Nested Graphs A Graph-based Knowledge Representation Model with FOL Semantics
Stata Graph Editor 用户指南说明书

15Editing graphsThe Graph EditorWith Stata’s Graph Editor,you can change almost anything on your graph;you can add text,lines, arrows,and markers wherever you like.We willfirst make a graph to edit and will then point out the tools in the Graph Editor.Start by opening the automobile dataset:sysuse auto.Here is the command that we will use to make the graph:.scatter mpg weight,name(mygraph)title(Mileage vs.vehicle weight)Start the Editor by right-clicking on your graph and selecting Start Graph Editor.Click once on the title of the graph.Here is a picture of the Graph Editor with its elements labeled.Main menu Standard toolbar Contextual toolbar Selected objectbrowserObjectSelect any of the tools along the left of the Graph Editor window to edit the graph.The Pointer(Select tool),,is selected by default.You can change the properties of objects or drag them to new locations by using the Pointer.As you select objects with the Pointer,a Contextual Toolbar will appear just above the graph.In the above example,the title of the graph is selected,so the Contextual Toolbar has controls that are relevant for editing titles.You can use any of the controls on the Contextual Toolbar to immediately change the most important properties of the selected object.Right-click on an object to access more properties and operations.Hold the Shift key when dragging objects to constrain the movement to horizontal or vertical directions.12[GSU]15Editing graphsAdd text,lines,or markers(with optional labels)to your graph by using the three Add...tools—,,and.Lines can be changed to arrows by using the Contextual Toolbar.If you do not like the default properties,simply change their settings in the Contextual Toolbar before adding the text,line,or marker.The new settings will then be applied to all added objects,even in future Stata sessions.Do not be afraid to try things.If you do not like a result,change it back by using the same tool or by clicking on the Undo button,,in the Standard Toolbar for the Graph Editor(below the main menu).Edit>Undo in the main menu does the same thing.Remember to reselect the Pointer tool when you want to drag objects or change their properties.You can move objects on the graph and have the rest of the objects adjust their position to accommodate the move with the Grid edit tool,.With this tool,you are repositioning objects in the underlying grid that holds the objects in the graph.Some graphs,for example,by graphs,are composed of nested grids.You can reposition objects only within the grid that contains them;they cannot be moved to other grids.You can also select objects in the Object Browser along the right of the graph.This window shows a hierarchical listing of the objects in the graph.Clicking or right-clicking on an object in the Object Browser is the same as clicking or right-clicking on the object in the graph.The Graph Editor has the ability to record your actions and play them back on later graphs.When you click on the Start recording button,,every editing action you take,including undos and redos,is recorded.If you would like to do some editing that is not recorded,you can click on the Pause recording button,.You can click on the Pause recording button again to resume recording. When you are done with your recording,click on the Start recording button.You will be prompted to save your recording.Any recording you save is available from the Play recording button,, and may be applied to future graphs.You can even play a recording in any Stata graph command by using the play option.See Graph Recorder in[G-1]Graph Editor for more information.Stop the editor by selecting File>Stop Graph Editor from the main menu or by clicking on the Graph Editor button.When you stop the Graph Editor,you will be prompted to save your graph if you have made any changes.If you do not save your graph,your changes will not be lost,but you will risk losing them if you create a new graph in the same Graph window.You must stop the Editor if you would like to work on other tasks in Stata.Here are a few of the things that you can do with the Editor:•Add annotations using lines,arrows,and text.•Add or remove grid lines or reference lines.•Add or modify titles,captions,and notes.•Change scatterplots to line plots,connected plots,areas,bars,spikes,or drop lines—and,of course,vice versa.•Change the size,color,margin,and other properties of your graph’s titles(or any other text on the graph).•Move your legend to another side of the graph,or even place it in the plot region.•Change the aspect ratio of your graph.•Stack the bars on a bar graph or turn them into percentages.•Rotate or change the angle of axis labels.•Add custom ticks and labels to the axes.•Change the rule for the number and spacing of ticks and labels on an axis.[GSU]15Editing graphs3•Emphasize a point on the graph,whether marker,bar,spike,or other plot,by making it a custom color,size,or symbol.•Change the text or properties of a marker label.Because you can edit every property of every object on the graph,you can change almost anything about your graph.To learn more,see[G-1]Graph Editor or type help graph editor.4[GSU]15Editing graphsStata,Stata Press,and Mata are registered trademarks of StataCorp LLC.Stata andStata Press are registered trademarks with the World Intellectual Property Organization®of the United Nations.Other brand and product names are registered trademarks ortrademarks of their respective companies.Copyright c 1985–2023StataCorp LLC,College Station,TX,USA.All rights reserved.。
