Parallelizing Dense Linear Algebra Operations with Task Queues in llc
Linear Algebra and its Applications
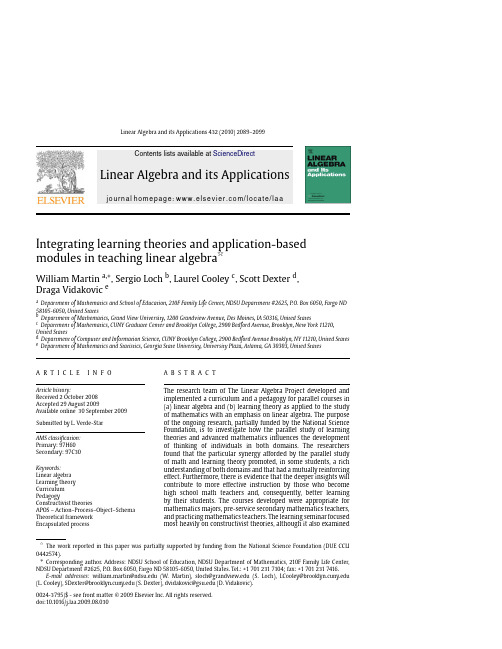
Linear Algebra and its Applications432(2010)2089–2099Contents lists available at ScienceDirect Linear Algebra and its Applications j o u r n a l h o m e p a g e:w w w.e l s e v i e r.c o m/l o c a t e/l aaIntegrating learning theories and application-based modules in teaching linear algebraୋWilliam Martin a,∗,Sergio Loch b,Laurel Cooley c,Scott Dexter d,Draga Vidakovic ea Department of Mathematics and School of Education,210F Family Life Center,NDSU Department#2625,P.O.Box6050,Fargo ND 58105-6050,United Statesb Department of Mathematics,Grand View University,1200Grandview Avenue,Des Moines,IA50316,United Statesc Department of Mathematics,CUNY Graduate Center and Brooklyn College,2900Bedford Avenue,Brooklyn,New York11210, United Statesd Department of Computer and Information Science,CUNY Brooklyn College,2900Bedford Avenue Brooklyn,NY11210,United Statese Department of Mathematics and Statistics,Georgia State University,University Plaza,Atlanta,GA30303,United StatesA R T I C L E I N F O AB S T R AC TArticle history:Received2October2008Accepted29August2009Available online30September2009 Submitted by L.Verde-StarAMS classification:Primary:97H60Secondary:97C30Keywords:Linear algebraLearning theoryCurriculumPedagogyConstructivist theoriesAPOS–Action–Process–Object–Schema Theoretical frameworkEncapsulated process The research team of The Linear Algebra Project developed and implemented a curriculum and a pedagogy for parallel courses in (a)linear algebra and(b)learning theory as applied to the study of mathematics with an emphasis on linear algebra.The purpose of the ongoing research,partially funded by the National Science Foundation,is to investigate how the parallel study of learning theories and advanced mathematics influences the development of thinking of individuals in both domains.The researchers found that the particular synergy afforded by the parallel study of math and learning theory promoted,in some students,a rich understanding of both domains and that had a mutually reinforcing effect.Furthermore,there is evidence that the deeper insights will contribute to more effective instruction by those who become high school math teachers and,consequently,better learning by their students.The courses developed were appropriate for mathematics majors,pre-service secondary mathematics teachers, and practicing mathematics teachers.The learning seminar focused most heavily on constructivist theories,although it also examinedThe work reported in this paper was partially supported by funding from the National Science Foundation(DUE CCLI 0442574).∗Corresponding author.Address:NDSU School of Education,NDSU Department of Mathematics,210F Family Life Center, NDSU Department#2625,P.O.Box6050,Fargo ND58105-6050,United States.Tel.:+17012317104;fax:+17012317416.E-mail addresses:william.martin@(W.Martin),sloch@(S.Loch),LCooley@ (L.Cooley),SDexter@(S.Dexter),dvidakovic@(D.Vidakovic).0024-3795/$-see front matter©2009Elsevier Inc.All rights reserved.doi:10.1016/a.2009.08.0302090W.Martin et al./Linear Algebra and its Applications432(2010)2089–2099Thematicized schema Triad–intraInterTransGenetic decomposition Vector additionMatrixMatrix multiplication Matrix representation BasisColumn spaceRow spaceNull space Eigenspace Transformation socio-cultural and historical perspectives.A particular theory, Action–Process–Object–Schema(APOS)[10],was emphasized and examined through the lens of studying linear algebra.APOS has been used in a variety of studies focusing on student understanding of undergraduate mathematics.The linear algebra courses include the standard set of undergraduate topics.This paper reports the re-sults of the learning theory seminar and its effects on students who were simultaneously enrolled in linear algebra and students who had previously completed linear algebra and outlines how prior research has influenced the future direction of the project.©2009Elsevier Inc.All rights reserved.1.Research rationaleThe research team of the Linear Algebra Project(LAP)developed and implemented a curriculum and a pedagogy for parallel courses in linear algebra and learning theory as applied to the study of math-ematics with an emphasis on linear algebra.The purpose of the research,which was partially funded by the National Science Foundation(DUE CCLI0442574),was to investigate how the parallel study of learning theories and advanced mathematics influences the development of thinking of high school mathematics teachers,in both domains.The researchers found that the particular synergy afforded by the parallel study of math and learning theory promoted,in some teachers,a richer understanding of both domains that had a mutually reinforcing effect and affected their thinking about their identities and practices as teachers.It has been observed that linear algebra courses often are viewed by students as a collection of definitions and procedures to be learned by rote.Scanning the table of contents of many commonly used undergraduate textbooks will provide a common list of terms such as listed here(based on linear algebra texts by Strang[1]and Lang[2]).Vector space Kernel GaussianIndependence Image TriangularLinear combination Inverse Gram–SchmidtSpan Transpose EigenvectorBasis Orthogonal Singular valueSubspace Operator DecompositionProjection Diagonalization LU formMatrix Normal form NormDimension Eignvalue ConditionLinear transformation Similarity IsomorphismRank Diagonalize DeterminantThis is not something unique to linear algebra–a similar situation holds for many undergraduate mathematics courses.Certainly the authors of undergraduate texts do not share this student view of mathematics.In fact,the variety ways in which different authors organize their texts reflects the individual ways in which they have conceptualized introductory linear algebra courses.The wide vari-ability that can be seen in a perusal of the many linear algebra texts that are used is a reflection the many ways that mathematicians think about linear algebra and their beliefs about how students can come to make sense of the content.Instruction in a course is based on considerations of content,pedagogy, resources(texts and other materials),and beliefs about teaching and learning of mathematics.The interplay of these ideas shaped our research project.We deliberately mention two authors with clearly differing perspectives on an undergraduate linear algebra course:Strang’s organization of the material takes an applied or application perspective,while Lang views the material from more of a“pure mathematics”perspective.A review of the wide variety of textbooks to classify and categorize the different views of the subject would reveal a broad variety of perspectives on the teaching of the subject.We have taken a view that seeks to go beyond the mathe-matical content to integrate current theoretical perspectives on the teaching and learning of undergrad-uate mathematics.Our project used integration of mathematical content,applications,and learningW.Martin et al./Linear Algebra and its Applications432(2010)2089–20992091 theories to provide enhanced learning experiences using rich content,student meta cognition,and their own experience and intuition.The project also used co-teaching and collaboration among faculty with expertise in a variety of areas including mathematics,computer science and mathematics education.If one moves beyond the organization of the content of textbooks wefind that at their heart they do cover a common core of the key ideas of linear algebra–all including fundamental concepts such as vector space and linear transformation.These observations lead to our key question“How is one to think about this task of organizing instruction to optimize learning?”In our work we focus on the conception of linear algebra that is developed by the student and its relationship with what we reveal about our own understanding of the subject.It seems that even in cases where researchers consciously study the teaching and learning of linear algebra(or other mathematics