统计学课件--概率论
合集下载
概率论与数理统计课件ppt

简化数据结构,解释变量间的关系。
操作步骤
计算相关系数矩阵、求特征值和特征 向量、确定主成分个数。
实例
分析消费者对不同品牌手机的偏好。
聚类分析
聚类分析
常见方法
目的
实例
将类似的对象归为同一 组,即“簇”,不同簇
的对象尽可能不同。
层次聚类、K均值聚类、 DBSCAN等。
揭示数据的内在结构, 用于分类、猜测和决策
用数学符号表示一个随机实验的结果 。
随机变量可以取到任何实数值,且取 每个结果的概率为一个确定的函数。
离散型随机变量
随机变量可以取到所有可能的结果, 且取每个结果的概率为一个确定的数 。
随机变量的函数变换
线性变换
对于随机变量X和常数a、b,有 aX+b的散布与X的散布不同。
非线性变换
对于随机变量X和函数g(x),g(X)的散 布与X的散布不同。
置信区间
根据样本数据对总体参数进行估计的一个范围,表示我们对 估计的可靠程度。
假设检验与置信水平
假设检验
通过样本数据对总体参数或散布进行 假设,然后根据检验结果判断假设是 否成立。
置信水平
假设检验中,我们相信结论正确的概 率,通常表示为百分比。
05 数理统计的应用
方差分析
方差分析(ANOVA)
随机进程在通讯、气象、物理等领域有广泛应用。
马尔科夫链蒙特卡洛方法
01
马尔科夫链蒙特卡洛方法是一种 基于蒙特卡洛模拟的统计推断方 法,通过构造一个马尔科夫链来 到达近似求解复杂问题的目的。
02
马尔科夫链蒙特卡洛方法在许多 领域都有应用,如物理学、化学 、经济学等。
04 数理统计基础
样本与样本空间
操作步骤
计算相关系数矩阵、求特征值和特征 向量、确定主成分个数。
实例
分析消费者对不同品牌手机的偏好。
聚类分析
聚类分析
常见方法
目的
实例
将类似的对象归为同一 组,即“簇”,不同簇
的对象尽可能不同。
层次聚类、K均值聚类、 DBSCAN等。
揭示数据的内在结构, 用于分类、猜测和决策
用数学符号表示一个随机实验的结果 。
随机变量可以取到任何实数值,且取 每个结果的概率为一个确定的函数。
离散型随机变量
随机变量可以取到所有可能的结果, 且取每个结果的概率为一个确定的数 。
随机变量的函数变换
线性变换
对于随机变量X和常数a、b,有 aX+b的散布与X的散布不同。
非线性变换
对于随机变量X和函数g(x),g(X)的散 布与X的散布不同。
置信区间
根据样本数据对总体参数进行估计的一个范围,表示我们对 估计的可靠程度。
假设检验与置信水平
假设检验
通过样本数据对总体参数或散布进行 假设,然后根据检验结果判断假设是 否成立。
置信水平
假设检验中,我们相信结论正确的概 率,通常表示为百分比。
05 数理统计的应用
方差分析
方差分析(ANOVA)
随机进程在通讯、气象、物理等领域有广泛应用。
马尔科夫链蒙特卡洛方法
01
马尔科夫链蒙特卡洛方法是一种 基于蒙特卡洛模拟的统计推断方 法,通过构造一个马尔科夫链来 到达近似求解复杂问题的目的。
02
马尔科夫链蒙特卡洛方法在许多 领域都有应用,如物理学、化学 、经济学等。
04 数理统计基础
样本与样本空间
概率论与数理统计ppt课件

04
理解基本概念和原理
做大量练习题,培养解题能力
05
06
阅读相关书籍和论文,拓宽知识面
02
概率论基础
概率的基本概念
试验
一个具有有限个或无限个 可能结果的随机试验。
事件
试验中的某些结果的总称 。
概率
