数据挖掘外文翻译
高级数据挖掘专业词汇100

高级数据挖掘专业词汇100高级数据挖掘课程相关专业词汇1001.Data Mining, DM数据挖掘2.Knowledge Discover In Database, KDD数据库知识发现3.Artificial Intelligence, AI人工智能4.Business Intelligence, BI商务智能5.Pattern Recognition 模式识别6.Machine Learning 机器学习7.Data Analysis 数据分析8.Cluster Analysis 聚类分析9.Associative Analysis 关联分析10.Data Warehouse, DW数据仓库11.On-Line Analytical Processing, OLAP联机分析处理12.On-Line Transaction Processing 联机事务处理13.Classification 分类14.Forecast Inginformation预测性信息15.Artificial Neural Networks 人工神经网络16.Data Visualization 数据可视化17.Decision Tree 决策树18.Genetic Algorithms 遗传算法19.Linear Model 线性模型20.Non-Linear Model 非线性模型21.Market Basket Analysis 购物篮分析22.Social Network Analysis 社交网络分析23.Unstructured Data 非结构化数据24.Activation 激励函数25.Cross Validation 交叉验证26.Database Management Systems 数据库管理系统27.Decision Tree 决策树28.Fuzzy Logic 模糊逻辑29.K-Nearest Neighbor K最近邻算法30.Least Squares 最小二乘法31.Logisitc Regression 逻辑回归32.Overfitting 过拟合33.Empirical Risk 经验风险34.Preprocess 预处理35.Tomb Data 历史数据36.Data Dredging 数据捕捞37.Credit Risk 信用风险38.Data Mart 数据集市39.Log File 日志文件40.Data Extraction 数据提取41.Feature Representation 特征表示42.Association Rules 关联规则43.Distributed Computing 分布式计算44.Pattern Matching 模式匹配45.Context Awareness情境感知46.Data Exchange 数据交换47.Feature Extraction 体征提取48.Sampling 抽样49.Supervised Learning 监督学习50.Unsupervised Learning 无监督学习51.Semi-Supervised Learning 半监督学习52.Data Structure 数据结构53.Data Retrieval 数据检索54.Link Structure 链路结构55.Time Sequence 时间序列56.Graph Theory 图形理论57.Hierarchical Structure 分层结构58.Spatio-Temporal Data 时空数据59.Remote Monitoring 远程控制60.Data Uncertainty 数据不确定性61.Geographic Information System 地理信息系统62.Data Stream 数据流63.Optimization 优化64.Incremental Learning 增量学习65.Semi-Structured Data 半结构化数据66.Structured Data 结构化数据67.Unstructured Data 非结构化数据68.Self-Organization Data 自组织数据69.Intrusion Detection 入侵检测70.AbnormityDetection 异常检测71.Sequence Similarity 序列相似性72.Feature Weighting 特征加权73.Data Constraints 数据约束74.Dimension Reduction 降维75.Data Partitioning 数据分割76.Decision Support 决策支持77.Frequent Items 频繁项集78.Match Degree 匹配度79.Support Vector Machine 支持向量机80.Neural Network 神经网络81.Route Analysis 路径分析82.Interest Pattern 兴趣模式83.Genetic Algorithm 遗传算法84.Rough Set 粗糙集85.Data Cleaning 数据清洗86.Temporal Data 时态数据87.Cloud Computing 云计算88.CollaborativeFiltering 协同过滤89.Grid Computing 网格计算90.Parallel Computing 并行计算91.Fuzzy Clustering 模糊聚类92.Data Prediction 数据预测93.Behavior Prediction 行为预测94.Personalized Recommendation 个性化推荐95.Semantic Rule 语义规则96.Real-Time Decisioning实时决策97.Deep Learning深度学习98.Attribute 属性99.Test Data 测试数据100.Train Data 训练数据。
数据挖掘中的名词解释

第一章1,数据挖掘(Data Mining),就是从存放在数据库,数据仓库或其他信息库中的大量的数据中获取有效的、新颖的、潜在有用的、最终可理解的模式的非平凡过程。
2,人工智能(Artificial Intelligence)它是研究、开发用于模拟、延伸和扩展人的智能的理论、方法、技术及应用系统的一门新的技术科学。
人工智能是计算机科学的一个分支,它企图了解智能的实质,并生产出一种新的能以人类智能相似的方式做出反应的智能机器。
3,机器学习(Machine Learning)是研究计算机怎样模拟或实现人类的学习行为,以获取新的知识或技能,重新组织已有的知识结构使之不断改善自身的性能。
4,知识工程(Knowledge Engineering)是人工智能的原理和方法,对那些需要专家知识才能解决的应用难题提供求解的手段。
5,信息检索(Information Retrieval)是指信息按一定的方式组织起来,并根据信息用户的需要找出有关的信息的过程和技术。
6,数据可视化(Data Visualization)是关于数据之视觉表现形式的研究;其中,这种数据的视觉表现形式被定义为一种以某种概要形式抽提出来的信息,包括相应信息单位的各种属性和变量。
7,联机事务处理系统(OLTP)实时地采集处理与事务相连的数据以及共享数据库和其它文件的地位的变化。
在联机事务处理中,事务是被立即执行的,这与批处理相反,一批事务被存储一段时间,然后再被执行。
8, 联机分析处理(OLAP)使分析人员,管理人员或执行人员能够从多角度对信息进行快速一致,交互地存取,从而获得对数据的更深入了解的一类软件技术。
8,决策支持系统(decision support)是辅助决策者通过数据、模型和知识,以人机交互方式进行半结构化或非结构化决策的计算机应用系统。
它为决策者提供分析问题、建立模型、模拟决策过程和方案的环境,调用各种信息资源和分析工具,帮助决策者提高决策水平和质量。
数据挖掘综述
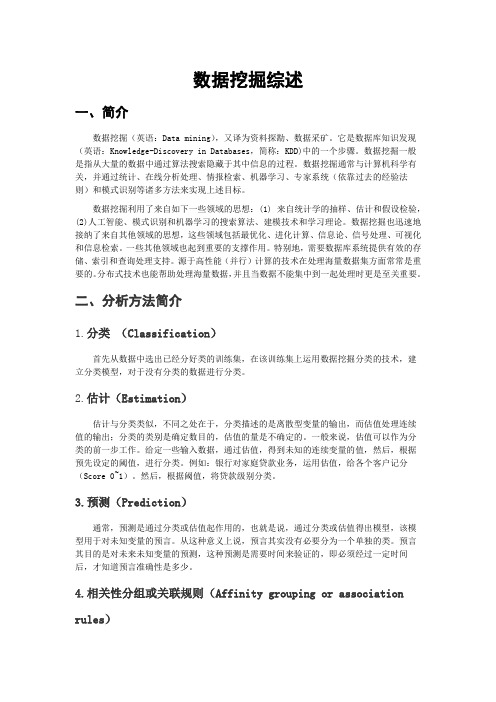
数据挖掘综述一、简介数据挖掘(英语:Data mining),又译为资料探勘、数据采矿。
它是数据库知识发现(英语:Knowledge-Discovery in Databases,简称:KDD)中的一个步骤。
数据挖掘一般是指从大量的数据中通过算法搜索隐藏于其中信息的过程。
数据挖掘通常与计算机科学有关,并通过统计、在线分析处理、情报检索、机器学习、专家系统(依靠过去的经验法则)和模式识别等诸多方法来实现上述目标。
数据挖掘利用了来自如下一些领域的思想:(1) 来自统计学的抽样、估计和假设检验,(2)人工智能、模式识别和机器学习的搜索算法、建模技术和学习理论。
数据挖掘也迅速地接纳了来自其他领域的思想,这些领域包括最优化、进化计算、信息论、信号处理、可视化和信息检索。
一些其他领域也起到重要的支撑作用。
特别地,需要数据库系统提供有效的存储、索引和查询处理支持。
源于高性能(并行)计算的技术在处理海量数据集方面常常是重要的。
分布式技术也能帮助处理海量数据,并且当数据不能集中到一起处理时更是至关重要。
二、分析方法简介1.分类(Classification)首先从数据中选出已经分好类的训练集,在该训练集上运用数据挖掘分类的技术,建立分类模型,对于没有分类的数据进行分类。
2.估计(Estimation)估计与分类类似,不同之处在于,分类描述的是离散型变量的输出,而估值处理连续值的输出;分类的类别是确定数目的,估值的量是不确定的。
一般来说,估值可以作为分类的前一步工作。
给定一些输入数据,通过估值,得到未知的连续变量的值,然后,根据预先设定的阈值,进行分类。
例如:银行对家庭贷款业务,运用估值,给各个客户记分(Score 0~1)。
然后,根据阈值,将贷款级别分类。
3.预测(Prediction)通常,预测是通过分类或估值起作用的,也就是说,通过分类或估值得出模型,该模型用于对未知变量的预言。
从这种意义上说,预言其实没有必要分为一个单独的类。
第七章 数据挖掘

数据挖掘语言
Automated vs. query-driven? queryFinding all the patterns autonomously in a database?—unrealistic database?— because the patterns could be too many but uninteresting Data mining should be an interactive process User directs what to be mined Users must be provided with a set of primitives to be used to communicate with the data mining system Incorporating these primitives in a data mining query language More flexible user interaction Foundation for design of graphical user interface Standardization of data mining industry and practice
Choosing the mining algorithm(s) Data mining: search for patterns of interest mining: Pattern evaluation and knowledge presentation
visualization, transformation, removing redundant patterns, etc.
relevant prior knowledge and goals of application
Data-mining-clustering数据挖掘—聚类分析大学毕业论文外文文献翻译及原文

Data-mining-clustering数据挖掘—聚类分析大学毕业论文外文文献翻译及原文毕业设计(论文)外文文献翻译文献、资料中文题目:聚类分析文献、资料英文题目:clustering文献、资料来源:文献、资料发表(出版)日期:院(部):专业:自动化班级:姓名:学号:指导教师:翻译日期: 2017.02.14外文翻译英文名称:Data mining-clustering译文名称:数据挖掘—聚类分析专业:自动化姓名:****班级学号:****指导教师:******译文出处:Data mining:Ian H.Witten, EibeFrank 著Clustering5.1 INTRODUCTIONClustering is similar to classification in that data are grouped. However, unlike classification, the groups are not predefined. Instead, the grouping is accomplished by finding similarities between data according to characteristics found in the actualdata. The groups are called clusters. Some authors view clustering as a special type of classification. In this text, however, we follow a more conventional view in that the two are different. Many definitions for clusters have been proposed:●Set of like elements. Elements from different clusters are not alike.●The distance between points in a cluster is less than the distance betweena point in the cluster and any point outside it.A term similar to clustering is database segmentation, where like tuple (record) in a database are grouped together. This is done to partition or segment the database into components that then give the user a more general view of the data. In this case text, we do not differentiate between segmentation and clustering. A simple example of clustering is found in Example 5.1. This example illustrates the fact that that determining how to do the clustering is not straightforward.As illustrated in Figure 5.1, a given set of data may be clustered on different attributes. Here a group of homes in a geographic area is shown. The first floor type of clustering is based on the location of the home. Homes that are geographically close to each other are clustered together. In the second clustering, homes are grouped based on the size of the house.Clustering has been used in many application domains, including biology, medicine, anthropology, marketing, and economics. Clustering applications include plant and animal classification, disease classification, image processing, pattern recognition, and document retrieval. One of the first domains in which clustering was used was biological taxonomy. Recent usesinclude examining Web log data to detect usage patterns.When clustering is applied to a real-world database, many interesting problems occur:●Outlier handling is difficult. Here the elements do not naturally fallinto any cluster. They can be viewed as solitary clusters. However, if aclustering algorithm attempts to find larger clusters, these outliers will beforced to be placed in some cluster. This process may result in the creationof poor clusters by combining two existing clusters and leaving the outlier in its own cluster.