topics)the questions are“What does it mean to understand linear algebra?”and“How do I organize instruction so that students develop that conception as fully as possible?”In broadest terms, our work involves(a)simultaneous study of linear algebra and learning theories,(b)having students connect learning theories to their study of linear algebra,and(c)the use of parallel mathematics and education courses and integrated workshops.As students simultaneously study mathematics and learning theory related to the study of mathe-matics,we expect that reflection or meta cognition on their own learning will enable them to construct deeper and more meaningful understanding in both domains.We chose linear algebra for several reasons:It has not been the focus of as much instructional research as calculus,it involves abstraction and proof,and it is taken by many students in different programs for a variety of reasons.It seems to us to involve important mathematical content along with rich applications,with abstraction that builds on experience and intuition.In our pilot study we taught parallel courses:The regular upper division undergraduate linear algebra course and a seminar in learning theories in mathematics education.Early in the project we also organized an intensive three-day workshop for teachers and prospective teachers that included topics in linear algebra and examination of learning theory.In each case(two sets of parallel courses and the workshop)we had students reflect on their learning of linear algebra content and asked them to use their own learning experiences to reflect on the ideas about teaching and learning of mathematics.Students read articles–in the case of the workshop,this reading was in advance of the long weekend session–drawn from mathematics education sources including[3–10].APOS(Action,Process,Object,Schema)is a theoretical framework that has been used by many researchers who study the learning of undergraduate and graduate mathematics[10,11].We include a sketch of the structure of this framework and refer the reader to the literature for more detailed descriptions.More detailed and specific illustrations of its use are widely available[12].The APOS Theoretical Framework involves four levels of understanding that can be described for a wide variety of mathematical concepts such as function,vector space,linear transformation:Action,Process,Object (either an encapsulated process or a thematicized schema),Schema(Intra,inter,trans–triad stages of schema formation).Genetic decomposition is the analysis of a particular concept in which developing understanding is described as a dynamic process of mental constructions that continually develop, abstract,and enrich the structural organization of an individual’s knowledge.We believe that students’simultaneous study of linear algebra along with theoretical examination of teaching and learning–particularly on what it means to develop conceptual understanding in a domain –will promote learning and understanding in both domains.Fundamentally,this reflects our view that conceptual understanding in any domain involves rich mental connections that link important ideas or facts,increasing the individual’s ability to relate new situations and problems to that existing cognitive framework.This view of conceptual understanding of mathematics has been described by various prominent math education researchers such as Hiebert and Carpenter[6]and Hiebert and Lefevre[7].2.Action–Process–Object–Schema theory(APOS)APOS theory is a theoretical perspective of learning based on an interpretation of Piaget’s construc-tivism and poses descriptions of mental constructions that may occur in understanding a mathematical concept.These constructions are called Actions,Processes,Objects,and Schema.2092W.Martin et al./Linear Algebra and its Applications432(2010)2089–2099 An action is a transformation of a mathematical object according to an explicit algorithm seen as externally driven.It may be a manipulation of objects or acting upon a memorized fact.When one reflects upon an action,constructing an internal operation for a transformation,the action begins to be interiorized.A process is this internal transformation of an object.Each step may be described or reflected upon without actually performing it.Processes may be transformed through reversal or coordination with other processes.There are two ways in which an individual may construct an object.A person may reflect on actions applied to a particular process and become aware of the process as a totality.One realizes that transformations(whether actions or processes)can act on the process,and is able to actually construct such transformations.At this point,the individual has reconstructed a process as a cognitive object. In this case we say that the process has been encapsulated into an object.One may also construct a cognitive object by reflecting on a schema,becoming aware of it as a totality.Thus,he or she is able to perform actions on it and we say the individual has thematized the schema into an object.With an object conception one is able to de-encapsulate that object back into the process from which it came, or,in the case of a thematized schema,unpack it into its various components.Piaget and Garcia[13] indicate that thematization has occurred when there is a change from usage or implicit application to consequent use and conceptualization.A schema is a collection of actions,processes,objects,and other previously constructed schemata which are coordinated and synthesized to form mathematical structures utilized in problem situations. Objects may be transformed by higher-level actions,leading to new processes,objects,and schemata. Hence,reconstruction continues in evolving schemata.To illustrate different conceptions of the APOS theory,imagine the following’teaching’scenario.We give students multi-part activities in a technology supported environment.In particular,we assume students are using Maple in the computer lab.The multi-part activities,focusing on vectors and operations,in Maple begin with a given Maple code and drawing.In case of scalar multiplication of the vector,students are asked to substitute one parameter in the Maple code,execute the code and observe what has happened.They are asked to repeat this activity with a different value of the parameter.Then students are asked to predict what will happen in a more general case and to explain their reasoning.Similarly,students may explore addition and subtraction of vectors.In the next part of activity students might be asked to investigate about the commutative property of vector addition.Based on APOS theory,in thefirst part of the activity–in which students are asked to perform certain operation and make observations–our intention is to induce each student’s action conception of that concept.By asking students to imagine what will happen if they make a certain change–but do not physically perform that change–we are hoping to induce a somewhat higher level of students’thinking, the process level.In order to predict what will happen students would have to imagine performing the action based on the actions they performed before(reflective abstraction).Activities designed to explore on vector addition properties require students to encapsulate the process of addition of two vectors into an object on which some other action could be performed.For example,in order for a student to conclude that u+v=v+u,he/she must encapsulate a process of adding two vectors u+v into an object(resulting vector)which can further be compared[action]with another vector representing the addition of v+u.As with all theories of learning,APOS has a limitation that researchers may only observe externally what one produces and discusses.While schemata are viewed as dynamic,the task is to attempt to take a snap shot of understanding at a point in time using a genetic decomposition.A genetic decomposition is a description by the researchers of specific mental constructions one may make in understanding a mathematical concept.As with most theories(economics,physics)that have restrictions,it can still be very useful in describing what is observed.3.Initial researchIn our preliminary study we investigated three research questions:•Do participants make connections between linear algebra content and learning theories?