衡量事件发生可能性的数 值,通常表示为0到1之间 的实数。
必然事件
概率等于1的事件。
不可能事件
概率等于0的事件。
01 点估计
用样本统计量估计总体参数,如用样本均值估计 总体均值。
02 区间估计
给出总体参数的估计区间,如95%置信区间。
03 估计量的性质
无偏性、有效性和一致性。
假设检验
假设检验的基本思想
先假设总体参数具有某种 特性,然后通过样本信息 来判断这个假设是否合理 。
双侧检验
当需要判断两个假设是否 相等时,如总体均值是否 等于某个值。
连续型随机变量
取值无限的随机变 量。
方差
衡量随机变量取值 分散程度的数值。
03
数理统计基础
总体与样本
总体
研究对象的全体。
抽样方法
简单随机抽样、分层抽样、系统抽样等。
样本
从总体中随机抽取的一部分个体,用于估 计和推断总体的特性。
样本大小
样本中包含的个体数量,需要根据研究目 的和资源来确定。
参数估计
单因素方差分析
单因素方差分析的定义
单因素方差分析是方差分析的一种形式,它只涉及一个实验因素。通过对不同组的均值进行比 较,可以确定这个因素对实验结果的影响是否显著。
单因素方差分析的步骤
单因素方差分析通常包括以下步骤:首先,对实验数据进行分组;其次,计算每组的均值;接 着,计算总的均值和总的变异性;然后,计算组间变异性和组内变异性;最后,通过比较这两 种变异,得出因素的显著性。
概率论与数理统计完整ppt课件
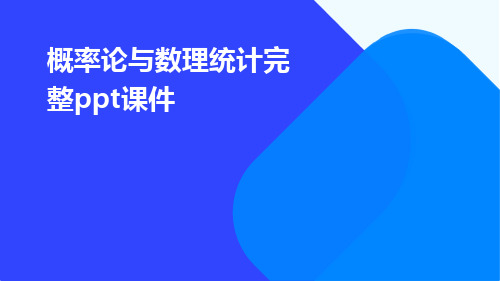
化学
在化学领域,概率论与数理统计被用于研究化学反应的速率和化 学物质的分布,如化学反应动力学、量子化学计算等。
生物
在生物学中,概率论与数理统计用于研究生物现象的变异和分布, 如遗传学、生态学、流行病学等。
在工程中的应用
通信工程
01
概率论与数理统计在通信工程中用于信道容量、误码率、调制
解调等方面的研究。
边缘分布
对于n维随机变量(X_1,...,X_n),在概 率论中,分别定义了X_1的边缘分布 、...、X_n的边缘分布。
04
数理统计基础
样本与抽样分布
01
02
03
总体与样本
总体是包含所有可能数据 的数据集合,样本是总体 的一个随机子集。
抽样方法
包括简单随机抽样、分层 抽样、系统抽样等。
样本分布
描述样本数据的分布情况 ,如均值、中位数、标准 差等。
参数估计与置信区间
参数估计
利用样本数据估计总体的 未知参数,如均值、方差 等。
点估计
用样本统计量作为总体参 数的估计值。
置信区间
给出总体参数的一个估计 区间,表示对总体的参数 有一个可信的估计范围。
假设检验与方差分析
假设检验
通过样本数据对总体参数提出 假设,然后根据假设进行检验
01
定义
设E是一个随机试验,X,Y是定义在E上,取值分别为实数的随机变量
。称有序实数对(X,Y)为一个二维随机变量。
02
分布函数
设(X,Y)是一个二维随机变量,对于任意实数x,y,二元函数
F(x,y)=P({X<=x,Y<=y})称为二维随机变量(X,Y)的分布函数。
03
边缘分布
对于二维随机变量(X,Y),在概率论中,分别定义了X的边缘分布和Y的
在化学领域,概率论与数理统计被用于研究化学反应的速率和化 学物质的分布,如化学反应动力学、量子化学计算等。
生物
在生物学中,概率论与数理统计用于研究生物现象的变异和分布, 如遗传学、生态学、流行病学等。
在工程中的应用
通信工程
01
概率论与数理统计在通信工程中用于信道容量、误码率、调制
