常见统计学错误(2013)
医学期刊论文中常见统计学错误
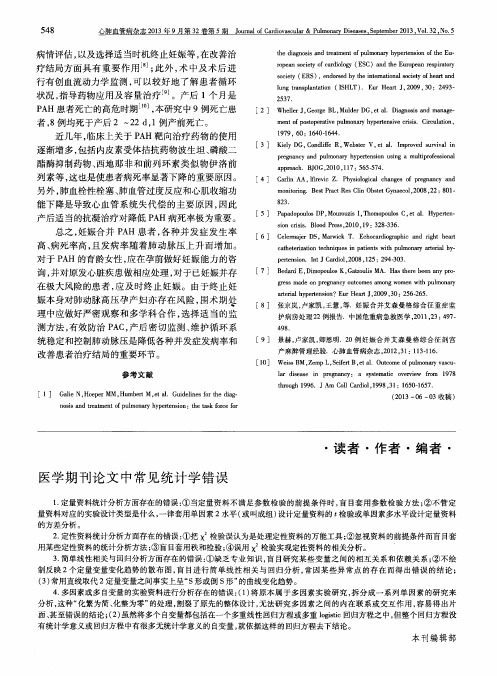
t h e d i a g n o s i s a n d t r e a t me n t o f p u l mo n a r y h y p e te r n s i o n o f t h e Eu —
me n t o f p o s t o p e r a t i v e p u l mo n a r y h y p e t r e n s i v e c is r i s .C i r c u l a t i o n,
1 9 7 9,6 0:1 6 40 — 1 6 4 4.
5 4 8
心肺血管病杂志 2 0 1 3年 9月第 3 2卷第 5期
J o u na r l o f C a r d i o v a s c u l a r&P u l mo n a r y D i s e a s e s
e r
. Biblioteka . 病情评估 , 以及选择适当时机终止妊娠等, 在改善治 疗结局方面具有重要 作用 ; 此外 , 术 中及术后进 行有 创血 流动力 学 监测 , 可 以较 好 地 了解 患 者循 环 状况 , 指导 药物应 用 及 容 量治 疗 J 。产 后 1个 月是 P A H患者死亡的高危时期 , 本研究 中9 例死亡患
l u n g t r a n s p l a n t a t i o n( I S HL T) .E u r He a r t J , 2 0 0 9 , 3 0 :2 4 9 3 —
2 5 3 7.
Wh e l l e r J, Ge o r g e BL, Mu l d e r DG, e t a 1 .Di a g n o s i s a n d ma n a g e —
医学科研中常见统计学错误(朱继民)总论
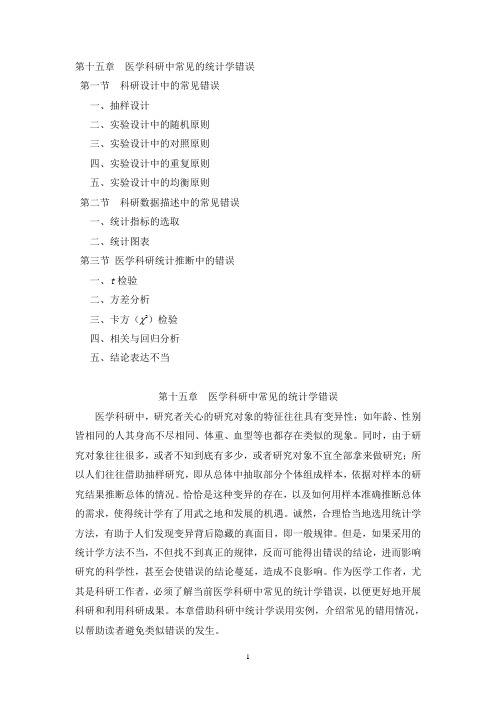
第十五章医学科研中常见的统计学错误第一节科研设计中的常见错误一、抽样设计二、实验设计中的随机原则三、实验设计中的对照原则四、实验设计中的重复原则五、实验设计中的均衡原则第二节科研数据描述中的常见错误一、统计指标的选取二、统计图表第三节医学科研统计推断中的错误一、t检验二、方差分析三、卡方( 2)检验四、相关与回归分析五、结论表达不当第十五章医学科研中常见的统计学错误医学科研中,研究者关心的研究对象的特征往往具有变异性;如年龄、性别皆相同的人其身高不尽相同、体重、血型等也都存在类似的现象。
同时,由于研究对象往往很多,或者不知到底有多少,或者研究对象不宜全部拿来做研究;所以人们往往借助抽样研究,即从总体中抽取部分个体组成样本,依据对样本的研究结果推断总体的情况。
恰恰是这种变异的存在,以及如何用样本准确推断总体的需求,使得统计学有了用武之地和发展的机遇。
诚然,合理恰当地选用统计学方法,有助于人们发现变异背后隐藏的真面目,即一般规律。
但是,如果采用的统计学方法不当,不但找不到真正的规律,反而可能得出错误的结论,进而影响研究的科学性,甚至会使错误的结论蔓延,造成不良影响。
作为医学工作者,尤其是科研工作者,必须了解当前医学科研中常见的统计学错误,以便更好地开展科研和利用科研成果。
本章借助科研中统计学误用实例,介绍常见的错用情况,以帮助读者避免类似错误的发生。
第一节科研设计中的常见错误统计学是一门重要的方法学,是一门研究数据的收集、整理和分析,从而发现变幻莫测的表面现象之后隐含的一般规律的科学。
医学科研是研究医学现象中隐含规律的科学,包括基础医学研究、临床医学研究和预防医学研究等,不管哪类医学科研都离不开统计学的支持。
要想做好医学科研,必须掌握一定的统计学知识,如总体与样本、小概率原理、资料的类型和分布、科研设计类型、统计分析的主要工作、常用统计方法以及方法的种类和应用条件等,尤其要了解当前医学科研中常见的统计学错误。
统计学中常见的错误

Chapter2What Can Go Wrong?■ Don’t label a variable as categorical or quantitative without thinkingabout the question you want it to answer. The same variable cansometimes take on different roles.■ Just because your variable’s values are numbers, don’t assume that it’s quantitative. Categories are often given numerical labels. Don’t let that fool you into thinking they have quantitative meaning. Look at thecontext.■ Always be skeptical. One reason to analyze data is to discover the truth.Even when you are told a context for the data, it may turn out that thetruth is a bit (or even a lot) different. The context colors our interpretationof the data, so those who want to influence what you think may slant thecontext. A survey that seems to be about all students mayin fact reportjust the opinions of those who visited a fan website. The question that respondentsanswered may have been posed in a way that influenced their responses.Chapter3Displaying and Summarizing Quantitative DataWhat Can Go Wrong?■ Don’t violate the area principle. This is probably the most common mistake in a graphical display. It is often made in the cause of artistic presentation.Here, for example, are two displays of the pie chart of the Titanicpassengers by clas、A’\‘GN;’{s:Crew Third ClassFirst Class Second Class First Class325Second Class285Third ClassCrew 70688550.0%31.5%26.7%UseMarijuanaUseAlcoholHeavyDrinkingThe one on the left looks pretty, doesn’t it? But showing the pie on a slantviolates the area principle and makes it much more difficult to comparefractions of the whole made up of each class—the principal feature that apie chart ought to show.■ Keep it honest. Here’s a pie chart that displays data on the percentage ofhigh school students who engage in specified dangerous behaviors as reportedby the Centers for Disease Control and Prevention. What’s wrongwith this plot?Try adding up the percentages. Or look at the 50% slice. Does it look right?Then think: What are these percentages of? Is there a “whole” that hasbeen sliced up? In a pie chart, the proportions shown by each slice of thepie must add up to 100% and each individual must fall into only one category.Of course, showing the pie on a slant makes it even harder to detectthe error.A data display should tell a story about the data. To do that, it must speak ina clear language, making plain what variable is displayed, what any axisshows, and what the values of the data are. And it must be consistent in thosedecisions.A display of quantitative data can go wrong in many ways. The most commonfailures arise from only a few basic errors:■ Don’t make a histogram of a categorical variable. Just because thevariable contains numbers doesn’t mean that it’s quantitative. Here’sa histogram of the insurance policy numbers of some workers.It’s not very informative because the policy numbers are just labels.A histogram or stem-and-leaf display of a categoricalvariable makesno sense. A bar chart or pie chart would be more appropriate.