•Do participants reflect upon their own learning in terms of studied learning theories?W.Martin et al./Linear Algebra and its Applications432(2010)2089–20992093•Do participants connect their study of linear algebra and learning theories to the mathematics content or pedagogy for their mathematics teaching?In addition to linear algebra course activities designed to engage students in explorations of concepts and discussions about learning theories and connections between the two domains,we had students construct concept maps and describe how they viewed the connections between the two subjects. We found that some participants saw significant connections and were able to apply APOS theory appropriately to their learning of linear algebra.For example,here is a sketch outline of how one participant described the elements of the APOS framework late in the semester.The student showed a reasonable understanding of the theoretical framework and then was able to provide an example from linear algebra to illustrate the model.The student’s description of the elements of APOS:Action:“Students’approach is to apply‘external’rules tofind solutions.The rules are said to be external because students do not have an internalized understanding of the concept or the procedure tofind a solution.”Process:“At the process level,students are able to solve problems using an internalized understand-ing of the algorithm.They do not need to write out an equation or draw a graph of a function,for example.They can look at a problem and understand what is going on and what the solution might look like.”Object level as performing actions on a process:“At the object level,students have an integrated understanding of the processes used to solve problems relating to a particular concept.They un-derstand how a process can be transformed by different actions.They understand how different processes,with regard to a particular mathematical concept,are related.If a problem does not conform to their particular action-level understanding,they can modify the procedures necessary tofind a solution.”Schema as a‘set’of knowledge that may be modified:“Schema–At the schema level,students possess a set of knowledge related to a particular concept.They are able to modify this set of knowledge as they gain more experience working with the concept and solving different kinds of problems.They see how the concept is related to other concepts and how processes within the concept relate to each other.”She used the ideas of determinant and basis to illustrate her understanding of the framework. (Another student also described how student recognition of the recursive relationship of computations of determinants of different orders corresponded to differing levels of understanding in the APOS framework.)Action conception of determinant:“A student at the action level can use an algorithm to calculate the determinant of a matrix.At this level(at least for me),the formula was complicated enough that I would always check that the determinant was correct byfinding the inverse and multiplying by the original matrix to check the solution.”Process conception of determinant:“The student knows different methods to use to calculate a determinant and can,in some cases,look at a matrix and determine its value without calculations.”Object conception:“At the object level,students see the determinant as a tool for understanding and describing matrices.They understand the implications of the value of the determinant of a matrix as a way to describe a matrix.They can use the determinant of a matrix(equal to or not equal to zero)to describe properties of the elements of a matrix.”Triad development of a schema(intra,inter,trans):“A singular concept–basis.There is a basis for a space.The student can describe a basis without calculation.The student canfind different types of bases(column space,row space,null space,eigenspace)and use these values to describe matrices.”The descriptions of components of APOS along with examples illustrate that this student was able to make valid connections between the theoretical framework and the content of linear algebra.While the2094W.Martin et al./Linear Algebra and its Applications432(2010)2089–2099descriptions may not match those that would be given by scholars using APOS as a research framework, the student does demonstrate a recognition of and ability to provide examples of how understanding of linear algebra can be organized conceptually as more that a collection of facts.As would be expected,not all participants showed gains in either domain.We viewed the results of this study as a proof of concept,since there were some participants who clearly gained from the experience.We also recognized that there were problems associated with the implementation of our plan.To summarize ourfindings in relation to the research questions:•Do participants make connections between linear algebra content and learning theories?Yes,to widely varying degrees and levels of sophistication.•Do participants reflect upon their own learning in terms of studied learning theories?Yes,to the extent possible from their conception of the learning theories and understanding of linear algebra.•Do participants connect their study of linear algebra and learning theories to the mathematics content or pedagogy for their mathematics teaching?Participants describe how their experiences will shape their own teaching,but we did not visit their classes.Of the11students at one site who took the parallel courses,we identified three in our case studies (a detailed report of that study is presently under review)who demonstrated a significant ability to connect learning theories with their own learning of linear algebra.At another site,three teachers pursuing math education graduate studies were able to varying degrees to make these connections –two demonstrated strong ability to relate content to APOS and described important ways that the experience had affected their own thoughts about teaching mathematics.Participants in the workshop produced richer concept maps of linear algebra topics by the end of the weekend.Still,there were participants who showed little ability to connect material from linear algebra and APOS.A common misunderstanding of the APOS framework was that increasing levels cor-responded to increasing difficulty or complexity.For example,a student might suggest that computing the determinant of a2×2matrix was at the action level,while computation of a determinant in the 4×4case was at the object level because of the increased complexity of the computations.(Contrast this with the previously mentioned student who observed that the object conception was necessary to recognize that higher dimension determinants are computed recursively from lower dimension determinants.)We faced more significant problems than the extent to which students developed an understanding of the ideas that were presented.We found it very difficult to get students–especially undergraduates –to agree to take an additional course while studying linear algebra.Most of the participants in our pilot projects were either mathematics teachers or prospective mathematics teachers.Other students simply do not have the time in their schedules to pursue an elective seminar not directly related to their own area of interest.This problem led us to a new project in which we plan to integrate the material on learning theory–perhaps implicitly for the students–in the linear algebra course.Our focus will be on working with faculty teaching the course to ensure that they understand the theory and are able to help ensure that course activities reflect these ideas about learning.4.Continuing researchOur current Linear Algebra in New Environments(LINE)project focuses on having faculty work collaboratively to develop a series of modules that use applications to help students develop conceptual understanding of key linear algebra concepts.The project has three organizing concepts:•Promote enhanced learning of linear algebra through integrated study of mathematical content, applications,and the learning process.