解调等方面的研究。
边缘分布
对于n维随机变量(X_1,...,X_n),在概 率论中,分别定义了X_1的边缘分布 、...、X_n的边缘分布。
04
数理统计基础
样本与抽样分布
01
02
03
总体与样本
总体是包含所有可能数据 的数据集合,样本是总体 的一个随机子集。
抽样方法
包括简单随机抽样、分层 抽样、系统抽样等。
样本分布
描述样本数据的分布情况 ,如均值、中位数、标准 差等。
参数估计与置信区间
参数估计
利用样本数据估计总体的 未知参数,如均值、方差 等。
点估计
用样本统计量作为总体参 数的估计值。
置信区间
给出总体参数的一个估计 区间,表示对总体的参数 有一个可信的估计范围。
假设检验与方差分析
假设检验
通过样本数据对总体参数提出 假设,然后根据假设进行检验
01
定义
设E是一个随机试验,X,Y是定义在E上,取值分别为实数的随机变量
。称有序实数对(X,Y)为一个二维随机变量。
02
分布函数
设(X,Y)是一个二维随机变量,对于任意实数x,y,二元函数
F(x,y)=P({X<=x,Y<=y})称为二维随机变量(X,Y)的分布函数。
03
边缘分布
对于二维随机变量(X,Y),在概率论中,分别定义了X的边缘分布和Y的
概率论高等院校概率论课件
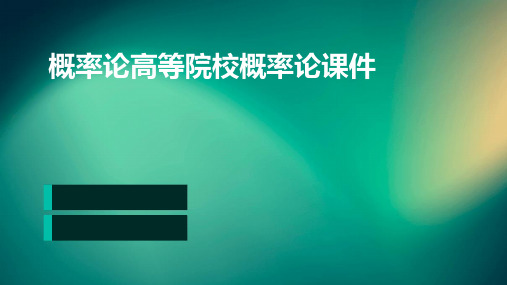
应用场景
强大数定律在统计学中用于 估计极端事件发生的概率和 风险,在决策理论中用于评 估最优策略和期望收益,在 可靠性工程中用于分析系统 的可靠性和寿命。
注意事项
强大数定律的应用有一定的 限制条件,例如随机序列必 须是独立同分布的。此外, 强大数定律并不能保证每个 随机事件的绝对正确性,而 只是给出了最大值分布的稳 定性。
连续随机过程
如布朗运动,每一步都是连续 的,每一步的状态都是连续的
。
随机游走与布朗运动
随机游走
一个随机过程,其中每一步都是随机的,通 常用来描述粒子的无规则运动。
布朗运动
一种连续随机过程,由大量微小粒子在流体 中无规则运动产生,通常用来描述微观粒子 的运动。
马尔科夫链与马尔科夫过程
马尔科夫链
一个随机过程,其中下一个状态只依赖于当前状态,与过去状态 无关。
注意事项
大数定律的前提是试验次数必须足够多,并且随 机事件之间必须是独立的。此外,大数定律并不 能保证每个随机事件的绝对正确性,而只是给出 了频率趋于概率的稳定性。
强大数定律
总结词
强大数定律是概率论中的重 要定理之一,它描述了随机 序列中最大值的分布性质。
详细描述
强大数定律指出,对于任意 给定的正整数序列$a_n$和 $b_n$,有$lim_{n to infty} frac{a_n}{b_n} = 1$的概率 为1。这个定理说明了随机 序列中最大值的分布具有很 强的稳定性。
随机变量的性质
随机变量具有可测性、可加性和有限 可加性。
离散型随机变量及其分布
离散型随机变量的定义
离散型随机变量是在样本空间中取有 限个或可数个值的随机变量。
离散型随机变量的分布
大学概率与统计课件
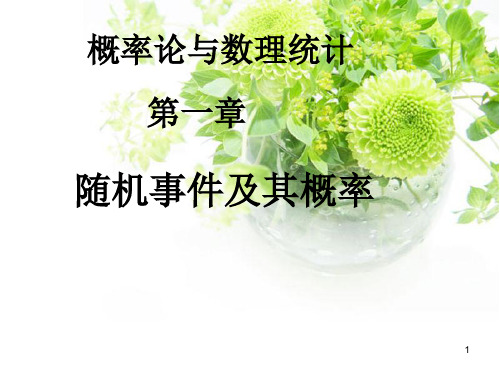
A B A (B A) B ( A B)
A B A AB A B B AB
A B C A(B C) A B C
28
例1.1 设A,B,C为3个事件,用A,B,C的运算式表示下列事件:
(1) A发生而B与C都不发生: ABC 或 A B C 或 A (B C).
