Adaptive Action Selection of Body Expansion Behavior in Multi-Robot System using Communicat
《2024年髋部骨折患者应用髂筋膜阻滞术前镇痛效果的Meta分析》范文
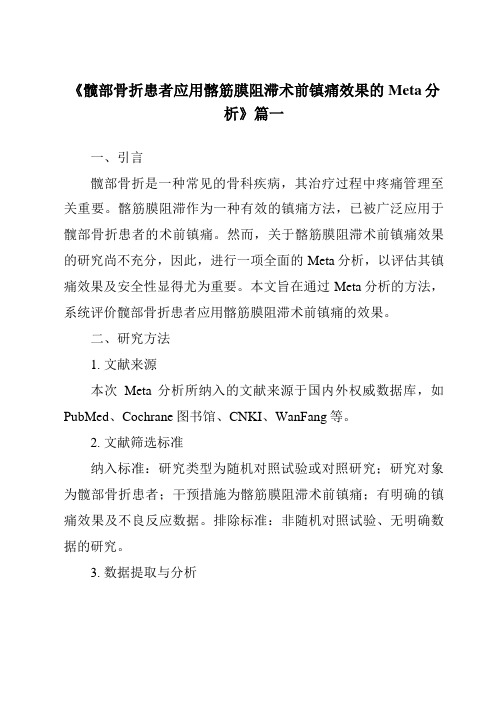
《髋部骨折患者应用髂筋膜阻滞术前镇痛效果的Meta分析》篇一一、引言髋部骨折是一种常见的骨科疾病,其治疗过程中疼痛管理至关重要。
髂筋膜阻滞作为一种有效的镇痛方法,已被广泛应用于髋部骨折患者的术前镇痛。
然而,关于髂筋膜阻滞术前镇痛效果的研究尚不充分,因此,进行一项全面的Meta分析,以评估其镇痛效果及安全性显得尤为重要。
本文旨在通过Meta分析的方法,系统评价髋部骨折患者应用髂筋膜阻滞术前镇痛的效果。
二、研究方法1. 文献来源本次Meta分析所纳入的文献来源于国内外权威数据库,如PubMed、Cochrane图书馆、CNKI、WanFang等。
2. 文献筛选标准纳入标准:研究类型为随机对照试验或对照研究;研究对象为髋部骨折患者;干预措施为髂筋膜阻滞术前镇痛;有明确的镇痛效果及不良反应数据。
排除标准:非随机对照试验、无明确数据的研究。
3. 数据提取与分析采用专业的文献管理软件进行数据提取与整理,包括研究基本信息、患者基本信息、干预措施、镇痛效果及不良反应等。
使用RevMan软件进行Meta分析。
三、结果1. 文献概况共纳入15篇研究,涉及患者总数为1236例。
其中,实验组(髂筋膜阻滞术前镇痛)与对照组(其他镇痛方法或无镇痛)各7篇研究。
2. 镇痛效果Meta分析结果显示,髋部骨折患者应用髂筋膜阻滞术前镇痛在术后各时间点的疼痛程度均明显低于对照组(P<0.05)。
具体表现为术后即刻、术后6小时、术后12小时及术后24小时的疼痛程度均有显著差异。
此外,髂筋膜阻滞在改善患者的疼痛程度、缓解疼痛的持续时间等方面也具有明显优势。
3. 安全性评价在安全性方面,髂筋膜阻滞术前镇痛并未增加患者的不良反应发生率,如恶心、呕吐、呼吸抑制等。
同时,髂筋膜阻滞对患者的生命体征无明显影响,具有良好的安全性。
四、讨论本Meta分析表明,髋部骨折患者应用髂筋膜阻滞术前镇痛效果显著,能显著降低术后各时间点的疼痛程度,且安全性良好。
康复英语词汇校正部分

1. 最大心率AAMHR2. 腹式呼吸运动abdominal breathing exercises3. 擦浴ablution4. 可进入;可使用accessibility5. 适应比值accommodation ratio6. 主动助力运动active assistant exercises7. 主动运动active exercises8. 日常生活活动activities of daily living, ADL9. 日常生活活动Activities of Daily Living,ADL10. 适应性活动adaptive activity11. 有氧训练aerobic training, aerobic exercise12. 空气离子化疗法aeroionotherapy13. 空气疗法aerotherapy14. 冲浴afusion15. 步行训练ambulation training16. 减痛步态antalgic gait17. 失语症aphasia18. 失用症apraxia19. 水疗,水中运动治疗aqua-therapy20. 氩离子激光疗法argon ion laser therapy21. 假肢artificial limb22. 工艺疗法arts and crafts therapy23. 助力运动assistant exercises24. 辅助器具Assistive Devices25. 联合运动associated movement26. 共济失调ataxia27. 手足徐动athetoid28. 自我训练autotraining29. 轴索断裂axonotmesis30. 八段锦Baduanjin31. 平衡运动balance exercises32. 无障碍通行barrier free accessibility, barrier free passage33. Barthel指数Bartbel index34. 巴氏指数评分Barthel Index, BI35. 狭义ADL,基本ADLBasic ADL, BADL36. 床上运动bed exercises37. 行为疗法behavior therapy38. 行为模式behavioral model39. 行为理论behavioral theory40. 阅读治疗bibliotherapy41. 生物反馈疗法biofeedback therapy, BFT42. 眨眼反射blink reflex43. 蓝紫光疗法blue and violet light therapy44. 支架brace45. 脑可塑性Brain Plasticity46. 脑干听觉诱发电位brainstem auditory evoked potential, BAEP47. 屏气试验breath holding test48. 呼吸运动breathing exercises49. 盐水浴brine bath50. 气泡浴bubble bath51. 蝶形槽浴butterfly shaped tank bath, Hubbard tank bath52. 手杖cane, walking stick53. 二氧化碳激光疗法carbondioxide laser therapy54. 木工疗法carpentry therapy55. 雕刻疗法carving therapy56. 厘米波疗法centimeter wave therapy57. 小脑共济失调步态cerebellar ataxic gait58. 脑瘫cerebral palsy59. 推拿Chinese manipulation, traditional manipulation60. 中医传统作业疗法Chinese Style OT61. 脊柱推拿法chiropractic62. 时值chronaxia, CHR63. 循环训练(法)circuit training64. 古典式电诊断法classical electrodiagnosis65. 以患者为中心的治疗Client-centered Intervention66. 气候疗法climatotherapy67. 攀登运动climbing exercises68. 认知干预Cognitive Intervention69. 认知疗法cognitive therapy70. 认知训练cognitive training71. 冷敷cold compress72. 冷疗法cold therapy73. 社基康复community-based-rehabilitation, CBR74. 补偿适应Compensation/Adaptation75. 全面康复comprehensive rehabilitation76. 压力衣Compression Garments, Pressure Suit77. 加压疗法compression therapy78. 向心收缩concentric contraction79. 保健操conditioning exercises80. 传导阻滞conduction block81. 