•Increase faculty understanding and application of mathematical learning theories in teaching linear algebra.•Promote and support improved instruction through co-teaching and collaboration among faculty with expertise in a variety of areas,such as education and STEM disciplines.W.Martin et al./Linear Algebra and its Applications432(2010)2089–20992095 For example,computer and video graphics involve linear transformations.Students will complete a series of activities that use manipulation of graphical images to illustrate and help them move from action and process conceptions of linear transformations to object conceptions and the development of a linear transformation schema.Some of these ideas were inspired by material in Judith Cederberg’s geometry text[14]and some software developed by David Meel,both using matrix representations of geometric linear transformations.The modules will have these characteristics:•Embed learning theory in linear algebra course for both the instructor and the students.•Use applied modules to illustrate the organization of linear algebra concepts.•Applications draw on student intuitions to aid their mental constructions and organization of knowledge.•Consciously include meta-cognition in the course.To illustrate,we sketch the outline of a possible series of activities in a module on geometric linear transformations.The faculty team–including individuals with expertise in mathematics,education, and computer science–will develop a series of modules to engage students in activities that include reflection and meta cognition about their learning of linear algebra.(The Appendix contains a more detailed description of a module that includes these activities.)Task1:Use Photoshop or GIMP to manipulate images(rotate,scale,flip,shear tools).Describe and reflect on processes.This activity uses an ACTION conception of transformation.Task2:Devise rules to map one vector to another.Describe and reflect on process.This activity involves both ACTION and PROCESS conceptions.Task3:Use a matrix representation to map vectors.This requires both PROCESS and OBJECT conceptions.Task4:Compare transform of sum with sum of transforms for matrices in Task3as compared to other non-linear functions.This involves ACTION,PROCESS,and OBJECT conceptions.Task5:Compare pre-image and transformed image of rectangles in the plane–identify software tool that was used(from Task1)and how it might be represented in matrix form.This requires OBJECT and SCHEMA conceptions.Education,mathematics and computer science faculty participating in this project will work prior to the semester to gain familiarity with the APOS framework and to identify and sketch potential modules for the linear algebra course.During the semester,collaborative teams of faculty continue to develop and refine modules that reflect important concepts,interesting applications,and learning theory:Modules will present activities that help students develop important concepts rather than simply presenting important concepts for students to absorb.The researchers will study the impact of project activities on student learning:We expect that students will be able to describe their knowledge of linear algebra in a more conceptual(structured) way during and after the course.We also will study the impact of the project on faculty thinking about teaching and learning:As a result of this work,we expect that faculty will be able to describe both the important concepts of linear algebra and how those concepts are mentally developed and organized by students.Finally,we will study the impact on instructional practice:Participating faculty should continue to use instructional practices that focus both on important content and how students develop their understanding of that content.5.SummaryOur preliminary study demonstrated that prospective and practicing mathematics teachers were able to make connections between their concurrent study of linear algebra and of learning theories relating to mathematics education,specifically the APOS theoretical framework.In cases where the participants developed understanding in both domains,it was apparent that this connected learning strengthened understanding in both areas.Unfortunately,we were unable to encourage undergraduate students to consider studying both linear algebra and learning theory in separate,parallel courses. Consequently,we developed a new strategy that embeds the learning theory in the linear algebra。
Linear Algebra_彭国华_第五章课后答案
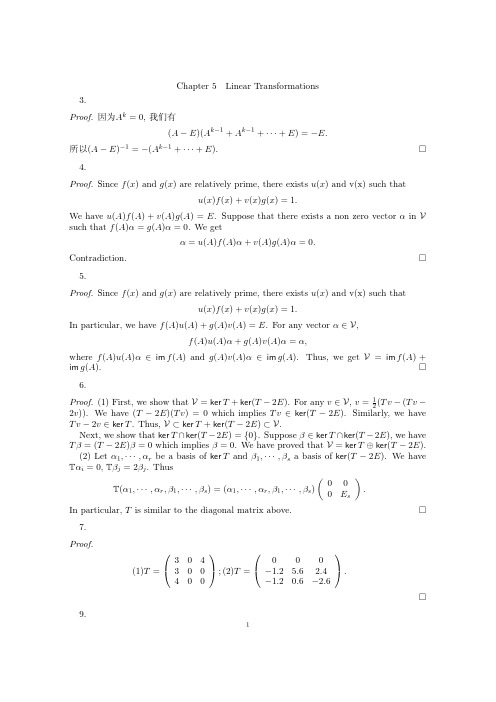
Introduction to Linear Algebra
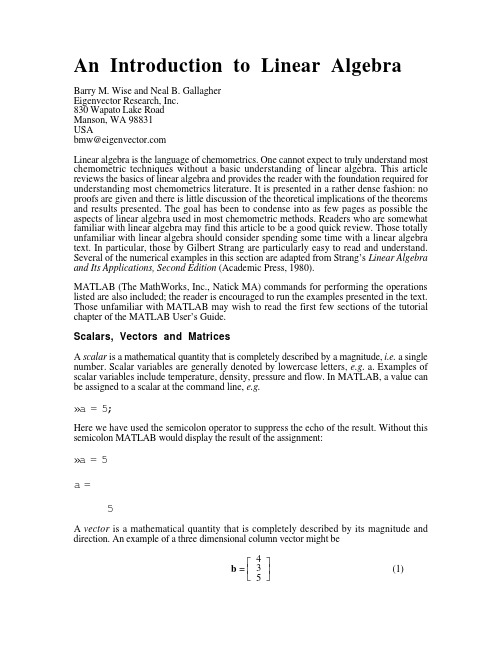
»a = 5 a= 5
A vector is a mathematical quantity that is completely described by its magnitude and direction. An example of a three dimensional column vector might be 4 b= 3 5 uld easily assign bT to another variable c, as follows:
»c = b' c= 4 3 5
A matrix is a rectangular array of scalars, or in some instances, algebraic expressions which evaluate to scalars. Matrices are said to be m by n, where m is the number of rows in the matrix and n is the number of columns. A 3 by 4 matrix is shown here 2 A= 7 5 5 3 2 3 2 0 6 1 3 (3)
»a = 5;
Here we have used the semicolon operator to suppress the echo of the result. Without this semicolon MATLAB would display the result of the assignment:
»A(2,4) ans = 1
The transpose operator “flips” a matrix along its diagonal elements, creating a new matrix with the ith row being equal to the jth column of the original matrix, e.g. T A = 2 5 3 6 7 3 2 1 5 2 0 3
全秩分解和佛兰德定理
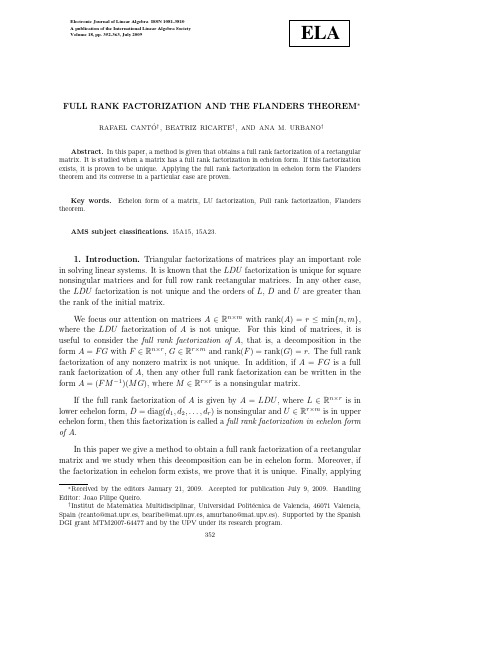
2. Quasi-Gauss elimination process. It is known that the Gauss elimination process consists of producing zeros in a column of a matrix by adding to each row an appropriate multiple of a fixed row, and the Neville elimination method obtains the zeros in a column by adding to each row an appropriate multiple of the previous one. In both processes, reordering of rows may be necessary. In this sense, the Gauss elimination method can be considered more general than the Neville elimination process, because if the Neville process with no pivoting can be applied, then the Gauss process with no pivoting can also be applied, but the converse is not true in general.
When we can apply the Gauss elimination process with no pivoting to a singular matrix, the factorization obtained is not unique and it is not a full rank factorization. Therefore, in this paper we consider a new method which allows us to obtain a full rank factorization of a singular matrix. This method, which we call quasi-Gauss elimination process, is based on the Gaussian and the quasi-Neville elimination [5].
LinearAlgebraStrang4thSolutionManual
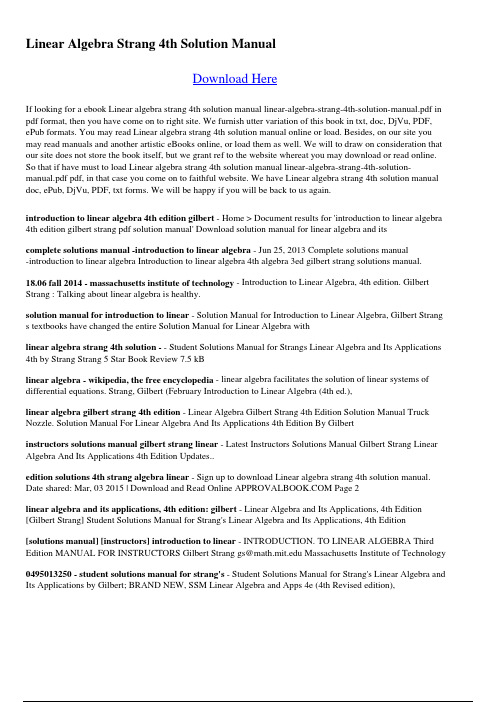
Linear Algebra Strang 4th Solution ManualDownload HereIf looking for a ebook Linear algebra strang 4th solution manual linear-algebra-strang-4th-solution-manual.pdf in pdf format, then you have come on to right site. We furnish utter variation of this book in txt, doc, DjVu, PDF, ePub formats. You may read Linear algebra strang 4th solution manual online or load. Besides, on our site you may read manuals and another artistic eBooks online, or load them as well. We will to draw on consideration that our site does not store the book itself, but we grant ref to the website whereat you may download or read online. So that if have must to load Linear algebra strang 4th solution manual linear-algebra-strang-4th-solution-manual.pdf pdf, in that case you come on to faithful website. We have Linear algebra strang 4th solution manual doc, ePub, DjVu, PDF, txt forms. We will be happy if you will be back to us again.introduction to linear algebra 4th edition gilbert - Home > Document results for 'introduction to linear algebra 4th edition gilbert strang pdf solution manual' Download solution manual for linear algebra and itscomplete solutions manual -introduction to linear algebra - Jun 25, 2013 Complete solutions manual-introduction to linear algebra Introduction to linear algebra 4th algebra 3ed gilbert strang solutions