● Dynamic data in the database implies that cluster membership may change over time.● Interpreting the semantic meaning of each cluster may be difficult. With classification, the labeling of the classes is known ahead of time. However, with clustering, this may not be the case. Thus, when the clustering process finishes creating a set of clusters, the exact meaning of each cluster may not be obvious. Here is where a domain expert is needed to assign a label or interpretation for each cluster.● There is no one correct answer to a clustering problem. In fact, many answers may be found. The exact number of clusters required is not easy to determine. Again, a domain expert may be required. For example, suppose we have a set of data about plants that have been collected during a field trip. Without any prior knowledge of plant classification, if we attempt to divide this set of data into similar groupings, it would not be clear how many groups should be created.● Another related iss ue is what data should be used of clustering. Unlike learning during a classification process, where there is some a priori knowledge concerning what the attributes of each classification should be, in clustering we have no supervised learning to aid the process. Indeed, clustering can be viewed as similar to unsupervised learning.We can then summarize some basic features of clustering (as opposed to classification):● The (best) number of clusters is not known.● There may not be any a priori knowledge co ncerning the clusters. ● Cluster results are dynamic.The clustering problem is stated as shown in Definition 5.1. Here we assume that the number of clusters to be created is an input value, k. The actual content (and interpretation) of each cluster,j k ,1j k ≤≤, is determined as a result of the function definition. Without loss of generality, we will view that the result of solving a clustering problem is that a set of clusters is created: K={12,,...,k k k k }.D EFINITION 5.1.Given a database D ={12,,...,n t t t } of tuples and aninteger value k , the clustering problem is to define a mapping f : {1,...,}D k → where each i t is assigned to one cluster j K ,1j k ≤≤. A cluster j K , contains precisely those tuples mapped to it; that is,j K ={|(),1,i i j t f t K i n =≤≤and i t D ∈}.A classification of the different types of clustering algorithms is shown in Figure 5.2. Clustering algorithms themselves may be viewed as hierarchical or partitional. With hierarchical clustering, a nested set of clusters is created. Each level in the hierarchy has a separate set of clusters. At the lowest level, each item is in itsown unique cluster. At the highest level, all items belong to the same cluster. With hierarchical clustering, the desired number of clusters is not input. With partitional clustering, the algorithm creates only one set of clusters. These approaches use the desired number of clusters to drive how the final set is created. Traditional clustering algorithms tend to be targeted to small numeric database that fit into memory .There are, however, more recent clustering algorithms that look at categorical data and are targeted to larger, perhaps dynamic, databases. Algorithms targeted to larger databases may adapt to memory constraints by either sampling the database or using data structures, which can be compressed or pruned to fit into memory regardless of the size of the database. Clustering algorithms may also differ based on whether they produce overlapping or nonoverlapping clusters. Even though we consider only nonoverlapping clusters, it is possible to place an item in multiple clusters. In turn, nonoverlapping clusters can be viewed as extrinsic or intrinsic. Extrinsic techniques use labeling of the items to assist in the classification process. These algorithms are the traditional classification supervised learning algorithms in which a special input training set is used. Intrinsic algorithms do not use any a priori category labels, but depend only on the adjacency matrix containing the distance between objects. All algorithms we examine in this chapter fall into the intrinsic class.The types of clustering algorithms can be furthered classified based on the implementation technique used. Hierarchical algorithms can becategorized as agglomerat ive or divisive. ”Agglomerative ” implies that the clusters are created in a bottom-up fashion, while divisive algorithms work in a top-down fashion. Although bothhierarchical and partitional algorithms could be described using the agglomerative vs. divisive label, it typically is more associated with hierarchical algorithms. Another descriptive tag indicates whether each individual element is handled one by one, serial (sometimes called incremental), or whether all items are examined together, simultaneous. If a specific tuple is viewed as having attribute values for all attributes in the schema, then clustering algorithms could differ as to how the attribute values are examined. As is usually done with decision tree classification techniques, some algorithms examine attribute values one at a time, monothetic. Polythetic algorithms consider all attribute values at one time. Finally, clustering algorithms can be labeled base on the mathematical formulation given to the algorithm: graph theoretic or matrix algebra. In this chapter we generally use the graph approach and describe the input to the clustering algorithm as an adjacency matrix labeled with distance measure.We discuss many clustering algorithms in the following sections. This is only a representative subset of the many algorithms that have been proposed in the literature. Before looking at these algorithms, we first examine possible similarity measures and examine the impact of outliers.5.2 SIMILARITY AND DISTANCE MEASURESThere are many desirable properties for the clusters created by a solution to a specific clustering problem. The most important one is that a tuple within one cluster is more like tuples within that cluster than it is similar to tuples outside it. As with classification, then, we assume the definition of a similarity measure, sim(,i l t t ), defined between any two tuples, ,i l t t D . This provides a more strict and alternative clustering definition, as found in Definition 5.2. Unless otherwise stated, we use thefirst definition rather than the second. Keep in mind that the similarity relationship stated within the second definition is a desirable, although not always obtainable, property.A distance measure, dis(,i j t t ), as opposed to similarity, is often used in。
数据挖掘技术中英文对照外文翻译文献
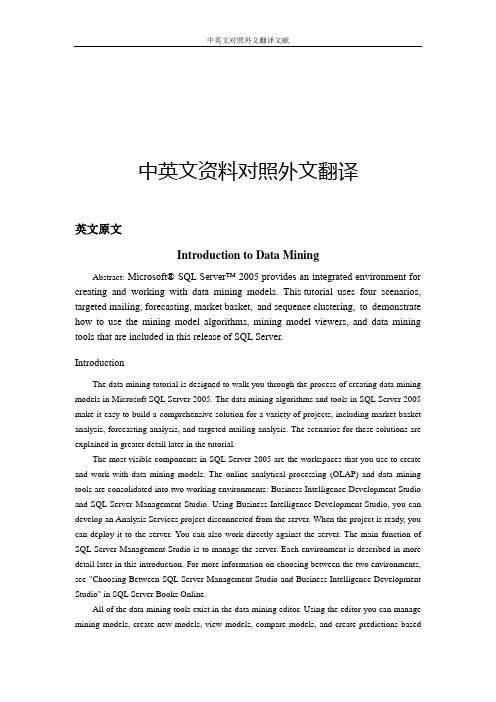
