随机过程作业和答案第三章
钱敏平龚光鲁随机过程答案(部分)

钱敏平龚光鲁随机过程答案(部分)随机过程课后习题答案第⼀章第⼆题:已知⼀列⼀维分布{();1}n F x n ≥,试构造⼀个概率空间及其上的⼀个相互独⽴的随机变量序列{(,);1}n n ξ?≥使得(,)n ξ?的分布函数为()n F x 。
解:有引理:设ξ为[0, 1]上均匀分布的随机变量,F(x)为某⼀随机变量的分布函数,且F(x)连续,那么1()F x η-=是以F(x)为分布的随机变量。
所以可以假设有相互独⽴的随机变量12,,...,n θθθ服从u[0, 1]分布,另有分布{()}n F x ,如果令1(,)()n n n F ξθ-?=,则有(,)n ξ?为服从分布()n F x 的随机变量。
⼜由假设条件可知,随机变量{(,),1}n n ξ?≥之间相互独⽴,则其中任意有限个随机变量12(,), (,),...,(,)n i i i ξξξ的联合分布为:11221122{(,),(,),...,(,)}()()()i i n in i i i i in in P i x i x i x F x F x F x ξξξ?≤?≤?≤=再令112{,,...,,...},,{|()[0,1],1,2,...}n i i i i w w w w A A x F x i -Ω=∈=∈=,令F 为Ω所有柱集的σ代数,则由Kolmogorov 定理可知,存在F 上唯⼀的概率测度P 使得:11221122{(,),(,),...,(,)}()()()i i n in i i i i in in P i w i w i w F w F w F w ξξξ?≤?≤?≤=则所构造的概率空间为(Ω,F , P)。
第⼋题:令{};1n X n ≥是⼀列相互独⽴且服从(0,1)N (正态分布)的随机变量。
⼜令1n n S X X =++22(1)n S n n ξ+=1(,,)n n F X X σ=试证明:,;1n n F n ξ≥()是下鞅(参见23题)。
第3章 随机过程及答案

互相关函数 R (t1 , t 2 ) E[ (t1 )(t 2 )]
式中 (t) 和 (t) 分别表示两个随机过程。 R(t1, t2)又称为自相关函数。
10
3.2 平稳随机过程 3.2.1 平稳随机过程的定义
12
数字特征:
E (t ) x1 f1 ( x1 )dx1 a
R( t1 , t 2 ) E[ ( t1 ) ( t1 )]
x1 x2 f 2 ( x1 , x2 ; )dx1dx2 R( )
可见,(1)其均值与t 无关,为常数a ; (2)自相关函数只与时间间隔 有关。
P ( f ) 0
P ( f ) P ( f )
这与R()的实偶性相对应。
23
例题
[例3-2] 求随机相位余弦波(t) = Acos(ct + )的功率谱密度。 [解] 在[例3-1]中,我们已经考察随机相位余弦波是一个平稳 过程,并且求出其相关函数为
1 (t ) 2 (t )
n (t )
0
t
3
角度2:随机过程是随机变量概念的延伸。
在一个固定时刻t1上,不同样本的取值{i (t1), i = 1, 2, …, n} 是一个随机变量,记为 (t1)。
样本空间
随机过程是在时间进程中处于不同时刻的随机变量的集合。
S1 x1(t)
t
T /2
T / 2
x( t ) x( t )dt
aa R( ) R( )
随机过程第3章习题
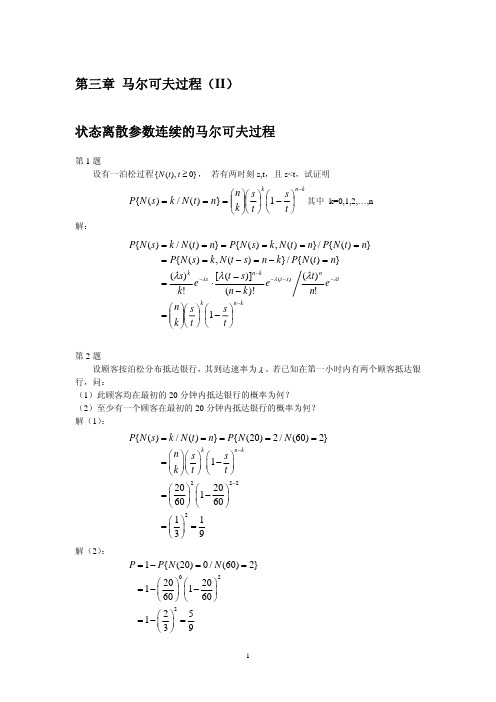
ψ N ( Δt ) ( s ) − 1
Δt
Δt → 0
= λ ( s − 1) 或
∂ψ N ( t ) ( s ) ∂t
(在证明过程中运用泊松过程的四个假设) 解(1) :
ψ N ( t ) ( s) = ∑ P{N (t = n)} ⋅ s n
n=0 ∞
∞
=∑
(λt ) n − λt n e s n! n=0 (λts) n n! n =0
⎛1⎞ 1 =⎜ ⎟ = ⎝ 3⎠ 9
解ቤተ መጻሕፍቲ ባይዱ2) :
P = 1 − P{N (20) = 0 / N (60) = 2} ⎛ 20 ⎞ = 1− ⎜ ⎟ ⎝ 60 ⎠
2 0
⎛ 20 ⎞ ⎜1 − ⎟ ⎝ 60 ⎠
2
⎛2⎞ 5 = 1− ⎜ ⎟ = ⎝3⎠ 9
1
第3题 设 {N (t ), t ≥ 0} 为泊松过程,其参数为 λ 。设ψ N ( t ) ( s ) 是随机变量 N(t)的母函数,证明 (1)ψ N ( t + Δt ) ( s ) = ψ N ( t ) ( s )ψ N ( Δt ) ( s )
无法写成泊松过程特征函数的形式
exp{λ t (e jv − 1)}
5
由于特征函数与概率有相同的特性
∴ N 0 (t ) = N1 (t ) − N 2 (t )不符合泊松过程的分布规律.