传导热疗法conductive heat therapy82. 冷热交替浴contrast bath83. 整理活动cooling-down84. 协调运动coordination exercises85. 紧张控制技术模式coping skill model86. 矫正运动corrective exercises87. 皮层重组Cortical Reorganization88. 爬行运动crawling exercises89. 矿泉疗养学crenology90. 矿泉疗法crenotherapy91. 危机理论crisis theory92. 腋杖crutch93. 冷冻疗法cryotherapy94. 舞蹈疗法,dance therapy95. 导引daoyin96. 达松伐电疗法d'Arsonval electrotherapy, d'Arsonvalization97. 分米波疗法decimeter wave therapy98. 失神经支配denervation99. 缺损defect100. 间动电疗法diadynamic current therapy, Bernard therapy 101-200-------1. 膈式呼吸运动diaphragmatic breathing exercises2. 透热疗法diathermy3. 残疾是病损、失能、残障三者之总称。
加压训练对受试者肌肉形态和功能的影响:Meta分析
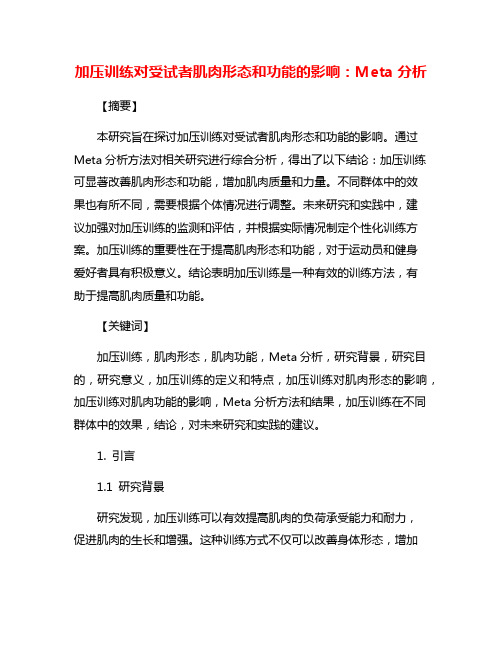
加压训练对受试者肌肉形态和功能的影响:Meta分析【摘要】本研究旨在探讨加压训练对受试者肌肉形态和功能的影响。
通过Meta分析方法对相关研究进行综合分析,得出了以下结论:加压训练可显著改善肌肉形态和功能,增加肌肉质量和力量。
不同群体中的效果也有所不同,需要根据个体情况进行调整。
未来研究和实践中,建议加强对加压训练的监测和评估,并根据实际情况制定个性化训练方案。
加压训练的重要性在于提高肌肉形态和功能,对于运动员和健身爱好者具有积极意义。
结论表明加压训练是一种有效的训练方法,有助于提高肌肉质量和功能。
【关键词】加压训练,肌肉形态,肌肉功能,Meta分析,研究背景,研究目的,研究意义,加压训练的定义和特点,加压训练对肌肉形态的影响,加压训练对肌肉功能的影响,Meta分析方法和结果,加压训练在不同群体中的效果,结论,对未来研究和实践的建议。
1. 引言1.1 研究背景研究发现,加压训练可以有效提高肌肉的负荷承受能力和耐力,促进肌肉的生长和增强。
这种训练方式不仅可以改善身体形态,增加肌肉量,还可以提高肌肉的爆发力和稳定性,从而提高运动表现和减少运动损伤的发生率。
虽然加压训练的效果被广泛认可,但是关于其对受试者肌肉形态和功能的影响,尤其是在不同群体中的表现,仍然存在一定的争议和待解答的问题。
有必要进行一项Meta分析研究,系统总结和分析现有的研究成果,探讨加压训练对受试者肌肉形态和功能的影响,为未来研究和实践提供科学依据和建议。
1.2 研究目的本研究的目的是通过Meta分析研究加压训练对受试者肌肉形态和功能的影响。
现有研究表明,加压训练可以有效提高肌肉力量和肌肉质量,并对肌肉形态和功能产生积极影响。
现有研究结果并不一致。
一些研究显示加压训练可以显著改善肌肉形态和功能,而另一些研究则得出相反结论。
本研究旨在通过Meta分析综合研究结果,进一步探讨加压训练对受试者肌肉形态和功能的影响规律,为加压训练的实践提供科学依据。
功能性动作筛查预测运动损伤的可行性一项前瞻性队列研究的meta分析
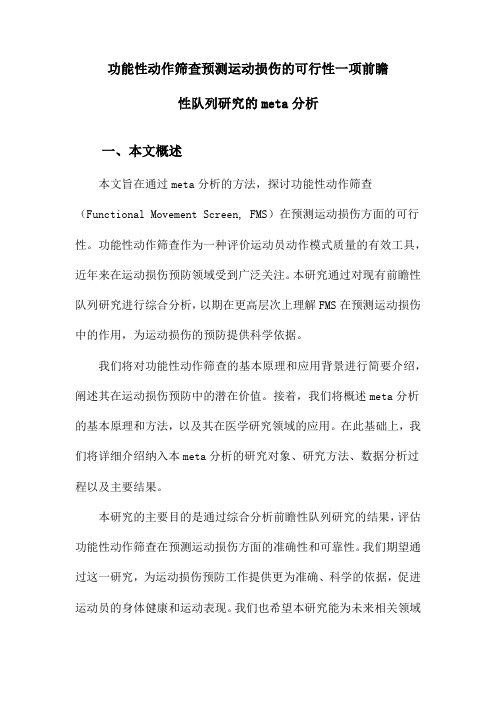
功能性动作筛查预测运动损伤的可行性一项前瞻性队列研究的meta分析一、本文概述本文旨在通过meta分析的方法,探讨功能性动作筛查(Functional Movement Screen, FMS)在预测运动损伤方面的可行性。
功能性动作筛查作为一种评价运动员动作模式质量的有效工具,近年来在运动损伤预防领域受到广泛关注。
本研究通过对现有前瞻性队列研究进行综合分析,以期在更高层次上理解FMS在预测运动损伤中的作用,为运动损伤的预防提供科学依据。
我们将对功能性动作筛查的基本原理和应用背景进行简要介绍,阐述其在运动损伤预防中的潜在价值。
接着,我们将概述meta分析的基本原理和方法,以及其在医学研究领域的应用。
在此基础上,我们将详细介绍纳入本meta分析的研究对象、研究方法、数据分析过程以及主要结果。
本研究的主要目的是通过综合分析前瞻性队列研究的结果,评估功能性动作筛查在预测运动损伤方面的准确性和可靠性。
我们期望通过这一研究,为运动损伤预防工作提供更为准确、科学的依据,促进运动员的身体健康和运动表现。
我们也希望本研究能为未来相关领域的研究提供有益的参考和启示。
二、文献综述功能性动作筛查(Functional Movement Screen, FMS)作为一种评估个体动作模式质量和对称性的工具,在运动损伤预防领域逐渐受到关注。
其理论基础在于,通过检测受试者完成一系列基础动作时的表现,可以预测其在未来运动过程中可能遭遇的损伤风险。
近年来,多项研究对FMS预测运动损伤的能力进行了探索,然而,这些研究的结果并不一致,有些支持FMS的有效性,有些则持怀疑态度。
因此,对这一问题进行系统性的综述和元分析显得尤为重要。
早期的研究主要集中在FMS与特定运动项目损伤风险的关系上。
例如,一些研究指出,在足球、篮球等高强度运动中,FMS得分较低的运动员更容易遭受下肢损伤。
这些研究通常通过对比受伤运动员与未受伤运动员的FMS得分,来验证FMS的预测价值。
功能性动作筛查预测运动损伤的可行性一项前瞻性队列研究的meta分析

功能性动作筛查预测运动损伤的可行性一项前瞻性队列研究的meta分析一、内容概要本研究旨在通过meta分析方法,评估功能性动作筛查(FEAS)在预测运动损伤方面的可行性。
FEAS是一种非侵入性的运动损伤风险评估工具,通过对运动员进行一系列的动作测试,以识别出可能导致运动损伤的关键动作模式。
本研究将对已发表的关于FEAS与运动损伤相关性的临床试验进行综合分析,以期为运动员预防运动损伤提供更为准确和可靠的依据。
本研究首先对文献进行了广泛的检索,筛选出与FEAS相关的临床试验,并对其进行质量评估。
然后采用统一的数据分析方法,对各篇文献中的数据进行整合和统计分析。
根据研究结果,对FEAS在预测运动损伤方面的准确性和可靠性进行综合评价。
本研究的结果将有助于提高运动员在预防运动损伤方面的表现,降低运动损伤的发生率,从而提高运动员的竞技水平和生活质量。
此外本研究还将为FEAS的进一步发展和完善提供理论依据和实践指导。
A. 研究背景和意义随着人们生活水平的提高和健康意识的增强,运动已经成为了许多人日常生活的重要组成部分。
然而长时间、高强度的运动训练可能导致运动损伤的发生,给运动员的身体健康带来严重影响。
因此预测运动损伤风险并采取相应的预防措施显得尤为重要,功能性动作筛查(FMS)作为一种评估运动员运动技能和损伤风险的方法,已经在许多体育项目中得到广泛应用。
然而目前关于FMS在预测运动损伤方面的研究仍存在一定的局限性,尤其是在长期跟踪研究方面。
本研究旨在通过meta分析方法对已发表的关于FMS预测运动损伤的前瞻性队列研究进行综合评价,以期为运动损伤的预防提供更为科学、准确的理论依据。
通过对这些研究的综合分析,我们可以更好地了解FMS在预测运动损伤方面的效果,以及可能的影响因素,从而为运动员的健康管理和训练计划制定提供有力支持。