manual.18.06 fall 2014 - massachusetts institute of technology - Introduction to Linear Algebra, 4th edition. Gilbert Strang : Talking about linear algebra is healthy.solution manual for introduction to linear - Solution Manual for Introduction to Linear Algebra, Gilbert Strang s textbooks have changed the entire Solution Manual for Linear Algebra withlinear algebra strang 4th solution - - Student Solutions Manual for Strangs Linear Algebra and Its Applications 4th by Strang Strang 5 Star Book Review 7.5 kBlinear algebra - wikipedia, the free encyclopedia - linear algebra facilitates the solution of linear systems of differential equations. Strang, Gilbert (February Introduction to Linear Algebra (4th ed.),linear algebra gilbert strang 4th edition - Linear Algebra Gilbert Strang 4th Edition Solution Manual Truck Nozzle. Solution Manual For Linear Algebra And Its Applications 4th Edition By Gilbertinstructors solutions manual gilbert strang linear - Latest Instructors Solutions Manual Gilbert Strang Linear Algebra And Its Applications 4th Edition Updates..edition solutions 4th strang algebra linear - Sign up to download Linear algebra strang 4th solution manual. Date shared: Mar, 03 2015 | Download and Read Online Page 2linear algebra and its applications, 4th edition: gilbert - Linear Algebra and Its Applications, 4th Edition [Gilbert Strang] Student Solutions Manual for Strang's Linear Algebra and Its Applications, 4th Edition [solutions manual] [instructors] introduction to linear - INTRODUCTION. TO LINEAR ALGEBRA Third***************************************************.eduMassachusettsInstituteofTechnology 0495013250 - student solutions manual for strang's - Student Solutions Manual for Strang's Linear Algebra and Its Applications by Gilbert; BRAND NEW, SSM Linear Algebra and Apps 4e (4th Revised edition),introduction to linear algebra 4th solution | - Tricia Joy. Register; Terms Sponsored High Speed Downloads introduction to linear algebra 4th edition solution manual Introduction To Linear Algebra Gilbertgilbert strang introduction to linear algebra 4th - Gilbert Strang Introduction To Linear Algebra 4th Edition Solutions Manual Pdf downloads Linear Algebra Strang 4th Solution Manual. Linear Algebra Gilbert Strangstudent solutions manual for strang's linear - : Student Solutions Manual for Strang's Linear Algebra and Its Applications, 4thstudent solutions manual for linear algebra and its - Student Solutions Manual for Linear Algebra and Its Applications Linear Algebra and Its Applications, 4th Editionintroduction to linear algebra, 4th edition - mit mathematics - Introduction to Linear Algebra, 4th I hope this website will become a valuable resource for everyone learning and doing linear algebra. 1.1 Vectors and Linear linear algebra and its applications 4th edition textbook - Access Linear Algebra and Its Applications 4th Edition solutions now. Linear Algebra and Its Applications | 4th Edition. Solutions Manual; Scholarships;introduction to linear algebra 4th edition - Access Introduction to Linear Algebra 4th Edition solutions now. Our solutions are written by Chegg experts so you can be assured of the Solutions Manual;linear algebra strang solutions manual 4th - Tricia's Compilation for 'linear algebra strang solutions manual 4th instructor' Follow. solutions manual to Linear Algebra, 4th Filetype: Submitter:gilbert strang linear algebra 4th edition - Gilbert Strang Linear Algebra 4th Edition Solutions Truck Nozzle. GILBERT STRANG LINEAR ALGEBRA 4TH EDITION SOLUTIONS. DOWNLOAD: GILBERT STRANG LINEAR ALGEBRA 4TH EDITIONfree! solution manual of linear algebra by gilbert - Download solution manual of linear algebra by gilbert strang 4th edition ebooks and manuals at PdfDigest: For: Solution manual of linear algebra by gilber introduction to linear algebra 4th edition by - Introduction to Linear Algebra 4th Edition by Gilbert Strang fully written solutions / or book Introduction to Linear Algebra 4th Edition bylinear algebra and its applications, 4th edition - Renowned professor and author Gilbert Strang demonstrates that linear algebra is a Linear Algebra and Its Applications, Student Solutions Manualinstructor's solutions manual for strang's linear algebra and - schema:name " Instructor's solutions manual for Strang's Linear algebra and its applications, fourth edition "@en; schema:productID " 85780336" ;student solutions manual for strang 's linear algebra and its - Student Solutions Manual for Strang's Linear Algebra and Its Applications, 4th 4 edition Published October 1, 2005 byneed a solutions manual-- linear algebra and its - Oct 07, 2009 Need a solutions manual--Linear Algebra and Its Applications, 4th Ed, by Gilbert Strang?solutions manual instructors introduction to - Instructor S Solutions Manual For Strang S Linear Algebra And Its Applications rapidshare links Strang Introduction Linear Algebra 4th Edition Solution ManualRelated PDFs:1991 yamaha yz125 service manual, briggs and stratton 18 hp ic manuals, math foundations 11 study guide, solution guide management accounting 6e, e6b flight manual, 06 ktm 250 xcw manual, haas vf2 service manual, awwa manual m 51, kia rio car manual, isuzu fvr 1000 manual, hayward abg 100 manual, iq 2020 control box manual, junior maths 3 by a dasgupta manual, 61h booster relay manual, joseph topich chemistry solutionsmanual 6th edition, science a closer look pacing guide, wisconsin civil service exam study guide maintenance, honda civic 1995 1996 1997 98 1999 workshop manual download, principles of macroeconomics 5th edition study guide, owners manual for mini chopper motorcycle, harley fxwg manual, ford transit mini bus manual, 97 cavalier haynes repair manual, peugeot 505 workshop manual, golf manual derkeiler com, repair manual kawasaki ninja 250r, nrx 1800 service manual, bait of satan leaders guide, buick lacrosse manual, does northstar study guide work, salvation army pricing guide, d3306 caterpillar operation manual, ford expedition factory service manual, manual for suzuki rm85, navigation manual 2015 crv, vw golf v5 manual, 1999 kawasaki nomad manual, mercedes c 180 workshop manual, honda crv 2015 factory manual, colt 1903 pocket hammerless manual。
introduction to linear algebra 每章开头方框-概述说明以及解释
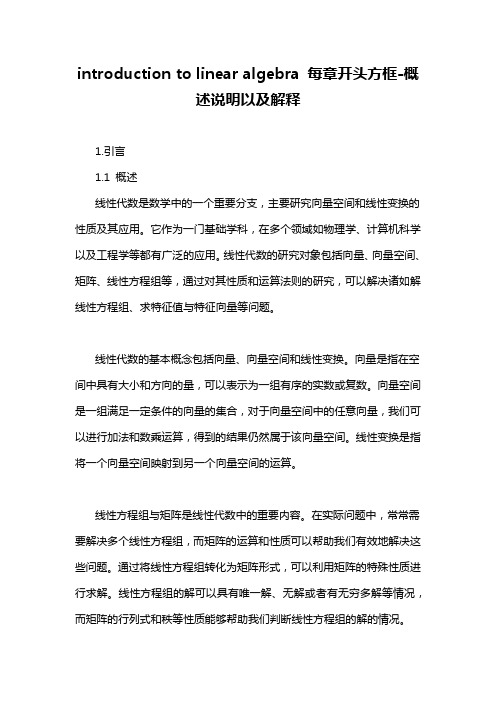
introduction to linear algebra 每章开头方框-概述说明以及解释1.引言1.1 概述线性代数是数学中的一个重要分支,主要研究向量空间和线性变换的性质及其应用。
它作为一门基础学科,在多个领域如物理学、计算机科学以及工程学等都有广泛的应用。
线性代数的研究对象包括向量、向量空间、矩阵、线性方程组等,通过对其性质和运算法则的研究,可以解决诸如解线性方程组、求特征值与特征向量等问题。
线性代数的基本概念包括向量、向量空间和线性变换。
向量是指在空间中具有大小和方向的量,可以表示为一组有序的实数或复数。
向量空间是一组满足一定条件的向量的集合,对于向量空间中的任意向量,我们可以进行加法和数乘运算,得到的结果仍然属于该向量空间。
线性变换是指将一个向量空间映射到另一个向量空间的运算。
线性方程组与矩阵是线性代数中的重要内容。
在实际问题中,常常需要解决多个线性方程组,而矩阵的运算和性质可以帮助我们有效地解决这些问题。
通过将线性方程组转化为矩阵形式,可以利用矩阵的特殊性质进行求解。
线性方程组的解可以具有唯一解、无解或者有无穷多解等情况,而矩阵的行列式和秩等性质能够帮助我们判断线性方程组的解的情况。
向量空间与线性变换是线性代数的核心内容。
向量空间的性质研究可以帮助我们理解向量的运算和性质,以及解释向量空间的几何意义。
线性变换是一种将一个向量空间映射到另一个向量空间的运算,通过线性变换可以将复杂的向量运算问题转化为简单的矩阵运算问题。
在线性变换中,我们需要关注其核、像以及变换的特征等性质,这些性质可以帮助我们理解线性变换的本质和作用。
综上所述,本章节将逐步介绍线性代数的基本概念、线性方程组与矩阵、向量空间与线性变换的相关内容。
通过深入学习和理解这些内容,我们能够掌握线性代数的基本原理和应用,为进一步研究更高级的线性代数问题打下坚实的基础。
1.2文章结构在文章结构部分,我们将介绍本文的组织结构和各章节的内容概述。
代数英语

(0,2) 插值||(0,2) interpolation0#||zero-sharp; 读作零井或零开。
0+||zero-dagger; 读作零正。
1-因子||1-factor3-流形||3-manifold; 又称“三维流形”。
AIC准则||AIC criterion, Akaike information criterionAp 权||Ap-weightA稳定性||A-stability, absolute stabilityA最优设计||A-optimal designBCH 码||BCH code, Bose-Chaudhuri-Hocquenghem codeBIC准则||BIC criterion, Bayesian modification of the AICBMOA函数||analytic function of bounded mean oscillation; 全称“有界平均振动解析函数”。
BMO鞅||BMO martingaleBSD猜想||Birch and Swinnerton-Dyer conjecture; 全称“伯奇与斯温纳顿-戴尔猜想”。
B样条||B-splineC*代数||C*-algebra; 读作“C星代数”。
C0 类函数||function of class C0; 又称“连续函数类”。
CA T准则||CAT criterion, criterion for autoregressiveCM域||CM fieldCN 群||CN-groupCW 复形的同调||homology of CW complexCW复形||CW complexCW复形的同伦群||homotopy group of CW complexesCW剖分||CW decompositionCn 类函数||function of class Cn; 又称“n次连续可微函数类”。
Cp统计量||Cp-statisticC。
线性代数-第一章第2节-矩阵的运算
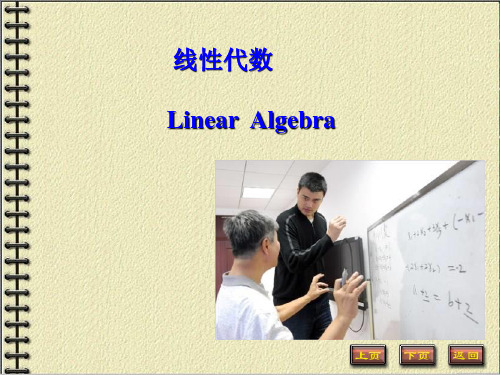
四、矩阵的转置
1. 定义
将矩阵 A m×n 的行换成同序数的列,列 换成同序数的行所得的 n×m 矩阵称为 A的转置矩阵,记作 AT 或 A'。
例如: A 1 0 2
4 3 0
则
AT
1 0
4 3
2 0
2)、转置矩阵的运算性质
1 AT T A;
2 A BT AT BT ;
阵,且HH T E.