第8题 有复合泊松过程 { X (t ) =
N (t ) n =1
∑Y , t ≥ 0} ,其中 Y , n = 1,2,3,L 是彼此统计独立、同分布的随
0
φN (v) = E{exp{ jvN0 (t )}
0
= E{exp{ jv( N1 (t ) − N 2 (t )} = E{exp{ jvN1 (t ) ⋅ exp{− jvN 2 (t )} = E{exp{ jvN1 (t )}E{exp{− jvN 2 (t )} = φN1 (v)φN2 (−v) = exp{λ1t (e jv − 1)}exp{λ2t (e− jv − 1)} = exp{λ1te jv + λ2te− jv + (λ1 + λ2 )t}
随机过程 第三章 马尔科夫链
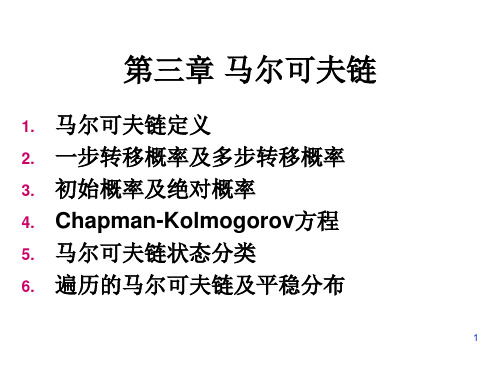
p p
iI
i ii1 pin1in
14
例:某计算机机房的一台计算机经常出故障,研究者每隔15分钟观察一次计 算机的运行状态,收集了24个小时的数(共作97次观察),用1表示正常状态, 用0表示不正常状态,所得的数据序列如下: 11100100111111100111101111110011111111100011 01101111011011010111101110111101111110011011 111100111
1
2
3
4
5
6
例:排队模型 设服务系统由一个服务员和只可以容纳两个人的等候室组 成。服务规则为:先到先服务,后来者需在等候室依次排队, 假设一个需要服务的顾客到达系统时发现系统内已有3个顾客, 则该顾客立即离去。 设时间间隔⊿t内有一个顾客进入系统的概率为q,有一接 受服务的顾客离开系统(即服务完毕)的概率为p,又设当⊿t充分 小时,在这时间间隔内多于一个顾客进入或离开系统实际上是 不可能的,再设有无顾客来到与服务是否完毕是相互独立的。
2
马尔可夫链定义
时间和状态都离散的马尔可夫过程称为马尔可夫链
定义:设有随机过程{Xn,n∈T},若对于任意的整数n∈T和任意的 i0,i1, …,in+1∈I,条件概率满足
P{ X n 1 in 1 | X 0 i0 , X 1 i1 ,, X n in } P{ X n 1 in 1 | X n in }
i
n 1
nfii( n )
表示由i出发再返回i的平均返回时间。
24
定义 如ui<∞,则称常返态i为正常返的;如ui= ∞,则称常返态i为零常返的。
非周期的正常返态称为遍历状态。 常返性的判别
随机过程2016作业及答案3
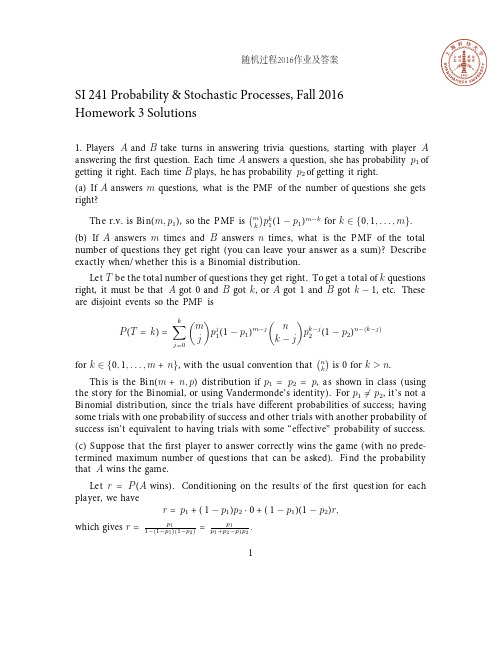
1.Players A and B take turns in answering trivia questions, starting with player A answering the first question. Each time A answers a question, she has probability p 1 of getting it right. Each time B plays, he has probability p 2 of getting it right.(a)If A answers m questions, what is the PMF of the number of questions she gets right?The r.v.is Bin(m,p 1),so the PMF is mkp k 1(1 p 1)m k for k 2{0,1,...,m }.(b)If A answers m times and B answers n times,what is the PMF of the total number of questions they get right (you can leave your answer as a sum)?Describe exactly when/whether this is a Binomial distribution.Let T be the total number of questions they get right.To get a total of k questions right,it must be that A got 0and B got k ,or A got 1and B got k 1,etc.These are disjoint events so the PMF isP (T =k )=k X j =0✓mj ◆p j 1(1 p 1)m j ✓n k j◆p k j 2(1 p 2)n (k j )for k 2{0,1,...,m +n },with the usual convention that n k is 0for k >n .This is the Bin(m +n,p )distribution if p 1=p 2=p ,as shown in class (using the story for the Binomial,or using Vandermonde’s identity).For p 1=p 2,it’s not a Binomial distribution,since the trials have di ↵erent probabilities of success;having some trials with one probability of success and other trials with another probability of success isn’t equivalent to having trials with some “e ↵ective”probability of success.(c)Suppose that the first player to answer correctly wins the game (with no prede-termined maximum number of questions that can be asked).Find the probability that A wins the game.Let r =P (A wins).Conditioning on the results of the first question for each player,we have r =p 1+(1 p 1)p 2·0+(1 p 1)(1 p 2)r,which gives r =p 11 (1 p 1)(1 p 2)=p 1p 1+p 2 p 1p 2.1SI 241 Probability & Stochastic Processes, Fall 2016Homework 3 Solutions随机过程2016作业及答案2.A message is sent over a noisy channel.The message is a sequence x1,x2,...,x n of n bits(x i2{0,1}).Since the channel is noisy,there is a chance tha t any bit might be corrupted,resulting in an error(a0becomes a1or vice versa).Assume that the error events are independent.Let p be the probability that an individual bit has an error(0<p<1/2).Let y1,y2,...,y n be the received message(so y i=x i if there is no error in that bit,but y i=1 x i if there is an error there).To help detect errors,the n th bit is reserved for a parit y check:x n is defined to be 0if x1+x2+···+x n 1is even,and1if x1+x2+···+x n 1is odd.When the message is received,the recipient checks whether y n has the same parit y as y1+y2+···+y n 1. If the parity is wrong,the recipient knows that at least one error occurred;otherwise, the recipient assumes that there were no errors.