灰度图像质心快速算法
CCD目标灰度质心算法的动态误差分析

Dy a i r r n m cEr o ay i f c l n r i g rt m s d o An l sso u aCe t o dAl o i Fa h Ba e n CCD
—
ai m c
f) 4
Px1 ie
其中:X 为像元尺寸。 p。 i1 根据质心算法 ,无噪声的 目标灰度质心为
X u:—
Sx— x  ̄ (d: + 声后的 目 标灰度质心为
∑ 。 ( () g。() ∑ ・u ) ∑ ( gi ) [ g + ) ( g( ) ・ i ] 。 + 。 ne ) - o( s 。 ∑ g() go() ( + ne ) i s ∑ ) ∑ g。() g( + 。 i
度值 。 因此 ,噪 声 引起 的灰 度质 心位 置变 化可 以表 示为
,
其 中 : i 第 i 像元 的位 置 , u 0 X为 个 g( 为第 i 像元 的无噪 声分 量灰 度值 , n X) 第 i X 个 g。e i i( 为 个像元 的噪 声分量 灰
第3 8卷第 6期
n
:
X =r o o o t () 2 () 3
动 态 目标 尺 寸可 以近 似表 示为
X” i +XⅢ d 。 = 。i 。
由于积分均化效应的影响 ,t 间内 C D 得到的灰度曲线如图 1 o 时 C 所示。对比( () 图可以看出,随 a b两 ) 着 目标移动位移 X 的增大 ,目 o 标灰度分布曲线逐渐变平变宽。
灰度重心法公式
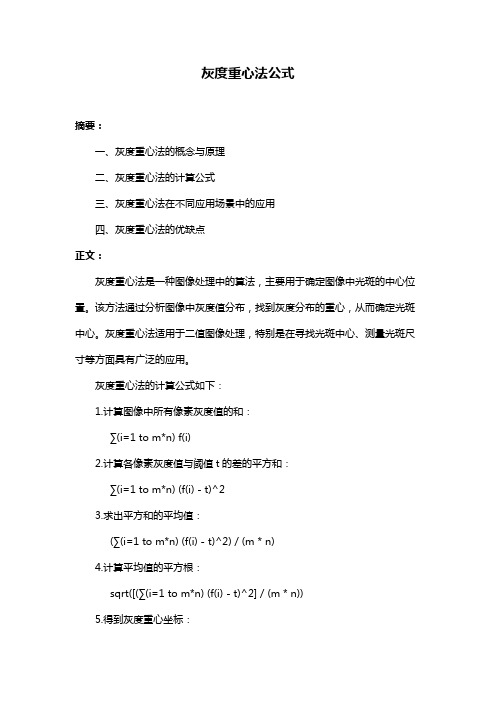
灰度重心法公式
摘要:
一、灰度重心法的概念与原理
二、灰度重心法的计算公式
三、灰度重心法在不同应用场景中的应用
四、灰度重心法的优缺点
正文:
灰度重心法是一种图像处理中的算法,主要用于确定图像中光斑的中心位置。
该方法通过分析图像中灰度值分布,找到灰度分布的重心,从而确定光斑中心。
灰度重心法适用于二值图像处理,特别是在寻找光斑中心、测量光斑尺寸等方面具有广泛的应用。
灰度重心法的计算公式如下:
1.计算图像中所有像素灰度值的和:
∑(i=1 to m*n) f(i)
2.计算各像素灰度值与阈值t的差的平方和:
∑(i=1 to m*n) (f(i) - t)^2
3.求出平方和的平均值:
(∑(i=1 to m*n) (f(i) - t)^2) / (m * n)
4.计算平均值的平方根:
sqrt([(∑(i=1 to m*n) (f(i) - t)^2] / (m * n))
5.得到灰度重心坐标:
(x_重心, y_重心) = (∑(i=1 to m*n) f(i)*i, ∑(i=1 to m*n) f(i)*j) / ∑(i=1 to m*n) f(i)
其中,m和n分别为图像的行和列,f(i)为图像中第i个像素的灰度值,i 和j分别为像素的行和列坐标。
灰度重心法在不同应用场景中有广泛应用,如在激光加工、光刻技术、光学测量等领域。
它具有简单、快速、准确的优点,但同时也存在一定的局限性,如对图像噪声敏感、对阈值选择敏感等。
总之,灰度重心法作为一种图像处理方法,在确定光斑中心方面具有实用性和可读性。
一种用于白天星敏感器的星点质心提取方法
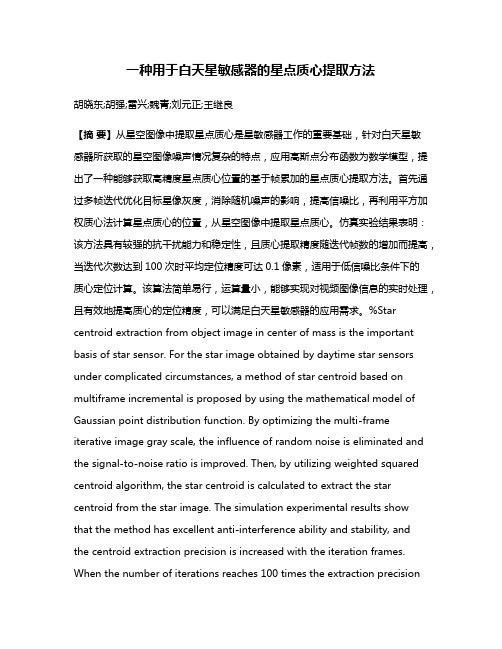
一种用于白天星敏感器的星点质心提取方法胡晓东;胡强;雷兴;魏青;刘元正;王继良【摘要】从星空图像中提取星点质心是星敏感器工作的重要基础,针对白天星敏感器所获取的星空图像噪声情况复杂的特点,应用高斯点分布函数为数学模型,提出了一种能够获取高精度星点质心位置的基于帧累加的星点质心提取方法。
首先通过多帧迭代优化目标星像灰度,消除随机噪声的影响,提高信噪比,再利用平方加权质心法计算星点质心的位置,从星空图像中提取星点质心。
仿真实验结果表明:该方法具有较强的抗干扰能力和稳定性,且质心提取精度随迭代帧数的增加而提高,当迭代次数达到100次时平均定位精度可达0.1像素,适用于低信噪比条件下的质心定位计算。
该算法简单易行,运算量小,能够实现对视频图像信息的实时处理,且有效地提高质心的定位精度,可以满足白天星敏感器的应用需求。
%Star centroid extraction from object image in center of mass is the important basis of star sensor. For the star image obtained by daytime star sensors under complicated circumstances, a method of star centroid based on multiframe incremental is proposed by using the mathematical model of Gaussian point distribution function. By optimizing the multi-frame iterative image gray scale, the influence of random noise is eliminated and the signal-to-noise ratio is improved. Then, by utilizing weighted squared centroid algorithm, the star centroid is calculated to extract the star centroid from the star image. The simulation experimental results showthat the method has excellent anti-interference ability and stability, andthe centroid extraction precision is increased with the iteration frames. When the number of iterations reaches 100 times the extraction precisionis increased with the increasing of iteration number and could approach 0.1 pixel, which is suitable for low signal-to-noise ratio. This algorithm is simple, less computations, and can achieve real-time processing of video image information. It can effectively improve the positioning precision, and satisfy the application requirements of daytime star sensors.