证明 HT E 2XXT T ET 2 XXT T
E 2XXT H , H是对称矩阵.
HH T H 2 E 2XX T 2 E 4XXT 4 XXT XXT E 4XXT 4X XT X XT
E 4XX T 4XX T E.
1.55 2.1 2.6
C (cik )32, A (aij )32, B (bjk )22
•而
2
cik aijbjk j 1
• (即A的第i行与B的第k列对应相乘再相加)
三、矩阵与矩阵相乘 定义 设 A = ( aij ) m×s , B = ( bij ) s×n ,
则 A 与 B 的乘积 C=AB = ( cij ) m×n
A
a21
a22
am1
am 2
a1n
a2n
amn
b11 b12
B
b21
b22
bm1 bm2
b1n
b2n
bmn
a11 b11
A
B
a21
b21
am1 bm1
a12 b12 a22 b22
am2 bm2
a1n b1n
a2n
b2n
amn
bmn
说明 只有当两个矩阵是同型矩阵时,才能进 行加法运算.
- 1、下载文档前请自行甄别文档内容的完整性,平台不提供额外的编辑、内容补充、找答案等附加服务。
- 2、"仅部分预览"的文档,不可在线预览部分如存在完整性等问题,可反馈申请退款(可完整预览的文档不适用该条件!)。
- 3、如文档侵犯您的权益,请联系客服反馈,我们会尽快为您处理(人工客服工作时间:9:00-18:30)。
Parallelizing Dense Linear Algebra Operationswith Task Queues in llcAntonio J.Dorta1,Jos´e M.Bad´ıa2,Enrique S.Quintana-Ort´ı2,andFrancisco de Sande11Depto.de Estad´ıstica,Investigaci´o n Operativa y Computaci´o nUniversidad de La Laguna,38271–La Laguna,Spain{ajdorta,fsande}@ull.es2Depto.de Ingenier´ıa y Ciencia de ComputadoresUniversidad Jaume I,12.071–Castell´o n,Spain{badia,quintana}@icc.uji.esAbstract.llc is a language based on C where parallelism is expressedusing compiler directives.The llc compiler produces MPI code whichcan be ported to both shared and distributed memory systems.In this work we focus our attention in the llc implementation of theWorkqueuing Model.This model is an extension of the OpenMP stan-dard that allows an elegant implementation of irregular parallelism.Weevaluate our approach by comparing the OpenMP and llc paralleliza-tions of the symmetric rank-k update operation on shared and distributedmemory parallel platforms.KeywordsMPI,OpenMP,Workqueuing,cluster computing,distributed memory.1IntroductionThe advances in high performance computing(HPC)hardware have not been followed by the software.The tools used to express parallel computations are nowadays one of the major obstacles for the massive use of HPC technology. Two of these tools are MPI[1]and OpenMP[2].Key advantages of MPI are its portability and efficiency,with the latter strongly influenced by the control given to the programmer of the parallel application.However,a deep knowledge of low-level aspects of parallelism(communications,synchronizations,etc.)is needed in order to develop an efficient MPI parallel application.On the other hand,OpenMP allows a much easier implementation.One can start from a sequential code and parallelize it incrementally by adding compiler directives to specific regions of the code.An additional advantage is that it This work has been partially supported by the EC(FEDER)and the Spanish MEC (Plan Nacional de I+D+I,TIN2005-09037-C02).follows the sequential semantic of the program.The main drawback of OpenMP is that it only targets shared memory architectures.As an alternative to MPI and OpenMP,we have designed llc[3]to exploit the best features of both approaches.llc shares the simplicity of OpenMP:we can start from a sequential code and parallelize it incrementally using OpenMP and/or llc directives and clauses.The code annotated with parallel directives is compiled by llCoMP,the llc compiler-translator,which produces an efficient and portable MPI parallel source code,valid for both shared and distributed memory architectures.An additional advantage of llc is that all the OpenMP directives and clauses are recognized by llCoMP.Therefore,we have three versions in the same code:sequential,OpenMP and llc/MPI,and we only need to choose the proper compiler to obtain the appropriate binary.Different directives have been designed in llc to support common parallel constructs in the past as forall,sections,and pipelines[4,5].In previous studies[4] we have investigated the implementation of Task Queues in llc.In this paper we focus our attention in the last feature added to llc:the support for the Workqueuing Model using Task Queues[6].In order to do so,we explore the possibilities of parallelizing(dense)linear algebra operations,as developed in the frame of the FLAME(Formal Linear Algebra Method Environment)project[7].The rest of the paper is organized as follows.In Section2we present the symmetric rank-k update(SYRK)operation as well as a FLAME code for its computation.Section3reviews the parallelization of this code using OpenMP and llc.Experimental results for both OpenMP and llc codes are reported and discussed in Section4.Finally,Section5offers some concluding remarks and hints on future research.2The SYRK operationThe SYRK operation is one of the Basic Linear Algebra Subprograms(BLAS) [8]most often used.It plays an important role,e.g.,in the formation of the normal equations in linear least-squares problems and the solution of symmetric positive definite linear systems via the Cholesky factorization[9].The operation computes the lower(or upper)triangular part of the result of the matrix product C:=βC+αAA T,where C is an m×m symmetric matrix,A is an m×k matrix, andα,βare scalars.Listing1presents the FLAME code for the SYRK operation[10].The par-titioning routines(FLA Part x,FLA Repart x to y and FLA Cont with x to y) are indexing operations that identify regions(blocks)into the matrices but do not modify their contents.Thus,e.g.,the invocation to FLA Part2x1in lines7–8“divides”matrix(object)A into two submatrices(blocks/objects),AT and AB, with thefirst one having0rows.Then,at each iteration of the loop,certain oper-ations are performed with the elements in these submatrices(routines FLA Gemm and FLA Syrk).More details can be consulted in[7].1int F L A_S y r k_l n_b l k_v a r1_s e q(F L A_O b j alpha,F L A_O b j A,2F L A_O b j beta,F L A_O b j C,int n b_a l g){3F L A_O b j AT,AB,CTL,CBL,CTR,CBR,4A0,A1,A2,C00,C01,C02,C10,C11,C12,C20,C21,C22;5int b;7F L A_P a r t_2x1(A,&AT,8&AB,0,F L A_T O P);9F L A_P a r t_2x2(C,&CTL,&CTR,10&CBL,&CBR,0,0,F L A_T L);12while(F L A_O b j_l e n g t h(AT)<F L A_O b j_l e n g t h(A)){13b=min(F L A_O b j_l e n g t h(AB),n b_a l g);14F L A_R e p a r t_2x1_t o_3x1(AT,&A0,15&A1,16AB,&A2,b,F L A_B O T T O M);17F L A_R e p a r t_2x2_t o_3x3(CTL,CTR,&C00,&C01,&C02,18&C10,&C11,&C12,19CBL,CBR,&C20,&C21,&C22,b,b,F L A_B R);20/∗−−−−−−−−−−−−−−−−−−−−−−−−−−−−−−−−−−−−−−−−−−−−−−−−−−−−−∗/21/∗C10:=C10+A1∗A0’∗/22F L A_G e m m(F L A_N O_T R A N S P O S E,F L A_T R A N S P O S E,alpha,A1,A0,23beta,C10,n b_a l g);24/∗C11:=C11+A1∗A1’∗/25F L A_S y r k(F L A_L O W E R_T R I A N G U L A R,F L A_N O_T R A N S P O S E,alpha,A1,26beta,C11,n b_a l g); 27/∗−−−−−−−−−−−−−−−−−−−−−−−−−−−−−−−−−−−−−−−−−−−−−−−−−−−−−∗/28F L A_C o n t_w i t h_3x1_t o_2x1(&AT,A0,29A1,30&AB,A2,F L A_T O P);31F L A_C o n t_w i t h_3x3_t o_2x2(&CTL,&CTR,C00,C01,C02,32C10,C11,C12,33&CBL,&CBR,C20,C21,C22,F L A_T L);34}35return F L A_S U C C E S S;36}Listing1.FLAME code for the SYRK operation3Parallelization of the SYRK operationA remarkable feature of FLAME