■ Don’t look for shape, center, and spread of a bar chart.A bar chart showingthe sizes of the piles displays the distribution of a categorical variable,but the bars could be arranged in any order left to right. Concepts likesymmetry, center, and spread make sense only for quantitative variables.■ Don’t use bars in every display—save them for histograms and barcharts. In a bar chart, the bars indicate how many cases of a categoricalvariable are piled in each category. Bars in a histogram indicate thenumber of cases piled in each interval of a quantitative variable. In bothbar charts and histograms, the bars represent counts of data values. Somepeople create other displays that use bars to representindividual data values.Beware: Such graphs are neither bar charts nor histograms. For example,a student was asked to make a histogram from data showing thenumber of juvenile bald eagles seen during each of the 13 weeks in thewinter of 2003–2004 at a site in Rock Island, IL. Instead, he made this plot:1 2 3 4 5 6 7的方差等于21 2 3 4 5 6的方差等于2.92。
医学期刊论文中常见统计学错误
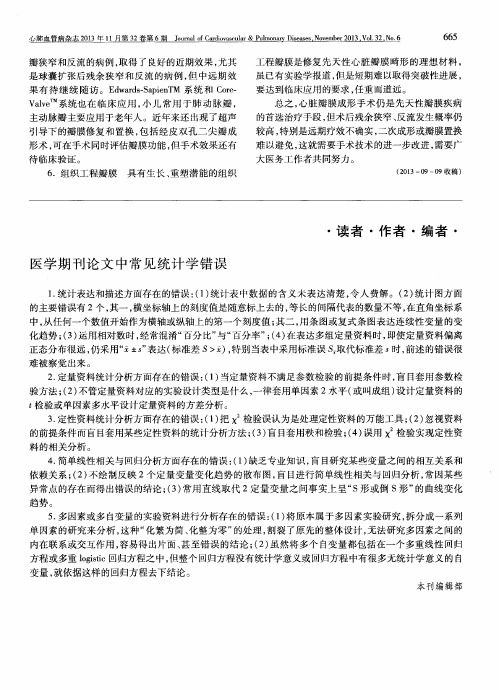
V a l v e 系统 也 在 临 床 应 用 , 小 儿 常 用 于肺 动 脉 瓣 ,
主 动脉 瓣 主要应 用于 老年人 。近年来 还 出现 了超声
总之 , 心 脏瓣 膜 成形 手术 仍是 先 天性 瓣 膜疾 病 的首选 治疗 手段 , 但术 后残余 狭 窄 、 反流 发生概 率仍 较高 , 特别 是远期 疗效 不确 实 , 二 次成形 或瓣膜 置换
肺血管病杂志 2 0 1 3 年1 1 月第 3 2卷第 6 期
J o u m a 1 o f c a r d i o v a s c u 1 a r &P u l m o n a r y D i s e a s e s , N o v b e r 2 0 1 3 , V 0 1 . 3 2 , N n . 6
t 检 验或 单 因素 多水平设 计 定量 资料 的方差 分析 。 3 . 定性 资 料统计 分析 方面存 在 的错误 : ( 1 ) 把x 检验误认 为是处 理定性 资料 的万 能 工具 ; ( 2 ) 忽视 资 料 的前 提条 件而 盲 目套 用 某些定 性 资料 的统计 分析 方法 ; ( 3 ) 盲 目套 用秩 和检验 ; ( 4 ) 误用 x 检 验 实现定 性 资 料 的相关 分析 。 4 . 简 单线 性相关 与 回归分 析 方面存 在 的错误 : ( 1 ) 缺乏专 业 知识 , 盲 目研 究某 些 变量 之 间 的相互 关 系和 依赖 关 系 ; ( 2 ) 不绘 制 反 映 2个 定 量 变量 变 化趋 势 的散 布 图 , 盲 目进行 简 单线 性相 关 与 回归分 析 , 常 因某 些 异常 点 的存在 而得 出错 误 的结论 ; ( 3 ) 常用 直 线 取代 2定量 变 量 之 间事 实上 呈 “ s形 或 倒 s形 ” 的 曲线 变 化
常见统计学错误
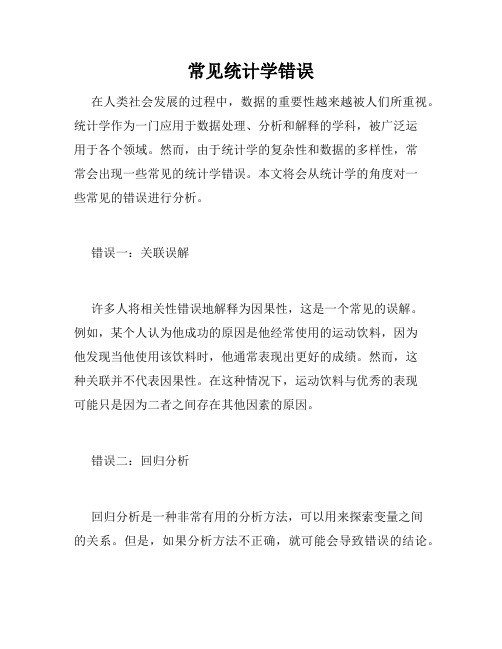
常见统计学错误在人类社会发展的过程中,数据的重要性越来越被人们所重视。
统计学作为一门应用于数据处理、分析和解释的学科,被广泛运用于各个领域。
然而,由于统计学的复杂性和数据的多样性,常常会出现一些常见的统计学错误。
本文将会从统计学的角度对一些常见的错误进行分析。
错误一:关联误解许多人将相关性错误地解释为因果性,这是一个常见的误解。
例如,某个人认为他成功的原因是他经常使用的运动饮料,因为他发现当他使用该饮料时,他通常表现出更好的成绩。
然而,这种关联并不代表因果性。
在这种情况下,运动饮料与优秀的表现可能只是因为二者之间存在其他因素的原因。
错误二:回归分析回归分析是一种非常有用的分析方法,可以用来探索变量之间的关系。
但是,如果分析方法不正确,就可能会导致错误的结论。
例如,如果回归模型中使用了错误的自变量或母体数据,甚至丢失了一些因素,那么得到的结果就可能是不准确的。
错误三:样本选择偏差样本选择偏差是指样本失去代表性,不符合总体规律的现象。
这种情况可能会导致结果的不准确,因为样本无法代表总体。
例如,在研究城市居民身体健康的研究中,如果仅仅选择某一小部分正常体型、有规律的情况,而忽略了任何超出这个范围的人,那么这个研究的结果将忽略其他身体健康状况的可能性。
错误四:误差概率统计分析必须包括在结果中发现的误差概率。
虽然有时误差会被忽略,但没考虑误差的影响会导致结果的不确定性和不准确性的增加。
例如,考虑一个零件生产厂家使用的质量控制方法。
如果该厂家仅仅进行一次样本检查,而没有考虑样本选取的偶然性,那么可能无法获得正确的结果。
错误五:推断推断通常用于从一个样本中推广一个总体结论。
但是,如果样本不够大或者不够代表性,那么结果就不能代表总体。
例如,在某一工厂中,如果只从少数员工中调查了病假的问题,那么结果可能并不具有代表性,不能推广到整个员工群体。
总之,正确的统计分析至关重要,结果的准确性直接影响到实际应用的结果。
因此,在进行统计分析时,务必要注意常见的统计学错误,避免这些错误并提高数据分析和结论推断的准确性。
统计学判断题
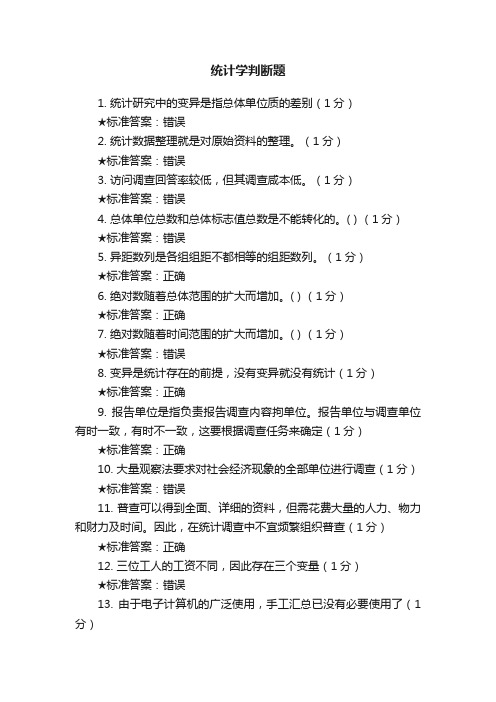
统计学判断题1. 统计研究中的变异是指总体单位质的差别(1分)★标准答案:错误2. 统计数据整理就是对原始资料的整理。
(1分)★标准答案:错误3. 访问调查回答率较低,但其调查咸本低。
(1分)★标准答案:错误4. 总体单位总数和总体标志值总数是不能转化的。
( ) (1分)★标准答案:错误5. 异距数列是各组组距不都相等的组距数列。
(1分)★标准答案:正确6. 绝对数随着总体范围的扩大而增加。
( ) (1分)★标准答案:正确7. 绝对数随着时间范围的扩大而增加。
( ) (1分)★标准答案:错误8. 变异是统计存在的前提,没有变异就没有统计(1分)★标准答案:正确9. 报告单位是指负责报告调查内容拘单位。
报告单位与调查单位有时一致,有时不一致,这要根据调查任务来确定(1分)★标准答案:正确10. 大量观察法要求对社会经济现象的全部单位进行调查(1分)★标准答案:错误11. 普查可以得到全面、详细的资料,但需花费大量的人力、物力和财力及时间。
因此,在统计调查中不宜频繁组织普查(1分)★标准答案:正确12. 三位工人的工资不同,因此存在三个变量(1分)★标准答案:错误13. 由于电子计算机的广泛使用,手工汇总已没有必要使用了(1分)14. 统计表是表达统计数据整理结果的唯一形式。
(1分)★标准答案:错误15. 统计分组的关键是正确选择分组标志和划分各组的界限。
(1分)★标准答案:正确16. 调查时间是指调查工作所需的时间(1分)★标准答案:错误17. 总体单位是标志的承担者,标志是依附于总体单位的(1分)★标准答案:正确18. 统计数据的效度和信度的含义是一致的。
(1分)★标准答案:错误19. 反映总体内部构成特征的指标只能是结构相对数。
( ) (1分)★标准答案:错误20. 年代都是以数字表示的,所以按年代排列各种指标属于按数量标志分组。
(1分)★标准答案:错误21. 综合为统计指标的前提是总体的同质性(1分)★标准答案:正确22. 统计表的主词是说明总体的各种指标。
统计学知识(一类错误和二类错误)
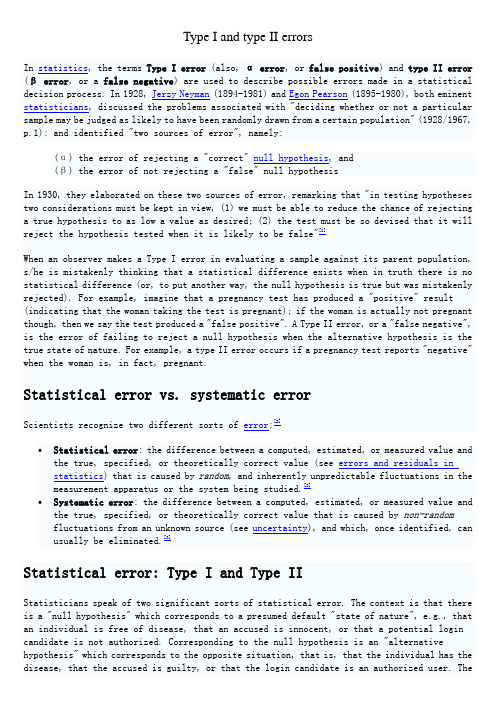
