MIT开放课程Dynamic Programming Lecture (14)
MIT课程列表

第 2 页,共 26 页
MIT课程列表
MIT OCW Networks for Learning Regression and Classification MIT OCW Statistical Learning Theory and Applications MIT OCW Investigating the Neural Substrates of Remote Memory using fMRI MIT OCW Topics in Brain and Cognitive Sciences Human Ethology MIT OCW Computational Cognitive Science MIT OCW Cellular and Molecular Computation MIT OCW Language Acquisition MIT OCW Language Processing MIT OCW Psycholinguistics MIT OCW Language Acquisition I MIT OCW Laboratory in Cognitive Science MIT OCW Introduction to Neural Networks MIT OCW Cognitive Processes MIT OCW Object and Face Recognition MIT OCW Affect Biological, Psychological, and Social Aspects of Feelings MIT OCW Foundations of Cognition MIT OCW Reasonable Conduct in Science MIT OCW Special Topics in Brain and Cognitive Sciences MIT OCW Modularity, Domain specificity, and the Organization of Knowledge MIT OCW Probability and Causality in Human Cognition MIT OCW Cognitive Neuroscience of Remembering Creating and Controlling Memory MIT OCW Language and Mind MIT OCW Media Education and the Marketplace MIT OCW Introduction to Psychology 2001 MIT OCW Introduction to Psychology 2002 MIT OCW Neuroscience and Behavior MIT OCW The Brain and Cognitive Sciences I MIT OCW The Brain and Cognitive Sciences II MIT OCW Brain Laboratory MIT OCW Evolutionary Psychology MIT OCW Introduction to Computational Neuroscience MIT OCW Social Psychology MIT OCW Functional MRI of High Level Vision MIT OCW Foundations of Human Memory and Learning MIT OCW Psychology of Gender MIT OCW Intensive Neuroanatomy MIT OCW Pattern Recognition for Machine Vision MIT OCW Research Topics in Neuroscience MIT OCW Experimental Methods of Adjustable Tetrode Array Neurophysiology MIT OCW Economic History MIT OCW Econometrics Spring MIT OCW Political Economy I Theories of the State and the Economy MIT OCW Political Economy of Western Europe MIT OCW Political Economy of Chinese Reform MIT OCW Political Economy of Latin America
国外开放课程及一些国外大学的网址
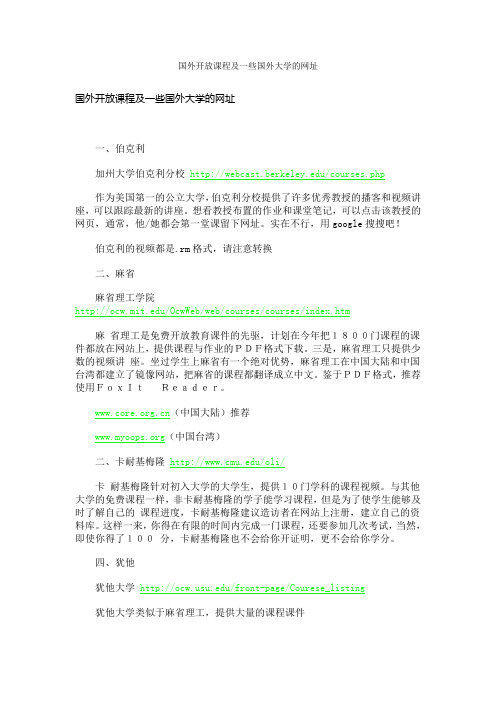
国外开放课程及一些国外大学的网址国外开放课程及一些国外大学的网址一、伯克利加州大学伯克利分校/courses.php作为美国第一的公立大学,伯克利分校提供了许多优秀教授的播客和视频讲座,可以跟踪最新的讲座。
想看教授布置的作业和课堂笔记,可以点击该教授的网页,通常,他/她都会第一堂课留下网址。
实在不行,用google搜搜吧!伯克利的视频都是.rm格式,请注意转换二、麻省麻省理工学院/OcwWeb/web/courses/courses/index.htm麻省理工是免费开放教育课件的先驱,计划在今年把1800门课程的课件都放在网站上,提供课程与作业的PDF格式下载。
三是,麻省理工只提供少数的视频讲座。
坐过学生上麻省有一个绝对优势,麻省理工在中国大陆和中国台湾都建立了镜像网站,把麻省的课程都翻译成立中文。
鉴于PDF格式,推荐使用FoxItReader。
(中国大陆)推荐(中国台湾)二、卡耐基梅隆/oli/卡耐基梅隆针对初入大学的大学生,提供10门学科的课程视频。
与其他大学的免费课程一样,非卡耐基梅隆的学子能学习课程,但是为了使学生能够及时了解自己的课程进度,卡耐基梅隆建议造访者在网站上注册,建立自己的资料库。
这样一来,你得在有限的时间内完成一门课程,还要参加几次考试,当然,即使你得了100分,卡耐基梅隆也不会给你开证明,更不会给你学分。