此外本研究还有助于推动功能性动作筛查在运动损伤预防领域的进一步发展和应用。
B. 目的和方法文献回顾:我们在PubMed、Web of Science、中国知网等数据库中检索与功能性动作筛查预测运动损伤相关的研究文献,筛选出符合纳入标准的研究。
美国运动医学学会运动强度分级 中 英文对照版

强度 吸氧量储备 (%)
非常轻
<20
相对运动强度(动力性运动)
最大吸氧量 (%)
<25
心率储备 (%)
小于20
最大心率 (%)
<35
主观用力程 度
<10
轻
20-39
25-44
20-39
35-54
10~11
中等
40-59
45-59
40-59
55-69
12~13
重
60-84
50-69
7.2-10.1 6.0-8.4 4.8-6.7 3.0-4.24
70-84
≥10.2
≥8.5
≥6.8
≥4.25
≥85
12
10
8
5
100
,可经由体适能检测的心肺耐力测试获得
动会有助于促进个体健康
60-84
60-84
70-89
14~16
非常重
≥85
≥85
≥85
≥90
17~19
最大
100
100
100
100
20
1、表格的基础是针对持续60分钟的动力性运动 2、吸氧量储备=最大吸氧量-安静时的吸氧量 3、最大吸氧量:运动过程中人体各系统发挥最大机能时的氧气的吸收利用量,可经由体适能检测的心 4、心率储备=最大心率-安静时的心率(或晨脉) 5、最大心率=220-年龄 6、主观用力程度:采用的是Borg的6~20分级量表 7、MET:代谢当量,以每分钟氧的代谢状况进行表示,1MET=3.5ml/kg/min 8、美国运动医学学会建议每周至少5天,每天至少30分钟的中等强度有氧运动会有助于促进个体健康
ACE-CPT备考每章习题与答案第八章生理评估
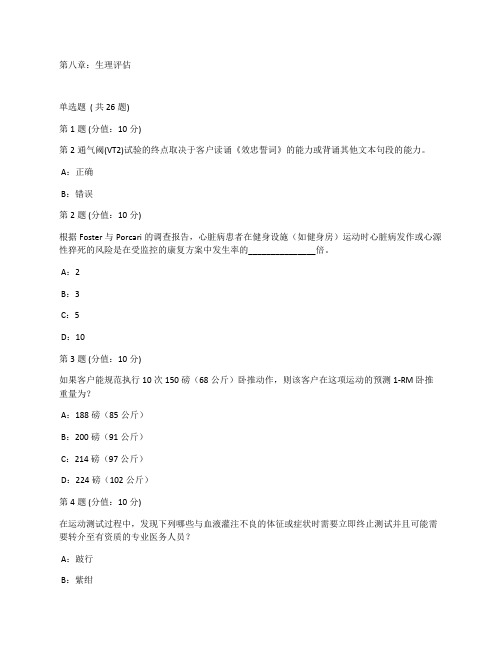
第八章:生理评估单选题 ( 共26题)第1题 (分值:10分)第2通气阈(VT2)试验的终点取决于客户读诵《效忠誓词》的能力或背诵其他文本句段的能力。
A:正确B:错误第2题 (分值:10分)根据Foster与Porcari的调查报告,心脏病患者在健身设施(如健身房)运动时心脏病发作或心源性猝死的风险是在受监控的康复方案中发生率的_______________倍。
A:2B:3C:5D:10第3题 (分值:10分)如果客户能规范执行10次150磅(68公斤)卧推动作,则该客户在这项运动的预测1-RM卧推重量为?A:188磅(85公斤)B:200磅(91公斤)C:214磅(97公斤)D:224磅(102公斤)第4题 (分值:10分)在运动测试过程中,发现下列哪些与血液灌注不良的体征或症状时需要立即终止测试并且可能需要转介至有资质的专业医务人员?A:跛行B:紫绀D:共济失调第5题 (分值:10分)测量最大摄氧量(VO2max)需要在实验室进行极量运动试验时收集并分析______________。
A:血氧水平B:呼出的气体C:核心温度D:消耗的热量第6题 (分值:10分)身材短小的个体可能不太适合进行哪种测试?A:踏车运动试验B:平板运动试验C:通气阈测试D:台阶测试第7题 (分值:10分)下列哪种身体成分评估法估算的体脂百分比会随受试者身体水合状况而每天都发生巨大变化? A:皮褶厚度测量法B:生物电抗阻分析法(BIA)C:水下皮脂测定法D:双能X线吸收测定法(DEXA)第8题 (分值:10分)当客户准备进行1-RM肌力测试时,在第一次热身组测试中,私人教练应鼓励客户采用的重量为1-RM百分之多少?A:25%B:50%D:90%第9题 (分值:10分)女性客户达到多大的腰臀比阈值时可视为存在着健康风险?A:0.79B:0.82C:0.86D:0.95第10题 (分值:10分)一名54岁的男性客户,虽无运动禁忌,但该客户超过10年未曾积极规律运动,请问进行哪一项评估最恰当?A:1.5英里快跑测试B:Rockport 健步行测试C:VT2测试D:1-RM倒蹬试验第11题 (分值:10分)_______________ 将体重与身高的关系描述为客观的比值。
BIODEX等速持续被动模式下向心离心肌力训练治疗脑卒中后足内翻的效果
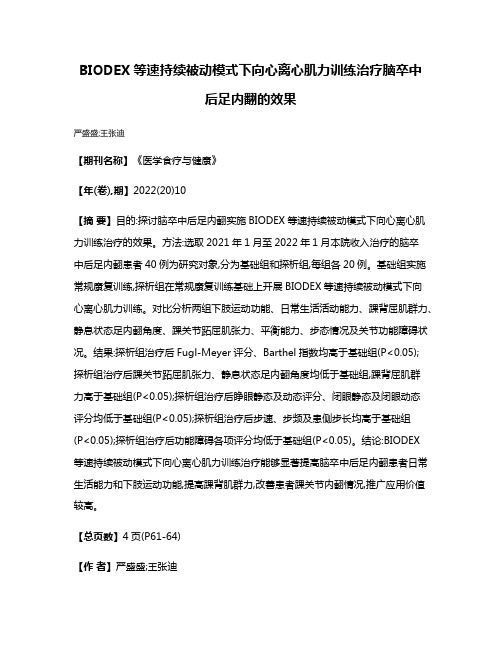
BIODEX等速持续被动模式下向心离心肌力训练治疗脑卒中后足内翻的效果严盛盛;王张迪【期刊名称】《医学食疗与健康》【年(卷),期】2022(20)10【摘要】目的:探讨脑卒中后足内翻实施BIODEX等速持续被动模式下向心离心肌力训练治疗的效果。
方法:选取2021年1月至2022年1月本院收入治疗的脑卒中后足内翻患者40例为研究对象,分为基础组和探析组,每组各20例。
基础组实施常规康复训练,探析组在常规康复训练基础上开展BIODEX等速持续被动模式下向心离心肌力训练。
对比分析两组下肢运动功能、日常生活活动能力、踝背屈肌群力、静息状态足内翻角度、踝关节跖屈肌张力、平衡能力、步态情况及关节功能障碍状况。
结果:探析组治疗后Fugl-Meyer评分、Barthel指数均高于基础组(P<0.05);探析组治疗后踝关节跖屈肌张力、静息状态足内翻角度均低于基础组,踝背屈肌群力高于基础组(P<0.05);探析组治疗后睁眼静态及动态评分、闭眼静态及闭眼动态评分均低于基础组(P<0.05);探析组治疗后步速、步频及患侧步长均高于基础组(P<0.05);探析组治疗后功能障碍各项评分均低于基础组(P<0.05)。
结论:BIODEX等速持续被动模式下向心离心肌力训练治疗能够显著提高脑卒中后足内翻患者日常生活能力和下肢运动功能,提高踝背肌群力,改善患者踝关节内翻情况,推广应用价值较高。
【总页数】4页(P61-64)【作者】严盛盛;王张迪【作者单位】余姚市人民医院康复医学科【正文语种】中文【中图分类】R743.3【相关文献】1.等速向心和离心肌力训练治疗膝关节骨性关节炎患者的有效性研究2.BIODEX多关节等速力量测试和训练系统在脑损伤后偏瘫足内翻中的治疗效果3.热敏灸联合等速向心肌力训练治疗膝关节骨性关节炎4.BIODEX等速持续被动模式下向心离心肌力训练治疗脑卒中后足内翻临床效果研究5.等速闭链训练与等速开链训练对脑卒中后运动障碍患者下肢肌力、平衡功能与步行能力影响研究因版权原因,仅展示原文概要,查看原文内容请购买。
- 1、下载文档前请自行甄别文档内容的完整性,平台不提供额外的编辑、内容补充、找答案等附加服务。
- 2、"仅部分预览"的文档,不可在线预览部分如存在完整性等问题,可反馈申请退款(可完整预览的文档不适用该条件!)。
- 3、如文档侵犯您的权益,请联系客服反馈,我们会尽快为您处理(人工客服工作时间:9:00-18:30)。