Type I and type II errors(α) the error of rejecting a "correct" null hypothesis, and(β) the error of not rejecting a "false" null hypothesisIn 1930, they elaborated on these two sources of error, remarking that "in testing hypotheses two considerations must be kept in view, (1) we must be able to reduce the chance of rejecting a true hypothesis to as low a value as desired; (2) the test must be so devised that it will reject the hypothesis tested when it is likely to be false"[1]When an observer makes a Type I error in evaluating a sample against its parent population, s/he is mistakenly thinking that a statistical difference exists when in truth there is no statistical difference (or, to put another way, the null hypothesis is true but was mistakenly rejected). For example, imagine that a pregnancy test has produced a "positive" result (indicating that the woman taking the test is pregnant); if the woman is actually not pregnant though, then we say the test produced a "false positive". A Type II error, or a "false negative", is the error of failing to reject a null hypothesis when the alternative hypothesis is the true state of nature. For example, a type II error occurs if a pregnancy test reports "negative" when the woman is, in fact, pregnant.Statistical error vs. systematic errorScientists recognize two different sorts of error:[2]Statistical error: Type I and Type IIStatisticians speak of two significant sorts of statistical error. The context is that there is a "null hypothesis" which corresponds to a presumed default "state of nature", e.g., that an individual is free of disease, that an accused is innocent, or that a potential login candidate is not authorized. Corresponding to the null hypothesis is an "alternative hypothesis" which corresponds to the opposite situation, that is, that the individual has the disease, that the accused is guilty, or that the login candidate is an authorized user. Thegoal is to determine accurately if the null hypothesis can be discarded in favor of the alternative. A test of some sort is conducted (a blood test, a legal trial, a login attempt), and data is obtained. The result of the test may be negative (that is, it does not indicate disease, guilt, or authorized identity). On the other hand, it may be positive (that is, it may indicate disease, guilt, or identity). If the result of the test does not correspond with the actual state of nature, then an error has occurred, but if the result of the test corresponds with the actual state of nature, then a correct decision has been made. There are two kinds of error, classified as "Type I error" and "Type II error," depending upon which hypothesis has incorrectly been identified as the true state of nature.Type I errorType I error, also known as an "error of the first kind", an α error, or a "false positive": the error of rejecting a null hypothesis when it is actually true. Plainly speaking, it occurs when we are observing a difference when in truth there is none. Type I error can be viewed as the error of excessive skepticism.Type II errorType II error, also known as an "error of the second kind", a βerror, or a "false negative": the error of failing to reject a null hypothesis when it is in fact false. In other words, this is the error of failing to observe a difference when in truth there is one. Type II error can be viewed as the error of excessive gullibility.See Various proposals for further extension, below, for additional terminology.Understanding Type I and Type II errorsHypothesis testing is the art of testing whether a variation between two sample distributions can be explained by chance or not. In many practical applications Type I errors are more delicate than Type II errors. In these cases, care is usually focused on minimizing the occurrence of this statistical error. Suppose, the probability for a Type I error is 1% or 5%, then there is a 1% or 5% chance that the observed variation is not true. This is called the level of significance. While 1% or 5% might be an acceptable level of significance for one application, a different application can require a very different level. For example, the standard goal of six sigma is to achieve exactness by 4.5 standard deviations above or below the mean. That is, for a normally distributed process only 3.4 parts per million are allowed to be deficient. The probability of Type I