人工智能领域中英文专有名词汇总
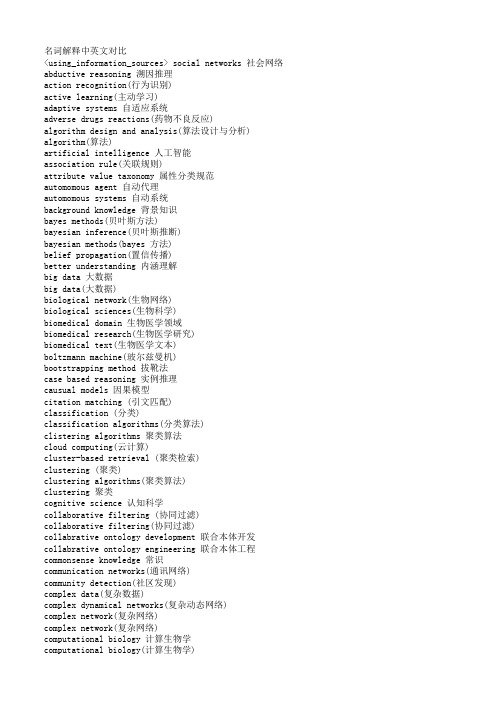
名词解释中英文对比<using_information_sources> social networks 社会网络abductive reasoning 溯因推理action recognition(行为识别)active learning(主动学习)adaptive systems 自适应系统adverse drugs reactions(药物不良反应)algorithm design and analysis(算法设计与分析) algorithm(算法)artificial intelligence 人工智能association rule(关联规则)attribute value taxonomy 属性分类规范automomous agent 自动代理automomous systems 自动系统background knowledge 背景知识bayes methods(贝叶斯方法)bayesian inference(贝叶斯推断)bayesian methods(bayes 方法)belief propagation(置信传播)better understanding 内涵理解big data 大数据big data(大数据)biological network(生物网络)biological sciences(生物科学)biomedical domain 生物医学领域biomedical research(生物医学研究)biomedical text(生物医学文本)boltzmann machine(玻尔兹曼机)bootstrapping method 拔靴法case based reasoning 实例推理causual models 因果模型citation matching (引文匹配)classification (分类)classification algorithms(分类算法)clistering algorithms 聚类算法cloud computing(云计算)cluster-based retrieval (聚类检索)clustering (聚类)clustering algorithms(聚类算法)clustering 聚类cognitive science 认知科学collaborative filtering (协同过滤)collaborative filtering(协同过滤)collabrative ontology development 联合本体开发collabrative ontology engineering 联合本体工程commonsense knowledge 常识communication networks(通讯网络)community detection(社区发现)complex data(复杂数据)complex dynamical networks(复杂动态网络)complex network(复杂网络)complex network(复杂网络)computational biology 计算生物学computational biology(计算生物学)computational complexity(计算复杂性) computational intelligence 智能计算computational modeling(计算模型)computer animation(计算机动画)computer networks(计算机网络)computer science 计算机科学concept clustering 概念聚类concept formation 概念形成concept learning 概念学习concept map 概念图concept model 概念模型concept modelling 概念模型conceptual model 概念模型conditional random field(条件随机场模型) conjunctive quries 合取查询constrained least squares (约束最小二乘) convex programming(凸规划)convolutional neural networks(卷积神经网络) customer relationship management(客户关系管理) data analysis(数据分析)data analysis(数据分析)data center(数据中心)data clustering (数据聚类)data compression(数据压缩)data envelopment analysis (数据包络分析)data fusion 数据融合data generation(数据生成)data handling(数据处理)data hierarchy (数据层次)data integration(数据整合)data integrity 数据完整性data intensive computing(数据密集型计算)data management 数据管理data management(数据管理)data management(数据管理)data miningdata mining 数据挖掘data model 数据模型data models(数据模型)data partitioning 数据划分data point(数据点)data privacy(数据隐私)data security(数据安全)data stream(数据流)data streams(数据流)data structure( 数据结构)data structure(数据结构)data visualisation(数据可视化)data visualization 数据可视化data visualization(数据可视化)data warehouse(数据仓库)data warehouses(数据仓库)data warehousing(数据仓库)database management systems(数据库管理系统)database management(数据库管理)date interlinking 日期互联date linking 日期链接Decision analysis(决策分析)decision maker 决策者decision making (决策)decision models 决策模型decision models 决策模型decision rule 决策规则decision support system 决策支持系统decision support systems (决策支持系统) decision tree(决策树)decission tree 决策树deep belief network(深度信念网络)deep learning(深度学习)defult reasoning 默认推理density estimation(密度估计)design methodology 设计方法论dimension reduction(降维) dimensionality reduction(降维)directed graph(有向图)disaster management 灾害管理disastrous event(灾难性事件)discovery(知识发现)dissimilarity (相异性)distributed databases 分布式数据库distributed databases(分布式数据库) distributed query 分布式查询document clustering (文档聚类)domain experts 领域专家domain knowledge 领域知识domain specific language 领域专用语言dynamic databases(动态数据库)dynamic logic 动态逻辑dynamic network(动态网络)dynamic system(动态系统)earth mover's distance(EMD 距离) education 教育efficient algorithm(有效算法)electric commerce 电子商务electronic health records(电子健康档案) entity disambiguation 实体消歧entity recognition 实体识别entity recognition(实体识别)entity resolution 实体解析event detection 事件检测event detection(事件检测)event extraction 事件抽取event identificaton 事件识别exhaustive indexing 完整索引expert system 专家系统expert systems(专家系统)explanation based learning 解释学习factor graph(因子图)feature extraction 特征提取feature extraction(特征提取)feature extraction(特征提取)feature selection (特征选择)feature selection 特征选择feature selection(特征选择)feature space 特征空间first order logic 一阶逻辑formal logic 形式逻辑formal meaning prepresentation 形式意义表示formal semantics 形式语义formal specification 形式描述frame based system 框为本的系统frequent itemsets(频繁项目集)frequent pattern(频繁模式)fuzzy clustering (模糊聚类)fuzzy clustering (模糊聚类)fuzzy clustering (模糊聚类)fuzzy data mining(模糊数据挖掘)fuzzy logic 模糊逻辑fuzzy set theory(模糊集合论)fuzzy set(模糊集)fuzzy sets 模糊集合fuzzy systems 模糊系统gaussian processes(高斯过程)gene expression data 基因表达数据gene expression(基因表达)generative model(生成模型)generative model(生成模型)genetic algorithm 遗传算法genome wide association study(全基因组关联分析) graph classification(图分类)graph classification(图分类)graph clustering(图聚类)graph data(图数据)graph data(图形数据)graph database 图数据库graph database(图数据库)graph mining(图挖掘)graph mining(图挖掘)graph partitioning 图划分graph query 图查询graph structure(图结构)graph theory(图论)graph theory(图论)graph theory(图论)graph theroy 图论graph visualization(图形可视化)graphical user interface 图形用户界面graphical user interfaces(图形用户界面)health care 卫生保健health care(卫生保健)heterogeneous data source 异构数据源heterogeneous data(异构数据)heterogeneous database 异构数据库heterogeneous information network(异构信息网络) heterogeneous network(异构网络)heterogenous ontology 异构本体heuristic rule 启发式规则hidden markov model(隐马尔可夫模型)hidden markov model(隐马尔可夫模型)hidden markov models(隐马尔可夫模型) hierarchical clustering (层次聚类) homogeneous network(同构网络)human centered computing 人机交互技术human computer interaction 人机交互human interaction 人机交互human robot interaction 人机交互image classification(图像分类)image clustering (图像聚类)image mining( 图像挖掘)image reconstruction(图像重建)image retrieval (图像检索)image segmentation(图像分割)inconsistent ontology 本体不一致incremental learning(增量学习)inductive learning (归纳学习)inference mechanisms 推理机制inference mechanisms(推理机制)inference rule 推理规则information cascades(信息追随)information diffusion(信息扩散)information extraction 信息提取information filtering(信息过滤)information filtering(信息过滤)information integration(信息集成)information network analysis(信息网络分析) information network mining(信息网络挖掘) information network(信息网络)information processing 信息处理information processing 信息处理information resource management (信息资源管理) information retrieval models(信息检索模型) information retrieval 信息检索information retrieval(信息检索)information retrieval(信息检索)information science 情报科学information sources 信息源information system( 信息系统)information system(信息系统)information technology(信息技术)information visualization(信息可视化)instance matching 实例匹配intelligent assistant 智能辅助intelligent systems 智能系统interaction network(交互网络)interactive visualization(交互式可视化)kernel function(核函数)kernel operator (核算子)keyword search(关键字检索)knowledege reuse 知识再利用knowledgeknowledgeknowledge acquisitionknowledge base 知识库knowledge based system 知识系统knowledge building 知识建构knowledge capture 知识获取knowledge construction 知识建构knowledge discovery(知识发现)knowledge extraction 知识提取knowledge fusion 知识融合knowledge integrationknowledge management systems 知识管理系统knowledge management 知识管理knowledge management(知识管理)knowledge model 知识模型knowledge reasoningknowledge representationknowledge representation(知识表达) knowledge sharing 知识共享knowledge storageknowledge technology 知识技术knowledge verification 知识验证language model(语言模型)language modeling approach(语言模型方法) large graph(大图)large graph(大图)learning(无监督学习)life science 生命科学linear programming(线性规划)link analysis (链接分析)link prediction(链接预测)link prediction(链接预测)link prediction(链接预测)linked data(关联数据)location based service(基于位置的服务) loclation based services(基于位置的服务) logic programming 逻辑编程logical implication 逻辑蕴涵logistic regression(logistic 回归)machine learning 机器学习machine translation(机器翻译)management system(管理系统)management( 知识管理)manifold learning(流形学习)markov chains 马尔可夫链markov processes(马尔可夫过程)matching function 匹配函数matrix decomposition(矩阵分解)matrix decomposition(矩阵分解)maximum likelihood estimation(最大似然估计)medical research(医学研究)mixture of gaussians(混合高斯模型)mobile computing(移动计算)multi agnet systems 多智能体系统multiagent systems 多智能体系统multimedia 多媒体natural language processing 自然语言处理natural language processing(自然语言处理) nearest neighbor (近邻)network analysis( 网络分析)network analysis(网络分析)network analysis(网络分析)network formation(组网)network structure(网络结构)network theory(网络理论)network topology(网络拓扑)network visualization(网络可视化)neural network(神经网络)neural networks (神经网络)neural networks(神经网络)nonlinear dynamics(非线性动力学)nonmonotonic reasoning 非单调推理nonnegative matrix factorization (非负矩阵分解) nonnegative matrix factorization(非负矩阵分解) object detection(目标检测)object oriented 面向对象object recognition(目标识别)object recognition(目标识别)online community(网络社区)online social network(在线社交网络)online social networks(在线社交网络)ontology alignment 本体映射ontology development 本体开发ontology engineering 本体工程ontology evolution 本体演化ontology extraction 本体抽取ontology interoperablity 互用性本体ontology language 本体语言ontology mapping 本体映射ontology matching 本体匹配ontology versioning 本体版本ontology 本体论open government data 政府公开数据opinion analysis(舆情分析)opinion mining(意见挖掘)opinion mining(意见挖掘)outlier detection(孤立点检测)parallel processing(并行处理)patient care(病人医疗护理)pattern classification(模式分类)pattern matching(模式匹配)pattern mining(模式挖掘)pattern recognition 模式识别pattern recognition(模式识别)pattern recognition(模式识别)personal data(个人数据)prediction algorithms(预测算法)predictive model 预测模型predictive models(预测模型)privacy preservation(隐私保护)probabilistic logic(概率逻辑)probabilistic