提出将萤火虫分成若干个子群,利用不同的寻优方式进行迭代,其中他设置了三次迭代对比最优值的检测环节来判断算法是否陷入最优发生早熟,此外决策层萤火虫的设置为子群向有着更优值的子群学习提供了很好的例子,能够很好地跳出震荡㊂李肇基[12]在萤火虫算法中加入了进化模型改进了萤火虫的随机分布的混沌优化策略,他关注到萤火虫的初始化分布通常会导致有规律可循等问题,导致种群分布不均,混沌优化很好地解决了这种初始就聚集的情况㊂另外也提到了改进萤火虫步长,他提出的是一种权重设置,步长的权重随着萤火虫取得的最优解来自适应确定,体现出了萤火虫的差异性,增加了算法的适应性㊂左仲亮[13]在文章中提出改进轮盘赌问题中的个别萤火虫在初始化中距离最优萤火虫较远而不移动,此外还吸引了周围更劣萤火虫聚集的问题,所以他提出让这些萤火虫在初始阶段先进行20次的无序随机移动,并且比较结果,选取中间最优的位置作为更新位置㊂这种思路可以很好地解决初始位置问题㊂王航星[14]则针对这种范围内没有目标而不更新的萤火虫提出了一种自适应吸引距离,根据萤火虫自身的荧光限制周围萤火虫的被吸引数量,减少了算法跟踪更新位置而造成的过量计算,能够很好地提升算法的速度,降低运行时间㊂左仲亮做出的改进是提出优化策略使算法的初始化更加随机增加算法的算力,避免振荡陷优;李肇基建立的是萤火虫的个体差异,每一个萤火虫都是独一无二的,增加算法随机性;王航星的自适应吸引范围能够限制萤火虫的劣性聚集;符强设置决策层为萤火虫更新提供模板,众学者的改进想法为进一步改进提供了思路㊂2.4㊀改进萤火虫算法(FAG )在本文中加入随机因素来进行扰动㊂根据已有公式构建了一个新的吸引度公式如下β=β0e-γr 2iyR i(9)其中,R i 取指数分布,并且在迭代的过程中会进行位置的判定,如果在某个最优点停留的次数超过某个阈值时,会让全体点中最好的百分之二十替换最差的百分之二十,其余的点进行高斯变异㊂此外参考了GAUSS 函数随机步长设计了一种新的递减步长,有利于迭代的前几轮能够大范围有效搜索,步长衰减有利于迭代后期的精细搜索[15]㊂95重庆工商大学学报(自然科学版)第40卷α=α0δt S(10)其中,衰减系数δ取值(0,1),t 为迭代时间,目标域S 为目标函数的待优化区域㊂于是新的位置更新移动公式为x ^i =x i +β0e-γr xy 2R i (x j -x i )+α0δt S(11)2.5㊀算法FAG -N -B 增强图像算法流程(1)读入图片并判断是否为灰度图片,彩色图则进行灰度化处理和归一化处理㊂(2)初始化算法环境并定义目标函数以及设置算法参数和迭代的回数㊂(3)利用改进算法,将原步长替换为改进的衰减步长,进行位置更新并迭代,标记停留最优解超过迭代次数阈值的嫌疑个体,选取全体百分之二十的优秀个体替代最差的百分之二十个体㊂找出最优解,并赋值给拟合函数[16]㊂(4)输出反归一化的图像增强后的图片㊂3㊀实验仿真部分设计基准函数的对比实验测试并检验新算法的性能,比较新型萤火虫算法在光电图像上的增强效果㊂基准函数对比实验的主要目的是体现出新老算法在性能上的优劣,有助于下一步改进方案,图像增强对比实验是从感官和数据两方面直观体现出算法的性能的优劣㊂此次实验环境为Window 10系统,处理器为Intercore -I5-5200u -2.20HZ,运行内存8GB 的计算机,开发软件使用的是MatlabR2020b㊂3.1㊀基准函数对比试验本文采用4组实验对照新老算法在基准函数上的收敛以及寻优情况(表1)㊂种群规模设置为30,迭代500次㊂变量的上边界以及下边界设置为10和0.5,初始步长设置为0.2,递减系数δ取0.95[17]㊂表1㊀基准函数Table 1㊀Reference function基准函数表达式F 1=1+14000ðDi =1x 2i-ᵑD i =1cosx ii()F 2=max x i x i ,1ɤi ɤn {}F 3=ðn i =1x2i -10cos 2πx i ()+10[]F 4=-20exp -151nðn i =1x2i()-exp1nðn i =1cos (2πx )i()(+20+e在基准函数F 2下可以看到改进FAG 收敛速度与趋势明显较传统FA 加快,且传统FA 算法在迭代50次附近,以及到100次附近跳出局部最优的效果并不好,在120次迭代附近彻底收敛㊂从F 1基准函数迭代曲线看出FAG 收敛速度前期20次迭代次数内不如原始FA,但是在解决陷入最优解的问题方面以及过早收敛的方面表现更优,F 3,F 4并且基本在迭代30次左右就能彻底超过FA,并且在基准函数F 3测试中FAG 在50次㊁100次左右以及250次以后的克服陷入局部最优的自动调节得到较好地体现(图1)㊂I t e r a t i o n 100200300400500O p t i m u m f i t n e s s1010-10B e s t s c o r e o b t a i n e d s o f a rF AF A G(a )F 1迭代寻优曲线I t e r a t i o n 100200300400500O p t i m u m f i t n e s s10010-10B e s t s c o r e o b t a i