1 Adaptive Action Selection of BodyExpansion Behavior in Multi-Robot System using CommunicationTomohisa Fujiki a,1,Kuniaki Kawabata b and Hajime Asama ca School of Engineering,The University of Tokyob RIKENc RACE,The University of TokyoAbstract.In multi-robot system,cooperation is needed to execute tasks efficiently.The purpose of this study is to realize cooperation among multiple robots using in-teractive communication.An important role of communication in multi-robot sys-tem is to make it possible to control other robots by intention transmission.Weconsider that multi-robot system can be more and more adaptive by treating com-munication as action.In this report,we adopt action adjustment function to achievecooperation between robots.We also run some computer simulations of collisionavoidance as an example of cooperative task,and discuss the results.Keywords.Q-learning,Multi-Robot System,Communication,Cooperation,MobileRobot1.INTRODUCTIONIn multi-robot systems,communication is thought as a necessary skill for robotsto co-operate,and a number of schemes have been proposed for it[1,2].However,these stud-ies may not be useful to adapt in a dynamic and complex environment as they set rules to communicate.To achieve cooperation effectively in such environments,we have to discuss the adaptable cooperation using communication.Yanco et al.tried to develop a method to acquire an adaptive communication for cooperation of two robots[3].Billard et al.proposed a learning method of communication through imitation[4].This is an interesting approach but the system needs a teacher robot.In these methods and most of robotics resesarch,the communication is treated as special function for the robotic systems.On the other hand,in developmental psychology,communication is considered as interaction between individuals[5].Moreover,communication is the transmission of in-tention,and those who received have to comprehend the intention.In conventional stud-ies on cooperation of robots based on communication as signal transmission,action is taken as a motion of its own body and they focused on decision making using sensory 1Correspondence to:Tomohisa Fujiki,5-1-5,Kashiwanoha,Kashiwa-shi,Chiba,277-8568,JAPAN.Tel.: +81-47-136-4260;Fax:+81-47-136-4242;E-mail:fujiki@race.u-tokyo.ac.jp.2T.Fujiki et al./Adaptive Action Selection of Body Expansion Behavior munication is signal transmission over wireless LAN or other devices, but it is not correct in developmental psychological sense.There should be a sort of protocol between robots to communicate,and the intention should be exchanged.Consequently transmitting one’s intention could be treated as an action and receiving other’s intention could be treated as perception in multi-robot system.By introducing this concept to their control architecture,robots can make an attempt to control other robots. This means that a robot can make an action over constraint of its own D.O.F.(body-expansion behavior),and multi-robot system can be moreflexible and adaptable.In this study,we take in communication to robot’s model both as perception and action.It means to achieve cooperation between robots,not only robot’s own movement but also sending message to other robots is treated as an action.We have previously developed an action selection method[6]which treats communication as above,but there was a problem of how to adjust different type of actions;self generated action and a requested one by communication.It seems that most effective strategy for