error is generally denoted with the Greek letter alpha.In more common parlance, a Type I error can usually be interpreted as a false alarm, insufficient specificity or perhaps an encounter with fool's gold. A Type II error could be similarly interpreted as an oversight, a lapse in attention or inadequate sensitivity.EtymologyIn 1928, Jerzy Neyman (1894-1981) and Egon Pearson (1895-1980), both eminent statisticians, discussed the problems associated with "deciding whether or not a particular sample may bejudged as likely to have been randomly drawn from a certain population" (1928/1967, p.1): and, as Florence Nightingale David remarked, "it is necessary to remember the adjective ‘random’ [in the term ‘random sample’] should apply t o the method of drawing the sample and not to the sample itself" (1949, p.28).They identified "two sources of error", namely:(a) the error of rejecting a hypothesis that should have been accepted, and(b) the error of accepting a hypothesis that should have been rejected (1928/1967, p.31). In 1930, they elaborated on these two sources of error, remarking that:…in testing hypotheses two considerations must be kept in view, (1) we must be able to reduce the chance of rejecting a true hypothesis to as low a value as desired; (2) the test must be so devised that it will reject the hypothesis tested when it is likely to be false (1930/1967, p.100).In 1933, they observed that these "problems are rarely presented in such a form that we can discriminate with certainty between the true and false hypothesis" (p.187). They also noted that, in deciding whether to accept or reject a particular hypothesis amongst a "set of alternative hypotheses" (p.201), it was easy to make an error:…[and] these errors will be of two kinds:(I) we reject H[i.e., the hypothesis to be tested] when it is true,(II) we accept H0when some alternative hypothesis Hiis true. (1933/1967, p.187)In all of the papers co-written by Neyman and Pearson the expression Halways signifies "the hypothesis to be tested" (see, for example, 1933/1967, p.186).In the same paper[4] they call these two sources of error, errors of type I and errors of type II respectively.[5]Statistical treatmentDefinitionsType I and type II errorsOver time, the notion of these two sources of error has been universally accepted. They are now routinely known as type I errors and type II errors. For obvious reasons, they are very often referred to as false positives and false negatives respectively. The terms are now commonly applied in much wider and far more general sense than Neyman and Pearson's original specific usage, as follows:Type I errors (the "false positive"): the error of rejecting the null hypothesis given that it is actually true; e.g., A court finding a person guilty of a crime that they did not actually commit.Type II errors(the "false negative"): the error of failing to reject the null hypothesis given that the alternative hypothesis is actually true; e.g., A court finding a person not guilty of a crime that they did actually commit.These examples illustrate the ambiguity, which is one of the dangers of this wider use: They assume the speaker is testing for guilt; they could also be used in reverse, as testing for innocence; or two tests could be involved, one for guilt, the other for innocence. (This ambiguity is one reason for the Scottish legal system's third possible verdict: not proven.)The following tables illustrate the conditions.Example, using infectious disease test results:Example, testing for guilty/not-guilty:Example, testing for innocent/not innocent – sense is reversed from previous example:Note that, when referring to test results, the terms true and false are used in two different ways: the state of the actual condition (true=present versus false=absent); and the accuracy or inaccuracy of the test result (true positive, false positive, true negative, false negative). This is confusing to some readers. To clarify the examples above, we have used present/absent rather than true/false to refer to the actual condition being tested.False positive rateThe false positive rate is the proportion of negative instances that were erroneously reported as being positive.It is equal to 1 minus the specificity of the test. This is equivalent to saying the false positive rate is equal to the significance level.