【期刊名称】《中国惯性技术学报》【年(卷),期】2014(000)004【总页数】5页(P481-485)【关键词】图像处理;质心提取;亚像素定位;帧累加;信噪比【作者】胡晓东;胡强;雷兴;魏青;刘元正;王继良【作者单位】中国航空工业集团西安飞行自动控制研究所,西安 710065;中国航空工业集团西安飞行自动控制研究所,西安 710065;中国航空工业集团西安飞行自动控制研究所,西安 710065;中国航空工业集团西安飞行自动控制研究所,西安 710065;中国航空工业集团西安飞行自动控制研究所,西安 710065;中国航空工业集团西安飞行自动控制研究所,西安 710065【正文语种】中文【中图分类】O436现今主要的三种导航模式分别是GPS导航,天文导航和惯性导航,其中GPS的导航精度最高,但易受干扰、且保密性差,因此具有高隐蔽性、高可靠性要求的导航任务不能依赖GPS导航模式。
超大视场太阳敏感器图像质心提取算法
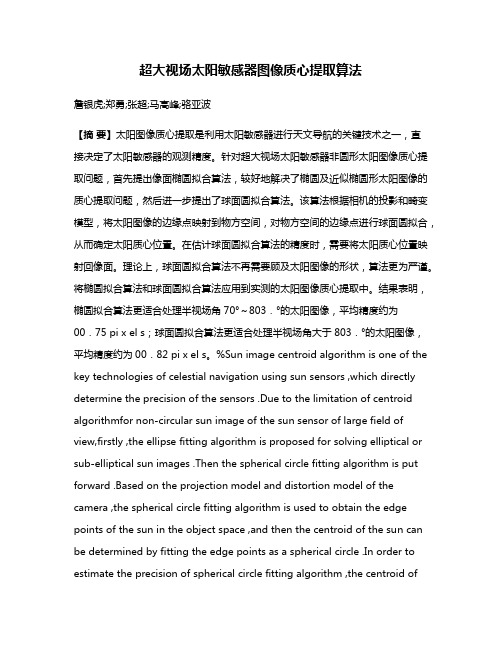
超大视场太阳敏感器图像质心提取算法詹银虎;郑勇;张超;马高峰;骆亚波【摘要】太阳图像质心提取是利用太阳敏感器进行天文导航的关键技术之一,直接决定了太阳敏感器的观测精度。
针对超大视场太阳敏感器非圆形太阳图像质心提取问题,首先提出像面椭圆拟合算法,较好地解决了椭圆及近似椭圆形太阳图像的质心提取问题,然后进一步提出了球面圆拟合算法。
该算法根据相机的投影和畸变模型,将太阳图像的边缘点映射到物方空间,对物方空间的边缘点进行球面圆拟合,从而确定太阳质心位置。
在估计球面圆拟合算法的精度时,需要将太阳质心位置映射回像面。
理论上,球面圆拟合算法不再需要顾及太阳图像的形状,算法更为严谨。
将椭圆拟合算法和球面圆拟合算法应用到实测的太阳图像质心提取中。
结果表明,椭圆拟合算法更适合处理半视场角70°~803.°的太阳图像,平均精度约为00.75 pi x el s;球面圆拟合算法更适合处理半视场角大于803.°的太阳图像,平均精度约为00.82 pi x el s。
%Sun image centroid algorithm is one of the key technologies of celestial navigation using sun sensors ,which directly determine the precision of the sensors .Due to the limitation of centroid algorithmfor non‐circular sun image of the sun sensor of large field of view,firstly ,the ellipse fitting algorithm is proposed for solving elliptical or sub‐elliptical sun images .Then t he spherical circle fitting algorithm is put forward .Based on the projection model and distortion model of the camera ,the spherical circle fitting algorithm is used to obtain the edge points of the sun in the object space ,and then the centroid of the sun can be determined by fitting the edge points as a spherical circle .In order to estimate the precision of spherical circle fitting algorithm ,the centroid ofthe sun should be projected back to the image space . Theoretically ,the spherical circle fitting algorithm is no longer need to take into account the shape of the sun image ,the algorithm is more precise .The results of practical sun images demonstrate that the ellipse fitting algorithm is more suitable for the sun image with 70°~80 .3° half angle o f view , and the mean precision is about 0 0.75 pixels;the spherical circle fitting algorithmis more suitable for the sun image with a half angle of view larger than 80 3.° ,and the mean precision is about 0 0.82 pixels .【期刊名称】《测绘学报》【年(卷),期】2015(000)010【总页数】7页(P1078-1084)【关键词】天文导航;太阳图像;质心提取算法;边缘检测;最小二乘拟合【作者】詹银虎;郑勇;张超;马高峰;骆亚波【作者单位】信息工程大学导航与空天目标工程学院,河南郑州450001;信息工程大学导航与空天目标工程学院,河南郑州450001;信息工程大学导航与空天目标工程学院,河南郑州450001;信息工程大学地理空间信息学院,河南郑州450001;郑州大学水利与环境学院,河南郑州450001【正文语种】中文【中图分类】P2281 引言太阳敏感器是一种通过观测太阳方向矢量确定载体姿态的传感器,在航空航天领域应用极其广泛。