(a)For n=5,p=0.1,what is the probabilit y that the received message has errors which go undetected?Note that P n i=1x i is even.If the number of errors is even(and nonzero),the errors will go undetected;otherwise,P n i=1y i will be odd,so the errors will be detected.The number of errors is Bin(n,p),so the probability of undetected errors when n=5,p=0.1is✓52◆p2(1 p)3+✓54◆p4(1 p)⇡0.073.(b)For general n and p,write down an expression(as a sum)for the probability that the received message has errors which go undetected.By the same reasoning as in(a),the probability of undetected errors isX k even,k 2✓n k◆p k(1 p)n k.(c)Give a simplified expression,not involving a sum of a large number of terms,for the probabilit y that the received message has errors which go undetected.Hint for(c):Lettinga=X k even,k 0✓n k◆p k(1 p)n k and b=X k odd,k 1✓n k◆p k(1 p)n k,the binomial theorem makes it possible tofind simple expressions for a+b and a b, which then makes it possible to obtain a and b.2Let a,b be as in the hint.Thena +b =X k 0✓n k ◆p k (1 p )n k =1,a b =X k 0✓n k ◆( p )k (1 p )n k =(1 2p )n .Solving for a and b gives a =1+(1 2p )n 2and b =1 (1 2p )n2.Xk even,k 0✓n k ◆p k (1 p )n k =1+(1 2p )n 2.Subtrac ting o ↵the possibility of no errors,we haveX k even,k 2✓n k ◆p k (1 p )n k =1+(1 2p )n 2 (1 p )n .Miracle check :note that letting n =5,p =0.1here gives 0.073,which agrees with (a);letting p =0gives 0,as it should;and letting p =1gives 0for n odd and 1for n even,which agai n makes sense.33.Let X be a r.v. whose possible values are 0, 1, 2,...,with CDF F .In some countries, rather than using a CDF, the convention is to use the function G defined by G (x )=P (X <x ) to specify a distribution. Find a way to convert from F to G , i.e., if F is a known function show how to obtain G (x )for all real x .Write G (x )=P (X x ) P (X = x )=F (x ) P (X = x ).If x is not a nonnegative integer, then P (X = x )=0so G (x )=F (x ). For x a nonnegative integer,P (X = x )=F (x ) F (x 1/2)since the PMF corresponds to the lengths of the jumps in the CDF. (The 1/2was chosen for concreteness; we also have F (x 1/2) = F (x a )for any a 2 (0, 1].)Thus,G (x )=(F (x )if x /2{0,1,2,...}F (x 1/2)if x 2{0,1,2,...}.t More compact ly, we can also write G (x )=lim !x F (t ), where the denotes taking a limit from the left (recall that F is right continuous), and G (x )=F (d x e 1),where d x e is the “ceiling” of x (the smallest integer greater than or equal to x ).4.There are n eggs, each of which hatches a chick with probability p (independently).Eac h of these chicks survives with probability r , independently. What is the distri-bution of the number of chicks that hatch? What is the distribution of the number of chicks that survive? (Give the PMFs; also give the names of the distributions and their parame ters, if they are distributions we have seen in class.)