中英文对照外文翻译文献中英文资料对照外文翻译英文原文Introduction to Data MiningAbstract:Microsoft® SQL Server™ 2005 provides an integrated environment for creating and working with data mining models. This tutorial uses four scenarios, targeted mailing, forecasting, market basket, and sequence clustering, to demonstrate how to use the mining model algorithms, mining model viewers, and data mining tools that are included in this release of SQL Server.IntroductionThe data mining tutorial is designed to walk you through the process of creating data mining models in Microsoft SQL Server 2005. The data mining algorithms and tools in SQL Server 2005 make it easy to build a comprehensive solution for a variety of projects, including market basket analysis, forecasting analysis, and targeted mailing analysis. The scenarios for these solutions are explained in greater detail later in the tutorial.The most visible components in SQL Server 2005 are the workspaces that you use to create and work with data mining models. The online analytical processing (OLAP) and data mining tools are consolidated into two working environments: Business Intelligence Development Studio and SQL Server Management Studio. Using Business Intelligence Development Studio, you can develop an Analysis Services project disconnected from the server. When the project is ready, you can deploy it to the server. You can also work directly against the server. The main function of SQL Server Management Studio is to manage the server. Each environment is described in more detail later in this introduction. For more information on choosing between the two environments, see "Choosing Between SQL Server Management Studio and Business Intelligence Development Studio" in SQL Server Books Online.All of the data mining tools exist in the data mining editor. Using the editor you can manage mining models, create new models, view models, compare models, and create predictions basedon existing models.After you build a mining model, you will want to explore it, looking for interesting patterns and rules. Each mining model viewer in the editor is customized to explore models built with a specific algorithm. For more information about the viewers, see "Viewing a Data Mining Model" in SQL Server Books Online.Often your project will contain several mining models, so before you can use a model to create predictions, you need to be able to determine which model is the most accurate. For this reason, the editor contains a model comparison tool called the Mining Accuracy Chart tab. Using this tool you can compare the predictive accuracy of your models and determine the best model.To create predictions, you will use the Data Mining Extensions (DMX) language. DMX extends SQL, containing commands to create, modify, and predict against mining models. For more information about DMX, see "Data Mining Extensions (DMX) Reference" in SQL Server Books Online. Because creating a prediction can be complicated, the data mining editor contains a tool called Prediction Query Builder, which allows you to build queries using a graphical interface. You can also view the DMX code that is generated by the query builder.Just as important as the tools that you use to work with and create data mining models are the mechanics by which they are created. The key to creating a mining model is the data mining algorithm. The algorithm finds patterns in the data that you pass it, and it translates them into a mining model — it is the engine behind the process.Some of the most important steps in creating a data mining solution are consolidating, cleaning, and preparing the data to be used to create the mining models. SQL Server 2005 includes the Data Transformation Services (DTS) working environment, which contains tools that you can use to clean, validate, and prepare your data. For more information on using DTS in conjunction with a data mining solution, see "DTS Data Mining Tasks and Transformations" in SQL Server Books Online.In order to demonstrate the SQL Server data mining features, this tutorial uses a new sample database called AdventureWorksDW. The database is included with SQL Server 2005, and it supports OLAP and data mining functionality. In order to make the sample database available, you need to select the sample database at the installation time in the “Advanced” d ialog for component selection.Adventure WorksAdventureWorksDW is based on a fictional bicycle manufacturing company named Adventure Works Cycles. Adventure Works produces and distributes metal and composite bicycles to North American, European, and Asian commercial markets. The base of operations is located in Bothell, Washington with 500 employees, and several regional sales teams are located throughout their market base.Adventure Works sells products wholesale to specialty shops and to individuals through theInternet. For the data mining exercises, you will work with the AdventureWorksDW Internet sales tables, which contain realistic patterns that work well for data mining exercises.For more information on Adventure Works Cycles see "Sample Databases and Business Scenarios" in SQL Server Books Online.Database DetailsThe Internet sales schema contains information about 9,242 customers. These customers live in six countries, which are combined into three regions:North America (83%)Europe (12%)Australia (7%)The database contains data for three fiscal years: 2002, 2003, and 2004.The products in the database are broken down by subcategory, model, and product.Business Intelligence Development StudioBusiness Intelligence Development Studio is a set of tools designed for creating business intelligence projects. Because Business Intelligence Development Studio was created as an IDE environment in which you can create a complete solution, you work disconnected from the server. You can change your data mining objects as much as you want, but the changes are not reflected on the server until after you deploy the project.Working in an IDE is beneficial for the following reasons:The Analysis Services project is the entry point for a business intelligence solution. An Analysis Services project encapsulates mining models and OLAP cubes, along with supplemental objects that make up the Analysis Services database. From Business Intelligence Development Studio, you can create and edit Analysis Services objects within a project and deploy the project to the appropriate Analysis Services server or servers.If you are working with an existing Analysis Services project, you can also use Business Intelligence Development Studio to work connected the server. In this way, changes are reflected directly on the server without having to deploy the solution.SQL Server Management StudioSQL Server Management Studio is a collection of administrative and scripting tools for working with Microsoft SQL Server components. This workspace differs from Business Intelligence Development Studio in that you are working in a connected environment where actions are propagated to the server as soon as you save your work.After the data has been cleaned and prepared for data mining, most of the tasks associated with creating a data mining solution are performed within Business Intelligence Development Studio. Using the Business Intelligence Development Studio tools, you develop and test the datamining solution, using an iterative process to determine which models work best for a given situation. When the developer is satisfied with the solution, it is deployed to an Analysis Services server. From this point, the focus shifts from development to maintenance and use, and thus SQL Server Management Studio. Using SQL Server Management Studio, you can administer your database and perform some of the same functions as in Business Intelligence Development Studio, such as viewing, and creating predictions from mining models.Data Transformation ServicesData Transformation Services (DTS) comprises the Extract, Transform, and Load (ETL) tools in SQL Server 2005. These tools can be used to perform some of the most important tasks in data mining: cleaning and preparing the data for model creation. In