单目视觉测量系统质心定位算法
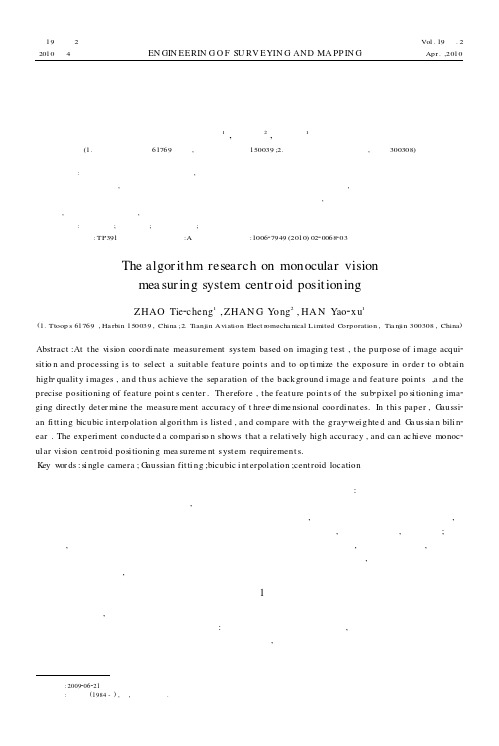
第19卷第2期 测 绘 工 程 Vol.19№.22010年4月 EN GIN EERIN G O F SU RV EYIN G AND MA PP IN G Apr.,2010单目视觉测量系统质心定位算法赵铁成1,张 勇2,韩曜旭1(1.中国人民解放军61769部队,黑龙江哈尔滨150039;2.天津航空机电有限公司,天津300308)摘 要:在测头成像视觉坐标测量系统中,对图像进行采集和处理是为选取合适的特征点并对其进行优化曝光以便获取高质量的图像,进而实现特征点与背景图像分离和特征点中心的精确定位。
因此,对于特征点成像的亚像素定位直接决定着三维空间坐标的测量精度。
文中提出高斯拟合双三次插值算法,并与灰度加权和高斯双线性进行对比,实验表明精度比较高,能够达到单目视觉测量系统质心定位要求。
关键词:单摄像机;高斯拟合;双三次插值;质心定位中图分类号:TP391 文献标志码:A 文章编号:100627949(2010)022*******The algor ithm research on monocular visionmea sur ing system centr oid positioningZHAO Tie 2cheng 1,ZHAN G Yong 2,HA N Yao 2xu1(1.Ttoop s 61769,Ha rbin 150039,China ;2.T ianjin Aviation Elect romecha nical Limited Corporation ,Tia njin 300308,China )Abstract :At t he vi sion coordi nate measurement system based on imaging t est ,t he purpose of i mage acqui 2sit io n and processi ng i s to sel ect a suit abl e feat ure point s and to op ti mize t he exposure in orde r t o obt ai n high 2qualit y i mages ,and t hus achieve t he separation of t he background i mage a nd feat ure poi nt s ,a nd t he precise positioni ng of feat ure poi nt s center.Therefore ,t he feat ure point s of t he sub 2pixel po si tioning ima 2gi ng direct ly det er mi ne t he measure ment accuracy of t hree 2di me nsional coordi nat es.In t hi s paper ,G aussi 2an fi tt ing bicubic i nt erpolat ion al gori t hm i s list ed ,and compare wit h t he gray 2wei ghte d and G a ussia n bili n 2ear .The experi ment conducte d a compari so n shows t hat a relati vely high accuracy ,and ca n ac hi eve monoc 2ul ar vi sion cent roi d posi tioning mea sureme nt syst em requirement s.K ey w or ds :si ngl e camera ;G aussian fit ti ng ;bicubic i nt erpol ation ;cent roid location 收稿日期262作者简介赵铁成(),男,助理工程师 应用在航天、航空、军事和工业领域的大型复杂构件需要越来越高的加工和装配精度,对高精度大尺寸三维整体现场测试技术与设备的需求日趋迫切。
基于距离平方模板的快速质心搜索算法

S in ea d Te h olg fChi ,Ch n d 1 05 ce c n c n o yo na e g u 6 0 4,Chn ) ia
Ab ta t n t e f l f n e l e tr c g i o s r c :I h i d o t l g n e o n t n,d ma d n h y t m a e r h a d ta k t e e i i i e n i g t e s s e c n s a c n r c h t r e e to d q ik y a d a c r t l , a f s e t o d s a c l o i m a e n Eu l e n a g t c n r i u c l n c u a e y a t c n r i e r h a g rt h b s d o ci a d
基 于 距 离 平 方模 板 的快 速 质 心 搜 索 算 法
*
徐 乃 昊 , 国成 , 尹璨 王 毛
( 电子科技 大学 微 电子与 固体 电子学院 , 四川 成都 605) 1 0 4
摘 要 : 能识 别领 域 , 格要 求 系统 能快速 准确 地搜 索跟 踪 目标 质 心 , 个基 于欧 式模板 的快速 智 严 一
第 3 3卷 第 6 期 21 年 1 01 2月
光
学
仪
器
Vo 3,No 6 L3 .