结果有可能出现正面也可能出现反面.
5
实例2 用同一门炮向同 一目标发射同一种炮弹多 发 , 观察弹落点的情况. 结果: 弹落点会各不相同.
实例3 抛掷一枚骰子,观 察出现的点数.
结果有可能为:
1, 2, 3, 4, 5 或 6.
6
实例4 从一批含有正品 和次品的产品中任意抽取 一个产品.
实例5 过马路交叉口时, 可能遇上各种颜色的交通 指挥灯.
个发生 A∪B
AB
AB
将事件 A的基本事件和 B的基本事件合在一起组成的 一个新事件,称为 A 和B 的和事件,记为A B ,可 读成 A并 B或 A加B.有时也可记为 A B .
实例 某种产品的合格与否是由该产品的长度与直径
是否合格所决定,因此 C=“产品不合格”是A=“长度
不合格”与B=“直径不合格”的并,即 C A B
其结果可能为: 正品 、次品.
7
实例6 出生的婴儿可 能是男,也可能是女. 实例7 明天的天气可 能是晴 , 也可能是多云 或雨.
8
说明 随机现象在一次观察中出现什么结果具有偶然 性, 但在大量试验或观察中, 这种结果的出现具有 一定的统计规律性 , 概率论就是研究随机现象规 律性的一门数学学科. 如何来研究随机现象? 随机现象是通过随机试验来研究的. 问题 什么是随机试验?
A B A AB A B B AB
A B C A(B C) A B C
28
例1.1 设A,B,C为3个事件,用A,B,C的运算式表示下列事件:
(1) A发生而B与C都不发生: ABC 或 A B C 或 A (B C).
结果有可能出现正面也可能出现反面.
5
实例2 用同一门炮向同 一目标发射同一种炮弹多 发 , 观察弹落点的情况. 结果: 弹落点会各不相同.
实例3 抛掷一枚骰子,观 察出现的点数.
结果有可能为:
1, 2, 3, 4, 5 或 6.
6
实例4 从一批含有正品 和次品的产品中任意抽取 一个产品.
实例5 过马路交叉口时, 可能遇上各种颜色的交通 指挥灯.
个发生 A∪B
AB
AB
将事件 A的基本事件和 B的基本事件合在一起组成的 一个新事件,称为 A 和B 的和事件,记为A B ,可 读成 A并 B或 A加B.有时也可记为 A B .
实例 某种产品的合格与否是由该产品的长度与直径
是否合格所决定,因此 C=“产品不合格”是A=“长度
不合格”与B=“直径不合格”的并,即 C A B
其结果可能为: 正品 、次品.
7
实例6 出生的婴儿可 能是男,也可能是女. 实例7 明天的天气可 能是晴 , 也可能是多云 或雨.
8
说明 随机现象在一次观察中出现什么结果具有偶然 性, 但在大量试验或观察中, 这种结果的出现具有 一定的统计规律性 , 概率论就是研究随机现象规 律性的一门数学学科. 如何来研究随机现象? 随机现象是通过随机试验来研究的. 问题 什么是随机试验?
《概率论》ppt课件
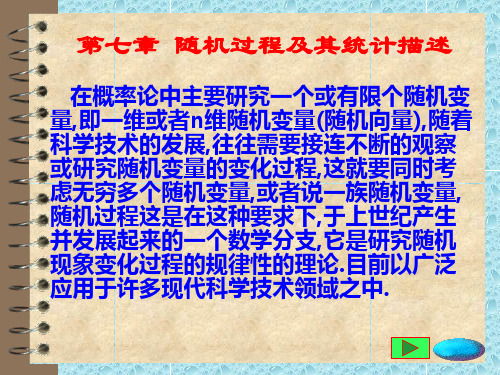
xi R, i 1, 2, , n.