data mining, you typically perform repetitive data transformations to clean the data before using the data to train a mining model. Using the tasks and transformations in DTS, you can combine data preparation and model creation into a single DTS package.DTS also provides DTS Designer to help you easily build and run packages containing all of the tasks and transformations. Using DTS Designer, you can deploy the packages to a server and run them on a regularly scheduled basis. This is useful if, for example, you collect data weekly data and want to perform the same cleaning transformations each time in an automated fashion.You can work with a Data Transformation project and an Analysis Services project together as part of a business intelligence solution, by adding each project to a solution in Business Intelligence Development Studio.Mining Model AlgorithmsData mining algorithms are the foundation from which mining models are created. The variety of algorithms included in SQL Server 2005 allows you to perform many types of analysis. For more specific information about the algorithms and how they can be adjusted using parameters, see "Data Mining Algorithms" in SQL Server Books Online.Microsoft Decision TreesThe Microsoft Decision Trees algorithm supports both classification and regression and it works well for predictive modeling. Using the algorithm, you can predict both discrete and continuous attributes.In building a model, the algorithm examines how each input attribute in the dataset affects the result of the predicted attribute, and then it uses the input attributes with the strongest relationship to create a series of splits, called nodes. As new nodes are added to the model, a tree structure begins to form. The top node of the tree describes the breakdown of the predicted attribute over the overall population. Each additional node is created based on the distribution of states of the predicted attribute as compared to the input attributes. If an input attribute is seen tocause the predicted attribute to favor one state over another, a new node is added to the model. The model continues to grow until none of the remaining attributes create a split that provides an improved prediction over the existing node. The model seeks to find a combination of attributes and their states that creates a disproportionate distribution of states in the predicted attribute, therefore allowing you to predict the outcome of the predicted attribute.Microsoft ClusteringThe Microsoft Clustering algorithm uses iterative techniques to group records from a dataset into clusters containing similar characteristics. Using these clusters, you can explore the data, learning more about the relationships that exist, which may not be easy to derive logically through casual observation. Additionally, you can create predictions from the clustering model created by the algorithm. For example, consider a group of people who live in the same neighborhood, drive the same kind of car, eat the same kind of food, and buy a similar version of a product. This is a cluster of data. Another cluster may include people who go to the same restaurants, have similar salaries, and vacation twice a year outside the country. Observing how these clusters are distributed, you can better understand how the records in a dataset interact, as well as how that interaction affects the outcome of a predicted attribute.Microsoft Naïve BayesThe Microsoft Naïve Bayes algorithm quickly builds mining models that can be used for classification and prediction. It calculates probabilities for each possible state of the input attribute, given each state of the predictable attribute, which can later be used to predict an outcome of the predicted attribute based on the known input attributes. The probabilities used to generate the model are calculated and stored during the processing of the cube. The algorithm supports only discrete or discretized attributes, and it considers all input attributes to be independent. The Microsoft Naïve Bayes algorithm produces a simple mining model that can be considered a starting point in the data mining process. Because most of the calculations used in creating the model are generated during cube processing, results are returned quickly. This makes the model a good option for exploring the data and for discovering how various input attributes are distributed in the different states of the predicted attribute.Microsoft Time SeriesThe Microsoft Time Series algorithm creates models that can be used to predict continuous variables over time from both OLAP and relational data sources. For example, you can use the Microsoft Time Series algorithm to predict sales and profits based on the historical data in a cube.Using the algorithm, you can choose one or more variables to predict, but they must be continuous. You can have only one case series for each model. The case series identifies the location in a series, such as the date when looking at sales over a length of several months or years.A case may contain a set of variables (for example, sales at different stores). The Microsoft Time Series algorithm can use cross-variable correlations in its predictions. For example, prior sales at one store may be useful in predicting current sales at another store.Microsoft Neural NetworkIn Microsoft SQL Server 2005 Analysis Services, the Microsoft Neural Network algorithm creates classification and regression mining models by constructing a multilayer perceptron network of neurons. Similar to the Microsoft Decision Trees algorithm provider, given each state of the predictable attribute, the algorithm calculates probabilities for each possible state of the input attribute. The algorithm provider processes the entire set of cases , iteratively comparing the predicted classification of the cases with the known actual classification of the cases. The errors from the initial classification of the first iteration of the entire set of cases is fed back into the network, and used to modify the network's performance for the next iteration, and so on. You can later use these probabilities to predict an outcome of the predicted attribute, based on the input attributes. One of the primary differences between this algorithm and the Microsoft Decision Trees algorithm, however, is that its learning process is to optimize network parameters toward minimizing the error while the Microsoft Decision Trees algorithm splits rules in order to maximize information gain. The algorithm supports the prediction of both discrete and continuous attributes.Microsoft Linear RegressionThe Microsoft Linear Regression algorithm is a particular configuration of the Microsoft Decision Trees algorithm, obtained by disabling splits (the whole regression formula is built in a single root node). The algorithm supports the prediction of continuous attributes.Microsoft Logistic RegressionThe Microsoft Logistic Regression algorithm is a particular configuration of the Microsoft Neural Network algorithm, obtained by eliminating the hidden layer. The algorithm supports the prediction of both discrete andcontinuous attributes.)中文译文数据挖掘技术简介摘要:微软® SQL Server™2005中提供用于创建和使用数据挖掘模型的集成环境的工作。
《数据挖掘简介》word版

数据挖掘简介数据挖掘简介2010-04-28 20:47数据挖掘数据挖掘(Data Mining)是采用数学、统计、人工智能和神经网络等领域的科学方法,从大量数据中挖掘出隐含的、先前未知的、对决策有潜在价值的关系、模式和趋势,并用这些知识和规则建立用于决策支持的模型,为商业智能系统服务的各业务领域提供预测性决策支持的方法、工具和过程。
数据挖掘前身是知识发现(KDD),属于机器学习的范畴,所用技术和工具主要有统计分析(或数据分析)和知识发现。
知识发现与数据挖掘是人工智能、机器学习与数据库技术相结合的产物,是从数据中发现有用知识的整个过程。