⇤⇥ ©⇤⇥ x ⇤⇥ ⇤⇥ ⇤⇥ ©⇤⇥ ©⇤⇥ x ⇤⇥ ©⇤⇥ ⇤⇥©Let H be the number of eggs that hatch and X be the number of hatchlings that survive.Think of each egg as a Ber noulli trial,where for H we define “success”to mean hatching,while for X we define “success”to mean surviving.For example,in the picture above,where ⇤⇥ ©denotes an egg that hatches with the chick surviving,⇤⇥ x denotes an egg that hatched but whose chick died,and ⇤⇥ denotes an egg that hatch,the events H =7,X =5occurred.By the of the Binomial,H ⇠Bin(n,p ),with PMF P (H =k )= n k p k (1 p )n k for k =0,1,...,n .The eggs independently have probability pr each of hatching a chick that survives.By the story of the Binomial,we have X ⇠Bin(n,pr ),with PMF P (X =k )= n k (pr )k (1 pr )n k for k =0,1,...,n .5.A scientist wishes to study whether men or women are more likely to have a certain disease, or whether they are equally likely. A random sample of m women and n men is gathered, and each person is tested for the disease (assume for this problem that the test is completely accurate). The numbers of women and men in he sa B n(n,w p ho ha He ve re p h e di and seas p e ar are e X unkno and Y wn,re p s and ec w tiv e e r a ly,Y i 2.1 2 e w in ith tereste d ⇠Bi in n(testin g p 1) a the le mp t t X ,m nd ⇠) “null hypothesis” p 1 = p 2.(a) Consider a 2 by 2 ta ble listing with rows corresponding to disease status and columns corresponding to gender, with each entry the count of how many people have that disease status and gender (so m + n is the sum of all 4 entries). Supp ose that it is observed that X + Y = r .The Fisher exact test is based on conditioning on both the row and column sums, so m, n, r are all treated as fixed, and then seeing if the observed value of X is “extreme” compared to this conditional distribution. Assuming the null hypothesis, use Ba yes’ Rule to find the conditional PMF of X given X + Y = r .Is this a distribution we have studied in class? If so, say which (and give its paramet ers).First let us build the 2 ⇥ 2 table (conditioning on the totals m, n, and r ).4Women Men Total Disease x r No Diseasem x r r x +n x m +n r Total n m m +nNext,let us compute P (X =x |X +Y =r ).By Ba yes’rule,P (X =x |X +Y =r )=P (X +Y =r |X =x )P (X =x )P (X +Y =r )=P (Y =r x )P (X =x )P (X +Y =r ).Y Assum Bi i n n (g n,th p e )w nu i l t l h h X ypot inde h p esi e s nde an n d t l of etti Y ng ,s p o =X p +1Y =p 2Bi ,w n(e n h +ave m,X p ).⇠T Bi h n us (,m,p )and ⇠⇠r x p r x p p )r n (1 r +x n m x p x (1 p )m x (1m + n r p )m +n r P (X =x |X +Y =r )== m nx m +r n rx .So the conditional distribution is Hypergeometric with parameters m, n, r.(b) Give an intuitive explanation for the distribution of (a), explaining how this problem relates to other problems we’ve seen, and why p 1 disappears (magica lly?) in the distribution found in (a).This problem has the same structure as the elk (capture-recapture) problem. In the elk problem, we take a sample of elk from a population, where earlier some were tagged, and we want to know the distribution of the number of tagged elk in the sample. By analogy, think of the women as corresponding to tagged elk, and men as corresponding to unta gged elk. Having r people be infected with the disease corresponds to capturing a new sample of r elk the number of women among the r diseased individuals corresponds to the number of tagged elk in the new sample.Under the null hypothesis and given that X + Y = r ,the set of diseased people is equally likely to be any set of r people.It makes sense that the conditional distribution of the number of diseased women does not depend on p ,since once we know tha t X + Y = r ,we can work directly in terms of the fact that we have a population with r diseased and m + n r undiseased people, without worrying about the value of p that originally generated the population characteristics.5。
(解答)《随机过程》第三章习题
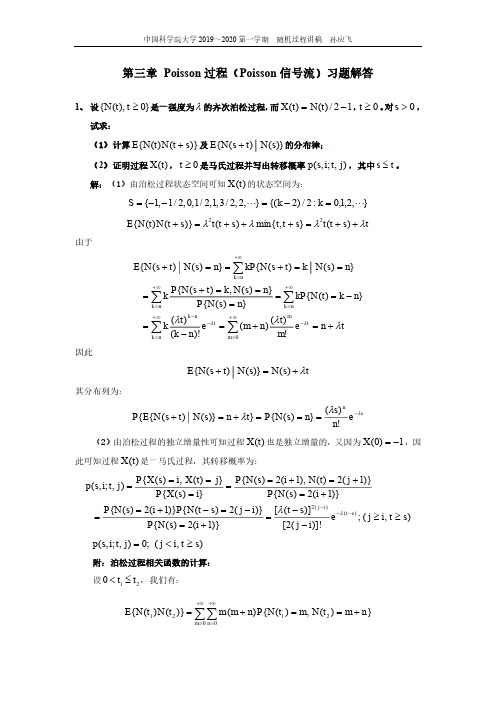
(1)试求随机过程{Z (t); t 0}的均值函数 E{Z (t)}和二阶矩 E{Z 2 (t)} ;
(2)试证明: pn (t)u n exp{(1 2 )t } exp{1ut 2u 1t }。 n
P{X (s) i}
P{N (s) 2(i 1)}
P{N (s) 2(i 1)}P{N (t s) 2( j i)} [(t s)]2( ji) e(ts) ; ( j i, t s)
P{N (s) 2(i 1)}
[2( j i)]!