n e d s o f a rF AF A G(b )F 2迭代寻优曲线I t e r a t i o nO p t i m u m f i t n e s s10010-20B e s t s c o r e o b t a i n e d s o f a rF AF A G(c )F 3迭代寻优曲线6第2期沈汝涵,等:基于改进FA 算法与不完全Beta 函数的图像增强技术I t e r a t i o n100200300400500O p t i m u m f i t n e s s1010-10B e s t s c o r e o b t a i n e d s o f a rF AF A G(d )F 4迭代寻优曲线图1㊀4种基准函数对照图Fig.1㊀Comparison diagram of four benchmark functions3.2㊀图像增强实验为了验证FAG 与不完全Beta 函数自适应图像增强算法的可行性,选取一幅低对比度图像进行增强实验并展示效果:种群规模MP =30,迭代500次Max Cycle =500,个体的范围大小为(0~255)㊂除了应该首先通过视觉上的主观感受来判断改良的算法在光电图像增强上的效果,还应该通过客观精确的评价标准来进行衡量,所以引入均方差(MSE )以及信息熵和交叉熵作为对比优劣的依据[18]㊂MSE 公式如下:MSE =ðM i =1ðNj =1(f (i ,j )-g (i ,j ))2MN(12)信息熵公式:E 2=ðL -1i =0P e (i )lb P e (i )[](13)以及交叉熵的计算公式:CE (T ,V ,F )=CE (T ,F )+CE (V ,F )2(14)CE (T ,F )=ð255i =0h T (i )log 2h T (i )h F (i )(15)CE (V ,F )=ð255i =0h V (i )log 2h V (i )h F(i )MSE 表示大小M ∗N 的原图f 和增强后图像g 的均方误差,对比度信息转变为数字的大小㊂方差较小则表示图像在此区域内较为平缓,变化的幅度有限,信息表现较少,方差大则刚好相反㊂信息熵则是最直接反映图像细节内容量的评价标准,信息熵数值与呈现的信息量成正比㊂图片通过增强之后,理论上在明暗变化上会更加强烈,信息熵相比会增加,信息熵越高也可以认为是图片的质量越高,交叉熵计算得到的值越小代表和原来两幅图像一致,保留的细节也就越多㊂扭曲程度反映了图像的光谱失真程度㊂通过对每幅图像独立运行20次求出原始图像与另外增强方法得到的图像的亮度㊁对比度和信息熵,将其看作客观定量评价指标,得到以下结果,请见下表2㊂表2㊀增强图像数据对比Table 2㊀Data comparison of enhanced images算㊀法FAG -N -betaFA -N -beta 直方图增强MSE 6.1938e +035.9654e +093.3421e +10信息熵(bit )7.887.237.50交叉熵7.42 5.067.65扭曲程度78.9555.00132.85标准差74.4342.5969.85图2(a)为原始图片,图2(b)表示的是FAG 算法结合非完全BETA 函数,图2(c)表示原始FA 算法,最后一张为直方图增强的结果㊂多次独立运行得出数据排除错误数据进行平均处理可以克服因智能算法的随机性带来的影响㊂图2(b)㊁图2(c)可以看出FAG 结合非完全Beta 在亮度,对比度方面相较于原图以及其他两种算法的提升颇为明显,说明了本文的增强算法性能取得较大改善,在图像清晰度的方面能够保证与原图的基本持平㊂而对比原始FA -N -B 增强算法以及FAG -N -B 增强算法,可以发现在细节处的明暗表现更加保真而不至于过曝光,最显而易见的就是4张图左上方的住宅楼的外窗轮廓,原图几乎不可见,增强后的图片都将这一部分信息体现了出来,而直方图增强算法的增强效果虽然突出了感兴趣的部分,但是地面阴影区域信息丢失严重,图像的深色区域出现过曝光的问题,增加了噪声,影响了图片的整体清晰度,且直方图增强后的图片的光谱色彩失真较为严重,在非线性的增强策略中FAG -N -B 取得了较好的效果㊂(a )原图像16重庆工商大学学报(自然科学版)第40卷(b )FAG -N -B增强图像(c )FA -N -B增强图像(d )直方图增强图像图2㊀4种算法图像增强效果图Fig.2㊀Image enhancement effect of four algorithmsMSE 对比中FAG -N -beta 的数据最大,数字大小体现出来FAG -N -beta 增强的图片明暗对比最大,区域变化较大,增强效果更好;信息熵的对比中同样也是FAG -N -beta 策略增强效果更好㊂在交叉熵中FAG -N -beta 与直方图数据近似但是都要优于FA -N -beta 的增强效果;扭曲程度的对比直方图的失真程度较大,FA -N -beta 增强效果虽然较FAG -N -beta 更好,这是我们需要继续改进的地方但是结合前几项对比,综合考量还是FAG -N -beta 的整体图片增强效果更优㊂4㊀结㊀论从图1的对照实验可以发现在四个基准函数下改进FAG 收敛速度与趋势明显较传统FA 