四、犹他犹他大学/front-page/Courese_listing犹他大学类似于麻省理工,提供大量的课程课件五、塔夫茨塔夫茨大学塔夫茨大学也是“开放式教育课程”的先驱之一,初期提供的课程着重在本校专长的生命科学、跨领域方法、国际观点以及对美国地区性、全国性社群服务的基础理论。
六、公开英国公开大学/course/index.php英国十几所大学联合起来,组建了英国公开大学。
有一部分课程是对注册学生开放的,但是有一批很好的课程是免费的,并提供视频。
每门课还设立了论坛,在社区中,大家发表意见,提供其他的学习资源,互相取经。
名校课堂——精选推荐
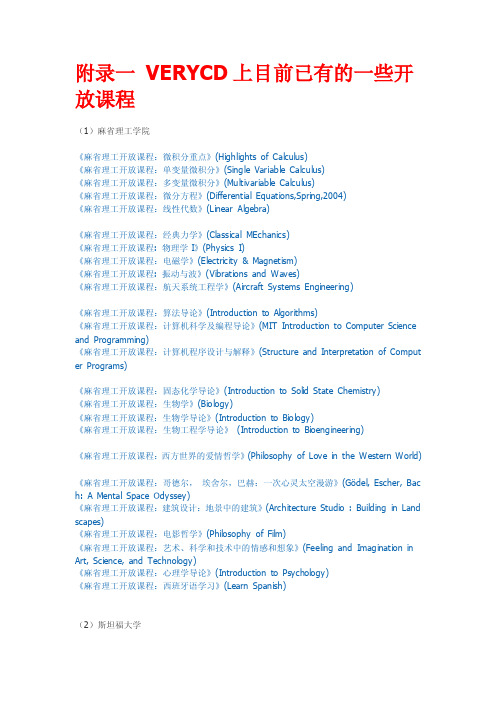
附录一VERYCD上目前已有的一些开放课程(1)麻省理工学院《麻省理工开放课程:微积分重点》(Highlights of Calculus)《麻省理工开放课程:单变量微积分》(Single Variable Calculus)《麻省理工开放课程:多变量微积分》(Multivariable Calculus)《麻省理工开放课程:微分方程》(Differential Equations,Spring,2004)《麻省理工开放课程:线性代数》(Linear Algebra)《麻省理工开放课程:经典力学》(Classical MEchanics)《麻省理工开放课程: 物理学I》(Physics I)《麻省理工开放课程:电磁学》(Electricity & Magnetism)《麻省理工开放课程: 振动与波》(Vibrations and Waves)《麻省理工开放课程:航天系统工程学》(Aircraft Systems Engineering)《麻省理工开放课程:算法导论》(Introduction to Algorithms)《麻省理工开放课程:计算机科学及编程导论》(MIT Introduction to Computer Science and Programming)《麻省理工开放课程:计算机程序设计与解释》(Structure and Interpretation of Comput er Programs)《麻省理工开放课程:固态化学导论》(Introduction to Solid State Chemistry)《麻省理工开放课程:生物学》(Biology)《麻省理工开放课程:生物学导论》(Introduction to Biology)《麻省理工开放课程:生物工程学导论》(Introduction to Bioengineering)《麻省理工开放课程:西方世界的爱情哲学》(Philosophy of Love in the Western World)《麻省理工开放课程:哥德尔,埃舍尔,巴赫:一次心灵太空漫游》(Gödel, Escher, Bac h: A Mental Space Odyssey)《麻省理工开放课程:建筑设计:地景中的建筑》(Architecture Studio : Building in Land scapes)《麻省理工开放课程:电影哲学》(Philosophy of Film)《麻省理工开放课程:艺术、科学和技术中的情感和想象》(Feeling and Imagination in Art, Science, and Technology)《麻省理工开放课程:心理学导论》(Introduction to Psychology)《麻省理工开放课程:西班牙语学习》(Learn Spanish)(2)斯坦福大学《斯坦福大学开放课程:编程范式》(Programming Paradigms )《斯坦福大学开放课程:抽象编程》(Programming Abstractions)《斯坦福大学开放课程:iPhone开发教程》(Phone Application Programming)《斯坦福大学开放课程:编程模式(C和C++)》(Introduction to Computer Science - Pro gramming Abstractions)《斯坦福大学开放课程:编程方法》(Programming Methodology)《斯坦福大学开放课程:人机交互研讨》(Human-Computer Interaction Seminar)《斯坦福大学开放课程:机器学习》(Engineering Everywhere - Machine Learning)《斯坦福大学开放课程:机器人学》(Introduction to robotics)《斯坦福大学开放课程:傅立叶变换及应用》(The Fourier Transform and Its Application s )《斯坦福大学开放课程:近现代物理专题课程-宇宙学》(Modern Physics - Cosmology)《斯坦福大学开放课程:近现代物理专题课程-经典力学》(Modern Physics - Classical Me chanics)《斯坦福大学开放课程:近现代物理专题课程-统计力学》(Modern Physics - Statistic Me chanics)[《斯坦福大学开放课程:近现代物理专题课程-量子力学》(Modern Physics - Quantum M echanics)《斯坦福大学开放课程:近现代物理专题课程-量子纠缠-part1》(Modern Physics - Quant um Entanglement, Part 1)《斯坦福大学开放课程:近现代物理专题课程-量子纠缠-part3》(Modern Physics - Quant um Entanglement, Part 3)《斯坦福大学开放课程:近现代物理专题课程-广义相对论》(Modern Physics - Einstein's Theory)《斯坦福大学开放课程:近现代物理专题课程-狭义相对论》(Modern Physics - Special Re lativ ity)《斯坦福大学开放课程:线性动力系统绪论》(Introduction to Linear Dynamical