[6]It is standard practice for statisticians to conduct tests in order to determine whether or not a "speculative hypothesis" concerning the observed phenomena of the world (or its inhabitants) can be supported. The results of such testing determine whether a particular set of results agrees reasonably (or does not agree) with the speculated hypothesis.On the basis that it is always assumed, by statistical convention, that the speculated hypothesis is wrong, and the so-called "null hypothesis" that the observed phenomena simply occur by chance (and that, as a consequence, the speculated agent has no effect) — the test will determine whether this hypothesis is right or wrong. This is why the hypothesis under test is often called the null hypothesis (most likely, coined by Fisher (1935, p.19)), because it is this hypothesis that is to be either nullified or not nullified by the test. When the null hypothesis is nullified, it is possible to conclude that data support the "alternative hypothesis" (which is the original speculated one).The consistent application by statisticians of Neyman and Pearson's convention of representing "the hypothesis to be tested" (or "the hypothesis to be nullified") with the expression H0has led to circumstances where many understand the term "the null hypothesis" as meaning "the nil hypothesis" — a statement that the results in question have arisen through chance. This is not necessarily the case — the key restriction, as per Fisher (1966), is that "the null hypothesis must be exact, that is free from vagueness and ambiguity, because it must supply the basis of the 'problem of distribution,' of which the test of significance is the solution."[9] As a consequence of this, in experimental science the null hypothesis is generally a statement that a particular treatment has no effect; in observational science, it is that there is nodifference between the value of a particular measured variable, and that of an experimental prediction.The extent to which the test in question shows that the "speculated hypothesis" has (or has not) been nullified is called its significance level; and the higher the significance level, the less likely it is that the phenomena in question could have been produced by chance alone. British statistician Sir Ronald Aylmer Fisher(1890–1962) stressed that the "null hypothesis":…is never proved or established, but is possibly disproved, in the course ofexperimentation. Every experiment may be said to exist only in order to give the factsa chance of disproving the null hypothesis. (1935, p.19)Bayes's theoremThe probability that an observed positive result is a false positive (as contrasted with an observed positive result being a true positive) may be calculated using Bayes's theorem.The key concept of Bayes's theorem is that the true rates of false positives and false negatives are not a function of the accuracy of the test alone, but also the actual rate or frequency of occurrence within the test population; and, often, the more powerful issue is the actual rates of the condition within the sample being tested.Various proposals for further extensionSince the paired notions of Type I errors(or "false positives") and Type II errors(or "false negatives") that were introduced by Neyman and Pearson are now widely used, their choice of terminology ("errors of the first kind" and "errors of the second kind"), has led others to suppose that certain sorts of mistake that they have identified might be an "error of the third kind", "fourth kind", etc.[10]None of these proposed categories have met with any sort of wide acceptance. The following is a brief account of some of these proposals.DavidFlorence Nightingale David (1909-1993),[3] a sometime colleague of both Neyman and Pearson at the University College London, making a humorous aside at the end of her 1947 paper, suggested that, in the case of her own research, perhaps Neyman and Pearson's "two sources of error" could be extended to a third:I have been concerned here with trying to explain what I believe to be the basic ideas[of my "theory of the conditional power functions"], and to forestall possible criticism that I am falling into error (of the third kind) and am choosing the test falsely to suit the significance of the sample. (1947), p.339)MostellerIn 1948, Frederick Mosteller (1916-2006)[11] argued that a "third kind of error" was required to describe circumstances he had observed, namely:∙Type I error: "rejecting the null hypothesis when it is true".