the whole system is to accept a request only when the situations for both robots seem to improve.In this paper,we propose an action adjustment function to achieve cooperation be-tween mobile robots.We also have some computer simulations of collision avoidance as an example of cooperative task,and discuss the results.2.ACTION SELECTION METHOD INCLUDING INTERACTIVECOMMUNICATION2.1.Reinforcement LearningReinforcement Learning(RL,[7]is widely used in robotic systems to emerge robots’ac-tions from the interaction between the environment.However,in multi-robot system, there is a possibility that the same action causes different state transition which mis-leads the learning.To avoid this problem,Q-Learning for Semi Markov Decision Pro-cess(SMDP,[8])which can handle discrete time series is utilized generally.Q-Learning algorithm for SMDP is as follows.1.Observe state s t at time t in the environment.2.Execute action a t selected by action selection node.3.Receive reward r and calculate the sum of discounted reward R sum until its statechanges.R sum=r t+γr t+1+γ2r t+2+···+γN−1r t+N−1(1) Here,r is a discount factor(0≤r≤1).4.Observe state s t+N at time t+N after the state change.5.Renew Q value by equation(2).Q(s t,a t)←(1−α)Q(s t,a t)+α[R sum+γN maxQ(s t+N,a )](2)aHere,αis a learning rate(0≤α≤1)and a is possible actions in state s t+N.6.Clear r.7.Renew time step t to t+N,and return to1.T.Fujiki et al./Adaptive Action Selection of Body Expansion Behavior3 2.2.Basic ActionsThere are a variety of tasks considered as cooperative tasks,but in this paper,we are going to discuss collision avoidance problem of mobile robots.This is because that al-though there are a lot of the rule based schemes proposed for it,it can still see the effect of the communication and the expansion in D.O.F.directly.We suppose omni-directional mobile robots which are equipped with omni-directional visual sensors.Considering communication as robots’action,basic actions for robots are set as Table1.Here,“Comminication”means intention transmission, which is a requesting action to other robot to make an asked action.This means that a robot can request any actions which the other robot can make.Robots acquire their state-action policy by RL.We also configure robot’s state space as Table2.Numbers in Table2shows the number of state space for each domains.Example for the visual sensory information is shown in Figure1.The size of the other robot on image plane is determined by the distance threshold.Both the direction of the other robot and the goal are devided into six state spaces.Direction of the wall hasfive state spaces,which are front,left,right,and the back of the robot,or,no walls.In Figure1,the white wall is placed above the distace threshold,so only the grey wall is considered as an obstacle in robot’s state space.In this framework,a robot selects,evaluates and learns its action from sensory information and other robots’intentions.Table1.Actions of robotMove Own Body Communication-No changes in speed or direction-No changes in speed or direction-Speed down(2[mm/sec])-Speed down(2[mm/sec])-Speed up(2[mm/sec])-Speed up(2[mm/sec])-Change direction(+45[deg])-Change direction(+45[deg])-Change