is its capability for hiding intricate indexing inlinear algebra computations.However,this feature is a drawback for the tradi-tional OpenMP method to obtain parallelism from a sequential code,based on exploiting the parallelism of for loops.Thus,the OpenMP approach requires loop indexes for expressing parallelism which are not available in FLAME codes.Task Queues[6]have been proposed for adoption in OpenMP3.0and are currently supported by the Intel OpenMP compilers.Their use allows an elegant implementation of loops when the space iteration is not known in advance or, as in the case of FLAME code,when explicit indexing is to be avoided.3.1OpenMP parallelizationThe parallelization of the SYRK operation using the Intel implementation of Task Queues is described in[10].The Intel extension provides two directives to spec-ify tasks queues.The omp parallel taskq directive specifies a parallel regionwhere tasks can appear.Each task found in this region will be queued for later computation.The omp task identifies the tasks.For the SYRK operation,thefirst clause is used to mark the while loop(line12in Listing1),while the second one identifies the invocations to FLA Gemm and FLA Syrk as tasks(lines21–26in Listing1).Listing2shows the parallelization using taskq of the loop in the 1#p r a g m a i n t e l omp p a r a l l e l t a s k q{2while(F L A_O b j_l e n g t h(AT)<F L A_O b j_l e n g t h(A)){3...4#p r a g m a i n t e l omp t a s k c a p t u r e p r i v a t e(A0,A1,C10,C11){5/∗C10:=C10+A1∗A0’∗/6F L A_G e m m(F L A_N O_T R A N S P O S E,F L A_T R A N S P O S E,alpha,A1,A0,7beta,C10,n b_a l g);8/∗C11:=C11+A1∗A1’∗/9F L A_S y r k(F L A_L O W E R_T R I A N G U L A R,F L A_N O_T R A N S P O S E,alpha,A1,10beta,C11,n b_a l g); 11}12...13}14}Listing2.FLAME code for the SYRK operation parallelized using OpenMP FLAME code for the SYRK operation.The directive omp task that appears in line4is used to identify the tasks.Function calls to FLA Gemm and FLA Syrk are in the scope of the taskq directive in line1and,therefore,a new task that computes both functions is created at each iteration of the loop.Thefirst of these functions computes C10:=C10+A1A T0,while the second one computes C11:=C11+A1A T1.All the variables involved in these computations have to be private to each thread(A0,A1,C10,and C11),ant thus they must be copied to each thread during execution time.The captureprivate clause that comple-ments the omp parallel task directive serves this purpose.3.2llc parallelizationIn this section we illustrate the use of llc to parallelize the SYRK code.Further information about the effective translation of the directives in the code to MPI can be found in[4].The parallelization using llc resembles that carried out using OpenMP,with a few differences that are illustrated in the following.After identifying the task code,we annotate the regions using llc and/or OpenMP di-rectives.All the OpenMP directives and clauses are accepted by llCoMP,though not all of them have meaning and/or effect in llc[4].We will start from the OpenMP parallel code shown in Listing2and we will add the necessary llc directives in order to complete the llc parallelization.The OpenMP captureprivate clause has no sense in llc,because llCoMP produces a MPI code where each processor has its private memory.(llc follows the OTOSP model[3],where all the processors on the same group have the same data in their private memories.)Unlike OpenMP,in llc all the variables are private by1#p r a g m a i n t e l omp t a s k2#p r a g m a l l c t a s k m a s t e r d a t a(&A0.m,1,&A1.offm,1,&A1.m,1)3#p r a g m a l l c t a s k m a s t e r d a t a(&C11.offm,1,&C11.o f f n,1,&C11.m,1,&C11.n,1)4#p r a g m a l l c t a s k m a s t e r d a t a(&C10.offm,1,&C10.o f f n,1,&C10.m,1,&C10.n,1)5#p r a g m a l l c t a s k s l a v e s e t d a t a(&A1.base,1,A.base,&A0.base,1,A.base)6#p r a g m a l l c t a s k s l a v e s e t d a t a(&C11.base,1,C.base,&C10.base,1,C.base)7#p r a g m a l l c t a s k s l a v e s e t d a t a(&A0.offm,1,A.offm,&A0.o f f n,1,A.o f f n,&A0.n,1,A.n)8#p r a g m a l l c t a s k s l a v e s e t d a t a(&A1.o f f n,1,A.o f f n,&A1.n,1,A.n)9#p r a g m a l l c t a s k s l a v e r n c d a t a((C10.base−>b u f f e r+((C10.o f f n∗C10.base−> ldim+C10.offm)∗s i z e o f(double))),(C10.m∗s i z e o f(double)),((C10.base−>ldim−C10.m)∗s i z e o f(double)),C10.n)10#p r a g m a l l c t a s k s l a v e r n c d a t a((C11.base−>b u f f e r+((C11.o f f n∗C11.base−> ldim+C11.offm)∗s i z e o f(double))),(C11.m∗s i z e o f(double)),((C11.base−>ldim−C11.m)∗s i z e o f(double)),C11.n)11{12/∗C10:=C10+A1∗A0’∗/13F L A_G e m m(F L A_N O_T R A N S P O S E,F L A_T R A N S P O S E,alpha,A1,A0,14beta,C10,n b_a l g);15/∗C11:=C11+A1∗A1’∗/16F L A_S y r k(F L A_L O W E R_T R I A N G U L A R,F L A_N O_T R A N S P O S E,alpha,A1,17beta,C11,n b_a l g); 18}Listing3.FLAME code for the SYRK operation parallelized using llc default,and we have to use llc directives to specify shared data.Listing3shows the parallelization of the FLAME code for the SYRK operation using llc.Afirst comparison of Listings2and3shows an apparent increase in the number of directives when llc is used.However,note that only three directives are actually needed,but we split those in order to improve the readability.Al-though llc code can be sometimes as simple as OpenMP code(see,e.g.,[4]), here we preferred to use an elaborated algorithm to illustrate how llc overcomes difficulties that usually appear when targeting parallel distributed memory ar-chitectures:references to specific data inside a larger data structure(submatrices instead of the whole matrix),access to non-contiguous memory locations,etc.In the llc implementation of Task Queues,a master processor handles the task queue,sends subproblems to the slaves,and gathers the partial results to construct the solution.Before the execution of each task,the master pro-cessor needs to communicate some initial data to the slaves,using the llc task master data directive.As the master and slaves processors are on the same group,they have the same values in each private memory region.Exploit-ing this,the master processor only sends those data that have been modified.With this approach the number of directives to be used is larger than in the OpenMP case,but the amount of communications is considerably reduced.The master needs to communicate to each slave the offset and number of elements of the objects A0,A1,C10,and C11(lines2–4).After each execution,the slave processors“remember”the last data used.To avoid this,we employ the llc task slave set data directives in lines5–8that initialize the variables before each task execution to certainfixed values(with no communications involved).The code inside the parallel task computes C10:=C10+A1A T0and C11:= C11+A1A T1.The slaves communicate to the master the results obtained(C10andC11).These data are not stored in contiguous memory positions and therefore can not be communicated as a single block.However,the data follow a regular pattern and can be communicated using the llc task slave rnc data directive (lines9–10).This directive specifies r egular n on-c ontiguous memory locations. 