Systems)《斯坦福大学开放课程:经济学》(Economics)《斯坦福大学开放课程:商业领袖和企业家》(Business Leaders and Entrepreneurs)《斯坦福大学开放课程:法律学》(Law)《斯坦福大学开放课程:达尔文的遗产》(Darwin's Legacy)《斯坦福大学开放课程:人类健康的未来:七个颠覆你思想的演讲》(The Future of Huma n Health: 7 Very Short Talks That Will Blow Your Mind)《斯坦福大学开放课程:迷你医学课堂:医学、健康及科技前沿》(Mini Med School:Med icine, Human Health, and the Frontiers of Science)《斯坦福大学开放课程:迷你医学课堂:人类健康之动态》(Mini Med School : The Dyna mics of Human Health)(3)耶鲁大学《耶鲁大学开放课程:基础物理》(Fundamentals of Physics)《耶鲁大学开放课程:天体物理学之探索和争议》(Frontiers and Controversies in Astrop hysics)《耶鲁大学开放课程:新生有机化学》(Freshman Organic Chemistry )《耶鲁大学开放课程:生物医学工程探索》(Frontiers of Biomedical Engineering)《耶鲁大学开放课程:博弈论》(Game Theory)《耶鲁大学开放课程:金融市场》(Financial Markets )《耶鲁大学开放课程:文学理论导论》(Introduction to Theory of Literature )《耶鲁大学开放课程:现代诗歌》(Modern Poetry)《耶鲁大学开放课程:1945年后的美国小说》(The American Novel Since 1945)《耶鲁大学开放课程: 弥尔顿》(Milton)《耶鲁大学开放课程:欧洲文明》(European Civilization )《耶鲁大学开放课程:旧约全书导论》(Introduction to the Old Testament (Hebrew Bibl e) )《耶鲁大学开放课程:新约及其历史背景》(Introduction to New Testament History and Literature)《耶鲁大学开放课程:1871年后的法国》(France Since 1871)《耶鲁大学开放课程:古希腊历史简介》(Introduction to Ancient Greek History )《耶鲁大学开放课程:美国内战与重建,1845-1877》(The Civil War and Reconstruction Era,1845-1877)《耶鲁大学开放课程:全球人口增长问题》(Global Problems of Population Growth)《耶鲁大学开放课程:进化,生态和行为原理》(Principles of Evolution, Ecology, and Be havior )《耶鲁大学开放课程:哲学:死亡》(Philosophy:Death)《耶鲁大学开放课程:政治哲学导论》(Introduction to Political Philosophy)《耶鲁大学开放课程:有关食物的心理学,生物学和政治学》(The Psychology, Biology a nd Politics of Food)《耶鲁大学开放课程:心理学导论》(Introduction to Psychology)《耶鲁大学开放课程:罗马建筑》(Roman Architecture)《耶鲁大学开放课程:聆听音乐》(Listening to Music)(4)哈佛大学《哈佛大学开放课程:哈佛幸福课》(Positive Psychology at Harvard)《哈佛大学开放课程:公正:该如何做是好?》(Justice: What's the Right Thing to Do? )《哈佛大学开放课程:构设动态网站》(Building Dynamic Websites)(5)牛津大学《牛津大学开放课程:尼采的心灵与自然》(Nietzsche on Mind and Nature)《牛津大学开放课程:哲学概论》(General Philosophy)《牛津大学开放课程:哲学入门》(Philosophy for Beginners)《牛津大学开放课程:批判性推理入门》(Critical Reasoning for Beginners) (6)其它名校《普林斯顿大学开放课程:人性》(InnerCore)《普林斯顿大学开放课程:自由意志定理》(The Free Will Theorem)《剑桥大学开放课程:人类学》(Anthropology)《沃顿商学院开放课程:沃顿知识在线》(Knowledge@Wharton)《哥伦比亚大学开放课程:房地产金融学I》(Real Estate Finance I)附录二部分英美名校开放课程网站美国1. 麻省理工学院/index.htm2. 卡内基梅隆大学/openlearning/forstudents/freecourses3. 约翰霍普金斯大学彭博公共卫生学院/4. 斯坦福大学/5. 圣母大学/courselist6. 杜克大学法律中心的公共领域/cspd/lectures7. 哈佛医学院/public/8. 普林斯顿大学/main/index.php9. 耶鲁大学/10. 加州大学伯克利分校英国1. 牛津大学的文字资料馆2. Greshem学院/default.asp3. 格拉斯哥大学/downloads.html4. 萨里大学/Teaching/5. 诺丁汉大学/6. 剑桥大学播客/main/Podcasts.html 参考资料:/thread-42142-1-1.html。
MIT14_15JF09_lec09
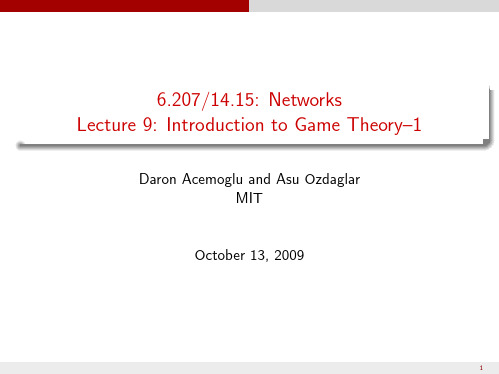