De e e .2 1 c mb r 0 1
图像区域求质心,面积
图像区域求质⼼,⾯积⼆值图像中⼤于0的像素个数就是图像的⾯积坐标x乘以每个像素点的值求和,除以图像中像素值的和得到质⼼注意图像的宽⾼范围从1-width,1-height。
/jia20003/article/details/17596645图像处理之计算⼆值连通区域的质⼼⼀:⼏何距(Geometric Moments)知识与质⼼寻找原理1. Image Moments是图像处理中⾮常有⽤的算法,可以⽤来计算区域图像的质⼼,⽅向等⼏何特性,同时Mpq的⾼阶具有旋转不变性,可以⽤来实现图像⽐较分类,正是因为Moments有这些特性,很多⼿绘油画效果也会基于该算法来模拟实现。
它的数学表达为:它的低阶M00,M01, M10可以⽤来计算质⼼,中⼼化以后M11,M02,M20可以⽤来计算区域的⽅向/⾓度2. 什么是质⼼就是通过该点,区域达到⼀种质量上的平衡状态,可能物理学上讲的⽐较多,简单点的说就是规则⼏何物体的中⼼,不规则的可以通过挂绳⼦的⽅法来寻找。
⼆:算法流程1. 输⼊图像转换为⼆值图像2. 通过连通组件标记算法找到所有的连通区域,并分别标记3. 对每个连通区域运⽤计算⼏何距算法得到质⼼4. ⽤不同颜⾊绘制连通区域与质⼼,输出处理后图像三:算法效果左边为原图,右边蓝⾊为连通组件标记算法处理以后结果,⽩⾊点为质⼼四:关键代码解析1. 计算⼏何距算法代码double m00 = moments(pixels, width, height, 0, 0);double xCr = moments(pixels, width, height, 1, 0) / m00;// rowdouble yCr = moments(pixels, width, height, 0, 1) / m00;// columnreturn new double[]{xCr, yCr};2. 连通组件标记算法代码参见这⾥:五:程序源代码[java]1. package com.gloomyfish.image.moments;2.3. import java.awt.image.BufferedImage;4.5. import com.gloomyfish.filter.study.AbstractBufferedImageOp;6. import com.gloomyfish.rice.analysis.FastConnectedComponentLabelAlg;7. // Geometric Moments Computing8. // low-order moments - calculate the center point9. // second-order moments - get angle size10. // projection -11. public class GeometricMomentsFilter extends AbstractBufferedImageOp {12.13. @Override14. public BufferedImage filter(BufferedImage src, BufferedImage dest) {15. int width = src.getWidth();16. int height = src.getHeight();17.18. if ( dest == null )19. dest = createCompatibleDestImage( src, null );20.21. // first step - make it as binary image output pixel22. int[] inPixels = new int[width*height];23. int[] outPixels = new int[width*height];24. getRGB( src, 0, 0, width, height, inPixels );25. int index = 0;26. for(int row=0; row<height; row++) {27. int tr = 0;28. for(int col=0; col<width; col++) {29. index = row * width + col;30. tr = (inPixels[index] >> 16) & 0xff;31. if(tr > 127)32. {33. outPixels[index] = 1;34. }35. else36. {37. outPixels[index] = 0;38. }39. }40. }41.42. // second step, connected component labeling algorithm43. FastConnectedComponentLabelAlg ccLabelAlg = new FastConnectedComponentLabelAlg();44. ccLabelAlg.setBgColor(0);45. int[] labels = ccLabelAlg.doLabel(outPixels, width, height);46. int max = 0;47. for(int i=0; i<labels.length; i++)48. {49. if(max < labels[i])50. {51. System.out.println("Label Index = " + labels[i]);52. max = labels[i];53. }54. }55.56. // third step, calculate center point of each region area(connected component)57. int[] input = new int[labels.length];58. GeometricMomentsAlg momentsAlg = new GeometricMomentsAlg();59. momentsAlg.setBACKGROUND(0);60. double[][] labelCenterPos = new double[max][2];61. for(int i=1; i<=max; i++)62. {63. for(int p=0; p<input.length; p++)64. {65. if(labels[p] == i)66. {67. input[p] = labels[p];68. }69. else70. {71. input[p] = 0;72. }73. }74. labelCenterPos[i-1] = momentsAlg.getGeometricCenterCoordinate(input, width, height);75. }76.77. // render the each connected component center