4Experimental ResultsAll the experiments reported in this section for the SYRK operation(C:=C+ AA T,with an m×m matrix C and an m×k matrix A)were performed using double-precisionfloating point arithmetic.The results correspond to the codes that have been illustrated previously in this paper(FLAME Variant1of the SYRK operation,Var1)as well as a second variant(Var2)for the same operation[10].Three different platforms were employed in the evaluation,with the common building block in all these being an Intel Itanium21.5GHz processor.Thefirst platform is a shared-memory(SM)Bull NovaScale6320with32processors.The second platform is a SM SGI Altix250with16processors.The third system is a hybrid cluster composed of9nodes connected via a10Gbit/s InfiniBand switch;each node is a SM architecture with4processors,yielding a total of 36processors in the system.An extensive experimentation was performed to determine the best block size(parameter nb alg in the algorithms)for each variant and architecture.Only those results corresponding to the optimal block size(usually,around96)are reported next.The OpenMP implementations were compiled with the Intel C compiler, while the llc binaries were produced with llCoMP combined with the mpich implementation of MPI on the SGI Altix and hybrid cluster,and MPIBull-Quadrics1.5on the NovaScale server.The goal of the experiments on SM platforms is to compare the performance of the SYRK implementation in OpenMP and llc.The results on the hybrid system are presented to demonstrate that high performance can be also achieved when the portability of llc is exploited.Table1reports the results for the SYRK codes.In particular,the second row of the table shows the execution time of the sequential code,while the remaining rows illustrate the speed-up of the OpenMP and llc parallelizations on the SGI Altix and the Bull NovaScale.The results show a similar performance for OpenMP and our approach on both architectures.OpenMP obtains a higher performance than llc when the number of processors is small.The reason for this behavior is that in the llc implementation one of the processors acts as the master.As the number of pro-cessors grows,the speed-up of llc increases faster than that of OpenMP.When the number of processors is large,llc yields better performance than OpenMP because it is less affected by memory bandwidth problems.The second variant of the algorithm exhibits a better performance than thefirst one,because it gen-erates a larger number of tasks withfiner granularity during the computations following a bidimensional partitioning of the work;see[10].#Proc.Var1SGI Var1Bull Var2SGI Var2Bullseq.19.0sec.176.5sec.19.0sec.176.5sec.–omp llc omp llc omp llc omp llc3 2.13 1.58 2.75 1.85 2.83 1.89 2.94 1.984 2.85 2.22 3.48 2.65 3.72 2.82 3.84 2.966 3.97 3.49 4.25 4.16 5.51 4.72 5.34 4.918 4.60 4.68 5.16 5.597.16 6.52 6.747.2110 5.78 5.70 6.83 6.988.828.338.168.6212 6.767.417.347.8110.2410.099.5310.6514 6.697.817.938.9011.6711.799.3713.20167.419.028.619.3512.7113.629.5613.76Table1.Sequential time and speed-up obtained on the SM platforms for Vari-ants1and2of the SYRK operation for both OpenMP and llc.For the Bull NovaScale6320(Bull),m=10000and k=7000.For the SGI Altix250(SGI), m=6000and k=3000.Figure1shows the speed-up obtained on the hybrid system.Again the second variant exhibits a better performance,and a maximum speed-up slightly above 25is attained using36processors.5Conclusions and Future Workllc is an language based on C that,given a sequential code annotated with directives and using the llCoMP translator-compiler,produces MPI parallel code. llc combines the high productivity in code development of OpenMP with the high performance and the portability of MPI.In this paper we have evaluated the performance of the Task Queues imple-mentation in llc using FLAME codes for the SYRK operation.We have shown that the llc directives facilitate optimization and tuning.The additional com-plexity introduced in the llc version with respect to the OpenMP version is clearly paid offby the portability of the code.The performance achieved with our approach is comparable to that obtained using OpenMP.Taking into ac-count the smaller effort to develop codes using llc compared with a direct MPI implementation,we conclude that llc is appropriate to implement some classes of parallel applications.Work in progress concerning this topic includes the following:–To study other variants and parallelization options for the SYRK operation, such as using two tasks per iteration or splitting the while loop.–To study other FLAME operations.We are currently working on the matrix-vector product.–To apply our approach to other scientific and engineering applications.–To extend the computational results to other machines and architectures.Fig.1.Speed-up on the hybrid system for Variants1and2of the SYRK operation parallelized using llc.On this system,m=5000and k=3000.References1.Message Passing Interface Forum,MPI:A Message-Passing Interface Standard,University of Tennessee,Knoxville,TN,1995,/.2.OpenMP Architecture Review Board,OpenMP Application Program Interface v.2.5(May2005).3. A.J.Dorta,J.A.Gonz´a lez,C.Rodr´ıguez,F.de Sande,llc:A parallel skeletallanguage,Parallel Processing Letters13(3)(2003)437–448.4. A.J.Dorta,P.Lopez,F.de Sande,Basic skeletons in llc,Parallel Computing32(7–8)(2006)491–506.5. A.J.Dorta,J.M.Bad´ıa,E.S.Quintana,F.de Sande,Implementing OpenMP forclusters on top of MPI,in:Proc.of the12th European PVM/MPI Users’Group Meeting,Vol.3666of LNCS,Springer-Verlag,Sorrento,Italy,2005,pp.148–155.6.S.Shah,G.Haab,P.Petersen,J.Throop,Flexible control structures for parallelismin OpenMP,Concurrency:Practice and Experience12(12)(2000)1219–1239. 7.P.Bientinesi,J.A.Gunnels,M.E.Myers,E.S.Quintana-Ort´ı,R.A.van deGeijn,The science of deriving dense linear algebra algorithms,ACM Trans.on Mathematical Software31(1)(2005)1–26.8. wson,R.J.Hanson,D.R.Kincaid,F.T.Krogh,Basic linear algebrasubprograms for fortran usage.,ACM Trans.Math.Softw.5(3)(1979)308–323.9.G.H.Golub,C.F.Van Loan,Matrix Computations,3rd Edition,Johns HopkinsUniversity Press,Baltimore,MD,1996.10. F.Van Zee,P.Bientinesi,T.M.Low,R.A.van de Geijn,Scalable parallelization ofFLAME code via the workqueuing model,ACM Trans.on Mathematical Software To appear.Electronically available at/users/flame/pubs.html.。