In all of these cases, interactions with other agents you are connected to affect your payoff, well-being, utility. How to make decisions in such situations? → “multiagent decision theory” or game theory.
Reading: Osborne, Chapters 1-2. EK, Chapter 6.
2
Networks: Lecture 9
Introduction
Motivation
In the context of social networks, or even communication networks, agents make a variety of choices. For example:
3
Networks: Lectu“Rational Decision-Making”
Powerful working hypothesis in economics: individuals act rationally in the sense of choosing the option that gives them higher “payoff”.
�
u (c ) dF a (c ) ≥ U (b ) =
�
u (c ) dF b (c ) .
7
Networks: Lecture 9
Introduction
From Single Person to Multiperson Decision Problems
全球50名校开放课程网址大全
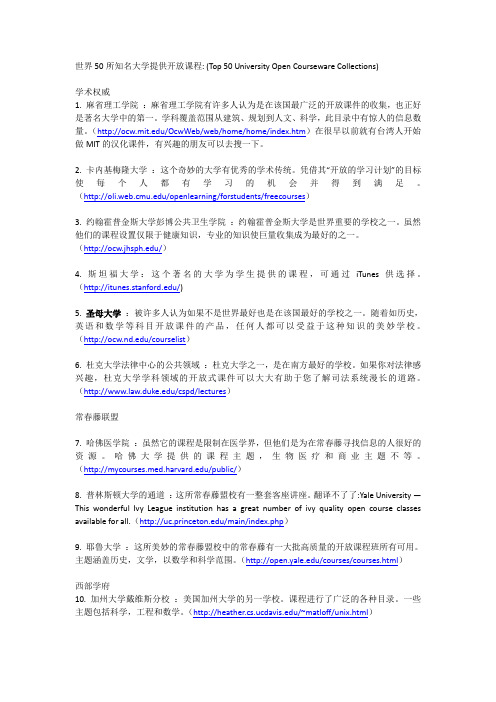
世界50所知名大学提供开放课程: (Top 50 University Open Courseware Collections)学术权威1. 麻省理工学院:麻省理工学院有许多人认为是在该国最广泛的开放课件的收集,也正好是著名大学中的第一。
学科覆盖范围从建筑、规划到人文、科学,此目录中有惊人的信息数量。
(/OcwWeb/web/home/home/index.htm)在很早以前就有台湾人开始做MIT的汉化课件,有兴趣的朋友可以去搜一下。
2. 卡内基梅隆大学:这个奇妙的大学有优秀的学术传统。
凭借其“开放的学习计划”的目标使每个人都有学习的机会并得到满足。
(/openlearning/forstudents/freecourses)3. 约翰霍普金斯大学彭博公共卫生学院:约翰霍普金斯大学是世界重要的学校之一。
虽然他们的课程设置仅限于健康知识,专业的知识使巨量收集成为最好的之一。
(/)4. 斯坦福大学:这个著名的大学为学生提供的课程,可通过iTunes供选择。
(/)5. 圣母大学:被许多人认为如果不是世界最好也是在该国最好的学校之一。
随着如历史,英语和数学等科目开放课件的产品,任何人都可以受益于这种知识的美妙学校。
(/courselist)6. 杜克大学法律中心的公共领域:杜克大学之一,是在南方最好的学校。
如果你对法律感兴趣,杜克大学学科领域的开放式课件可以大大有助于您了解司法系统漫长的道路。
(/cspd/lectures)常春藤联盟7. 哈佛医学院:虽然它的课程是限制在医学界,但他们是为在常春藤寻找信息的人很好的资源。
哈佛大学提供的课程主题,生物医疗和商业主题不等。
(/public/)8. 普林斯顿大学的通道:这所常春藤盟校有一整套客座讲座。
翻译不了了:Yale University —This wonderful Ivy League institution has a great number of ivy quality open course classes available for all.(/main/index.php)9. 耶鲁大学:这所美妙的常春藤盟校中的常春藤有一大批高质量的开放课程班所有可用。
MIT开放课程Dynamic Programming Lecture (22)
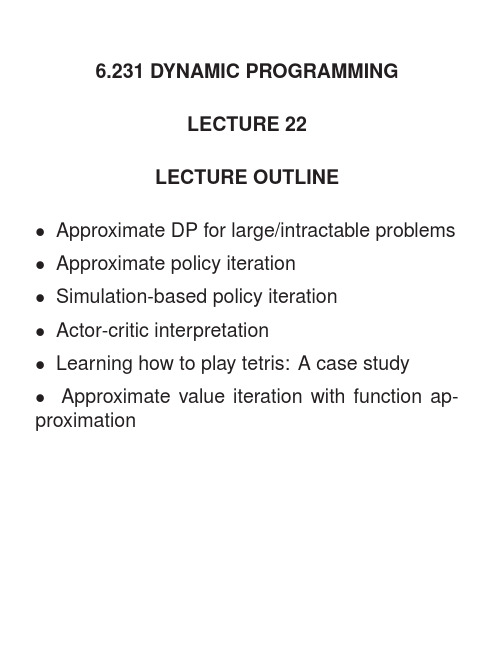
6.231DYNAMIC PROGRAMMINGLECTURE22LECTURE OUTLINE•Approximate DP for large/intractable problems •Approximate policy iteration•Simulation-based policy iteration•Actor-critic interpretation•Learning how to play tetris:A case study •Approximate value iteration with function ap-proximationAPPROX.POLICY ITERATION-DISCOUNTED CASE •Suppose that the policy evaluation is approxi-mate,according to,maxx|J k(x)−Jµk(x)|≤δ,k=0,1,...and policy improvement is also approximate,ac-cording to,maxx|(Tµk+1J k)(x)−(T J k)(x)|≤ ,k=0,1,...whereδand are some positive scalars.•Error Bound:The sequence{µk}generatedby the approximate policy iteration algorithm sat-isfieslim sup k→∞maxx∈SJµk(x)−J∗(x)≤ +2αδ(1−α)2•Typical practical behavior:The method makes steady progress up to a point and then the iteratesJµkoscillate within a neighborhood of J∗.APPROXIMATE POLICY ITERATION-SSP •Suppose that the policy evaluation is approxi-mate,according to,maxi=1,...,n|J k(i)−Jµk(i)|≤δ,k=0,1,...and policy improvement is also approximate,ac-cording to,maxi=1,...,n|(Tµk+1J k)(i)−(T J k)(i)|≤ ,k=0,1,... whereδand are some positive scalars.