机器学习(Machine Learning)是用计算机模拟人类学习的一门科学,由于在专家系统开发中存在知识获取的瓶颈现象,所以采用机器学习来完成知识的自动获取。
数据挖掘是KDD过程中的一个特定步骤,它用专门算法从数据中抽取模式(Patterns)。
1996年,Fayyad、Piatetsky-Shapiror和Smyth将KDD 过程定义为:从数据中鉴别出有效模式的非平凡过程,该模式是新的、可能有用的和最终可理解的;KDD是从大量数据中提取出可信的、新颖的、有效的,并能被人理解的模式的处理过程,这种处理过程是一种高级的处理过程。
数据挖掘则是按照既定的业务目标,对大量的企业数据进行探索,揭示隐藏其中的规律性,并进一步将其设计为先进的模型和有效的操作。
在日常的数据库操作中,经常使用的是从数据库中抽取数据以生成一定格式的报表。
KDD与数据库报表工具的区别是:数据库报表制作工具是将数据库中的某些数据抽取出来,经过一些数学运算,最终以特定的格式呈现给用户;而KDD则是对数据背后隐藏的特征和趋势进行分析,最终给出关于数据的总体特征和发展趋势。
报表工具能制作出形如"上学期考试未通过及成绩优秀的学生的有关情况"的表格;但它不能回答"考试未通过及成绩优秀的学生在某些方面有些什么不同的特征"的问题,而KDD就可以回答。
数据挖掘技术综述毕业论文外文翻译
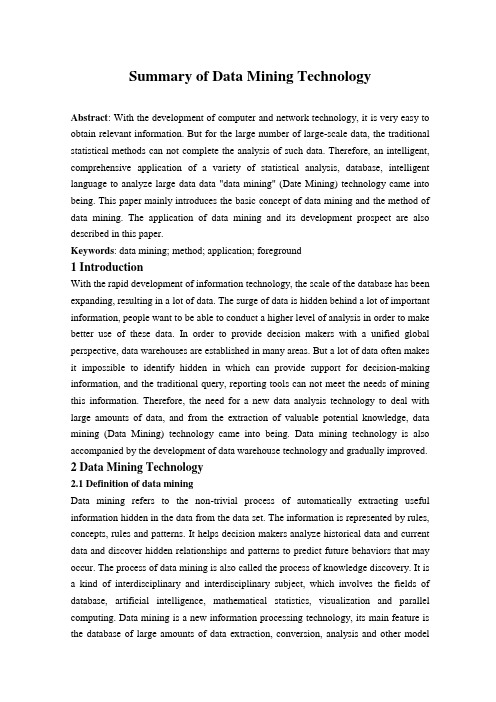
Summary of Data Mining TechnologyAbstract: With the development of computer and network technology, it is very easy to obtain relevant information. But for the large number of large-scale data, the traditional statistical methods can not complete the analysis of such data. Therefore, an intelligent, comprehensive application of a variety of statistical analysis, database, intelligent language to analyze large data data "data mining" (Date Mining) technology came into being. This paper mainly introduces the basic concept of data mining and the method of data mining. The application of data mining and its development prospect are also described in this paper.Keywords: data mining; method; application; foreground1 IntroductionWith the rapid development of information technology, the scale of the database has been expanding, resulting in a lot of data. The surge of data is hidden behind a lot of important information, people want to be able to conduct a higher level of analysis in order to make better use of these data. In order to provide decision makers with a unified global perspective, data warehouses are established in many areas. But a lot of data often makes it impossible to identify hidden in which can provide support for decision-making information, and the traditional query, reporting tools can not meet the needs of mining this information. Therefore, the need for a new data analysis technology to deal with large amounts of data, and from the extraction of valuable potential knowledge, data mining (Data Mining) technology came into being. Data mining technology is also accompanied by the development of data warehouse technology and gradually improved.2 Data Mining Technology2.1 Definition of data miningData mining refers to the non-trivial process of automatically extracting useful information hidden in the data from the data set. The information is represented by rules, concepts, rules and patterns. It helps decision makers analyze historical data and current data and discover hidden relationships and patterns to predict future behaviors that may occur. The process of data mining is also called the process of knowledge discovery. It is a kind of interdisciplinary and interdisciplinary subject, which involves the fields of database, artificial intelligence, mathematical statistics, visualization and parallel computing. Data mining is a new information processing technology, its main feature is the database of large amounts of data extraction, conversion, analysis and other modelprocessing, and extract the auxiliary decision-making key data. Data mining is an important technology in KDD (Knowledge Discovery in Database). It does not use the standard database query language (such as SQL) to query, but the content of the query to summarize the pattern and the inherent law of the search. Traditional query and report processing are only the result of the incident, and there is no in-depth study of the reasons for the occurrence of data mining is the main understanding of the causes of occurrence, and with a certain degree of confidence in the future forecast for the decision-making behavior to provide favorable stand by.2.2 Methods of data miningData mining research combines a number of different disciplines in the field of technology and results, making the current data mining methods show a variety of forms. From the perspective of statistical analysis, the data mining models used in statistical analysis techniques are linear and non-linear analysis, regression analysis, logistic regression analysis, univariate analysis, multivariate analysis, time series analysis, recent sequence analysis, and recent Oracle algorithm and clustering analysis and other methods. Using these techniques, you can examine the data in those unusual forms, and then interpret the data using various statistical models and mathematical models to explain the market rules and business opportunities that are hidden behind those data. Knowledge discovery class Data mining technology is a kind of mining technology which is completely different from the statistical analysis class data mining technology, including artificial neural network, support vector machine, decision tree, genetic algorithm, rough set, rule discovery and association order.2.2.1 Statistical methodsTraditional statistics provide a number of discriminant and regression analysis methods for data mining. Commonly used techniques such as Bayesian reasoning, regression analysis, and variance analysis. Bayesian reasoning is the basic principle of correcting the probability distribution of data sets after knowing new information Tools, to deal with the classification of data mining problems, regression analysis used to find an input variable and the relationship between the output variables of the best model, in the regression analysis used to describe a variable trends and other variables of the relationship between the linear regression, There is also a logarithmic regression for predicting the occurrence of certain events. The variance analysis in the statistical method is generally used to analyze the effects of estimating the regression line's performance and the independent variables on the final regression, which is the result of many mining applications One of the powerful tools.2.2.2 Association rulesThe association rule is a simple and practical analysis rule, which describes the law and pattern of some attributes in one thing at the same time, which is one of the most mature and important technologies in data mining. It is made by R. Agrawal et al. First proposed that the most classical association rule mining algorithm is Apriori, which first digs out all frequent itemsets, and then generates association rules from frequent itemsets. Many mining rules of frequent rule sets are It evolved from the evolution of the rules in the field of data mining is widely used in large data sets to find a meaningful relationship between the data, one of the reasons is that it is not only a choice of a dependent variable, the association rules in the data The most typical application of the mining area is the shopping basket analysis. Most association rule mining algorithms can discover all the associated relationships hidden in the mining data, and the amount of association rules is often very large. However, not all the relationships between the attributes obtained through the association are practical. Value, the effective evaluation of these association rules, screening out the user is really interested, meaningful association rules is particularly important.2.2.3 Clustering analysisCluster analysis is based on the criteria associated with the selected samples to be divided into several groups, the same group of samples with high similarity, different groups are different, commonly used techniques have split algorithm, cohesion algorithm, Clustering and incremental clustering. The clustering method