lim
h0
Pt
2
h 2
S2
t2
h 2 ,t5 h2
h 2
S5
t5
h
2
5 2
t2 (t5
t2 )2 et5
,
0 t2 t5
(2)由于{N (t) 1} {S1 t} ,由泊松过程与指数分布的关系可知,在{S1 t} 条件 下, S1 的分布密度函数为
(3)由于{N (t) 1} {S1 t S2} ,令: 0 t1 t t2 ,取充分小的 h1, h2 0 ,
使得: t1 h1 t1 t t2 h2 t2 ,由
t1 h1 S1 t1, t2 h2 S2 t2 N t1 h1 0, N t1 N t1 h1 1,
3、 设{N1 (t); t 0}和{N 2 (t); t 0} 是相互独立的 Poisson过程,其参数分别为 1 和 2 .若 N0 (t) N1 (t) N 2 (t) ,问: (1) {N0 (t); t 0} 是否为 Poisson 过程,请说明理由; (2) {N0 (t); t 0} 是否为平稳过程,请说明理由。 解:(1)由于 N 0 (t) 的状态空间为 S {,1, 0,1,} ,因此 N 0 (t) 不是计数过程,更
随机过程作业和答案第三章
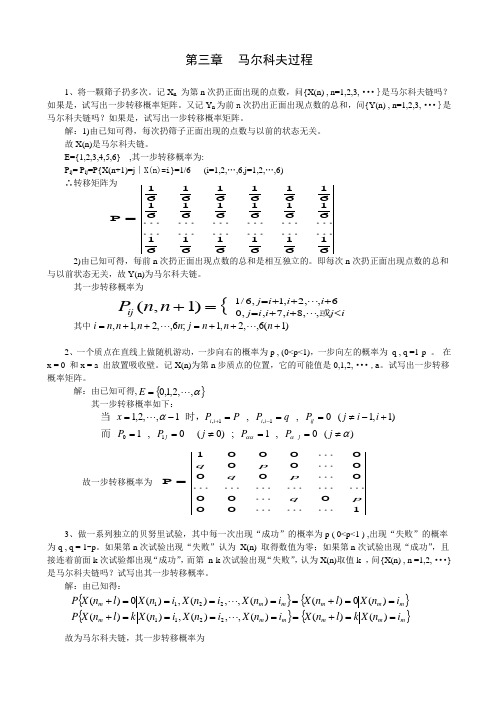
第三章 马尔科夫过程1、将一颗筛子扔多次。
记X n 为第n 次扔正面出现的点数,问{X(n) , n=1,2,3,···}是马尔科夫链吗?如果是,试写出一步转移概率矩阵。
又记Y n 为前n 次扔出正面出现点数的总和,问{Y(n) , n=1,2,3,···}是马尔科夫链吗?如果是,试写出一步转移概率矩阵。
解:1)由已知可得,每次扔筛子正面出现的点数与以前的状态无关。
故X(n)是马尔科夫链。
E={1,2,3,4,5,6} ,其一步转移概率为:P ij = P ij =P{X(n+1)=j ∣X(n)=i }=1/6 (i=1,2,…,6,j=1,2,…,6) ∴转移矩阵为2)由已知可得,每前n 次扔正面出现点数的总和是相互独立的。
即每次n 次扔正面出现点数的总和与以前状态无关,故Y(n)为马尔科夫链。
其一步转移概率为其中2、一个质点在直线上做随机游动,一步向右的概率为p , (0<p<1),一步向左的概率为 q , q =1-p 。
在x = 0 和x = a 出放置吸收壁。
记X(n)为第n 步质点的位置,它的可能值是0,1,2,···,a 。
试写出一步转移概率矩阵。
解:由已知可得, 其一步转移概率如下:故一步转移概率为3、做一系列独立的贝努里试验,其中每一次出现“成功”的概率为p ( 0<p<1 ) ,出现“失败”的概率为q , q = 1-p 。
如果第n 次试验出现“失败”认为 X(n) 取得数值为零;如果第n 次试验出现“成功”,且接连着前面k 次试验都出现“成功”,而第 n-k 次试验出现“失败”,认为X(n)取值k ,问{X(n) , n =1,2,···}是马尔科夫链吗?试写出其一步转移概率。
解:由已知得:故为马尔科夫链,其一步转移概率为616161616161616161616161616161616161P ={6,,2,1,6/1,,8,7,,0)1,(+++=<++==+i i i j i j i i i j ij n n P 或)1(6,,2,1;6,,2,1,+++=++=n n n j n n n n i {}α,,2,1,0 =E )(0,1;)0(0,1)1,1(0,,1,,2,1101,1,ααααα≠==≠==+-≠===-=-+j P P j P P i i j P q P P P x j j ij i i i i 而时,当 10000000000000001Pp q p q p q ={}{}m m m m m m i n X l n X i n X i n X i n X l n X P ==+=====+)(0)()(,,)(,)(0)(2211 {}{}mm m m m m in X k l n X i n X i n X i n X k l n X P ==+=====+)()()(,,)(,)()(22114、在一个罐子中放入50个红球和50个蓝球。
随机过程第三章作业答案

Yk-1 ]] ≤ b ⋅ ∑ E[I{T ≥ k} ]
k =0
= b ⋅ ∑ P(T ≥ k) = b(1 + E[T]) < ∞,即E[W] < ∞
10证明:利用停时定理2 由已知P(T<∞)=1,得条件1已满足。
2 2 又∀n ≥ 1,E[X T ∧ n ]=E[|X T ∧ n | ] ≤ c;
利用柯西-施瓦茨不等式(E[XY])2 ≤ E[X 2 ]E[Y 2 ]: 令Y=1,(E[|X T ∧ n |])2 ≤ E[|X T ∧ n |2 ]E[12 ] ≤ c ∴ E[|X T ∧ n |] ≤ c,进而有E[ sup|X T ∧ n |] ≤ c < ∞,