logic(概率逻辑)probabilistic model(概率模型)probabilistic model(概率模型)probability distribution(概率分布)probability distribution(概率分布)project management(项目管理)pruning technique(修剪技术)quality management 质量管理query expansion(查询扩展)query language 查询语言query language(查询语言)query processing(查询处理)query rewrite 查询重写question answering system 问答系统random forest(随机森林)random graph(随机图)random processes(随机过程)random walk(随机游走)range query(范围查询)RDF database 资源描述框架数据库RDF query 资源描述框架查询RDF repository 资源描述框架存储库RDF storge 资源描述框架存储real time(实时)recommender system(推荐系统)recommender system(推荐系统)recommender systems 推荐系统recommender systems(推荐系统)record linkage 记录链接recurrent neural network(递归神经网络) regression(回归)reinforcement learning 强化学习reinforcement learning(强化学习)relation extraction 关系抽取relational database 关系数据库relational learning 关系学习relevance feedback (相关反馈)resource description framework 资源描述框架restricted boltzmann machines(受限玻尔兹曼机) retrieval models(检索模型)rough set theroy 粗糙集理论rough set 粗糙集rule based system 基于规则系统rule based 基于规则rule induction (规则归纳)rule learning (规则学习)rule learning 规则学习schema mapping 模式映射schema matching 模式匹配scientific domain 科学域search problems(搜索问题)semantic (web) technology 语义技术semantic analysis 语义分析semantic annotation 语义标注semantic computing 语义计算semantic integration 语义集成semantic interpretation 语义解释semantic model 语义模型semantic network 语义网络semantic relatedness 语义相关性semantic relation learning 语义关系学习semantic search 语义检索semantic similarity 语义相似度semantic similarity(语义相似度)semantic web rule language 语义网规则语言semantic web 语义网semantic web(语义网)semantic workflow 语义工作流semi supervised learning(半监督学习)sensor data(传感器数据)sensor networks(传感器网络)sentiment analysis(情感分析)sentiment analysis(情感分析)sequential pattern(序列模式)service oriented architecture 面向服务的体系结构shortest path(最短路径)similar kernel function(相似核函数)similarity measure(相似性度量)similarity relationship (相似关系)similarity search(相似搜索)similarity(相似性)situation aware 情境感知social behavior(社交行为)social influence(社会影响)social interaction(社交互动)social interaction(社交互动)social learning(社会学习)social life networks(社交生活网络)social machine 社交机器social media(社交媒体)social media(社交媒体)social media(社交媒体)social network analysis 社会网络分析social network analysis(社交网络分析)social network(社交网络)social network(社交网络)social science(社会科学)social tagging system(社交标签系统)social tagging(社交标签)social web(社交网页)sparse coding(稀疏编码)sparse matrices(稀疏矩阵)sparse representation(稀疏表示)spatial database(空间数据库)spatial reasoning 空间推理statistical analysis(统计分析)statistical model 统计模型string matching(串匹配)structural risk minimization (结构风险最小化) structured data 结构化数据subgraph matching 子图匹配subspace clustering(子空间聚类)supervised learning( 有support vector machine 支持向量机support vector machines(支持向量机)system dynamics(系统动力学)tag recommendation(标签推荐)taxonmy induction 感应规范temporal logic 时态逻辑temporal reasoning 时序推理text analysis(文本分析)text anaylsis 文本分析text classification (文本分类)text data(文本数据)text mining technique(文本挖掘技术)text mining 文本挖掘text mining(文本挖掘)text summarization(文本摘要)thesaurus alignment 同义对齐time frequency analysis(时频分析)time series analysis( 时time series data(时间序列数据)time series data(时间序列数据)time series(时间序列)topic model(主题模型)topic modeling(主题模型)transfer learning 迁移学习triple store 三元组存储uncertainty reasoning 不精确推理undirected graph(无向图)unified modeling language 统一建模语言unsupervisedupper bound(上界)user behavior(用户行为)user generated content(用户生成内容)utility mining(效用挖掘)visual analytics(可视化分析)visual content(视觉内容)visual representation(视觉表征)visualisation(可视化)visualization technique(可视化技术) visualization tool(可视化工具)web 2.0(网络2.0)web forum(web 论坛)web mining(网络挖掘)web of data 数据网web ontology lanuage 网络本体语言web pages(web 页面)web resource 网络资源web science 万维科学web search (网络检索)web usage mining(web 使用挖掘)wireless networks 无线网络world knowledge 世界知识world wide web 万维网world wide web(万维网)xml database 可扩展标志语言数据库附录 2 Data Mining 知识图谱(共包含二级节点15 个,三级节点93 个)间序列分析)监督学习)领域 二级分类 三级分类。
知识图谱 概念与技术:第12章 基于知识图谱的搜索与推荐
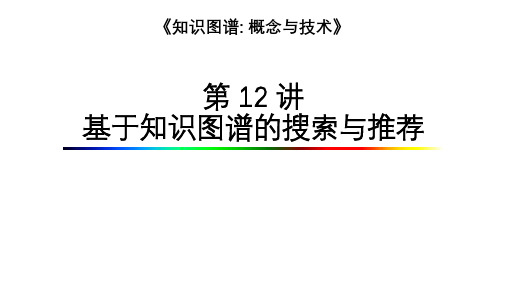
《知识图谱: 概念与技术》第12 讲基于知识图谱的搜索与推荐•基于知识图谱的搜索•搜索意图理解•实体探索•基于知识图谱的推荐•基于传统知识的推荐•基于知识图谱的物品画像•基于知识图谱的用户画像•基于知识图谱的跨领域推荐•基于知识图谱的搜索•搜索意图理解•实体探索•基于知识图谱的推荐•基于传统知识的推荐•基于知识图谱的物品画像•基于知识图谱的用户画像•基于知识图谱的跨领域推荐搜索的进化•Web搜索用户到底想搜什么内容?除了返回姚明的信息还能提供其他内容么?•Web搜索的进化•Web 搜索的进化keyword/stringthing/entity related things相关实体、概念潜在关系有关的属性确定搜索目标发现匹配结果匹配结果排序相关结果推荐搜索意图理解:•分词•规则解析•实体识别•实体链接•……•目标实体的属性展示•相关实体、概念的推荐•展现目标实体与相关实体间的关系•……•排序学习•目标实体、属性的查找•关联计算知识图谱•Web搜索的工作流程内容提纲•基于知识图谱的搜索•搜索意图理解•实体探索•基于知识图谱的推荐•基于传统知识的推荐•基于知识图谱的物品画像•基于知识图谱的用户画像•基于知识图谱的跨领域推荐搜索意图理解•因为搜索/查询语句一般都是短文本,因此搜索意图的理解最主要的挑战是短文本的实体链接•实体链接的基本任务•将指代实体的文本mention链接到知识库中特定实体的过程•实体链接的相关问题与挑战•实体解析/命名实体识别entity resolution/name entity recognition•共指消解co-reference resolution•词义消岐word sense disambiguation•……短文本实体链接•实体链接为什么是一个挑战?•同一个实体在广泛的文本中可能有多个mention(指代词)•Barack Obama•Barack H. Obama都是指美国前任总统奥巴马•President Obama•Senator Obama•President of the United States•同一个指代词可能指代多个不同实体•Michael Jordan 到底是指篮球巨星还是机器学习大牛?•“苹果”是指能吃的水果还是时尚的公司/手机?