position78. for(int row=0; row<height; row++) {79. for(int col=0; col<width; col++) {80. index = row * width + col;81. if(labels[index] == 0)82. {83. outPixels[index] = (255 << 24) | (0 << 16) | (0 << 8) | 0; // make it as black for background84. }85. else86. {87. outPixels[index] = (255 << 24) | (0 << 16) | (0 << 8) | 100; // make it as blue for each region area88. }89. }90. }91.92. // make it as white color for each center position93. for(int i=0; i<max; i++)94. {95. int crow = (int)labelCenterPos[i][0];96. int ccol = (int)labelCenterPos[i][1];97. index = crow * width + ccol;98. outPixels[index] = (255 << 24) | (255 << 16) | (255 << 8) | 255;99. }100.101. setRGB( dest, 0, 0, width, height, outPixels );102. return dest;103. }104.105. }package com.gloomyfish.image.moments;import java.awt.image.BufferedImage;import com.gloomyfish.filter.study.AbstractBufferedImageOp;import com.gloomyfish.rice.analysis.FastConnectedComponentLabelAlg;// Geometric Moments Computing// low-order moments - calculate the center point// second-order moments - get angle size// projection -public class GeometricMomentsFilter extends AbstractBufferedImageOp {@Overridepublic BufferedImage filter(BufferedImage src, BufferedImage dest) {int width = src.getWidth();int height = src.getHeight();if ( dest == null )dest = createCompatibleDestImage( src, null );// first step - make it as binary image output pixelint[] inPixels = new int[width*height];int[] outPixels = new int[width*height];getRGB( src, 0, 0, width, height, inPixels );int index = 0;for(int row=0; row<height; row++) {int tr = 0;for(int col=0; col<width; col++) {index = row * width + col;tr = (inPixels[index] >> 16) & 0xff;if(tr > 127){outPixels[index] = 1;}else{outPixels[index] = 0;}}}// second step, connected component labeling algorithmFastConnectedComponentLabelAlg ccLabelAlg = new FastConnectedComponentLabelAlg();ccLabelAlg.setBgColor(0);int[] labels = ccLabelAlg.doLabel(outPixels, width, height);int max = 0;for(int i=0; i<labels.length; i++){if(max < labels[i]){System.out.println("Label Index = " + labels[i]);max = labels[i];}}// third step, calculate center point of each region area(connected component)int[] input = new int[labels.length];GeometricMomentsAlg momentsAlg = new GeometricMomentsAlg();momentsAlg.setBACKGROUND(0);double[][] labelCenterPos = new double[max][2];for(int i=1; i<=max; i++){for(int p=0; p<input.length; p++){if(labels[p] == i){input[p] = labels[p];}else{input[p] = 0;}}labelCenterPos[i-1] = momentsAlg.getGeometricCenterCoordinate(input, width, height);}// render the each connected component center positionfor(int row=0; row<height; row++) {for(int col=0; col<width; col++) {index = row * width + col;if(labels[index] == 0){outPixels[index] = (255 << 24) | (0 << 16) | (0 << 8) | 0; // make it as black for background}else{outPixels[index] = (255 << 24) | (0 << 16) | (0 << 8) | 100; // make it as blue for each region area }}}// make it as white color for each center positionfor(int i=0; i<max; i++){int