加快,且传统FA 算法在迭代50次附近,以及到100次附近跳出局部最优的效果并不好,而改进FAG 在100次不到的迭代的次数内已经彻底超过FA㊂通过观察改进算法FAG 与原FA 算法的寻优迭代实验结果,可以得出FAG 算法在收敛速度以及精度上都有很好的提升,算法跳出局部最优的能力和鲁棒性相较于原有FA 算法效果更好㊂从表2的对比原始图像的数据上看,FAG -N -B 与FA -N -B 对比MSE 以及信息熵均是改进算法FAG 结合非完全beta 取得更好的效果,图片的信息表现得更充分㊂所以从两方面的数据显示,以及最后图片呈现的效果来说改进FAG 算法比原FA 算法表现更好,FAG -N -B 算法在增强图片的性能方面较原始的算法来说有不小的提升㊂FA 作为一种新型群智能算法,相比粒子群和蚁群等具有良好的全局收敛性能和稳定性,但仍然存在一系列问题㊂利用萤火虫算法的快速搜索的特性,应用在图像处理领域,针对其发现率低㊁求解精度不高㊁求解速度慢等因素改进原算法,为了提高算法的性能,引入了随机因素来产生扰动破坏局部最优解,以及加入了疑似陷入最优解后的自检步骤,另外补充了改进版本的递减步长,在4种不同类型的基准函数上也取得了不错的结果,稳定收敛㊂非完全Beta 拟合灰度图像变换函数后,FAG 算法寻优动态α,β利用最佳的灰度曲线增强图像㊂多种改进机制的融合,算法的初期全局的探测的水平得以提升,并且在后期的局部搜索的能力也并没有降低,达到了两者很好地结合,体现了新策略的有效性㊂参考文献 References1 ㊀古良玲 王玉菡.基于模拟退火遗传算法的图像增强 J .激光杂志 2015 36 2 19 22.GU Liang-ling WANG Yu-han.Image enhancement based onsimulated annealing genetic algorithm J .Laser Magazine2015 36 2 19 22.2 ㊀王小芳 邹倩颖 彭林子 等.融合模糊聚类的蚁群图像增强算法 J .数据采集与处理 2020 35 3 506 515.WANG Xiao-fang ZOU Qian-ying PENG Lin-zi et al .Ant colony imageenhancementalgorithmbasedonfuzzyclustering J .Journal of Data Acquisition &Processing2020 35 3 506 515.3 ㊀FATEEN S E K BONILLA-PETRICIOLET A.Intelligent fireflyalgorithm for global optimization M .Cuckoo Search and FireflyAlgorithm.Springer vol 516 2014 .4 ㊀张慧宁 郭红山.基于改进萤火虫算法的模糊农业遥感图26第2期沈汝涵,等:基于改进FA算法与不完全Beta函数的图像增强技术像增强效应J .江苏农业科学2017 45 1 205 208. ZHANG Hui-ning GUO Hong-shan.Enhancement effect of fuzzy agricultural remote sensing image based on improved firefly algorithm J .Jiangsu Agricultural Sciences 2017 451 205 208.5 ㊀俞文静刘航李梓瑞等.基于改进萤火虫优化算法的视频监控图像增强J .计算机技术与发展2020 304 195 199.YU Wen-jing LIU Hang LI Zi-rui et al.Video surveillance image enhancement based on improved firefly optimization algorithm J .Computer Technology and Development 2020 30 4 195 199.6 ㊀郭红山张慧宁.基于萤火虫算法的农业遥感图像增强研究J .浙江农业学报2016 28 6 1076 1081. GUO Hong-shan ZHANG Hui-ning.Research on agricultural remote sensing image enhancement based on firefly algorithm J .Acta Agriculturae Zhejiangensis 2016 28 6 1076 1081.7 ㊀王沈娟高晓智.萤火虫算法研究综述J .微型机与应用2015 34 8 8 11.WANG shen-juan GAO Xiao-zhi.A survey of firefly algorithms J .Microcomputer&Its Applications 2015 348 8 11.8 ㊀ZHOU L DING L MA M et al.An accurate partially attracted firefly algorithm J .CrossRef 2018 1015 477 493.9 ㊀丁生荣马苗郭敏.