•算法目标•利用实体指代词m 与候选实体e 的上下文等相关特征计算两者的匹配度分数φe,m ,按分数进行排序,并选择分数最大的实体e best 作为m 的链接结果,即e best =argmax e φe,m •局部模型•为短文本中的每个指代词及其链接的实体单独计算φe,m ,每个链接实体都是独立产生•全局模型•考虑文本中多个指代词所链接的实体间联系,对上下文内所有歧义的实体指代一同消歧•令Γ={m 1,e 1,m 2,e 2…}为一个全局实体链接方案,则目标函数为:Γbest =argmax ΓO Γ=argmax Γi=1N φm i ,e i +t j ∈Γψe i ,e jψe i ,e j 是实体e i ,e j 之间的相关度分数不光考虑实体和指代词之间的相关度,还考虑•上下文特征•示例:...when did Steve leave apple...{Steve Jobs,Steve Wozniak,Steve Ballmer,...}句中提的到底是哪个Steve?•上下文特征•文本相似度•计算候选实体上下文文本(如百科页面)与指代词上下文的相似度•候选实体上下文:实体的百科页面(或摘要文字)、实体锚文本•指代词上下文:指代词所在的段落/文档、紧挨指代词前后的n个词•相似度模型:词袋向量、概念(主题)向量•上下文特征•实体间的相似度•计算候选实体e1与上下文中的实体e2的相似度分数,通常利用两个实体的邻居集合U1和U2进行比较•相似度/相关度计算指标:•Jaccard e1,e2=|U1∩U2||U1∪U2|•PMI e1,e2=U1∩U2/|W|U1/|W|∗|U2|/|W|•NGD e1,e2=1−log max|U1|,|U2|−log U1∩U2log|W|−log min|U1|,|U2|•AdamicAdar e1,e2=σn∈U1∩U2log1degree n•短文本实体链接的挑战•训练数据缺乏•难以训练出符合应用环境的监督模型•上下文中的其他实体少•局部模型起主导作用•上下文中的词语少•通常只提供了模糊的主题信息•“红楼梦是谁写的”•“写”->“文学作品”->红楼梦(四大名著之一)•主题的作用•因为短文本上下文的信息量少,利用主题是比较流行的做法•现有主题方法的不足•潜在的主题(LDA)•难以捕捉到实体的细粒度特征•难以解释•手工构造的主题•难以扩展•利用实体的概念作为主题•可以捕捉实体细粒度特征•大量的信息(实体的文章和属性)•大覆盖度(甚至囊括新实体或者长尾实体)•计算主题凝聚度[1]•首先实体与指代词之间的主题/概念相似度sim c m,e=cos v c m,v c ev c e是实体的概念向量,v c m是指代词上下文的概念向量,计算如下:v c m=w∈CT(m)v c w D(w,m)CT(m)是上下文词集合•词的概念向量v c w的每一维如下计算r w,c=e∈E n w,e·r(e,c)σr(e,c′)•再计算实体与指代词之间的文本相似度cos v w w c,v w w d sim t m,e=max wc∈CT m,w d∈KP(e)KP(e)是关键词组集合,从实体相关文档与属性中抽取•另外再考虑与上下文无关的特征相似度•实体流行度•实体名与指代词的相似度•综合几类相似度分数得到实体与指代词间的最终相似度φe,m•全局目标函数Γ=arg maxΓ′i=1Nφe,m i+ψΓ′•其中实体凝聚度(实体间的相关度)为ψΓ=e i∈Γ,e j∈Γcoℎe i,e j •短文本上下文中的实体少,因此•NP难的全局算法复杂度可以接受•不需要近似算法•计算实体凝聚度•结合实体相似度和相关度coℎe1,e2=γ·sim e e1,e2+1−γ·rel e1,e2•sim e e1,e2相似度:可考虑NGD距离•rel e1,e2相关度首先计算rel′e1,e2=r∈R(e1,e2)2T e1,r+|H(r,e2)|R(e1,e2)是e1,e2之间的关系集合,T e1,r={e′|e1,r,e′∈KG},H(r,e2)={e′|e′,r,e2∈KG}•像“配偶” 和“父母”这样对应少的关系会有大权值•像“国家” 和“出生地”这样对应多的关系会有小权值•由于逆关系通常对应的是同一个关系,因此两个方向取最大值得到rel e1,e2=max(rel′e1,e2,rel′e2,e1)内容提纲•基于知识图谱的搜索•搜索意图理解•实体探索•基于知识图谱的推荐•基于传统知识的推荐•基于知识图谱的物品画像•基于知识图谱的用户画像•基于知识图谱的跨领域推荐实体探索•探索目标实体本身以外更多的内容•展现实体的属性信息•发现(推荐)更多相关实体•KG中的邻居实体(包括直接邻居和高阶邻居)•对目标实体进行概念化的说明/解释•展现目标实体与相关实体间的关系•……•More than oneentity•问题定义[2]•对于给定的一个实体e s,并针对目标实体e所属的类型T及其与给定实体的关系描述R,为目标实体计算如下的概率,最后按照此概率对所有相关的目标实体进行排序并输出•实体共现相关度•实体类型过滤•利用百科实体页面中的分类信息•利用命名实体识别工具•上下文建模t 是关系描述R 中的一个词,是实体e 与e s 的共现语言模型,t 越多地出现在e 与e s 的共现文档集合中,则越大前述的计算两个实体相似度的方法都可适用•问题定义[3]•给定由一组实体代表的查询q ,产生一个(组)概念能完美解释给定实体间的潜在联系•q 中包括搜索实体与推荐的相关实体,因此产生的概念是发现相关实体的基础BRICEmerging economy Growing Market Chinese internet giantChinese companyCountryCompany应该推荐什么相关实体?•算法描述•寻找的概念c i应满足下述目标1. Probabilistic Relevance Modelargmax e∈E−q rel q,e=iP e c i P c i qδ(c i)2. Relative Entropy Modelargmin e∈E−q KL P C q,P C q,e=i=1nδ(c i)P c i q log(P(c i|q)P(c i|q,e))利用Probase发现概念与实体间的关系找到的概念既要有代表性又要能很好地在最优的粒度层级上解释所推荐的相关实体•计算P(c i |q)1. Naïve Bayes ModelP c i q =P(q|c i )P(c i )P(q)∝ෑe j ∈qP(e j |c i )P(c i )∝P(c i )ෑe j ∈q,n e j ,c i >0λP(e j |c i )ෑe j ∈q,n e j ,c i =0(1−λ)P(e j )2. Noisy-or ModelP c i q =1−ෑe j ∈q(1−P(c i |e j ))•计算δ(c i)•用于度量目标概念的粒度,好的概念既不能太一般化也不能太具体化Concept Number of EntitiesCountry2648Developing country149Growing market18 Entity-based ApproachChina India BrazilDevelopingCountryCountryHierarchy-based Approach 距离q中实体更近的概念更值得考虑•计算δ(c i)1. Entity-based Approach•Penalize popular concepts•δc i=1P(c i)2. Hierarchy-based Approach(Average first passage time)•argmaxC q k σc∈C q kσqi∈qℎ(q i|c)•൝ℎq i c=0,if q i=c ℎq i c=1+σc′∈c(c′)P c′q iℎ(c′|c)if q i≠c•发现实体间的潜在关联具有重要应用价值•KG为实体间的关系提供了数据支撑•挑战:两个实体间的关联路径可能有多条哪种关系才是最该展现的?ISIS头目与一位伊朗少将之间的关联路径[4]•问题转化为对实体间的各条路径进行排序,主要考虑三个要素[4]•Specificity:流行的实体得分要低(类似IDF基本思想)score1p=σe∈p spec e,spe p=log(1+1/docCount(e))•Connectivity:路径中一条边e1,e2的权重与e1和e2的相似度成正比score2p=σ(e1,e2)∈p sim e1,e2,sim e1,e2=cos(e1,e2)•Cohesiveness:要考虑紧挨着的两条边(三个实体)之间的凝聚度score3p=(e1,e2,e3)∈psim e1+e2,e3•最终,score p=score1p×score2p×score3pe1是DSM模型[5]产生的实体向量,也可以用前述的方法计算两个实体的相似度•展现实体关系图谱•基于实体间发现的重要关系,可将目标实体与所有挖掘出的相关实体一同展现到一个关系图谱中,为搜索用户提供更加丰富的信息参考文献[1]L.Chen,J.Liang,C.Xie and Yanghua Xiao.“Short Text Entity Linking with Fine-grained Topics”.CIKM(2018).[2]Bron, Marc, K. Balog, and M. D. Rijke. “Ranking related entities: components and analyses.”ACM International Conference on Information and Knowledge Management ACM, 2010:1079-1088.[3]Y.Zhang, Yanghua Xiao et al. “Entity Suggestion with Conceptual Expanation”.IJCAI(2017).[4]Aggarwal, Nitish, S. Bhatia, and V. Misra. “Connecting the Dots: Explaining Relationships Between Unconnected Entities in a Knowledge Graph.” (2016).[5]N. Aggarwal and P. Buitelaar. Wikipedia-based distributional semantics for entity relatedness. In AAAI Fall Symposium Series, 2014.内容提纲•基于知识图谱的搜索•搜索意图理解•实体探索•基于知识图谱的推荐•基于传统知识的推荐•基于知识图谱的物品画像•基于知识图谱的用户画像•基于知识图谱的跨领域推荐电影(豆瓣)图书(亚马逊)推荐系统应用挂广泛•推荐算法的目标p(i|u)或者f:U×I Rargmaxi∈I•推荐算法分类•基于协同过滤:p(i|u,behavior(u))•基于记忆•基于模型•基于内容:p(i|u,content(u,i))•混合方法•基于知识:p(i|u,knowledge)•推荐算法的基本框架精准的用户/物品画像是关键•传统推荐算法的挑战•基于协同过滤•冷启动•数据稀疏•可扩展性•……•基于内容•特征描述•同义/多义词•结果同质性•……❑提高精准度(precision )❑知识图谱为物品引入了更多的语义关系❑知识图谱可以深层次地发现用户兴趣推荐系统中引入知识图谱的优势:喜欢盗梦空间小李子泰坦尼克号主演主演可能喜欢❑增加多样性(diversity)❑知识图谱提供了不同的关系连接种类❑有利于推荐结果的发散,避免推荐结果越来越局限于单一类型推荐系统中引入知识图谱的优势:喜欢盗梦空间小李子泰坦尼克号主演主演科幻黑客帝国克里斯托弗·若兰敦刻尔克题材题材导演导演推荐系统中引入知识图谱的优势:❑可解释性(interpretability)❑知识图谱可以连接用户的兴趣历史和推荐结果❑提高用户对推荐结果的满意度和接受度,增强用户对推荐系统的信任喜欢盗梦空间你可能也喜欢:泰坦尼克号,因为它们有相同的主演;黑客帝国,因为它们有相同的题材;敦刻尔克,因为它们有相同的导演;……内容提纲•基于知识图谱的搜索•搜索意图理解•实体探索•基于知识图谱的推荐•基于传统知识的推荐•基于知识图谱的物品画像•基于知识图谱的用户画像•基于知识图谱的跨领域推荐•基于约束的知识化推荐•什么是约束知识?