对于固定的 n ,我们称{FX (x1, x2, , xn;t1,t2, ,tn ),ti T}
为随机过程{X (t),t T}的 n 维分布函数族。
注:可以证明(柯尔莫哥洛夫),在一定条件下 ,随机过程的统计特性完全由它的有限维分布函 数族决定。
(二)二维随机过程的联合分布函数
p
2 (1, )
2 1 2
(0, 1 ) 4
1
2
三 随机过程的数字特征
1.单个随机过程的情况
① 函数 X (t) E[X (t)], t T
为{X(t),tT}的均值函数.
②
2 X
(t)
E[ X
2
(t )]
为{X(t),tT}的均方值函数.
③
2 X
(t
)
DX (t) D[ X (t)]
为{X(t),tT}的方差函数.
例3: 考虑抛掷一颗骰子的试验,(i)设 X是n 第n次 (n )1 抛掷的点数,对于n=1,2…的不同值, 是X不n 同的随机变量,因而 { Xn构, n成 1一} 随机过程,称为 贝努利过程或贝努利随机序列,(ii)设Xn是前n次
抛掷中出现的最大点数,
也{是X一n , n随机1}过程。
例 4 在时间 [0,t]内某地段出现的交通事故次数
2. n维分布函数族
对 任 意 正 整 数 n 可 取 定 t1,t2, ,tn T 则 (X (t1), X (t2 ), , X (tn )) 是一个n 维随机变量,他的分 布函数为
FX (x1, x2 , , xn; t1, t2, , tn )
P( X (t1) x1, X (t2 ) x2, , X (tn ) xn ),
对于固定的 n ,我们称{FX (x1, x2, , xn;t1,t2, ,tn ),ti T}
为随机过程{X (t),t T}的 n 维分布函数族。
注:可以证明(柯尔莫哥洛夫),在一定条件下 ,随机过程的统计特性完全由它的有限维分布函 数族决定。
(二)二维随机过程的联合分布函数
p
2 (1, )
2 1 2
(0, 1 ) 4
1
2
三 随机过程的数字特征
1.单个随机过程的情况
① 函数 X (t) E[X (t)], t T
为{X(t),tT}的均值函数.
②
2 X
(t)
E[ X
2
(t )]
为{X(t),tT}的均方值函数.
③
2 X
(t
)
DX (t) D[ X (t)]
为{X(t),tT}的方差函数.
例3: 考虑抛掷一颗骰子的试验,(i)设 X是n 第n次 (n )1 抛掷的点数,对于n=1,2…的不同值, 是X不n 同的随机变量,因而 { Xn构, n成 1一} 随机过程,称为 贝努利过程或贝努利随机序列,(ii)设Xn是前n次
抛掷中出现的最大点数,
也{是X一n , n随机1}过程。
例 4 在时间 [0,t]内某地段出现的交通事故次数
2. n维分布函数族
对 任 意 正 整 数 n 可 取 定 t1,t2, ,tn T 则 (X (t1), X (t2 ), , X (tn )) 是一个n 维随机变量,他的分 布函数为
FX (x1, x2 , , xn; t1, t2, , tn )
P( X (t1) x1, X (t2 ) x2, , X (tn ) xn ),
《概率论》课件
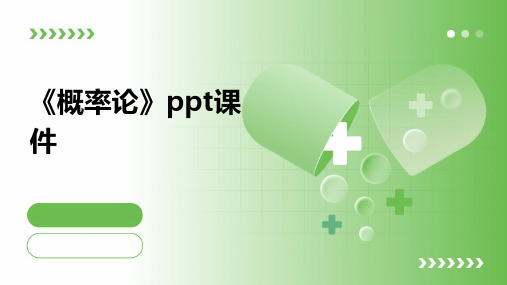
物理学
描述粒子在气体或液体中的运动状态。
金融学
用于股票价格和收益率的分析。
隐马尔科夫模型
定义
隐马尔科夫模型是一种特殊的马尔科夫模型 ,其中观测状态与隐藏状态有关,而隐藏状 态之间相互独立。
应用
语音识别、手写识别、生物信息学等领域。
05
大数定律与中心极限定理
大数定律及其应用
大数定律
在独立重复试验中,当试验次数趋于无穷时,事件发 生的频率趋于该事件发生的概率。
《概率论》ppt课 件
目录
• 概率论简介 • 概率的基本性质 • 随机变量及其分布 • 随机过程与马尔科夫链 • 大数定律与中心极限定理 • 贝叶斯统计推断
01
概率论简介
概率论的定义