•Assume that all policies generated by the method are proper(they are guaranteed to be ifδ= =0, but not in general).•Error Bound:The sequence{µk}generated by approximate policy iteration satisfieslim sup k→∞maxi=1,...,nJµk(i)−J∗(i)≤n(1−ρ+n)( +2δ)(1−ρ)2whereρ=max i=1,...,nµ:properP{x n=t|x0=i,µ}SIMULATION-BASED POLICY EVALUATION •Givenµ,suppose we want to calculate Jµby simulation.•Generate by simulation sample costs.Approx-imation:Jµ(i)≈1M iM im=1c(i,m)c(i,m):m th sample cost starting from state i •Approximating each Jµ(i)is impractical for a large state space.Instead,a“compact represen-tation”˜Jµ(i,r)may be used,where r is a tunable parameter vector.We may calculate an optimal value r∗of r by a least squaresfitr∗=arg minrni=1M im=1c(i,m)−˜Jµ(i,r)2•This idea is the starting point for more sophisti-cated simulation-related methods,to be discussed in the next lecture.ACTOR-CRITIC INTERPRETATION•The critic calculates approximately(e.g.,using some form of a least squaresfit)Jµk by processing state/sample cost pairs,which are generated by the actor by simulation•Given the approximate Jµk,the actor imple-ments the improved policy Jµk+1byJ k)(i)=(T J k)(i)(Tµk+1•The state consists of the board position i,and the shape of the current falling block(astronomi-cally large number of states).•It can be shown that all policies are proper!!•Use a linear approximation architecture with feature extraction˜J(i,r)=sm=1φm(i)r m,where r=(r1,...,r s)is the parameter vector and φm(i)is the value of m th feature associated w/i.•Approximate policy iteration was implemented with the following features:−The height of each column of the wall−The difference of heights of adjacent columns −The maximum height over all wall columns −The number of“holes”on the wall−The number1(provides a constant offset)•Playing data was collected for afixed value of the parameter vector r(and the corresponding policy);the policy was approximately evaluated by choosing r to match the playing data in some least-squares sense.•The method used for approximate policy eval-uation was theλ-least squares policy evaluation method,to be described in the next lecture.•See:Bertsekas and Ioffe,“Temporal Differences-Based Policy Iteration and Applications in Neuro-Dynamic Programming,”in:8001//people/dimitrib/publ.htmlVALUE ITERATION W/FUNCTION APPROXIMATION •Suppose we use a linear approximation archi-tecture ˜J(i,r )=φ(i ) r ,or ˜J=Φr where r =(r 1,...,r s )is a parameter vector,and Φis a full rank n ×s feature matrix.