∙Type II error: "accepting the null hypothesis when it is false".∙Type III error: "correctly rejecting the null hypothesis for the wrong reason". (1948, p.61)KaiserIn his 1966 paper, Henry F. Kaiser (1927-1992) extended Mosteller's classification such that an error of the third kind entailed an incorrect decision of direction following a rejected two-tailed test of hypothesis. In his discussion (1966, pp.162-163), Kaiser also speaks of α errors, β errors, and γ errors for type I, type II and type III errors respectively.KimballIn 1957, Allyn W. Kimball, a statistician with the Oak Ridge National Laboratory, proposed a different kind of error to stand beside "the first and second types of error in the theory of testing hypotheses". Kimball defined this new "error of the third kind" as being "the error committed by giving the right answer to the wrong problem" (1957, p.134).Mathematician Richard Hamming (1915-1998) expressed his view that "It is better to solve the right problem the wrong way than to solve the wrong problem the right way".The famous Harvard economist Howard Raiffa describes an occasion when he, too, "fell into the trap of working on the wrong problem" (1968, pp.264-265).[12]Mitroff and FeatheringhamIn 1974, Ian Mitroff and Tom Featheringham extended Kimball's category, arguing that "one of the most important determinants of a problem's solution is how that problem has been represented or formulated in the first place".They defined type III errors as either "the error… of having solved the wrong problem… when one should have solved the right problem" or "the error… [of] choosing the wrong problem representation… when one should have… chosen the right problem representation" (1974), p.383).RaiffaIn 1969, the Harvard economist Howard Raiffa jokingly suggested "a candidate for the error of the fourth kind: solving the right problem too late" (1968, p.264).Marascuilo and LevinIn 1970, Marascuilo and Levin proposed a "fourth kind of error" -- a "Type IV error" -- which they defined in a Mosteller-like manner as being the mistake of "the incorrect interpretation of a correctly rejected hypothesis"; which, they suggested, was the equivalent of "a physician's correct diagnosis of an ailment followed by the prescription of a wrong medicine" (1970, p.398).Usage examplesStatistical tests always involve a trade-off between:(a) the acceptable level of false positives (in which a non-match is declared to be amatch) and(b) the acceptable level of false negatives (in which an actual match is not detected).A threshold value can be varied to make the test more restrictive or more sensitive; with the more restrictive tests increasing the risk of rejecting true positives, and the more sensitive tests increasing the risk of accepting false positives.ComputersThe notions of "false positives" and "false negatives" have a wide currency in the realm of computers and computer applications.Computer securitySecurity vulnerabilities are an important consideration in the task of keeping all computer data safe, while maintaining access to that data for appropriate users (see computer security, computer insecurity). Moulton (1983), stresses the importance of:∙avoiding the type I errors (or false positive) that classify authorized users as imposters.∙avoiding the type II errors (or false negatives) that classify imposters as authorized users (1983, p.125).False Positive (type I) -- False Accept Rate (FAR) or False Match Rate (FMR)False Negative (type II) -- False Reject Rate (FRR) or False Non-match Rate (FNMR)The FAR may also be an abbreviation for the false alarm rate, depending on whether the biometric system is designed to allow access or to recognize suspects. The FAR is considered to be a measure of the security of the system, while the FRR measures the inconvenience level for users. For many systems, the FRR is largely caused by low quality images, due to incorrect positioning or illumination. The terminology FMR/FNMR is sometimes preferred to FAR/FRR because the former measure the rates for each biometric comparison, while the latter measure the application performance (ie. three tries may be permitted).Several limitations should be noted for the use of these measures for biometric systems:(a) The system performance depends dramatically on the composition of the test database(b) The system performance measured in this way is the zero-effort error rate. Attackersprepared to use active techniques such as spoofing will decrease FAR.