第三章习题解答
3-(1) ∵{ X n , n ≥ 0}是鞅, ∴ E[X 0 ] = E[X n ] = 0,且有 E[Yk ]=E[X k -X k-1 ]=0;Var(Yk )=E[Yk2 ];Var(X n )=E[X 2 n ];
2 E[Yk2 ]=E[(X k -X k-1 )2 ]=E[X k +X 2 k-1 -2X k X k-1 ] 2 =E[X k ]+E[X 2 其中 k-1 ]-2E[X k X k-1 ],
9 (一)常规证明: 右侧不等号: E[X T ∧ n ]=E[X T ∧ n ⋅ I{T ≥ n} ]+E[X T ∧ n ⋅ I{T<n} ]=E[X n ⋅ I{T ≥ n} ]+E[X T ⋅ I{T<n} ] =E[X n ⋅ I{T ≥ n} ]+E[∑ X k ⋅ I{T=k} ]
k =0 n-1
E[X k X k-1 ]=E[E[X k X k-1|X 0 X1 =E[X k-1E[X k |X 0 X1
- 1、下载文档前请自行甄别文档内容的完整性,平台不提供额外的编辑、内容补充、找答案等附加服务。
- 2、"仅部分预览"的文档,不可在线预览部分如存在完整性等问题,可反馈申请退款(可完整预览的文档不适用该条件!)。
- 3、如文档侵犯您的权益,请联系客服反馈,我们会尽快为您处理(人工客服工作时间:9:00-18:30)。
第三章 马尔科夫过程1、将一颗筛子扔多次。
记X n 为第n 次扔正面出现的点数,问{X(n) , n=1,2,3,···}是马尔科夫链吗?如果是,试写出一步转移概率矩阵。
又记Y n 为前n 次扔出正面出现点数的总和,问{Y(n) , n=1,2,3,···}是马尔科夫链吗?如果是,试写出一步转移概率矩阵。
解:1)由已知可得,每次扔筛子正面出现的点数与以前的状态无关。
故X(n)是马尔科夫链。
E={1,2,3,4,5,6} ,其一步转移概率为:P ij = P ij =P{X(n+1)=j ∣X(n)=i }=1/6 (i=1,2,…,6,j=1,2,…,6) ∴转移矩阵为2)由已知可得,每前n 次扔正面出现点数的总和是相互独立的。
即每次n 次扔正面出现点数的总和与以前状态无关,故Y(n)为马尔科夫链。
其一步转移概率为其中2、一个质点在直线上做随机游动,一步向右的概率为p , (0<p<1),一步向左的概率为 q , q =1-p 。
在x = 0 和x = a 出放置吸收壁。
记X(n)为第n 步质点的位置,它的可能值是0,1,2,···,a 。
试写出一步转移概率矩阵。
解:由已知可得, 其一步转移概率如下:故一步转移概率为3、做一系列独立的贝努里试验,其中每一次出现“成功”的概率为p ( 0<p<1 ) ,出现“失败”的概率为q , q = 1-p 。
如果第n 次试验出现“失败”认为 X(n) 取得数值为零;如果第n 次试验出现“成功”,且接连着前面k 次试验都出现“成功”,而第 n-k 次试验出现“失败”,认为X(n)取值k ,问{X(n) , n =1,2,···}是马尔科夫链吗?试写出其一步转移概率。
解:由已知得:故为马尔科夫链,其一步转移概率为616161616161616161616161616161616161P ={6,,2,1,6/1,,8,7,,0)1,(+++=<++==+i i i j i j i i i j ij n n P 或)1(6,,2,1;6,,2,1,+++=++=n n n j n n n n i {}α,,2,1,0 =E )(0,1;)0(0,1)1,1(0,,1,,2,1101,1,ααααα≠==≠==+-≠===-=-+j P P j P P i i j P q P P P x j j ij i i i i 而时,当 10000000000000001Pp q p q p q ={}{}m m m m m m i n X l n X i n X i n X i n X l n X P ==+=====+)(0)()(,,)(,)(0)(2211 {}{}mm m m m m in X k l n X i n X i n X i n X k l n X P ==+=====+)()()(,,)(,)()(22114、在一个罐子中放入50个红球和50个蓝球。
每随机地取出一球后,再放入一个新球进去,新球为红球和兰球的概率各为1/2。
第n 次取出一球后,又放入一个新球,留下的红球数记为X(n)。
问{X(n) , n =1,2,···}是马尔科夫链吗?试写出一步转移概率矩阵(当 n ≥50)。
解:由已知得,将来状态X(n)只与当前状态X(n-k)有关,故X(n)为马尔科夫链5、随机地扔两枚分币,每枚分币的面有“国徽”和“分值”之分。
X(n) 表示两枚分币分扔 n 次后面正面出现“国徽”的总个数。