direction(-45[deg])-Change direction(-45[deg])2.3.Action Selection and RewardThere are a lot of action selection models for Q-Learning like Max Selection or Random Selection.One of the methods to improve its adaptability gradually by RL is probabilisticTable2.Configuration of state spaceVisual sensory information-Size of other robot on image plane2-Direction of other robot6-Direction of the goal6-Wall direction inside the sensing area4+1(none)Communication-Other robot’s request5+1(none)Other Information-Own Speed2Number of the State Space43204T.Fujiki et al./Adaptive Action Selection of Body ExpansionBehaviorroobt on the image planedirection of other robots direction of the goal(distance threshold 600[mm])Figure1.Visual Sensory Informationaction selection using Boltzmann distribution(Boltzmann selection).It is used widely and is reported that probabilistic selection works better than deterministic policy in multi-agent systems[9].In Boltzmann Selection model,probability p(a|s)to make action a in state s is defined as equation(3).p(a|s)=exp Q(s,a)/Ta i∈Aexp Q(s,a i)/T(3)Here,T is temperature constant.If T is near zero,action selection will be deterministic, and if T becomes large,action selection will be more random and will do aggressive search for state-action policy.Evaluation of the selected action is done by using the distance from the goal g(t)in time t.Reward r t is defined by equation(4).r t=µ(g(t)−g(t−∆t))(4)Here,µis a weight value and represents effectiveness of the reward.∆t is cycle time for decision making.3.ACTION ADJUSTMENT FUNCTIONWhen communication is treated as an action for intention transmission,accepting all the requested actions will only to improve other robots’situations.However,for the whole system,it is seems that most effective way is to accept the request only when the situations of both robots can be improved.To accept such requests,there is a need for action adjustment function to compare the actions which are self determined action and a requested one by communication.It makes the robots to create better situations,and will be able to cooperate efficiently.For this action adjustment,we introduce the algorithm which is illustrated in Figure 2.First,a robot decides whether to move itself or to make other robot move by commu-nication.This is a selfish action selection which doesn’t consider the state of other robot.T.Fujiki et al./Adaptive Action Selection of Body Expansion Behavior5 Of course there is a probability that the request will be refused,but whether to accept or reject the request is determined by the receiver.Next,a robot will determine which action to make;the selfish action that is decided atfirst step or a requested action by other robot.By those two steps,a robot can select an action considering a request from other robot.This adjustment algorithm can be utilized generally by giving numeric values for each actions.In this paper,we use Q-Learning algorithm for SMDP and the Q values from the RL are used as numeric values for two step action selection.The implemented algorithm for the robot is shown in Figure3.Figure2.Action selection processFigure3.Algorithm for action selection including