is suitable for the internal relationship between the samples, so as to make a reasonable evaluation of the sample structure. In addition, the cluster analysis is also used to detect the isolated points. Sometimes clustering is not intended to get objects together but to make it easier for an object to be separated from other objects. Cluster analysis has been applied to a variety of areas such as economic analysis, pattern recognition, image processing, and especially in business. Clustering analysis can help marketers discover different groups of characteristics that exist in customer groups. The key to clustering analysis In addition to the choice of algorithms, it is the choice of metrics for the sample. The classes that are not derived from the clustering algorithm are effective for decision making. Before applying an algorithm, the clustering trend of the data is usually checked first.2.2.4 Decision tree methodDecision tree learning is a method of approximating discrete objective functions by classifying instances from a root node to a leaf node to classify an instance. The leaf node is the classification of the instance. Each node on the tree illustrates a test of anattribute of the instance, and each subsequent branch of the node corresponds to a possible value of the attribute. The method of sorting the instance is from the root node of the tree, Test the properties specified by this node, and then move down the corresponding branch of the attribute value for the given instance. Decision tree method is to be applied to the classification of data mining.2.2.5 neural networkThe neural network is based on the mathematical model of self-learning, which can analyze a large number of complex data and can complete the extremely complex pattern extraction and trend analysis for human brain or other computer. The neural network can be expressed as guidance The learning can also be a non-guided cluster, whichever is the value entered into the neural network. Artificial neural network is used to simulate the structure of human brain neurons. Based on MP model and Hebb learning rules, three kinds of neural networks are established, which have non-linear mapping characteristics, information storage, parallel processing and global collective action, High degree of self-learning, self-organizing and adaptive ability. The feedforward neural network is represented by the sensor network and BP network, which can be used for classification and prediction. The feedback network is represented by Hopfield network for associative memory and optimization. The self-organizing network is based on ART model, Kohonon The model is represented for clustering.2.2.6 support vector machineSupport vector machine (SVM) is a new machine learning method developed on the basis of statistical learning theory. It is based on the principle of structural risk minimization, as far as possible to improve the learning machine generalization ability, has good promotion performance and good classification accuracy, can effectively solve the learning problem, has become a training multi-layer sensor, RBF An Alternative Method for Neural Networks and Polynomial Neural Networks. In addition, the support vector machine algorithm is a convex optimization problem, the local optimal solution must be the global optimal solution, these features are including the neural network, including other algorithms can not and. Support vector machine can be applied to the classification of data mining, regression, the exploration of unknown things and so on. In addition to the above methods, there are ways to convert data and results into visualization techniques, cloud model methods, and inductive logic programs.In fact, any kind of excavation tool is often based on specific issues to select the appropriate mining method, it is difficult to say which method is good, that method is inferior, but depending on the specific problems.2.3 data mining processFor data mining, we can be divided into three main stages: data preparation, data mining, evaluation and expression of results. The results of the evaluation and expression can also be broken down into: assessment, interpretation model model, consolidation, the use of knowledge. Knowledge discovery in the database is a multi-step process, but also the three stages of the repeated process,2.3.1 Data PreparationKDD processing object is a lot of data, these data are generally stored in the database system, the long-term accumulation of the results. But often not suitable for direct knowledge mining on these data, need to do data preparation, generally including the choice of data (select the relevant data), clean (eliminate noise, data), speculate (estimate missing data), conversion (discrete Data conversion between data and continuous value data, packet classification of data values, calculation combinations between data items, etc.), data reduction (reduction of data volume). These jobs are often prepared when the data warehouse is generated. Data preparation is the first step in KDD. Whether data preparation is good will affect the efficiency and accuracy of data mining and the effectiveness of the final model.2.3.2 Data miningData mining is the most critical step KDD, but also technical difficulties. Most of the research KDD personnel are studying data mining technology, using more technology to have decision tree, classification, clustering, rough set, association rules, neural network, genetic algorithm and so on. Data mining According to the goal of KDD, select the parameters of the corresponding algorithm, analyze the data, and get the model model of the possible model layer knowledge.2.3.3 Results evaluation and expressionEvaluation model: the model model obtained above, there may be no practical significance or no use value, it may not be able to accurately reflect the true meaning of the data, even in some cases is contrary to the facts, so need Evaluate, determine which are valid and useful patterns. Evaluation can be based on years of experience, some models can also be used directly to test the accuracy of the data. This step also includes presenting the pattern to the user in an easy-to-understand manner.Consolidate knowledge: the user understands and is considered to be consistent with the actual and valuable model of the model that forms the knowledge. But also pay attention to the consistency of knowledge to check, with the knowledge obtained before the conflict, contradictory embankment, so that knowledge is consolidated.The use of knowledge: to find knowledge is to use, how to make knowledge can be used is one of the steps of KDD. There are two ways to use knowledge: one is to rely on the relationship or result described by the knowledge itself to support decision-making; the other is to require the use of new data knowledge, which may produce new problems, and Need to further optimize the knowledge. The process of KDD may need to be repeated multiple times. Once each step does not match the expected target, go back to the previous step, re-adjust, and re-execute.3 data mining applicationsThe potential application of data mining is very broad: government management decision-making, business management, scientific research and industrial enterprise decision support and other fields.3.1 Applied in scientific researchFrom the point of view of scientific research methodology, scientific research can be divided into three categories: theoretical science, experimental science and computational science. Computational