人工鱼群算法在自适应图像增强中的应用J .计算机工程与应用2012 48 2 185 187. DING Sheng-rong MA Miao GUO Min.Application of artificial fish swarm algorithm in adaptive image enhancement J .Computer Engineering and Applications 2012 482 185 187.10 FARAHANI S M ABSHOURI A A NASIRI B et al.An improved firefly algorithm with directed movement C // Proceedings of20114th IEEE International Conference on Computer Science and Information Technology ICCSIT2011 VOL04.Institute of Electrical and Electronics Engineers 2011 4.11 符强童楠赵一鸣.一种基于多种群学习机制的萤火虫优化算法J .计算机应用研究2013 30123600-3602 3617.FU Qiang TONG Nan ZHAO Yi-ming.A firefly optimization algorithm based on multi population learning mechanism J .Computer Application Research 2013 30123600-3602 3617.12 李肇基程科王万耀等.一种改进的进化模型和混沌优化的萤火虫算法J .计算机与数字工程2019 4771605 1612.LI Zhao-ji CHENG Ke WANG Wan-yao et al.An improved evolutionary model and chaotic optimized firefly algorithm J .Computer and Digital Engineering 2019 4771605 1612.13 左仲亮郭星李炜.一种改进的萤火虫算法J .微电子学与计算机2018 35 2 61 66.ZUO Zhong-liang GUO Xing LI Wei.An improved firefly algorithm J Microelectronics and Computer 2018 35261 66.14 王航星潘巍.基于自适应吸引半径的萤火虫算法的粒子滤波J .计算机应用研究2019 36 12 3632 3636.WANG Hang-xing PAN Wei.Particle filter of firefly algorithm based on adaptive attraction radius J .Computer Application Research 2019 3612 3632 3636.15 王奎黄福珍.基于光照补偿的HSV空间多尺度Retinex图像增强J .激光与光电子学进展1 20.WANG Kui HUANG Fu-zhen.HSV spatial multi-scale Retinex image enhancement based on illumination compensation J .Laser&Optoelectronics Progress 1 20.16 PENG Qiong.A self-adaptive step glowworm swarm optimizationapproach J .International Journal of Computational Intelligence and Applications 2019 18 1 2022 59 10 102 113.17 宋娟全惠云.图像增强技术中的智能算法J .华东师范大学学报自然科学版2007 3 93 99.SONG Juan QUAN Hui-yun.Intelligent algorithm in image enhancement technology J .Journal of East China Normal University Natural Science 2007 3 93 99.18 刘畅刘利强张丽娜等.改进萤火虫算法及其在全局优化问题中的应用J .哈尔滨工程大学学报2017 38 4569 577.LIU Chang LIU Li-qiang ZHANG Li-na et al.Improved firefly algorithm and its application in global optimization problems J .Journal of Harbin Engineering University 2017 38 4 569 577.责任编辑:陈㊀芳36。
新闻英语测试题及答案