•通过用户的输入限定物品属性值形成规则集合,形成候选物品的范围约束——关于用户的知识•例如:电影的演员、歌曲的演唱者、餐馆的菜系、手机的价位等类似基于输入条件的查询大众点评餐馆查询/推荐的属性选择页面•基于个案的知识化推荐•什么是个案知识?•先通过某种算法产生一组候选物品给用户选择,将用户的选择作为参照物,再通过物品间的相似性计算找出其他与参照物品高度相似的候选物品,再让用户进一步选择,多次与用户的迭代交互,直至最终产生用户最想要的物品类似问答式的搜索•传统的推荐系统对知识的理解不同于KG的知识•用户的标签、社交网络、商品的目录等信息,只要是有助于发现用户个性偏好和物品特征的数据都曾被看作是知识传统用户/物品知识vs知识图谱知识•传统知识化推荐的挑战•物品知识的获取•系统需要人工构建知识,对长尾实体的覆盖有限•用户知识的获取•系统需要用户输入信息,甚至要反复交互,体验感差知识图谱的出现为解决这些问题带来契机!内容提纲•基于知识图谱的搜索•搜索意图理解•实体探索•基于知识图谱的推荐•基于传统知识的推荐•基于知识图谱的物品画像•基于知识图谱的用户画像•基于知识图谱的跨领域推荐•基本算法目标p(i|u,knowledge(i))argmaxi∈I•基于向量空间模型[2]•为每种属性生成一个表示向量,每一维对应该属性的某个值的权重•例如,电影的演员属性可以表示成一个向量,第一维的值可以是第1号演员对该电影的TF-IDF权重值TF-IDF值如何计算?•两部电影在某种属性上的相似度可以计算为该属性的两个向量的距离•两部电影的相似度则是所有属性相似度的综合,例如加权和或加权平均每种属性的权重如何考虑?•基于向量空间模型[2]用户u对电影m i的喜好评分按照以下公式计算:属性p的权重电影I和j在属性p上的相似度。
算法常用术语中英对照

算法常用术语中英对照Data Structures 基本数据结构Dictionaries 字典Priority Queues 堆Graph Data Structures 图Set Data Structures 集合Kd-Trees 线段树Numerical Problems 数值问题Solving Linear Equations 线性方程组Bandwidth Reduction 带宽压缩Matrix Multiplication 矩阵乘法Determinants and Permanents 行列式Constrained and Unconstrained Optimization 最值问题Linear Programming 线性规划Random Number Generation 随机数生成Factoring and Primality Testing 因子分解/质数判定Arbitrary Precision Arithmetic 高精度计算Knapsack Problem 背包问题Discrete Fourier Transform 离散Fourier变换Combinatorial Problems 组合问题Sorting 排序Searching 查找Median and Selection 中位数Generating Permutations 排列生成Generating Subsets 子集生成Generating Partitions 划分生成Generating Graphs 图的生成Calendrical Calculations 日期Job Scheduling 工程安排Satisfiability 可满足性Graph Problems -- polynomial 图论-多项式算法Connected Components 连通分支Topological Sorting 拓扑排序Minimum Spanning Tree 最小生成树Shortest Path 最短路径Transitive Closure and Reduction 传递闭包Matching 匹配Eulerian Cycle / Chinese Postman Euler回路/中国邮路Edge and Vertex Connectivity 割边/割点Network Flow 网络流Drawing Graphs Nicely 图的描绘Drawing Trees 树的描绘Planarity Detection and Embedding 平面性检测和嵌入Graph Problems -- hard 图论-NP问题Clique 最大团Independent Set 独立集Vertex Cover 点覆盖Traveling Salesman Problem 旅行商问题Hamiltonian Cycle Hamilton回路Graph Partition 图的划分Vertex Coloring 点染色Edge Coloring 边染色Graph Isomorphism 同构Steiner Tree Steiner树Feedback Edge/Vertex Set 最大无环子图Computational Geometry 计算几何Convex Hull 凸包Triangulation 三角剖分Voronoi Diagrams Voronoi图Nearest Neighbor Search 最近点对查询Range Search 范围查询Point Location 位置查询Intersection Detection 碰撞测试Bin Packing 装箱问题Medial-Axis Transformation 中轴变换Polygon Partitioning 多边形分割Simplifying Polygons 多边形化简Shape Similarity 相似多边形Motion Planning 运动规划Maintaining Line Arrangements 平面分割Minkowski Sum Minkowski和Set and String Problems 集合与串的问题Set Cover 集合覆盖Set Packing 集合配置String Matching 模式匹配Approximate String Matching 模糊匹配Text Compression 压缩Cryptography 密码Finite State Machine Minimization 有穷自动机简化Longest Common Substring 最长公共子串Shortest Common Superstring 最短公共父串DP——Dynamic Programming——动态规划recursion ——递归编程词汇A2A integration A2A整合abstract 抽象的abstract base class (ABC)抽象基类abstract class 抽象类abstraction 抽象、抽象物、抽象性access 存取、访问access level访问级别access function 访问函数account 账户action 动作activate 激活active 活动的actual parameter 实参adapter 适配器add-in 插件address 地址address space 地址空间address-of operator 取地址操作符ADL (argument-dependent lookup)ADO(ActiveX Data Object)ActiveX数据对象advanced 高级的aggregation 聚合、聚集algorithm 算法alias 别名align 排列、对齐allocate 分配、配置allocator分配器、配置器angle bracket 尖括号annotation 注解、评注API (Application Programming Interface) 应用(程序)编程接口app domain (application domain)应用域application 应用、应用程序application framework 应用程序框架appearance 外观append 附加architecture 架构、体系结构archive file 归档文件、存档文件argument引数(传给函式的值)。
中山大学计算机学院离散数学基础教学大纲(2019)
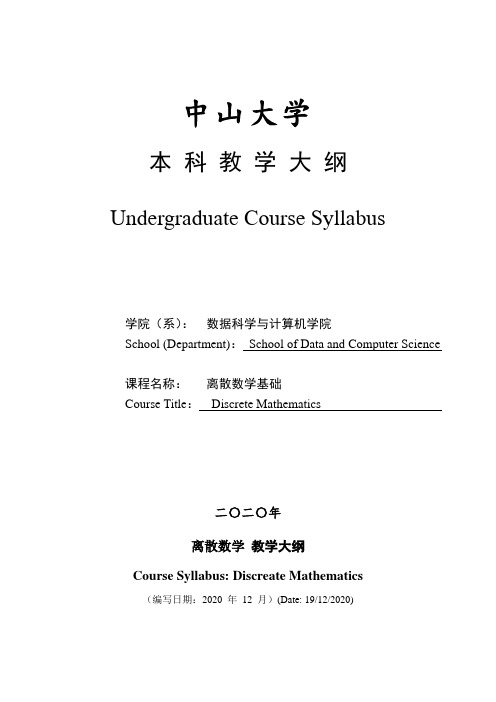
中山大学本科教学大纲Undergraduate Course Syllabus学院(系):数据科学与计算机学院School (Department):School of Data and Computer Science课程名称:离散数学基础Course Title:Discrete Mathematics二〇二〇年离散数学教学大纲Course Syllabus: Discreate Mathematics(编写日期:2020 年12 月)(Date: 19/12/2020)一、课程基本说明I. Basic Information二、课程基本内容 II. Course Content(一)课程内容i. Course Content1、逻辑与证明(22学时) Logic and Proofs (22 hours)1.1 命题逻辑的语法和语义(4学时) Propositional Logic (4 hours)命题的概念、命题逻辑联结词和复合命题,命题的真值表和命题运算的优先级,自然语言命题的符号化Propositional Logic, logic operators (negation, conjunction, disjunction, implication, bicondition), compound propositions, truth table, translating sentences into logic expressions1.2 命题公式等值演算(2学时) Logical Equivalences (2 hours)命题之间的关系、逻辑等值和逻辑蕴含,基本等值式,等值演算Logical equivalence, basic laws of logical equivalences, constructing new logical equivalences1.3 命题逻辑的推理理论(2学时)论断模式,论断的有效性及其证明,推理规则,命题逻辑中的基本推理规则(假言推理、假言易位、假言三段论、析取三段论、附加律、化简律、合取律),构造推理有效性的形式证明方法Argument forms, validity of arguments, inference rules, formal proofs1.4 谓词逻辑的语法和语义 (4学时) Predicates and Quantifiers (4 hours)命题逻辑的局限,个体与谓词、量词、全程量词与存在量词,自由变量与约束变量,谓词公式的真值,带量词的自然语言命题的符号化Limitations of propositional logic, individuals and