science is an important symbol of modern science. Computing scientists work with data and analyze a wide variety of experimental or observational data every day. With the use of advanced scientific data collection tools, such as observing satellites, remote sensors, DNA molecular technology, the amount of data is very large, the traditional data analysis tools can not do anything, so there must be a strong intelligent automatic data analysis tools Caixing. Data mining in astronomy has a very famous application system: SKICAT (Sky Image Cataloging andAnalysis Tool). It is a tool developed by the California Institute of Technology's Jet Propulsion Laboratory (a laboratory designed to design a Mars probe rover) and astronomical scientists to help astronomers discover distant quasars. SKICAT is both the first successful data mining application and one of the first successful applications of artificial intelligence in astronomy and space science. Using SKICAT, astronomers have discovered 16 new and distant quasars that help astronomers better study the formation of quasars and the structure of the early universe. The application of data mining in biology is mainly focused on the study of molecular biology, especially genetic engineering. Gene research, there is a well-known international research project - the human genome project.3.2 in the commercial applicationIn the business sector, especially in the retail industry, the use of data mining is more successful. As the MIS system in the commercial use of universal, especially the use of code technology, you can collect a lot of data on the purchase situation, and the amount of data in the surge. The use of data mining technology can provide managers with theright decision-making means, so to promote sales and improve competitiveness is of great help.3.3 in the financial applicationIn the financial sector, the amount of data is very large, banks, securities companies and other transaction data and storage capacity is great. And for credit card fraud, the bank's annual loss is very large. Therefore, you can use data mining to analyze the customer's reputation. Typical financial analysis areas include investment assessment and stock trading market forecasts.3.4 in medical applicationsData mining in the medical application is very wide, from molecular medicine to medical diagnosis, can use data mining means to improve efficiency and efficiency. In the case of drug synthesis, the analysis of the chemical structure of the drug molecule can determine which of the atoms or atomic genes in the drug can play a role in the disease, so that in the synthesis of new drugs, according to the molecular structure of the drug to determine the drug will be possible What kind of disease? Data mining can also be used in industry, agriculture, transportation, telecommunications, military, Internet and other industries. Data mining has a wide range of application prospects, it can be applied to decision support, can also be applied to the database management system (DBMS). Data mining as a tool for decision support and analysis can be used to construct a knowledge base. In DBMS, data mining can be used for semantic query optimization, integrity constraints and inconsistent checks.4 Development Trend of Data MiningDue to the diversity of data, data mining tasks and data mining methods, many challenging topics are proposed for data mining. At the same time, the design of data mining language, efficient and useful data mining methods and system development, interactive and integrated data mining environment, as well as the application of data mining technology to solve large application problems, are currently data mining researchers, systems And the main problems faced by application developers. At present, the development trend of data mining is mainly as follows: application exploration; scalable data mining method; data mining and database system, data warehouse system and Web database system integration; data mining language standardization; visual data mining; Complex mining of new data types; Web mining; data mining in the privacy protection and information security.5 concluding remarksAt present, although the data mining technology has been applied to a certain degree, andachieved remarkable results, but there are still many unresolved problems, such as data preprocessing, mining algorithms, pattern recognition and interpretation, visualization problems. For the business process, the most critical issue of data mining is how to combine the spatial and temporal characteristics of business data, will be excavated out of knowledge, that is, time and space knowledge expression and interpretation mechanism. With the deepening of data mining technology, data mining technology will be applied in a wider range of areas, and achieved more significant results.Reference[1] HAN Jia-wei,KAMBER M. Data Mining Concepts and Technigues [M]. FAN Ming,MENG Xiao-feng,trrnsl. Beijing:China Ma-chine Press,2010. 305-307.(in Chinese)[2] ZHOU Bin,LIU Ya-ping,WU Ouan-yuan. The design and implementations issues of a data mining systems for eIectronic commerce[J]. Computer Engineering,2012,26 (6) :18-20.(in Chinese)[3] WANG Jia-cai,CHEN Oi,ZHAO Jie-yu,etla. VISMiner:An interactive visua I data mining prototyped system [J] . Computer Engi-neering,2003,29 (1) :17-19.(in Chinese)[4] LIU Kan,ZHOU Xiao-zheng,ZHOU Dong-ru. Visua I data mining based on para IIe I coordinates [J]. Computer Engineering and Ap-p Iications,2013,39 (5) : 193-196.(in Chinese)[5] NETZA,CHAUDHURI S,FAYYAD U,et al. Integrating data mining with SOL databases:OLE DB for data mining [A] . Pro 17th Int Conf on Data Engineering [C]. Heide Iberg:IEEE,2001. 379-387.[6] ZHAO Zhi-hong,LUO Bin,CHEN Shi-fu. A structure of data mining system based on data warehouse [J] . Computer App Iications and Software,2012,19 (4) :27-30.(in Chinese)[7] OIAN Wei-ning,WEI Li,WANG Yan,et a I. A data mining system for very Iarge databases [J]. Journa I of Software, 2012, 13 (8) :1540-1545.(in Chinese)[8] Quanyin Zhu,Jin Ding,Yonghua Yin,et al. A HybridApproach for New Products Discovery of Cell PhoneBased on Web Mining[J]. Journal of Information andComputational Science. 2012,9( 16) : 5039-5046.[9]Quanyin Zhu,Pei Zhou,Sunqun Cao,et al. A novel RDB-SW approach for commodities price dynamic trend a-nalysis based on Web extracting[J]. Journal of Digital In-formation Management,2012,10( 4) : 230-235.[10]Quanyin Zhu,Pei Zhou. The System Architecture for theBasic Information of Science and Technology ExpertsBased on Distributed Storage and Web Mining[C]. Pro-ceedings of the International Conference on ComputerScience and Service System,2012: 661-664.数据挖掘技术综述摘要:随着计算机、网络技术的发展,获得有关资料非常简单易行。
- 1、下载文档前请自行甄别文档内容的完整性,平台不提供额外的编辑、内容补充、找答案等附加服务。
- 2、"仅部分预览"的文档,不可在线预览部分如存在完整性等问题,可反馈申请退款(可完整预览的文档不适用该条件!)。
- 3、如文档侵犯您的权益,请联系客服反馈,我们会尽快为您处理(人工客服工作时间:9:00-18:30)。
Applied intelligence, 2005, 22,47-60.一种用于零售银行客户流失分析的数据挖掘方法
作者:胡晓华
作者单位:美国费城卓克索大学信息科学学院
摘要在金融服务业中解除管制,和新技术的广泛运用在金融市场上增加了竞争优势。
每一个金融服务公司的经营策略的关键是保留现有客户,和挖掘新的潜在客户。
数据挖掘技术在这些方面发挥了重要的作用。
在本文中,我们采用数据挖掘方法对零售银行客户流失进行分析。
我们讨论了具有挑战性的问题,如倾向性数据、数据按时序展开、字段遗漏检测等,以及一项零售银行损失分析数据挖掘任务的步骤。
我们使用枚举法作为损失分析的适当方法,用枚举法比较了决策树,选择条件下的贝叶斯网络,神经网络和上述分类的集成的数据挖掘模型。
一些有趣的调查结果被报道。
而我们的研究结果表明,数据挖掘技术在零售业银行中的有效性。
关键词数据挖掘分类方法损失分析
1.简介
在金融服务业中解除管制,和新技术的广泛运用在金融市场上增加了竞争优势。
每一个金融服务公司经营策略的关键是保留现有客户,和挖掘新的潜在客户。
数据挖掘技术在这些方面中发挥了重要的作用。
数据挖掘是一个结合商业知识,机器学习方法,工具和大量相关的准确信息的反复过程,使隐藏在组织中的企业数据的非直观见解被发现。
这个技术可以改善现有的进程,发现趋势和帮助制定公司的客户和员工的关系政策。
在金融领域,数据挖掘技术已成功地被应用。
•谁可能成为下两个月的流失客户?