•Approximate value iteration method:Start with initial guess r 0;given r t ,generate r t +1byr t +1=arg min rΦr −T (Φr t ) where · is some norm.•Questions:Does r t converge to some r ∗?How close is Φr ∗to J ∗?•Convergence Result:If T is a contraction with respect to a weighted Euclidean norm ( J 2=J DJ ,where D is positive definite,symmetric),then r t converges to (the unique)r ∗satisfying r ∗=arg min rΦr −T (Φr ∗)GEOMETRIC INTERPRETATION•Consider the feature subspaceS ={Φr |r ∈ s }of all cost function approximations that are linear combinations of the feature vectors.Let Πdenote projection on this subspace.•The approximate value iteration is r t +1=ΠT (Φr t )=arg min rΦr −T (Φr t ) and amounts to starting at the point Φr t of S ap-plying T to it and then projecting on S .•Proof Idea:Since T is a contraction with re-spect to the norm of projection,and projection is nonexpansive,ΠT (which maps S to S )is a con-traction (with respect to the same norm).PROOF•Consider two vectorsΦr andΦr in S.The(Eu-clidean)projection is a nonexpansive mapping,so ΠT(Φr)−ΠT(Φr ) ≤ T(Φr)−T(Φr ) Since T is a contraction mapping(with respect to the norm of projection),T(Φr)−T(Φr ) ≤β Φr−Φrwhereβ∈(0,1)is the contraction modulus,so ΠT(Φr)−ΠT(Φr ) ≤β Φr−Φrand it follows thatΠT is a contraction(with respect to the same norm and with the same modulus).•In general,it is not clear how to obtain a Eu-clidean norm for which T is a contraction.•Important fact:In the case where T=Tµ, whereµis a stationary policy,T is a contraction for the norm J 2=J DJ,where D is diagonal with the steady-state probabilities along the diagonal.ERROR BOUND•If T is a contraction with respect to a weighted Euclidean norm · with modulus β,and r ∗is the limit of r t ,i.e.,r ∗=arg min rΦr −T (Φr ∗) then Φr ∗−J ∗ ≤ ΠJ ∗−J ∗ 1−βwhere J ∗is the fixed point of T ,and ΠJ ∗is the projection of J ∗on the feature subspace S (with respect to norm · ).Proof:Using the triangle inequality,Φr ∗−J ∗ ≤ Φr ∗−ΠJ ∗ + ΠJ ∗−J ∗= ΠT (Φr ∗)−ΠT (J ∗) + ΠJ ∗−J ∗ ≤β Φr ∗−J ∗ + ΠJ ∗−J ∗ Q.E.D.•Note that the error Φr ∗−J ∗ is proportional to ΠJ ∗−J ∗ ,which can be viewed as the “power of the approximation architecture”(measures how well J ∗can be represented by the chosen fea-tures).。
10960-运筹学-21_Dynamic+Programming+I
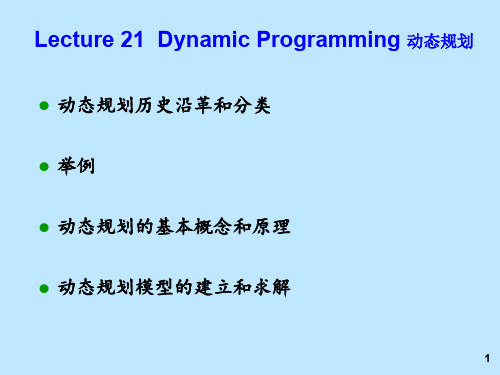
11 12 13 14 15 16 17 18 19 20
8
Decisions with 4 or 5 matches left.
Take 1
You
win.
Take 2
4
Your opponent is Y. 3 Y loses 2 Y wins
Take 6
11
Decisions with n matches left.
Take 1
f(n)
Take 2
n
Take 6
n-1 f(n-1) n-2 f(n-2) n-6 f(n-6)
n The state
The optimal f(n-1) value
function.