概率论
研究随机现象的数学学科,通过数学模型和公式 来描述随机事件、随机变量和随机过程。
随机变量
表示随机现象的数值变量,其取值具有随机性。
THANKS
感谢观看
计算机科学
概率论在计算机科学中用于算法设计和数据 挖掘等领域。
02
概率的基本性质
概率的公理化定义
概率的公理化定义是概率论的基础,它规定了概率的几个基本性质,包括非负性 、规范性、可加性和有限可加性。
非负性指的是任何事件的概率都不小于0;规范性指的是必然事件的概率为1;可 加性指的是两个独立事件的概率等于它们各自概率的和;有限可加性指的是任意 有限个两两独立的事件的概率等于这些事件概率的和。
应用
在统计学中,大数定律用于估计样本的统计量和参数 ,如平均值、方差等。
中心极限定理及其应用
中心极限定理
无论随机变量的分布是什么,当样本量足够大时,样 本均值的分布近似正态分布。
概率论与数理统计基本概念及抽样分布PPT课件
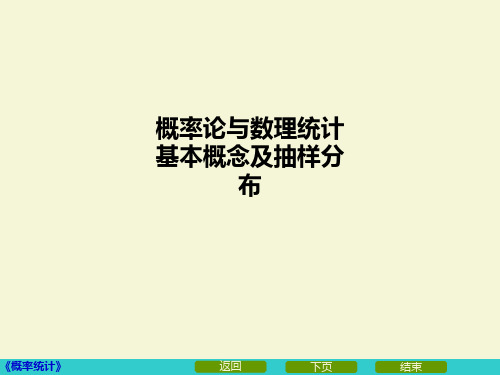
~
2 (n1 ),
2 2
~
2 (n2 ), 且它们相互独立,
则
2 1
2 2
~
2 (n1
n2 )
《概率统计》
返回
下页
结束
4. 2分布的百分位点
对给定的α(0<α<1)
(1)称满足
P{ 2
2
(n)}
,即
f ( y)dy
x2 ( n)
的点为 2分布的上100α百分位点。
f(y)
(2)称满足
注:在研究中,往往关心每个个体的一个(或几个)数量指标和 该数量指标在总体中的分布情况. 这时,每个个体具有的数量 指标的全体就是总体.
或,总体:研究对象的某项数量指标的值的全体.
《概率统计》
某批 灯泡的 寿命
该批灯泡寿命的 全体就是总体
返回
下页
结束
为推断总体分布及各种特征,按一定规则从总体中抽取若 干个体进行观察试验,以获得有关总体的信息,这一抽取过程 为 “抽样”.
( x)
(1)称满足条件 P{X>Xα} =α,
α
即
( x)dx
X
的点Xα为N(0,1)分布的上100α百分位点.
X1-α
0
由于 P{X X } 1 记 -Xα= X1-α
(2)称满足条件 P {| X | X }
2
2
的点 X 为N(0,1)分布的双侧100α百分位点.
X
2
则
E(X )
E(1 n
n i 1
Xi)
1 n
n i 1
E(Xi )
1 n
n
D(X ) D(1 n
n i1
Xi)
- 1、下载文档前请自行甄别文档内容的完整性,平台不提供额外的编辑、内容补充、找答案等附加服务。
- 2、"仅部分预览"的文档,不可在线预览部分如存在完整性等问题,可反馈申请退款(可完整预览的文档不适用该条件!)。
- 3、如文档侵犯您的权益,请联系客服反馈,我们会尽快为您处理(人工客服工作时间:9:00-18:30)。
• Distribution and Statistic
• Chebyshev’s Theorem
3
Outline
• Probability Concepts
– Basic Concepts – Conditioning Concepts
• Law of Total Expectation/Variance, Bayes’ Theorem
Outline
• Probability Concepts
– Basic Concepts – Conditioning Concepts
• Law of Total Expectation/Variance, Bayes’ Theorem
• Limit Theorems
– Modes of Convergence – Law of Large Numbers (LLN) – Central Limit Theorem (CLT)
probability of the event – the probability of A, P(A), regardless of whether B has occurred.