(c) Such error rates only apply properly to biometric verification (or one-to-onematching)systems. The performance of biometric identification or watch-list systems is measured with other indices (such as the cumulative match curve (CMC))∙Screening involves relatively cheap tests that are given to large populations, none of whom manifest any clinical indication of disease (e.g., Pap smears).∙Testing involves far more expensive, often invasive, procedures that are given only to those who manifest some clinical indication of disease, and are most often applied to confirm a suspected diagnosis.test a population with a true occurrence rate of 70%, many of the "negatives" detected by the test will be false. (See Bayes' theorem)False positives can also produce serious and counter-intuitive problems when the condition being searched for is rare, as in screening. If a test has a false positive rate of one in ten thousand, but only one in a million samples (or people) is a true positive, most of the "positives" detected by that test will be false.[17]Paranormal investigationThe notion of a false positive has been adopted by those who investigate paranormal or ghost phenomena to describe a photograph, or recording, or some other evidence that incorrectly appears to have a paranormal origin -- in this usage, a false positive is a disproven piece of media "evidence" (image, movie, audio recording, etc.) that has a normal explanation.[18]。
统计分析中常见的错误与注意事项
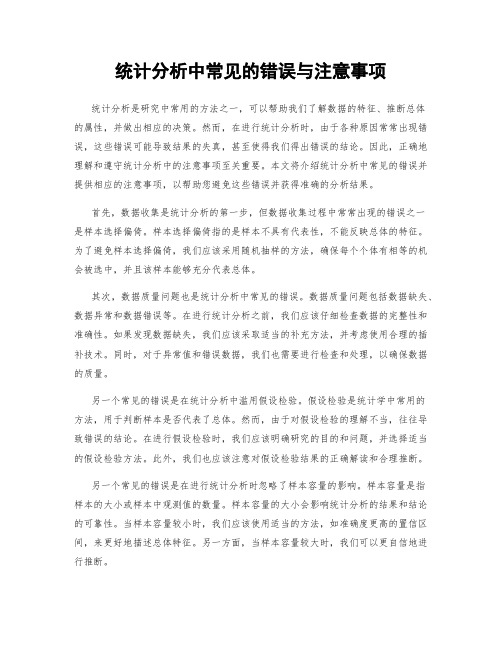
统计分析中常见的错误与注意事项统计分析是研究中常用的方法之一,可以帮助我们了解数据的特征、推断总体的属性,并做出相应的决策。
然而,在进行统计分析时,由于各种原因常常出现错误,这些错误可能导致结果的失真,甚至使得我们得出错误的结论。
因此,正确地理解和遵守统计分析中的注意事项至关重要。
本文将介绍统计分析中常见的错误并提供相应的注意事项,以帮助您避免这些错误并获得准确的分析结果。
首先,数据收集是统计分析的第一步,但数据收集过程中常常出现的错误之一是样本选择偏倚。
样本选择偏倚指的是样本不具有代表性,不能反映总体的特征。
为了避免样本选择偏倚,我们应该采用随机抽样的方法,确保每个个体有相等的机会被选中,并且该样本能够充分代表总体。
其次,数据质量问题也是统计分析中常见的错误。
数据质量问题包括数据缺失、数据异常和数据错误等。
在进行统计分析之前,我们应该仔细检查数据的完整性和准确性。
如果发现数据缺失,我们应该采取适当的补充方法,并考虑使用合理的插补技术。
同时,对于异常值和错误数据,我们也需要进行检查和处理,以确保数据的质量。
另一个常见的错误是在统计分析中滥用假设检验。
假设检验是统计学中常用的方法,用于判断样本是否代表了总体。
然而,由于对假设检验的理解不当,往往导致错误的结论。
在进行假设检验时,我们应该明确研究的目的和问题,并选择适当的假设检验方法。
此外,我们也应该注意对假设检验结果的正确解读和合理推断。
另一个常见的错误是在进行统计分析时忽略了样本容量的影响。
样本容量是指样本的大小或样本中观测值的数量。
样本容量的大小会影响统计分析的结果和结论的可靠性。
当样本容量较小时,我们应该使用适当的方法,如准确度更高的置信区间,来更好地描述总体特征。
另一方面,当样本容量较大时,我们可以更自信地进行推断。
此外,我们在进行统计分析时还需要注意多重比较的问题。
多重比较指的是对多个假设进行多次比较,从而增加发生错误的概率。
为了避免多重比较问题,我们可以使用适当的校正方法,如Bonferroni校正,来控制错误的发生。
- 1、下载文档前请自行甄别文档内容的完整性,平台不提供额外的编辑、内容补充、找答案等附加服务。
- 2、"仅部分预览"的文档,不可在线预览部分如存在完整性等问题,可反馈申请退款(可完整预览的文档不适用该条件!)。
- 3、如文档侵犯您的权益,请联系客服反馈,我们会尽快为您处理(人工客服工作时间:9:00-18:30)。
6.5
胆固醇(mg%)的对数
6.0 5.5 5.0 4.5 4.0 3.5 实验前
血药浓度(μ mol/L)
处理组 对照组
180 150 120 90 60 30
旧剂型 新剂型
5周后
10周后
0 图22.2
4
8
图22.1 两组家兔血清胆固醇的对数随时间的变化
回归方程: Y=61.786 - 6.886 log(剂量) 决定系数: R2=0.914。
) 数 均 ( 率 菌 噬
对 数 剂 量
为什麽不对? 均数做因变量造成“好”的假象 ! * 回归方程是否有统计学意义与反应的变异状况有关 * 以诸个体反应值的均数作回归计算, 掩盖变异性
正确作法:用个体资料作回归分析
家兔号 1 2 3 4 5 6 7 处理组 实验前 0.744741 0.904141 0.357641 1.077741 0.584441 0.985041 1.050841 5周后 2.013341 2.054141 1.137841 1.948741 1.668441 1.926241 1.638641 10周后 2.621341 1.628441 2.196741 2.239241 0.985041 2.915641 1.225541 家兔号 8 9 10 11 12 13 14 对照组 实验前 0.375741 0.994741 0.598841 0.719741 0.157041 0.861241 0.872141 5周后 0.667841 0.584441 0.955541 1.354241 0.246141 0.882941 0.555041 10周后 0.569941 0.461241 0.598841 1.032441 0.613041 0.757041 0.540041
错在哪里?
哪些指标可能有组间差异,必须心中有数。 科研的结果应当预见 —— 假说是科研的灵 魂 心中无数,不要“先上马再说” 指标多,实验工作量大。 大海捞针—— 碰运气,不是科研 指标多,翻来覆去分析,制造假阳性 Nature杂志统计学指南:常见错误之一
为何翻来覆去分析,会制造假阳性?
常见统计学错误与纠正
---- 设计与分析
方积乾
中山大学公共卫生学院 医学统计与流行病学系
2013年12月
1. 终点指标过多, 大海捞针
临床试验时,不知道哪个指标在组与组间有差 异; “确定某个指标后,万一组间没有差异,岂 不被动!” 生理、生化、组织学、基因,都做; “内容丰富,显得水平高!” 许多仪器一下子可以做许多项目; “许多项目一一分析,哪个有意义,就报告 哪个指标标”
90
80
噬 菌 率 ( 原
70
60
50
40
30
始 数 据
20 -.5 0.0 .5 1.0 1.5 2.0 2.5
对数剂量
回归方程: Y = 61.782-6.884 log(剂量) 决定系数: R2=0.095 回归方程无统计学意义,无剂量-反应关系!