TImTfcosfnrrothhpooaatmeetmmtthicemsoDPpifahsmt(tnpuraynhnaeicttlnTadatis)iiasvemnakhct=fstamigiept(dhanpelonWuoealitgD,l)chemesitvany=itescarfPolhnatlerlLfofrefukaoitm(oobhpOnemeinorgfeaat-p.paif1irsfpitmcun(tat)rnoidianmsePa=m-nelc.v1llrmaceLatoi)vtiolilhoisgan=otnudanilyrerougsfeta.e(ffnecmni(d(sRsTn,ni-ftssmeh2aua-)ni2c)entotndeioe)un=dnceeos=rgktdspfst.wi(nhLcoeRitnohiorednm-eoinin6wblcrfieu)noeiupfstm=sri(hrrsnnsotmeWtth-diahsc6oaent;ee)neakcuem=vtiimndnesaeLgiblfonto;urhep.anoeortmditdomoeftocadhfiloeslyiaotn.
MIT公开课程介绍
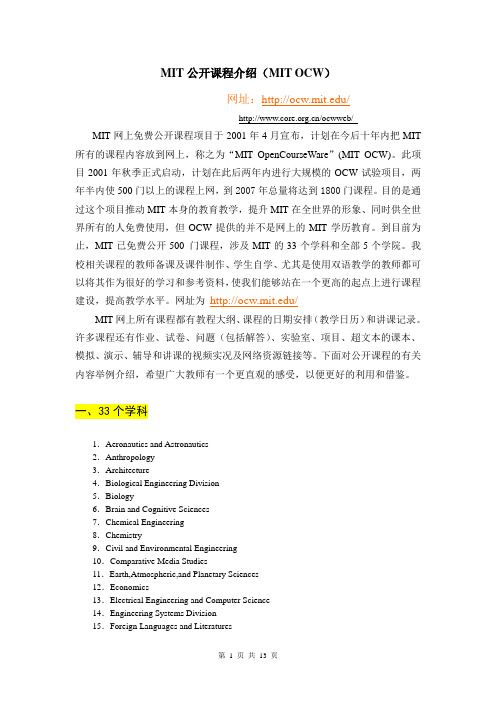
MIT公开课程介绍(MIT OCW)网址://ocwweb/MIT网上免费公开课程项目于2001年4月宣布,计划在今后十年内把MIT 所有的课程内容放到网上,称之为“MIT OpenCourseWare”(MIT OCW)。
此项目2001年秋季正式启动,计划在此后两年内进行大规模的OCW试验项目,两年半内使500门以上的课程上网,到2007年总量将达到1800门课程。
目的是通过这个项目推动MIT本身的教育教学,提升MIT在全世界的形象、同时供全世界所有的人免费使用,但OCW提供的并不是网上的MIT学历教育。
到目前为止,MIT已免费公开500 门课程,涉及MIT的33个学科和全部5个学院。
我校相关课程的教师备课及课件制作、学生自学、尤其是使用双语教学的教师都可以将其作为很好的学习和参考资料,使我们能够站在一个更高的起点上进行课程建设,提高教学水平。
网址为/MIT网上所有课程都有教程大纲、课程的日期安排(教学日历)和讲课记录。
许多课程还有作业、试卷、问题(包括解答)、实验室、项目、超文本的课本、模拟、演示、辅导和讲课的视频实况及网络资源链接等。
下面对公开课程的有关内容举例介绍,希望广大教师有一个更直观的感受,以便更好的利用和借鉴。
一、33个学科1.Aeronautics and Astronautics2.Anthropology3.Architecture4.Biological Engineering Division5.Biology6.Brain and Cognitive Sciences7.Chemical Engineering8.Chemistry9.Civil and Environmental Engineering10.Comparative Media Studies11.Earth,Atmospheric,and Planetary Sciences12.Economics13.Electrical Engineering and Computer Science14.Engineering Systems Division15.Foreign Languages and Literatures16.Health Science and Technology17.History18.Linguistics and Philosophy19.Literature20.Material Science and Technology21.Mathematics22.Mechanical Engineering23.Media Arts and Sciences24.Music and Theater Arts25.Nuclear Engineering26.Ocean Engineering27.Physics28.Political Science29.Science ,Technology and Society30.Sloan School of Management31.Urban Studies and Planning32.Women’s Studies33.Writing and Humanistic Studies二、课程大纲我们以建筑学系为例。
- 1、下载文档前请自行甄别文档内容的完整性,平台不提供额外的编辑、内容补充、找答案等附加服务。
- 2、"仅部分预览"的文档,不可在线预览部分如存在完整性等问题,可反馈申请退款(可完整预览的文档不适用该条件!)。
- 3、如文档侵犯您的权益,请联系客服反馈,我们会尽快为您处理(人工客服工作时间:9:00-18:30)。
6.231DYNAMIC PROGRAMMINGLECTURE14LECTURE OUTLINE•Limited lookahead policies •Performance bounds •Computational aspects•Problem approximation approach •Vehicle routing example •Heuristic cost-to-go approximation •Computer chessLIMITED LOOKAHEAD POLICIES •One-step lookahead(1SL)policy:At each k and state x k,use the controlµk(x k)thatminu k∈U k(x k)Eg k(x k,u k,w k)+˜J k+1f k(x k,u k,w k),where−˜J N=g N.−˜J k+1:approximation to true cost-to-go J k+1•Two-step lookahead policy:At each k and x k,use the control˜µk(x k)attaining the minimum above, where the function˜J k+1is obtained using a1SL approximation(solve a2-step DP problem).•If˜J k+1is readily available and the minimization above is not too hard,the1SL policy is imple-mentable on-line.•Sometimes one also replaces U k(x k)above with a subset of“most promising controls”U k(x k).•As the length of lookahead increases,the re-quired computation quickly explodes.PERFORMANCE BOUNDS•Let J k(x k)be the cost-to-go from(x k,k)of the 1SL policy,based on functions˜J k.•Assume that for all(x k,k),we haveˆJk(x k)≤˜J k(x k),(*) whereˆJ N=g N and for all k,ˆJ k (x k)=minu k∈U k(x k)Eg k(x k,u k,w k)+˜J k+1f k(x k,u k,w k),[soˆJ k(x k)is computed along withµk(x k)].ThenJ k(x k)≤ˆJ k(x k),for all(x k,k).•Important application:When˜J k is the cost-to-go of some heuristic policy(then the1SL policy is called the rollout policy).