新闻英语测试题及答案一、选择题(每题1分,共10分)1. What is the main topic of the news article?A. The economic situationB. A new technological breakthroughC. A political eventD. A cultural festival2. According to the article, which of the following statements is NOT true?A. The event attracted a large audience.B. The speaker emphasized the importance of education.C. The event was held in a remote area.D. The speaker called for environmental protection.3. What is the purpose of the new policy mentioned in the article?A. To reduce traffic congestion.B. To increase tax revenue.C. To promote tourism.D. To improve public health.4. The article suggests that the company has:A. Expanded its market share.B. Faced financial difficulties.C. Launched a new product line.D. Merged with a competitor.5. What does the term "sustainable development" refer to in the context of the article?A. Economic growth without environmental harm.B. The development of new technologies.C. The increase in population.D. The improvement of living standards.6. The article reports that the government has:A. Introduced new regulations.B. Allocated additional funding.C. Imposed a new tax.D. Announced a public inquiry.7. The author of the article seems to have a(n) ________ attitude towards the issue discussed.A. PositiveB. NegativeC. NeutralD. Critical8. Which of the following is NOT mentioned as a benefit of the new technology?A. Increased efficiency.B. Reduced costs.C. Improved safety.D. Decreased demand.9. According to the article, the majority of the public:A. Supports the proposed changes.B. Opposes the proposed changes.C. Is indifferent to the proposed changes.D. Has mixed opinions about the proposed changes.10. The article concludes by highlighting the need for:A. Greater international cooperation.B. Stricter domestic regulations.C. More public awareness campaigns.D. Additional scientific research.二、填空题(每空1分,共10分)11. The ________ of the new law has been met with mixed reactions from the public.[答案] introduction12. The company has seen a ________ in profits over the past year.[答案] decline13. The ________ of the old bridge has been postponed due to budget constraints.[答案] construction14. The ________ of the new policy is expected to have a significant impact on the industry.[答案] implementation15. The ________ of the event was attended by numerous dignitaries and celebrities.