crow = (int)labelCenterPos[i][0];int ccol = (int)labelCenterPos[i][1];index = crow * width + ccol;outPixels[index] = (255 << 24) | (255 << 16) | (255 << 8) | 255;}setRGB( dest, 0, 0, width, height, outPixels );return dest;}}Moment算法代码:[java]1. package com.gloomyfish.image.moments;2.3. public class GeometricMomentsAlg {4. private int BACKGROUND = 0; // background color5. private int labelIndex = 1;6.7. public GeometricMomentsAlg()9. System.out.println("Geometric Moments Algorithm Initialziation...");10. }11.12. public int getLabelIndex() {13. return labelIndex;14. }15.16. public void setLabelIndex(int labelIndex) {17. belIndex = labelIndex;18. }19.20. public int getBACKGROUND() {21. return BACKGROUND;22. }23.24. public void setBACKGROUND(int bACKGROUND) {25. BACKGROUND = bACKGROUND;26. }27.28. public double[] getGeometricCenterCoordinate(int[] pixels, int width, int height)29. {30. double m00 = moments(pixels, width, height, 0, 0);31. double xCr = moments(pixels, width, height, 1, 0) / m00; // row32. double yCr = moments(pixels, width, height, 0, 1) / m00; // column33. return new double[]{xCr, yCr};34. }35.36. public double moments(int[] pixels, int width, int height, int p, int q)37. {38. double mpq = 0.0;39. int index = 0;40. for(int row=0; row<height; row++)41. {42. for(int col=0; col<width; col++)43. {44. index = row * width + col;45. if(pixels[index] == BACKGROUND) continue;46. mpq += Math.pow(row, p) * Math.pow(col, q);47. }48. }49. return mpq;50. }51.52. public double centralMoments(int[] pixel, int width, int height, int p, int q)53. {54. double m00 = moments(pixel, width, height, 0, 0);55. double xCr = moments(pixel, width, height, 1, 0) / m00;56. double yCr = moments(pixel, width, height, 0, 1) / m00;57. double cMpq = 0.0;58. int index = 0;59. for(int row=0; row<height; row++)60. {61. for(int col=0; col<width; col++)62. {63. index = row * width + col;64. if(pixel[index] == BACKGROUND) continue;65. cMpq += Math.pow(row - xCr, p) * Math.pow(col - yCr, q);66. }67. }68. return cMpq;69. }70.71. public double normalCentralMoments(int[] pixel, int width, int height, int p, int q)72. {73. double m00 = moments(pixel, width, height, 0, 0);74. double normal = Math.pow(m00, ((double)(p+q+2))/2.0d);75. return centralMoments(pixel, width, height, p, q)/normal;77. }。
灰度质心法公式
灰度质心法公式灰度质心法(Grayscale Centroid Method)是一种常用于图像处理中的颜色特征提取方法。
它可以通过计算图像中灰度值的加权平均来得到图像的质心位置,从而描述图像的整体亮度分布情况。
相比于直接计算图像亮度的平均值,灰度质心法考虑了不同灰度级别的权重,能更准确地反映图像的亮度变化。
灰度质心法的计算公式如下:x_c = ∑(i * f(i)) / Ny_c = ∑(j * f(i)) / N其中,x_c和y_c分别表示图像的质心的x和y坐标,i和j表示图像的像素的灰度级别,f(i)表示该灰度级别出现的频率,N 为图像的像素总数。
根据上述公式,可以通过以下步骤来计算图像的灰度质心:1. 将图像转换为灰度图像。
2. 对灰度图像的每个像素进行遍历,统计每个灰度级别的出现频率。
3. 根据公式计算x和y的质心坐标。
4. 将计算得到的质心坐标应用于图像处理的后续任务。
灰度质心法的应用范围广泛,以下是一些常见的应用场景:1. 图像分类和识别:通过计算图像的质心,可以用于图像分类和识别任务中。
不同类别的图像在质心坐标上往往有明显的差异,因此可以利用质心作为特征进行分类和识别。
2. 图像定位和目标检测:通过计算图像的质心,可以用于图像定位和目标检测任务中。
对于特定目标或感兴趣区域,可以通过计算质心坐标来确定目标位置。
3. 图像增强和处理:通过计算图像的质心,可以用于图像增强和处理任务中。
质心坐标可以提供图像亮度分布的信息,可以用于调整图像的亮度和对比度,改善图像的视觉效果。
总结来说,灰度质心法是一种用于图像处理中的颜色特征提取方法。