•谁可能变成你的盈利客户?
•你的盈利客户经济行为是什么?
•什么产品的不同部分可能被购买?
•不同的群体的价值观是什么?
•不同部分的特征是什么和每个部分在个人利益中扮演的角色是什么?
在本论文中,我们关注的是应用数据挖掘技术来帮助分析零售银行损失分析。
损失分析的目的是确定一组高流失率的客户,然后公司可以控制市场活动来改变所需方向的行为(改变他们的行为,降低流失率)。
在直接营销活动的数据挖掘中,每一个目标客户是无利可图的,无效的,这个概
念很容易被理解。
因为有限的营销预算和员工,所以数据挖掘模型过去常常被用来排列客户组成,且只有一定比例的客户通过邮件,电话等联系。
如果建立更完善的数据挖掘模型和定义正确的目标,该公司便就能够接触潜在的高密度客户流失的集中群体。
下面描述了银行流失分析的数据挖掘过程的步骤:
1.商业问题的定义:在客户保留的领域中商业问题的明确说明
2.数据审查和初步筛选
3.在现有的数据方面问题的说明
4.数据集成,编目和格式化
5.数据预处理:(a)数据清洗,数据展开和定义时间敏感度的变量定义,定义目标变量,(b)统计分析,(C)敏感度分析,(d)漏泄检测,(e)特征选择
6.通过分类模型建立数据模型:决策树,神经网络,促进朴素贝叶斯网络,自然选择条件下的贝叶斯网络,分类器的集成
7.结果表达与分析:用数据挖掘模型来预测当前用户中可能的流失客户
8.调度展示:定义可能成为流失客户的对象(称为正式)
这篇论文描述了一种用来分析零售银行客户流失的数据挖掘方法。
目的是确认规则、趋向、模式和能够被作为潜在的流失指标的群体和提前确定潜在流失客户,因此银行能够采取积极主动地预防措施来降低流失指数。
本论文安排如下:首先我们在第二部分定义客户保留区域上的问题和商业问题的说明,接着我们在第三部分讨论数据选择、数据审查和初步筛选,然后是数据集成、数据目录的编辑和数据格式化、数据演变和时间敏感度变量的定义。
接着我们讨论敏感度分析、遗漏侦测和特征选择。
在第四部分我们通过决策树,神经网络和贝叶斯网络和自然选择条件下的贝叶斯网络和上述四种分类器的集成来描述数据模型。
在第五部分,我们主要讨论调查结果、字段检测结果。
最后,我们在第六部分得出结论。
2. 商业问题
2.1. 主要问题的解释
我们的客户是世界十大零售银行之一,这些银行根据不同的客户提供各种种类的金融产品。
本论文中讨论的产品属于一项特定的贷款服务。
目前超过750,000的客户正在使用这项仍有150亿美元的资金未解决的产品,这项产品已经有了显著的的高流失率。
由于高流失率,税收受到了挑战:每个月呼叫中心会受到超过4500个要求注销银行账户的电话;另外接近1200条记录属于缓慢流失(连续超过12个月以上处于不平衡状态),同时非法账户对于产品收益率构成了一系列的挑战,由于指数、贷款限额以及佣金的影响,每月零售银行的流失指数总计达到5700。
另外,很多客户
只在优惠价时才使用该产品,过期后便作废。
每一个账户都有客户管理项目成本和客户获得成本,邮递需要在每个客户上花1美元,电话营销需要在每个客户上花5美元。
而刺激成本(比如降低利率来留住客户)能够被考虑,主要取决于你提供了什么样的产品。
我们的客户没有主动性的或者反应性。
在大多数情况下,尽管有人认为价格下降并不是仅有的或者最好的策略,但是这还是一种主要的方法。
我们以上描述的情况已经使得我们客户的商务和技术部门的管理者们开始审视采取相关知识为基础通过一系列有效的客户分类、客户概况了解、数据挖掘和信用积分的结合来保留更多的客户以达到收益最大化的可能性。
在下文中,我们将描述首次使用这个计划的结果。
2.2. 问题定义
在这个部分描述了在基于现有的数据,时间周期以及目标字段如何理解和定义问题的步骤。
在此步骤上,所有数据挖掘中,最冗长和最费力的部分是数据选择、数据准备、数据结构[1, 6, 7]。
在生产线上有五种流失因素:
•缓慢流失客户:指到冻结帐户时才还款的客户。
自主性流失因素有多种行为表现而在此处可以被全面地理解。
•快速流失客户:指快速还款后立即通过电话或写信销户的客户。
•交叉销售:指的是可能购买现有贷款客户提供的诸如人生保险之类的替代产品的客户。
不断增加的联系被认为是减少客户流失的一种手段。
•高风险:可能变成高风险的客户。
•客户挖掘:可能放弃我们的产品而选择我们竞争对手产品的客户。
这种情况不是单一的个例:一个客户能够在贷款周期中显示这类情况的子集。
此时,他/她能够通过有效的被刺激手段和策略影响来改变他们的行为。
鉴于此,这些客户的态度可以被量化表现在状态图表1上。
表1表达了客户管理的优势以及预测问题。
1.确定缓慢客户流失。
2.交叉销售产品。
3.确定高风险客户。
4.确定客户可能被竞争对手挖掘。
如上图中所示,一个客户通过他的行为,能够按组别属性在每个状态被定义时,在活跃和流失之间活动。
基础上图,我们决定聚焦到两个流失问题上:(1)利用过去连续4个月所开的账户为数据,在提前60天的情况下,预测特定客户是否会自主通过电话或写信注销她/他的账户。
(2)利用过去连续4个月所开的账户为数据,在提前60天情况下,预测一个特定客户是否可能会将他的账户转移到竞争对手手上。
而账户不一定仍保持开通。
模型的发展和随后的活动焦点将会聚集到提高产品线业务及改善该项产品客户维持度和客户活跃度的问题上:
问题1:保留现有客户
为了划分不同客户层这个问题需要如下规则来制定模型:。