[答案] inauguration16. The ________ of the project is scheduled for next month. [答案] completion17. The ________ of the company has been attributed to its innovative approach.[答案] success18. The ________ of the new initiative has been widely praised by the media.[答案] launch19. The ________ of the old factory site has been approved by the city council.[答案] redevelopment20. The ________ of the new technology is expected to revolutionize the industry.[答案] introduction三、简答题(每题5分,共20分)21. What are the key points of the new policy discussed in the article?[答案] The key points of the new policy include a focus on environmental sustainability, incentives for businesses to adopt green practices, and penalties for non-compliance.22. How does the article describe the impact of the recent economic downturn on the job market?[答案] The article describes the impact as significant, with many industries facing layoffs and a rise in unemployment rates, particularly among young professionals.23. What are the main features of the new technologyintroduced in the article?[答案] The main features of the new technology include advanced data processing capabilities, user-friendly interface, and compatibility with various platforms.24. How does the article summarize the public's reaction to the proposed changes?[答案] The article summarizes the public's reaction as generally positive, with many expressing support for the changes and their potential benefits to society.四、阅读理解题(每题5分,共20分)25. Read the following excerpt from the article and answer the question:"The new initiative aims to bridge the gap between traditional and modern education methods, providing students with a more holistic learning experience."What does the initiative aim to achieve?[答案] The initiative aims to achieve a more holistic learning experience by integrating traditional and modern education methods.26. According to the article。
高考英语《语法填空+阅读理解+应用文写作》真题含答案
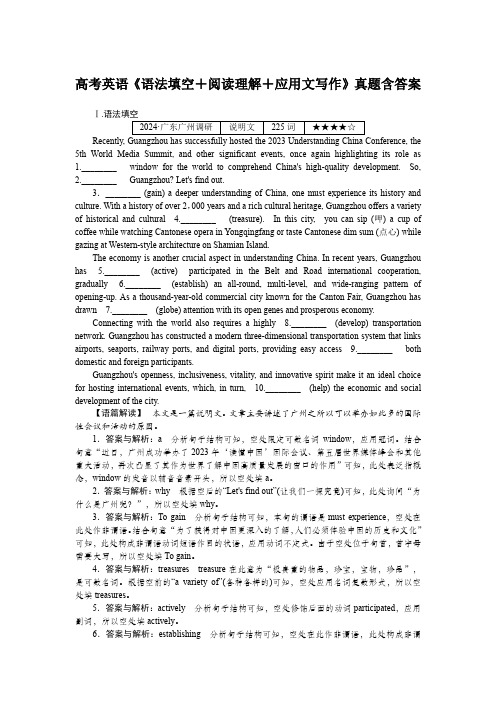