试问X(n)是否是马尔科夫链?写出一步转移概率。
解:由已知得,将来状态X(n)只与当前状态X(n-k)有关。
故X(n)是马尔科夫链。
6、扔一颗筛子,如果前n 次扔出现点数的最大值为j ,就说X(n)的值等于。
试问{X(n) , n =1,2,···}是不是马尔科夫链?并写出一步转移概率矩阵。
解:由已知得,将来状态X(n)只与当前状态X(n-k)有关。
故X(n)是马尔科夫链。
⎪⎩⎪⎨⎧+===+,取其他值01,0,)1,(P j j p j q n n ij)1(6,,2,1;6,,2,1,+++=++=n n n j n n n n i 其中{}21210020012110099010099212001000100992110010020099212001002121)99(0)1(0211001i )2200i -10021100i -100P 200i 21100i P )()(2121100i 10021100i P 9932i 150,100,,2,1,0100,1100,100100,992,11,1,,ij一步转移概率矩阵为时,球)蓝个红球又放回蓝个红(取出时,,,)时,一步转移概率当<=>=======•==•==•-+•==≥=+-i P j P P P P P i i n E i j j i i j i i ⎪⎪⎪⎩⎪⎪⎪⎨⎧+=+==+取其他值其一步转移概率为j i j i i j n n ,01,212,,41)1,(P ij )1(2,,1,0;2,,1,0+==n j n i 其中其一步转移概率矩阵为7、假定随机变量X 0的概率分布为 P{X 0=1}=p , P{X 0= -1}=1-p ,0<p<1,对n = 0,1,2,3,…, 定义 1)画出{X(n) , n = 0,1,2,3,…}的所有样本函数。
2)说明{X(n) , n = 0,1,2,3,…}不具有马尔科夫性(既无后效性。
解:1)2)当n 取值时,X(2n+1)的值先于当n-1时的X(2n)的值∴{X(n) , n = 0,1,2,…}不具有马尔科夫性,即不具有无后效性。
8、将适当的数字填入下面空白处,使矩阵是一步转移概率矩阵。
解:()为偶数当为奇数当n n 00{2X X n X -=()012=+n X )6,,2,1,()(0,)(61,6 =<=>==j i i j P i j P i P j i j i i i 10000061650000616164000616161630061616161620616161616161P =其一步转移概率矩阵为43411101101101313131=P 00434110001011011071013131310=P9、设马尔科夫链的一步转移概率矩阵为试求二步转移概率解:10、设马尔科夫链的一步转移概率为,其中p>0,q<0,p+q=1。
试求二步转移概率矩阵和三步转移概率矩阵,并用数学归纳法证明一般n 步转移概率矩阵为解:1)⎥⎦⎤⎢⎣⎡++==2222222)2(q p pqpq q p P P ⎥⎦⎤⎢⎣⎡++++==2332322333333)3(pq p q q p q q p pq p P P2)证明:a)当n=1时,等式成立b)假设当n=k 时,等式成立 即:则当n=k+1时,即当n=k+1时,等式成立。
综合a),b)得,一般n 步转移概率矩阵位11、设马尔科夫链具有状态空间E={1,2,3},初始概率分布为612131313131613121=P ⎥⎥⎥⎥⎥⎥⎦⎤⎢⎢⎢⎢⎢⎢⎣⎡=⎥⎥⎥⎥⎥⎥⎦⎤⎢⎢⎢⎢⎢⎢⎣⎡==4136131879218718792125125612131313131613121612131313131613121)2(2P P p q q p P =n n nn q p q p q p q p P )(1)(1)(1)(121-+-----+=p q q p P =)1(⎥⎦⎤⎢⎣⎡-+-----+=⎥⎦⎤⎢⎣⎡-++--+--+-++=⎥⎦⎤⎢⎣⎡-+-----+=⋅=+++++++++11111111)(1)(1)(1)(121)()()()(21)(1)(1)(1)(121)()1(k k k k k k k k nn nn q p q p q p q p q p q p q p q p q p q p q p q p p q q p q p q p q p q p P k P k Pk k kk q p q p q p q p k P )(1)(1)(1)(121)(-+-----+=n n nn q p q p q p q p P )(1)(1)(1)(121-+-----+=412141)0(3)0(2)0(1===P P P和一步转移概率矩阵为(1) 计算P{X(0)=1,X(1)=2,X(2)=2};(2) 试证P{X(1)=2,X(2)=2 | X(0)=1}=p 12p 22 (3) 计算p 12(2)。