• Joint probability 联合概率: the probability of two
events in conjunction – P(A∩B), P(AB), P(A,B) – If there are two possible outcomes for r.v. X with events B and Bc, then P(A)=P(A∩B)+ P(A∩Bc) Law of Total Probability
16
Conditioning Concepts
• Conditional expectation 条件期望
– the expected value of a r.v. with respect to a conditional probability distribution p ( xy ) E[Y | X x] y pY | X ( y | X x) y p X ( x) y y
– P(A) = prior probability 先验概率
• the probability of A given only initial information
– P(A|B) = posterior probability 后验概率
• the updated conditional probability of A, given initial information and the outcome of B
– the occurrence of A/B makes it neither more nor less probable that B/A occurs,
• P(A ∩ B) = P(A) * P(B) • P(A U B) = P(A) + P(B) – P(A ∩B) – Independent events A = heads on one toss of fair coin B = heads on second toss of same coin – Dependent events A = rain forecasted on the news B = take umbrella to work
• Random Experiment 随机试验
– procedure whose outcome cannot be predicted in advance
• e.g., toss a coin twice
• Sample Space (S or
) 样本空间
– the set of all possible outcomes
• Intersection (and) 交集 & &&
– e.g., A=heads on first, B=heads on second – A ∩ B = {H,H}
• Complement 补集 !
– sets of all outcomes not in A – e.g., A={T,T}, Ac={H,H},{H,T},{T,H}
p
y
Y|X
( y | x) 1
f
y
Y|X
( y | x) 1
15
Conditioning Concepts
• Bayes’ theorem 贝叶斯定理
– relates conditional and marginal probabilities of events A and B, where B has a non-zero probability:
13
Conditioning Concepts
• Conditional probability
– the probability of A, given the occurrence of B
• P(A|B) = P(A∩B)/P(B) → P(A∩B) = P(A|B)P(B) Rule of multiplication 乘法原则 • Independence P(A|B)=? Disjoint events P(A|B)=?
Random Variable
Discrete Random Variable Continuous Random Variable
11
Conditioning Concepts
• Conditioning Concepts
– Conditional Probability
• Law of Total Probability • Bayes’ Theorem
9
Basic Concepts
• If A and B are disjoint,
– they have no element in common. – and if A occurs, then B cannot occur.
• P(A ∩B) =0 • P(A U B) = P(A) + P(B) A Black Cards B Red Cards
– i.e., a function from events to probability levels
6
Basic Concepts
• Union (or) 并集 | ||
– e.g., A=heads on first, B=heads on second – A U B= {H,T},{H,H},{T,H}
E[XY] = E[X]E[Y|X] = E[Y]E[X|Y]
17
Conditioning Concepts
• Law of Total/Iterated Expectation (LIE) 全/重期望律
E[X]=E[E[X|Y]]
• Applications of LIE – Other versions: E[X|I1]=E[E[X|I2]|I1] where the value of I1 is determined by that of I2.
f XY ( x, y ) E[Y | X x] y fY | X ( y | x)dy y dy f X ( x) y y
– Conditional expectation & regression 回归:
regression line: y = E[Y|X]
– Independence & Disjoint: E[Y|X=x] = ? E[XY] = ?
• Probability 概率论
(mathematical foundations of statistics)
Inductive 归纳 (particular → general)
Given the information in the box, what is in your hand?
– Assume known population and parameters, and compute the probability of drawing a particular sample. Deductive 演绎 (general → particular) 2
• conditional probability density function (pdf) of Y given X
f XY ( x, y ) fY | X ( y | x ) f X ( x)
14
Conditioning Concepts
• Comments:
– Conditional distribution can be viewed as a probability distribution defined on a reduced sample space.
A card cannot be Black and Red at the same time.
10
Basic Concepts
• Random Variable (X) 随机变量 r.v.
– represents a possible numerical value from a random event.
– Conditional Expectation
• Law of Total Expectation
– Conditional Variance
• Law of Total Variance
12
Conditioning Concepts
• Marginal probability 边际概率: the unconditional