高考英语《语法填空+阅读理解+应用文写作》真题含答案Ⅰ.语法填空5th World Media Summit, and other significant events, once again highlighting its role as1.________ window for the world to comprehend China's highquality development. So,2.________ Guangzhou? Let's find out.3.________ (gain) a deeper understanding of China, one must experience its history and culture. With a history of over 2,000 years and a rich cultural heritage, Guangzhou offers a variety of historical and cultural 4.________ (treasure). In this city, you can sip (呷) a cup of coffee while watching Cantonese opera in Yongqingfang or taste Cantonese dim sum (点心) while gazing at Westernstyle architecture on Shamian Island.The economy is another crucial aspect in understanding China. In recent years, Guangzhou has 5.________ (active) participated in the Belt and Road international cooperation, gradually 6.________ (establish) an allround, multilevel, and wideranging pattern of openingup. As a thousandyearold commercial city known for the Canton Fair, Guangzhou has drawn 7.________ (globe) attention with its open genes and prosperous economy.Connecting with the world also requires a highly 8.________ (develop) transportation network. Guangzhou has constructed a modern threedimensional transportation system that links airports, seaports, railway ports, and digital ports, providing easy access 9.________ both domestic and foreign participants.Guangzhou's openness, inclusiveness, vitality, and innovative spirit make it an ideal choice for hosting international events, which, in turn, 10.________ (help) the economic and social development of the city.【语篇解读】本文是一篇说明文。
中英命名实体识别及对齐中的中文分词优化论文笔记

中英命名实体识别及对齐中的中⽂分词优化论⽂笔记论⽂名和编号摘要/引⾔相关背景和⼯作论⽂⽅法/模型实验(数据集)及分析(⼀些具体数据)未来⼯作/不⾜是否有源码问题原因解决思路优势中英命名实体识别及对齐中的中⽂分词优化编号:0372-2112(2015)08-1481-071.命名实体识别是⾃然语⾔处理的⼀项重要⼯作,对于跨语⾔的⾃然语⾔处理应⽤,除了命名实体识别,命名实体的翻译对于提升机器翻译质量、跨语⾔信息检索效果等也有着重要作⽤。
由于命名实体更新较快,⼤多数命名实体都属于词典的未登录词,这为命名实体识别和翻译带来了困难。
1. 1.基于双语平⾏语料进⾏命名实体的识别和翻译。
2.对于双语命名实体词汇进⾏置信度评估,根据置信度⾼的实体对齐,对命名实体相关的分词错误进⾏修正从⽽提⾼命名实体识别的效果,调整命名实体词汇的分词粒度,优化双语命名实体对齐,提⾼双语命名实体翻译抽取的效果,进⽽提⾼统计机器翻译质量。
1.通过利⽤实体词汇的对其信息修正命名实体识别结果,再根据实体对齐结果调整分词粒度、修正错误分词。
分词优化后使得双语命名实体尽可能多地⼀⼀对应,进⽽提⾼了中英命名实体翻译抽取和统计机器翻译地效果。
1.通过双语词对齐信息可以修正命名实体识别的错误。
2.双语命名实体对齐的⽅法主要由两种:在双语实体识别的基础上利⽤对齐模型寻找两者的对齐关系;仅在⼀种语⾔中识别命名实体,再利⽤融合多特征的对齐模型,在另⼀个语⾔中寻找它们对应的翻译。
第⼆种⽅法可以减少双语命名实体识别错误的影响,但是会丢失另⼀种语⾔中有⽤的命名实体信息。
3.Chen等提出了⼀种双语命名实体识别和对齐进⾏交互的模型,利⽤双语对齐信息对识别结果和对齐结果进⾏修正,不仅提⾼了实体对齐的效果,也有效提⾼了实体识别的正确率和召回率。
然⽽,这个模型并没有修正中⽂分词对命名实体识别和对齐的影响。