communicationPUTER SIMULATION OF COLLISION A VOIDANCE PROBLEMIn this section,we are going to have some computer simulations to test our approach and discuss the results.6T.Fujiki et al./Adaptive Action Selection of Body Expansion Behavior4.1.SettingsThere are two omni-directional mobile robots in simulationfield,and the task is colli-sion avoidance as an example of cooperative task.To compare our approach to general approach of communication in robotic researchfield,we set three conditions.Case A is for our proposed approach and robots can use communication to move the other robot by intention transmission.In case B,robots can inform their adjacent action which they made by signal transmission.By this,we can eliminate an influence on the size of state space and robots in case B have same state number as in case A.Robots in case C cannot use communication.Common settings are as follows.Robot is cylinder shaped and its diameter is 300[mm].Start position of each robot is set500[mm]from longitudinal sides of the environment,symmetry in ups and downs.Goal position is the starting point of the other robot,and is set face to face in initial condition.Maximum speed of the robots is 40[mm/sec],and minimum is10[mm/sec].It assumes that robots can output their speed without a time lag.A trial is terminated under four conditions,which are the goal of the both robots, the collision of robots,the collision of either robot against walls or simulation area,or when the time step reached to3000.The parameters for RL are set experimentally as ∆t=1.0[sec],µ=0.1,α=0.04,γ=0.9and T=0.2.Reward for the robots are calculated by equation(4),but in case of any collisions,r=−5is given as punishment value.4.1.1.Simulation1In simulation1,straightway environment in Figure4is utilized.Width800≤x≤3000[mm]is changed by100[mm]and computer simulation is run for four times in every situation,and the learning is episodic for each simulation.Maximum trial number is30000for every experiment.4.1.2.Simulation2In simulation2,crossroad environment in Figure4is utilized.Simulation area is 3000[mm]square,and the width of both roads are x[mm],which changes600≤x≤3000[mm]by200[mm].Four black pieces in Figure4are walls(obstacles).Computer simulation is run for four times in every situation,and Maximum trial number is100000 for every experiment.Learning is episodic for each simulation.All settings has the same distance for goal,to make it easy to compare the results. In simulation2,when a robot moves,physical relationship against the walls change,and it affects robot’s state space.Consequently,robots’state change frequently when x is small,and the problem will be much difficult compared to the same x in Simulation1.4.2.ResultsFigure5shows the number of trials for convergence.In this report,“convergence”means 100continuous goals.Horizontal axis shows the width of the road x.Data on those graphs are the average of four trials.It shows some oscillation,but the aptitude can been comprehended.T.Fujiki et al./Adaptive Action Selection of Body Expansion Behavior7StraightwayCrossroadFigure 4.Over view ofenvironment100020003000400050006000700080009000300280260240220200180160140120100800xN u m b e r o f