6. 重复测量资料不能时点间两两比较
例 各取7只兔子,分别以正常食物和待研究食物喂 养,在实验前、喂养5周、10周后,各取血测量其中 胆固醇浓度,自然对数转换后, 数据见表22.1, 问血清 胆固醇浓度随时间变化的趋势是否受该食物影响。
2
2
2
5.剂量-反应关系 不能作均数比较或回归
例 有人分析蛇毒因子(CVF)的剂量对血液白细 胞噬菌率的影响,得如下数据,欲讨论剂量-反应 关系。
组数 1 2 3 4 5 6 CVF 剂量 0 10 20 40 80 160 例数 5 5 5 5 5 5 噬菌率(均数) 60.0±17.0 57.0±15.2 54.0±16.6 51.0±17.2 48.0±16.0 45.0±16.4
Nature常见错误之一
多重比较: 对一组数据作多项比较时,必须 说明如何校正α 水平,以避免增大第一类错 误的机会
应当如何?
主要终点(primary end point) :只能一个 次要终点(secondary end point) : 可以几个, 但勿过多 Bonfferoni 校正 当同一组数据同时作k次分析时,若限定 犯假阳性错误的概率总共不超过 , 则每次分析要用 / k 来控制假阳性的概率。 例
做法 1:单因素方差分析?!
F=0.701,P>0.5, 均数间差别无统计学意义
为什麽不对?
有负初衷 —— 探讨反应随剂量变化的趋势 * 由多个剂量组的比较只能得知均数间是否有差异 * 有统计学差异也不等于有剂量-反应关系
做法 2: 反应的均数关于剂量作回归分析 ?!
62 60 58 56 54 52 50 48 46 44 -. 5 0. 0 .5 1. 0 1. 5 2. 0 2. 5
参加者的流程图 (强烈推荐)
合格对象82例
拒绝参与7例
随机分组75例 分配至实验组38例 接受干预38例
随访例数: 7 周 n=38 , 11 周 n=38 , 15 周 n=38 , 19 周n=36 分配至对照组37例。接受 干预36例,1例因颈部损伤未 接受干预
随访例数:7周n=37,11 周 n=36 , 15 周 n=36 , 19 周n=35
仅分析一个指标时, P(假阳性) 0.05, P(一次分析不犯错误) 0.95 同时分析 2 个指标时, 2 P(两次分析均不犯错误) [ P(两次分析均不犯错误) ]
P(假阳性) 1 - 0.952 1 0.90 同时分析 3 个指标时, P(假阳性) 1- 0.953 1 0.86 0.14 同时分析 10 个指标时, 10 P(假阳性) 1 - 0.95 1 0.60 0.40
2
2 1.96 0.14(1 0.14) 0.84 2 0.20(1 0.20) 2 0.08(1 0.08) 0 . 20 0 . 08 2 1.96 0.14(1 0.14) 0.84 2 0.20(1 0.20) 2 0.08(1 0.08) 0 . 20 0 . 08 1.3602 0.5742 259.85 0.12
例 某药物有新、旧两种剂型。为比较两种剂型的 代谢情况,对16例某病患者服药后0、4、8、12小 时的血药浓度作了测量,问该药新旧两种剂型的 血药浓度-时间曲线的差别是否具有统计学意义。
表 5 4 个时点的某药新旧剂型血药浓度( m o l / L) 编 号 1 2 3 4 5 6 7 0 小时 90.53 88.43 100.01 46.32 73.69 105.27 86.32 旧剂型 4 小时 142.12 163.17 144.75 126.33 138.96 126.33 121.06 8 小时 65.54 48.95 86.06 48.95 70.02 75.01 78.95 12 小时 73.28 71.77 80.01 39.54 60.89 83.66 70.24 编 号 8 9 10 11 12 13 14 15 16 新剂型 0 小时 70.53 68.43 57.37 105.80 80.01 56.32 53.69 85.27 66.32 4 小时 97.38 95.27 78.43 120.54 104.75 75.27 110.02 110.01 115.27 8 小时 112.12 133.17 83.16 136.33 114.75 96.33 138.96 126.33 129.06 12 小时 58.50 56.90 48.34 84.03 65.61 47.52 45.44 69.47 55.29
欧洲研究的样本量估算
( Z / 2 Z ) N 4
2
(1.96 0.84) 0.35 4 0.18
2
(1.96 0.84) 0.35 4 118.6 0.18
2
决定每组含61名患者。
比较两组发生某结局的百分比
处理分配的随机化为什么这么重要? (1) 消除分配处理有意或无意的偏倚。 (2) 为实施盲法创造条件。 (3) 使有可能利用概率论来描述各干预组之间 的差异有多大可能仅仅是由偶然性造成的。 将随机化当作“廉价名词”,实际没做,却 写“随机分成两组” —— 科研道德?
说错和做错
将随机化当作“廉价名词”,实际没做,却 写“随机分成两组” —— 科研道德? 将“随意分组”当作随机化 将“机械分组”当作随机化 略去筛选过程,简单地报告将多少人随机分 组 略去实施过程中丢失对象,将最后两组人数 说成是随机分组人数
应当如何?
成功的随机化取决ation concealment )这个 序列,直到分配完毕(必须建立一个分配处 理的系统) 。 报告如何随机分组,如何“隐蔽” :谁做随 机序列,谁收病人,谁分药和发药;分组方 案如何保管……
(1)预计两组发生某结局的百分比约为 (2)允许犯假阳性错误的机会 (3)允许犯假阴性错误的机会
1, 2
c
1 2
2
2
2Z / 2 c (1 c ) Z 21 (1 1 ) 2 2 (1 2 ) N 1 2
南韩对比剂研究
南韩研究
(1)预计两组发生某结局的百分比约为 20%和 8% (2)允许犯假阳性错误的机会 5% (3)允许犯假阴性错误的机会 1 80% 20% 可能会有一部分患者失访、数据不全、违反研究方案, 计划每组 150 名
南韩研究的样本量估算
2 Z / 2 c (1 c ) Z 2 1 (1 1 ) 2 2 (1 2 ) N 1 2
0.05, k 10,
/ k 0.005