predicates, quantifiers, the universal quantification and conjunction, the existential quantification and disjunction, free variables and bound variables, logic equivalences involving quantifiers, translating sentences into quantified expressions.1.4 谓词公式等值演算(2学时) Nested Quantifiers (2 hours)谓词公式之间的逻辑蕴含与逻辑等值,带嵌套量词的自然语言命题的符号化,嵌套量词与逻辑等值Understanding statements involving nested quantifiers, the order of quantifiers, translating sentences into logical expressions involving nested quantifiers, logical equivalences involving nested quantifiers.1.5谓词逻辑的推理规则和有效推理(4学时) Rules of Inference (4 hours)证明的基本含和证明的形式结构,带量词公式的推理规则(全程量词实例化、全程量词一般化、存在量词实例化、存在量词一般化),证明的构造Arguments, argument forms, validity of arguments, rules of inference for propositional logic (modus ponens, modus tollens, hypothetical syllogism, disjunctive syllogism, addition, simplication, conjunction), using rules of inference to build arguments, rules of inference for quantified statements (universal instantiation, universal generalization, existential instantiation, existential generalization)1.6 数学证明简介(2学时) Introduction to Proofs (2 hours)数学证明的相关术语、直接证明、通过逆反命题证明、反证法、证明中常见的错误Terminology of proofs, direct proofs, proof by contraposition, proof by contradiction, mistakes in proofs1.7 数学证明方法与策略初步(2学时) Proof Methods and Strategy (2 hours)穷举法、分情况证明、存在命题的证明、证明策略(前向与后向推理)Exhaustive proof, proof by cases, existence proofs, proof strategies (forward and backward reasoning)2、集合、函数和关系(18学时)Sets, Functions and Relations(18 hours)2.1 集合及其运算(3学时) Sets (3 hours)集合与元素、集合的表示、集合相等、文氏图、子集、幂集、笛卡尔积Set and its elements, set representations, set identities, Venn diagrams, subsets, power sets, Cartesian products.集合基本运算(并、交、补)、广义并与广义交、集合基本恒等式Unions, intersections, differences, complements, generalized unions and intersections, basic laws for set identities.2.2函数(3学时) Functions (3 hours)函数的定义、域和共域、像和原像、函数相等、单函数与满函数、函数逆与函数复合、函数图像Functions, domains and codomains, images and pre-images, function identity, one-to-one and onto functions, inverse functions and compositions of functions.2.3. 集合的基数(1学时)集合等势、有穷集、无穷集、可数集和不可数集Set equinumerous, finite set, infinite set, countable set, uncountable set.2.4 集合的归纳定义、归纳法和递归(3学时)Inductive sets, inductions and recursions (3 hours)自然数的归纳定义,自然数上的归纳法和递归函数;数学归纳法(第一数学归纳法)及应用举例、强归纳法(第二数学归纳法)及应用举例;集合一般归纳定义模式、结构归纳法和递归函数。
哈工大知识图谱(KnowledgeGraph)课程概述
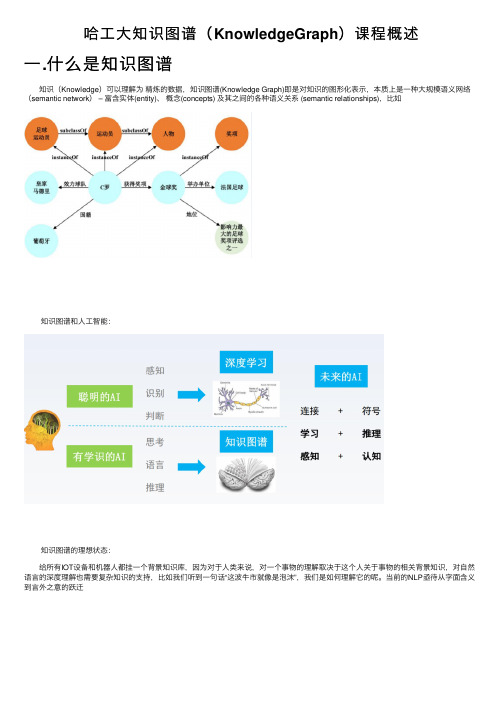
1.知 识 图 谱 中 的 概 念
实体 (entity):现实世界中可区分、可识别的事物或概念。 ➢ 客观对象:人物、地点、机构 ➢ 抽象事件:电影、奖项、赛事 关系 (relation):实体和实体之间的语义关联。 事实 (fact):陈述两个实体之间关系的断言,通常表示为 (head entity, relation, tail entity) 三元组形式。
四 .实体识别
1.信 息 抽 取
概念:从自然语言文本中抽取指定类型的实体、关系、事件等事实信息, 并形成结构化数据输出的文本处理技术
主要任务:实体识别与抽取,关系抽取,时间抽取,实体消歧
2.命 名 实 体 识 别 ( Named Entity Recognition, 简 称 NER)
定义:狭义地讲,命名实体指现实世界中具体或抽象的实体 , 如人(张三)、机构(哈尔滨工业大学)、地点等,通常用唯一的标志符(专 有名称)表示。
Ontology(本体):通过对概念的严格定义和概念与概念之间的关系来确定概念的精确含义,表示共同认可的、可共享的知识,对于 ontology来说,author,creator和writer是同一个 概念,而doctor在大学和医院分别表示的是两个概念。因 此在语义网中,ontology具有非常 重要的地位,是解决语义层次上Web信息共享和交换的基础。简单理解就是某个领域关于自身和相关关系的描述
2.知 识 图 谱 的 特 性
知识图谱不太专注于对知识框架的定义,而专注于如何以工程的方式,从文本中自动抽取或依靠众包的方式获取并 组建广泛的、具有平铺结 构的知识实例,最后再要求使用 它的方式具有容错、模糊匹配等机制。 知识图谱的真正魅力在于其图结构,可以在知识图谱上运行搜索、 随机游走、网络流等大规模图算法,使知识图谱与图论、概率图等碰撞出火花。
人工智能之知识图谱

图表目录图1知识工程发展历程 (3)图2 Knowledge Graph知识图谱 (9)图3知识图谱细分领域学者选取流程图 (10)图4基于离散符号的知识表示与基于连续向量的知识表示 (11)图5知识表示与建模领域全球知名学者分布图 (13)图6知识表示与建模领域全球知名学者国家分布统计 (13)图7知识表示与建模领域中国知名学者分布图 (14)图8知识表示与建模领域各国知名学者迁徙图 (14)图9知识表示与建模领域全球知名学者h-index分布图 (15)图10知识获取领域全球知名学者分布图 (23)图11知识获取领域全球知名学者分布统计 (23)图12知识获取领域中国知名学者分布图 (23)图13知识获取领域各国知名学者迁徙图 (24)图14知识获取领域全球知名学者h-index分布图 (24)图15 语义集成的常见流程 (29)图16知识融合领域全球知名学者分布图 (31)图17知识融合领域全球知名学者分布统计 (31)图18知识融合领域中国知名学者分布图 (31)图19知识融合领域各国知名学者迁徙图 (32)图20知识融合领域全球知名学者h-index分布图 (32)图21知识查询与推理领域全球知名学者分布图 (39)图22知识查询与推理领域全球知名学者分布统计 (39)图23知识查询与推理领域中国知名学者分布图 (39)图24知识表示与推理领域各国知名学者迁徙图 (40)图25知识查询与推理领域全球知名学者h-index分布图 (40)图26知识应用领域全球知名学者分布图 (46)图27知识应用领域全球知名学者分布统计 (46)图28知识应用领域中国知名学者分布图 (47)图29知识应用领域各国知名学者迁徙图 (47)图30知识应用领域全球知名学者h-index分布图 (48)图31行业知识图谱应用 (68)图32电商图谱Schema (69)图33大英博物院语义搜索 (70)图34异常关联挖掘 (70)图35最终控制人分析 (71)图36企业社交图谱 (71)图37智能问答 (72)图38生物医疗 (72)图39知识图谱领域近期热度 (75)图40知识图谱领域全局热度 (75)表1知识图谱领域顶级学术会议列表 (10)表2 知识图谱引用量前十论文 (56)表3常识知识库型指示图 (67)1.概念篇1.1.知识图谱概念和分类知识图谱(Knowledge Graph)以结构化的形式描述客观世界中概念、实体及其之间的关系,将互联网的信息表达成更接近人类认知世界的形式,提供了一种更好地组织、管理和理解互联网海量信息的能力。
knowledge graph例子
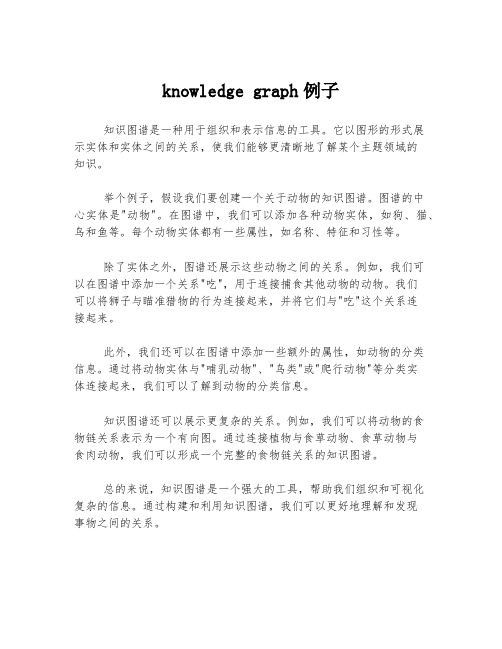
knowledge graph例子
知识图谱是一种用于组织和表示信息的工具。
它以图形的形式展
示实体和实体之间的关系,使我们能够更清晰地了解某个主题领域的
知识。
举个例子,假设我们要创建一个关于动物的知识图谱。
图谱的中
心实体是"动物"。
在图谱中,我们可以添加各种动物实体,如狗、猫、鸟和鱼等。
每个动物实体都有一些属性,如名称、特征和习性等。
除了实体之外,图谱还展示这些动物之间的关系。
例如,我们可
以在图谱中添加一个关系"吃",用于连接捕食其他动物的动物。
我们
可以将狮子与瞄准猎物的行为连接起来,并将它们与"吃"这个关系连
接起来。
此外,我们还可以在图谱中添加一些额外的属性,如动物的分类
信息。
通过将动物实体与"哺乳动物"、"鸟类"或"爬行动物"等分类实
体连接起来,我们可以了解到动物的分类信息。
知识图谱还可以展示更复杂的关系。
例如,我们可以将动物的食
物链关系表示为一个有向图。
通过连接植物与食草动物、食草动物与
食肉动物,我们可以形成一个完整的食物链关系的知识图谱。
总的来说,知识图谱是一个强大的工具,帮助我们组织和可视化
复杂的信息。
通过构建和利用知识图谱,我们可以更好地理解和发现
事物之间的关系。
- 1、下载文档前请自行甄别文档内容的完整性,平台不提供额外的编辑、内容补充、找答案等附加服务。
- 2、"仅部分预览"的文档,不可在线预览部分如存在完整性等问题,可反馈申请退款(可完整预览的文档不适用该条件!)。