解:1)2)3)12、设马尔科夫链具有状态空间E={1,2 },初始概率分布为和一步转移概率矩阵为(1) 计算P{X(0)=1,X(1)=2,X(2)=2};(2) 计算P{X(n)=1,X(n+1)=2,X(n+2)=2},n=1,2,3; (3) 计算P{X(n)=1, X(n+2)=2},n=1,2,3; (4) 计算P{ X(n+2)=2},n=1,2,3; (5) 在(1),(2),(3),(4)中哪些依赖于n ,哪些不依赖于n 。
解:1)⎥⎥⎥⎥⎥⎥⎦⎤⎢⎢⎢⎢⎢⎢⎣⎡=4341031313104341P ()()(){}161314304131430213143141)1()1()0()1()1()0()1()1()0()1()1()0(22,21,10221231)0(3221221)0(231221211)0(13122121)0(=⨯⨯⨯+⨯⨯⨯+⨯⨯⨯=++=====∑∑==p p p p p p p p p p p p p p p p X X X P i i i i ()()(){}()()(){}{}2212221211)0(1221211)0(1)1()1()0()1()1()0(1)0(22,21,1010|22,21p p p p p p p p p p X P X X X P X X X P ===========16741031434341)1()1()1()1()1()1()1()1()2(323122211211312112=⨯+⨯+⨯=++==∑=P P P P P P P P P r r r 100)0(2)0(1=+>>==b a b a b P a P ⎥⎥⎥⎦⎤⎢⎢⎢⎣⎡=21213132P ()()(){}62131021311)1()1()0()1()1()0()1()1()0(22,21,10221221)0(231221211)0(12122121)0(ab a p p p p p p p p p p p p X X X P i i i i =⨯⨯⨯+⨯⨯⨯=+=====∑∑==2)()()(){}()()(){}()()(){}()()()(){}()()(){}()(){}[]()(){}[]⎪⎭⎫⎝⎛+=+======⎪⎭⎫⎝⎛+=⎪⎭⎫ ⎝⎛⨯+⨯=+=======⨯+⨯=+===∴⎥⎥⎥⎦⎤⎢⎢⎢⎣⎡=⎥⎥⎥⎦⎤⎢⎢⎢⎣⎡===+=+=⎪⎭⎫ ⎝⎛⨯+⨯⨯=+======⎥⎥⎥⎦⎤⎢⎢⎢⎣⎡==+=⎪⎭⎫ ⎝⎛⨯+⨯⨯=+======⎥⎥⎥⎦⎤⎢⎢⎢⎣⎡==+=⨯⨯⨯+⨯⨯⨯=+=========+=+=∑∑∑∑∑∑∑∑========36127187)2()2()2()2()2(24,12,26211872132187)1()1()2()2()1(23,11,118721313132)1()1()1()1()1()1()2(187)2(1251271871811)2(31213132)1()1()0(22,1)3)2167243(6172432161302131)3()3()1()1()1()1()3(25,24,1372297243216862161303)36127(6112718112131)2()2()1()1()1()1()2(24,23,1212512718718112)621(61213121213132)1()0()1()1()0()1()1()0(23,22,11,1)1()1()0(22,21,1210211011221121)0(210211011221121)0(22121211221112122121121)0(21)0(211)0(121122121121)0(321)0(211)0(121122121121)0(2211221)0(2211211)0(12121121)0(2121121)0(a p p p p p p p p X X P n a b a p p p p p p p p X X P n P P P P P P P P P P p p p p n X n X P ab a p p p p p p p p p p X X X P P n ab a p p p p p p p p p p X X X P P n ab a p p p p p p p p p p p p X X X P n p p p p n X n X n X P i i i i i i r r r i i i i i i i i i i i i i i i 原式当原式当原式时,当原式时,当原式当13、在12题中,初始概率分布为试对n=1,2,3计算其绝对概率分布 解:14、设马尔科夫链的一步转移概率矩阵为其中p<0,q>0,r>0,且p+q+r=1,初始概率分布为 试对n=1,2,3计算其绝对概率分布()(){}(){}(){}(){}(){}。