它通过计算图像中灰度值的加权平均来得到图像的质心位置,从而描述图像的整体亮度分布情况。
灰度质心法可以应用于图像分类、识别、定位、目标检测、增强和处理等各个领域。
灰度归一化的概念
灰度归一化的概念灰度归一化是数字图像处理中的一种非常重要的技术,其目的是将图像的灰度值重新映射到0至255(或其他范围)之间的值,以便更好地显示和分析图像。
在本文中,我们将详细介绍灰度归一化的概念和步骤。
1. 理解什么是灰度归一化灰度归一化是对图像亮度值进行统一变换的技术。
它的目的是通过拉伸灰度级的范围和增加对比度来改善图像的质量。
灰度归一化是将图像的灰度范围(如0-255)重新映射到另一个更适合特定应用程序的范围。
2. 灰度归一化的步骤2.1 计算像素点的最大值和最小值首先,需要计算图像中所有像素点的最大值和最小值。
这一步可以通过程序或手动方式来实现。
2.2 计算拉伸比例计算拉伸比例是灰度归一化的一个重要步骤。
拉伸比例是一个变换因子,用于将图像的灰度范围重新映射到更适合特定应用程序的范围。
拉伸比例的公式如下:stretch_ratio = (new_max - new_min) / (max - min)其中,new_max和new_min是新的灰度级范围,max和min是原始灰度值的最大和最小值。
2.3 执行灰度归一化现在可以将拉伸比例应用到图像上了。
这意味着将根据拉伸比例重新映射像素值。
具体实施时,每个像素的新值都是通过将原始值减去最小值,将其余数乘以拉伸比例,再加上新的最小值来获得的。
公式如下:new_pixel_value = (pixel_value - min) * stretch_ratio + new_min在这个公式中,pixel_value表示原始的像素灰度值,new_pixel_value是经过灰度归一化后的像素值。
3. 总结灰度归一化是数字图像处理中的一项基本技术,它通过重新映射像素的灰度值,进一步优化图像的质量,以使其更适用于不同的应用程序。
本文介绍了灰度归一化的基本概念和步骤,包括计算像素最大值和最小值、计算拉伸比例以及执行灰度归一化等。
在实际应用中,灰度归一化的方法应该根据具体情况加以定制,以获得更好的效果。
目标跟踪算法的分类
主要基于两种思路:a)不依赖于先验知识,直接从图像序列中检测到运动目标,并进行目标识别,最终跟踪感兴趣的运动目标;b)依赖于目标的先验知识,首先为运动目标建模,然后在图像序列中实时找到相匹配的运动目标。
一.运动目标检测对于不依赖先验知识的目标跟踪来讲,运动检测是实现跟踪的第一步。
运动检测即为从序列图像中将变化区域从背景图像中提取出来。
运动目标检测的算法依照目标与摄像机之间的关系可以分为静态背景下运动检测和动态背景下运动检测〔一〕静态背景1.背景差2.帧差3.GMM4.光流背景减算法可以对背景的光照变化、噪声干扰以及周期性运动等进行建模,在各种不同情况下它都可以准确地检测出运动目标。
因此对于固定摄像头的情形,目前大多数的跟踪算法中都采用背景减算法来进行目标检测。
背景减算法的局限性在于它需要一个静态的固定摄像头。
〔二〕运动场通常情况下,摄像机的运动形式可以分为两种:a)摄像机的支架固定,但摄像机可以偏转、俯仰以及缩放; b)将摄像机装在某个移动的载体上。
由于以上两种情况下的背景及前景图像都在做全局运动,要准确检测运动目标的首要任务是进行图像的全局运动估计与补偿。
考虑到图像帧上各点的全局运动矢量虽不尽相同(摄像机做平移运动除外),但它们均是在同一摄像机模型下的运动,因而应遵循相同的运动模型,可以用同一模型参数来表示。
全局运动的估计问题就被归结为全局运动模型参数的估计问题,通常使用块匹配法或光流估计法来进行运动参数的估计。
块匹配基于块的运动估算和补偿可算是最通用的算法。
可以将图像分割成不同的图像块,假定同一图像小块上的运动矢量是相同的,通过像素域搜索得到最正确的运动矢量估算。
块匹配法主要有如下三个关键技术:a)匹配法则,如最大相关、最小误差等b)搜索方法,如三步搜索法、交叉搜索法等。
c) 块大小确实定,如分级、自适应等。
光流法光流估计的方法都是基于以下假设:图像灰度分布的变化完全是目标或者场景的运动引起的,也就是说,目标与场景的灰度不随时间变化。
- 1、下载文档前请自行甄别文档内容的完整性,平台不提供额外的编辑、内容补充、找答案等附加服务。
- 2、"仅部分预览"的文档,不可在线预览部分如存在完整性等问题,可反馈申请退款(可完整预览的文档不适用该条件!)。
- 3、如文档侵犯您的权益,请联系客服反馈,我们会尽快为您处理(人工客服工作时间:9:00-18:30)。
计算机辅助设计与图形学学报
J OU RNAL OF COMPU TER2A IDED DESIGN & COMPU TER GRAPHICS
Vol116 , No110
Oct1 , 2004
灰度图像质心快速算法
王 冰 职秦川 张仲选 耿国华 周明全
1362
计算机辅助设计与图形学学报
2004 年
NN
∑∑ m 10 =
i f ( i , j) ,
j =1 i =1
NN
∑∑ m 01 =
j f ( i , j) 1
j =1 i =1
ic =
m 10 m 00
(4)
jc =
m 01 m 00
(5)
点 p ( ic , jc) 即为质心坐标1
令
N
∑q) 和中心矩 (μpq) 定义为
∞∞
∫∫ m pq =
x pyqf ( x , y) d x d y ,
- ∞- ∞
∞∞
∫∫ μpq =
( x - x ) p ( y - y) qf ( x , y) d x d y ,
- ∞- ∞
其中 p 和 q 是非负的整数1 对离散化的数字图像 ,
m 03 , m 12 , m 21) 描述物体的方位和斜度[1 ]1 基于低
阶的 10 个矩 , Hu[2 ] 给出一组不变矩 , 不变矩有平 移 、旋转 、缩放不变的特性1 不变矩独特的特性获得 广大图像处理工作者的青睐 ,并在图像分类 、模式识 别 、计算机视觉等图像处理和分析领域获得广泛的 应用1 由于求矩过程复杂 , 运算量大 , 限制了它的应 用1 自 20 世纪 80 年代以来 , 快速求矩算法不断涌 现1 从算法原理分析 ,文献[3 26 ]基于 δ方法将图像 分解为行 ,图像的质心或矩是各行质心或矩的综合 ; 文献[7 29 ]运用格林理论方法 ,该方法基于格林公式 f ( x , y) d x d y = ∮[ M d x + N d y ] , 把一个图像函数 求质心或矩的二重积分转换为对图像边缘的一重积 分 ;文献[10 211 ]采用图像变换方法将图像函数转换 为其他函数 ,利用变换后函数的独有特性简化计算1 在这些算法中 ,大都计算到第 3 阶矩1 由于第 0 、第 1 阶矩 (即求物体的质心) 具有最为基础和最为广泛的 应用 ,并且由矩和中心矩定义知 ,质心又是求中心矩 的基础 ,人们对快速求取质心或前 2 阶矩给予更多 的关注[12214 ]1 文献 [ 12 214 ]介绍和发展了一种称之 为快速搜索质心算法 , 利用目标质心与目标上所有 各点间距离之和值最小的原理 , 快速求取质心1 从 算法适用性上看 ,以上算法大都适用于二值图像 ,只 有文献[9 ,11 ]中算法适用于灰度图像1 由于实际中 的图像大都是灰度图像 , 因此对灰度图像求质心具 有更广泛的用途和实际的意义1 本文提出一种基于 函数转换的求质心算法 ( Function Transformation Al2 gorit hm ,F TA) ,该算法适用于灰度图像1 和其他求 灰度图像质心算法相比 ,本文算法具有更高的计算 效率1