- 3、如文档侵犯您的权益,请联系客服反馈,我们会尽快为您处理(人工客服工作时间:9:00-18:30)。
1 Introduction
Di erent kinds of labelled graphs have long been used for representing knowledge. In arti cial intelligence, they have been investigated under the name of semantic networks (see for instance Leh92] for an overview of recent research in this domain). Sow76] proposed a model of this family, named conceptual graphs (CGs), which was developed in Sow84]. The formal aspects of this model have two mathematical bases: logic and graph theory. Emphasizing one or the other leads to di erent research lines. The former, a continuation of Peirce's work on existential graphs Rob92], investigates CGs as a diagrammatic system of logic (for instance Sow94] vdB94]). The later develops CG as a graphical knowledge representation model, where \graphical" is used in the sense
application we are currently involved in. In this application, NGs are used to represent and simulate the required behavior of human agents in case of emergency situations, for instance a re in a chemical plant BBV97]. This behavior is described by NGs which represent situations, pre-conditions and postconditions of actions to be performed, each of these elements being themselves described by NGs. Knowledge is structured by hierarchical levels and reasonings must respect these levels. The paper is organized as follows. In section 2, basic de nitions and results on SGs are provided. In section 3, co-reference links are added to SGs and we propose a new logical semantics, which is compared to . We prove that projection is sound and complete w.r.t. this semantics. The model is then extended to NGs with co-reference links (section 4). Two logical semantics for NGs are developed, for which projection is proven to be sound and complete (sections 5 and 6). Section 7 is devoted to related works.
Person:Peter
2
ation
2
Billboard:*
object
2
Announcement:*
This graph may represent the information \Peter sticks an announcement on a billboard".
Figure 1: A simple graph to the degree of the relation node. The ith neighbor of a relation r is denoted by Gi (r). Each node has a label given by the mapping l. A concept node c is labelled by a couple (type(c); ref (c)), where type(c), the type of c, belongs to TC , and ref (c), the referent of c, either belongs to I | then c is said to be an individual concept node | or is the generic marker | then c is said to be a generic concept node. A relation node r is labelled by a relation type, type(r), and the number of edges incident on r is equal to the arity of type(r). Figure 1 gives an example of a SG. SGs are given a semantics in FOL, denoted by Sow84]. Given a support S , a constant is assigned to each individual marker and an n-adic (resp. unary) predicate is assigned to each n-adic relation (resp. concept) type. For simplicity, we consider that each constant or predicate has the same name as the associated element of the support. To S is assigned a set of formulas, (S ), which corresponds to the interpretation of the partial orderings of TR and TC . For all types t1 and t2 such that t1 t2 , one has the formula 8x1 :::xp (t2 (x1 ; :::; xp ) ! t1 (x1 ; :::; xp )), where p = 1 for concept types, and p is otherwise the arity of the relation. maps any graph G on S into a formula (G) in the following way. First assign to each concept node c a term which is a variable if c is generic, and otherwise the constant corresponding to ref (c). Two distinct generic nodes receive distinct variables. Then assign an atom to each node of G: the atom tc (e) to a concept c where tc stands for the type of c and e is the term associated with c; the atom tr (e1 ; :::; ep ) to a relation r where tr stands for the type of r and ei is the term associated with the ith neighbor of r. Finally, the conjunction of these atoms is made and the obtained formula is existentially closed. E.g. let us consider the graph G of Figure 1. We call c1 , c2 , c3 , c4 the concept nodes of respective types Person, Stick, Announcement, Billboard and assign the variable yi to the generic concept node ci for i = 2; 3; 4. The formula associated with G is
M. Chein
M.L. Mugnier
LIRMM
G. Simonet
LIRMM
Abstract
We present a graph-based KR model issued from Sowa's conceptual graphs but studied and developed with a speci c approach. Formal objects are kinds of labelled graphs, which may be simple graphs or nested graphs. The fundamental notion for doing reasonings, called projection (or subsumption), is a kind of labelled graph morphism. Thus, we propose a graphical KR model, where \graphical " is used in the sense of Sch91], i.e. a model that \uses graph-theoretic notions in an essential and nontrivial way". Indeed, morphism, which is the fundamental notion for any structure, is at the core of our theory. We de ne two rst order logic semantics, which correspond to di erent intuitive semantics, and prove in both cases that projection is sound and complete with respect to deduction.