1.计算命名实体词汇对齐的置信度:对⼀个中英双语句对,中⽂在初始分词的基础上进⾏命名实体识别。
北京大学现代汉语语料库基本加工规范

北京大学现代汉语语料库基本加工规范俞士汶段慧明朱学锋孙斌(北京大学计算机系,北京大学计算语言学研究所 北京 100871)摘要:北京大学计算语言学研究所已经完成了一个有2700万汉字的现代汉语语料库的基本加工。
加工项目除词语切分和词性标注外,还包括专有名词(人名、地名、团体机构名称等)标注、语素子类标注以及动词、形容词的特殊用法标注。
这项大规模语言工程的顺利完成得益于事先制订并不断完善的规范。
发表《北京大学现代汉语语料库基本加工规范》是为了抛砖引玉,更广泛地向专家、同行征询意见,以便进一步修订。
关键词:现代汉语;语料库;词语切分;词性标注;规范中图分类号:TP391The Basic Processing of Contemporary Chinese Corpus at Peking UniversitySPECIFICATIONYU Shi-wen DUAN Hui-ming ZHU Xue-feng Bing SWEN(Institute of Computational Linguistics, Peking University, Beijing, 100871) Abstract: The Institute of Computational Linguistics, Peking University has completed the basic processing of a contemporary Chinese corpus that has 27 million Chinese Characters. In addition to word segmentation and part-of-speech tagging, the processing involves the tagging of proper nouns (person names, place names, organization names and so on), morpheme subcategories and the special usages of verbs and adjectives. The success of this large-scale language engineering is attributed to the SPECIFICATION, which had been made beforehand and was being perfected while in use. We are hereby making an introduction to the SPECIFICA TION through this publication, thus inviting the comments from all the experts and our colleagues for the improvement of it.Keywords: contemporary Chinese; corpus; word segmentation; part-of-speech tagging; specification⒈ 前言关于汉语语料库的全面情况,冯志伟教授的文章已有详细介绍[1]。
基于自适应对偶字典的磁共振图像的超分辨率重建
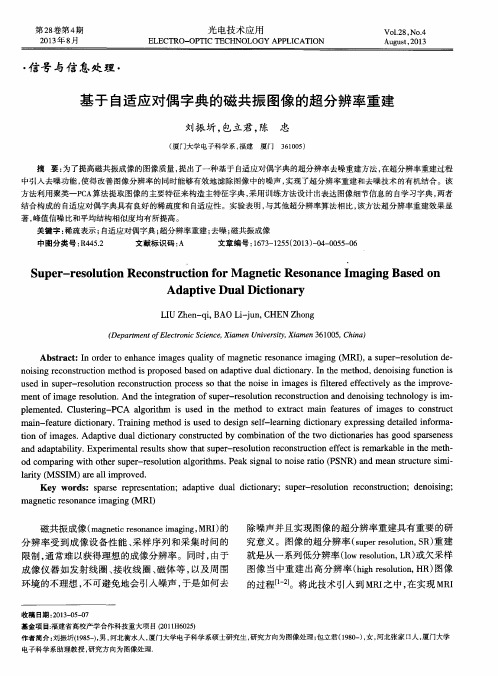
L I U Z h e n - q i , B A 0 L i - j u n , C HE N Z h o n g
r De p a r t m e n t o f E l e c t r o n i c S c i e n c e , X i a me n U n i v e r s i t y , Xi a me n 3 6 1 0 0 5 , C h i n a )
刘振 圻 , 包立君 , 陈 忠
( 厦 门大学电子科 学系, 福建 厦门 3 6 1 0 0 5 )
摘 要: 为了提高磁共振成像的图像 质量 , 提 出了一种基于 自适应对偶字典的超分辨率 去噪重建方法 , 在超分辨率重建过程 中引入去噪功能 , 使 得改善图像 分辨率的同时能够有效地滤除 图像 中的噪声 , 实现 了超分辨率重建和去噪技术 的有机结合 。该 方法利用聚类一P c A算 法提取图像的主要特征来构造主特征字典 , 采用 训练方法设计 出表达图像 细节信 息的 自学 习字 典 , 两者 结合构成的 自适应对偶字典具有 良好 的稀疏度和 自适应性 。实验表 明, 与其他超分辨率算法相 比, 该方法超分辨率重建效果显 著, 峰值信噪 比和平均结构相似度均有所提高。
第2 8 卷第 4 期
2 0 1 3 年8 月
பைடு நூலகம்光 电技术 应 用
EL ECT RO一 0P T I C T ECHNOLOGY AP P LI CAT1 0N
V O1 . 28. NO. 4
Au g u s t , 2 01 3
・
信号 与信息处理 ・
基 于 自适应对偶 字典的磁共振 图像 的超 分辨率重建