设
f 1 ( n) = [5 ,4 ,6 ,2 ,3 ] ,
f 2 ( n) = [1 ,3 ,4 ,2 ,5 ]1 则
F1 ( n) = [1 , - 2 ,4 , - 1 ,3 ] ,
F2 ( n) = [1 ,4 ,8 ,10 ,15 ]1
N
∑f 1 ( n) f 2 ( n) = 5 ×1 + 4 ×3 +
可见 ,质心即是第 0 和第 1 阶矩1
通常 ,前 10 个矩 ( p + q ≤3) 获得最为广泛的应
原稿收到日期 :2003206220 ;修改稿收到日期 :20032102131 本课题得到国家自然科学基金 (60271032) 资助1 王 冰 ,男 ,1954 年生 ,副教授 , 主要研究方向为图像处理和分析 、计算机视觉 、模式识别及算法1 职秦川 ,男 ,1964 年生 ,硕士 ,讲师 ,主要研究方向为图像处理 、计算机图形学 等1 张仲选 ,男 ,1962 年生 ,硕士 ,讲师 ,主要研究方向为图像处理 、信息安全等1 耿国华 ,女 ,1955 年生 ,博士 ,教授 ,博士生导师 ,主要研究方向 为人工智能 、信息技术1 周明全 ,男 ,1954 年生 ,教授 ,博士生导师 ,主要研究方向为图像处理和分析 、虚拟现实 、可视化技术1
令 S (1 , j) = f (1 , j)
(12)
S ( i , j) = S ( i - 1 , j) + f ( i , j) ,
i = 2 ,3 , …, N ; j = 1 ,2 , …, N
(13)
首先证明式 (10)1
证明1 按照式 ( 12) , ( 13) , 并注意到式 ( 6) , 式
1
此时 ,
N
N
∑ ∑ f 1 ( n) f 2 ( n) =
F1 ( n) F2 ( n) = F2 ( N )
n =1
n =1
(2)
如果 f 1 ( n) = n ,则
- 1 , n = 1 ,2 , …, N - 1
F1 ( n) =
1
N, n = N
此时 ,
N
N
∑ ∑ f 1 ( n) f 2 ( n) =
以上二式变为
NN
∑∑ m pq =
ipjqf ( i , j) ,
j =1 i =1
NN
∑∑ μpq =
( i - ic) p ( j -
j =1 i =1
其中 ( ic , jc) 为质心坐标 ,且
jc) qf ( i , j) ,
ic = m 10 / m 00 , jc = m 01 / m 001
(10)
N- 1
∑ m 10 j = N ×S ( N , j) -
S ( i , j)
(11)
i =1
式 (10) , (11) 中的 S ( N , j) 相当于式 (2) 的 F2 ( N ) ,
S ( i , j) 相当于式 (3) 的 F2 ( n) 1
下面证明式 (10) , (11)1
n =1
6 ×4 + 2 ×2 + 3 ×5 = 60 ,
N
∑F1 ( n) F2 ( n) = 1 ×1 - 2 ×4 +
n =1
4 ×8 - 1 ×10 + 3 ×15 = 601
如果 f 1 ( n) = 1 ,则
0 , n = 1 ,2 , …, N - 1
F1 ( n) = 1 , n = N
(6)
i =1
N
∑ m 10 j = i f ( i , j)
(7)
i =1
则
N
∑ m 00 =
m 00 j
(8)
j =1
N
∑ m 10 =
m 10 j
(9)
j =1
注意到式 (6) 满足式 (2) 的条件 , 式 (7) 满足式 (3) 的
条件1 从式 (2) , (3) 有
m 00 j = S ( N , j)
Key words geomet ric moment s ; center of mass ; fast algorit hm ; pattern recognition ; computational co mple xit y
1 引 言
对一幅 2D 连续图像 f ( x , y) ( ≥0) , p + q 阶矩
F1 ( n) F2 ( n)
(1)
n =1
n =1
其中 ,
F1 ( n) = f 1 ( n) - f 1 ( n + 1) ,
F2 ( n) = F2 ( n - 1) + f 2 ( n) ,
F2 (0) = 0 ,
f 1 ( N + 1) = 01 式 (1) 证明见正文后附录1
下面验证式 (1) 的正确性1
© 1995-2005 Tsinghua Tongfang Optical Disc Co., Ltd. All rights reserved.
10 期
王 冰等 :灰度图像质心快速算法
1361
用 ,而且它们都有实在的物理意义10 阶矩 ( m 00) 为 物体的质量 ; 1 阶矩 ( m 10 , m 01) 表示物体的质心 ; 2 阶矩 ( m 20 , m 02 , m 11 ) 表示旋转半径 ; 3 阶矩 ( m 30 ,
运算1
212 算法原理
几何矩 m pq ( p , q = 1 ,2 , …) 定义为
NN
∑∑ m pq =
ipjqf ( i , j) ,
j =1 i =1
则第 0 阶和第 1 阶矩为
NN
∑∑ m 00 =
f ( i , j) ,
j =1 i =1
© 1995-2005 Tsinghua Tongfang Optical Disc Co., Ltd. All rights reserved.
2 算 法
211 基本思想
设有二 个 大 小 同 为 N 的 一 维 数 组 f 1 ( n ) 和 f 2 ( n) , n = 1 , 2 , …, N 1 则这两个数组的乘积等于
将其中的一个数组 f 1 ( n) 差分与另一个数组 f 2 ( n) 累进求和后的乘积 ,即
N
N
∑ ∑ f 1 ( n) f 2 ( n) =
(西北大学计算机科学系 西安 710069)
摘 要 对矩因子 x pyq 做差分变换为函数 F1 () ,将图像函数 f ( x , y) 做累进求和变换为函数 F2 ()1 用 F1 () 和 F2 () 相乘求取质心1 由于 0 阶和 1 阶矩因子中的 p , q 不大于 1 ,经差分后的 F1 () 除右端点外 ,其值都为 1 ,乘 1 的运算当 然可以不做 ,从而消去了乘法运算1 对任意大小和任意级别的灰度图像 ,乘除法运算次数仅为 3 次 ,而加法运算次数 也有降低1 文中算法计算结果精确 ,其运算效率高于已有其他算法1