•The bound can be extended to the case where there is aδk in the RHS of(*).ThenJ k(x k)≤˜J k(x k)+δk+···+δN−1COMPUTATIONAL ASPECTS •Sometimes nonlinear programming can be used to calculate the1SL or the multistep version[par-ticularly when U k(x k)is not a discrete set].Con-nection with the methodology of stochastic pro-gramming.•The choice of the approximating functions˜J k is critical,and is calculated with a variety of methods.•Some approaches:(a)Problem Approximation:Approximate the op-timal cost-to-go with some cost derived froma related but simpler problem(b)Heuristic Cost-to-Go Approximation:Approx-imate the optimal cost-to-go with a functionof a suitable parametric form,whose param-eters are tuned by some heuristic or system-atic scheme(Neuro-Dynamic Programming) (c)Rollout Approach:Approximate the optimalcost-to-go with the cost of some suboptimalpolicy,which is calculated either analyticallyor by simulationPROBLEM APPROXIMATION •Many(problem-dependent)possibilities−Replace uncertain quantities by nominal val-ues,or simplify the calculation of expectedvalues by limited simulation−Simplify difficult constraints or dynamics •Example of enforced decomposition:Route m vehicles that move over a graph.Each node has a“value.”Thefirst vehicle that passes through the node collects its value.Max the total collected value,subject to initial andfinal time constraints (plus time windows and other constraints).•Usually the1-vehicle version of the problem is much simpler.This motivates an approximation obtained by solving single vehicle problems.•1SL scheme:At time k and state x k(position of vehicles and“collected value nodes”),consider all possible k th moves by the vehicles,and at the resulting states we approximate the optimal value-to-go with the value collected by optimizing the vehicle routes one-at-a-timeHEURISTIC COST-TO-GO APPROXIMATION •Use a cost-to-go approximation from a paramet-ric class˜J(x,r)where x is the current state and r=(r1,...,r m)is a vector of“tunable”scalars (weights).•By adjusting the weights,one can change the “shape”of the approximation˜J so that it is reason-ably close to the true optimal cost-to-go function.•Two key issues:−The choice of parametric class˜J(x,r)(the approximation architecture).−Method for tuning the weights(“training”the architecture).•Successful application strongly depends on how these issues are handled,and on insight about the problem.•Sometimes a simulator is used,particularly when there is no mathematical model of the sys-tem.APPROXIMATION ARCHITECTURES •Divided in linear and nonlinear[i.e.,linear or nonlinear dependence of˜J(x,r)on r].•Linear architectures are easier to train,but non-linear ones(e.g.,neural networks)are richer.•Architectures based on feature extraction(•Ideally,the features will encode much of the nonlinearity that is inherent in the cost-to-go ap-proximated,and the approximation may be quite accurate without a complicated architecture.•Sometimes the state space is partitioned,and “local”features are introduced for each subset of the partition(they are0outside the subset).•With a well-chosen feature vector y(x),we can use a linear architecture˜J(x,r)=ˆJ y(x),r =r i y i(x)i•Programs use a feature-based position evalua-tor that assigns a score to each move/position•Most often the weighting of features is linear but multistep lookahead is involved.•Most often the training is done by trial and error.•Additional features:−Depthfirst search−Variable depth search when dynamic posi-tions are involved−Alpha-beta pruning•Multistep lookahead tree+8+20+18+16+24+20+10+12-4+8+21+11-5+10+32+27+10+9+3•Alpha-beta pruning:As the move scores are evaluated by depth-first search,branches whose consideration(based on the calculations so far) cannot possibly change the optimal move are ne-glected。