T r i a l s f o C o n v e r g e n c eSimulation103000280026002400220020001800160014001200100080060x N u m b e r o f T r i a l s f o C o n v e r g e n c eSimulation 2Figure 5.Number of trials for convergence4.2.1.Convergence PropertiesWhen the width x is large enough for robots and the problem can be solved easily,Case C achieves convergence faster than other cases.We believe that this occurs because the state space of Case C is one fifth of other cases and therefore it is easy to acquire the state-action policy.The result of Case B shows large oscillation in both graphs.In this case,communication changes the state of the other robot,and it makes difficult to search state-action munication as signal transmission doesn’t show its superiority in any case of our experiments.It only multiplies the number of states and prevents system from fast achieving of cooperation.Finally,Case A has superiority to other methods when x is small.This is the condition which the problem is hard to solve and is difficult to cooperate with others.Results show that our approach can solve the problem cooperatively even when the other approaches cannot solve it.It is a difficult situation for robots to cooperate without communication,and comparing Case A to Case B,our proposed system works better than usual usage of the communication such as information transmission.4.2.2.Quality of the SolutionFigure 6shows the number of steps to converge,which shows the quality of the solution achieved by the system.Data on those graphs are the average of four trials.Although there are many spikes,Case A apts to generate better solutions than the other methods.We consider that intention transmission worked effectively by affectiong the other robots,only when the communication is needed.This result supports our approach that it is not only in the fastness in finding solutions but also in the quality of the solution.8T.Fujiki et al./Adaptive Action Selection of Body ExpansionBehavior2040608010012014016018030002800260024002200200018001600140012001000xN u m b e r o f S t e p sSimulation12040608010012014016018020030002800260024002200200018001600140012001000800600xN u m b e r o f S t e p sSimulation 2Figure 6.Number of steps5.CONCLUSIONSIn this paper,we proposed a method to adjust different type of actions which include communication as intention transmission.By using this method,we enabled to treat com-munication as intention transmission action in multi-robot system and also examined its performance by computer simulations.The results show that our approach can find solu-tion in difficult situation where cooperation is hardly achieved without communication,and is also excels in the quality of solution achieved by the system than ordinal way of communication or without using communication.In our future work,we will try our approach in more complex environments or other tasks.References[1]Y .Arai,S.Suzuki,S.Kotosaka,H.Asama,H.Kaetsu and I.Endo:Collision Avoidanceamong Multiple Autonomous Mobile Robots using LOCISS (LOcally Communicable In-frared Sensory System),Proceedings of the IEEE International Conference on Robotics and Automation ,pp.2091–2096,1996.[2]N.Hutin,C.Pegard,E.Brassart,A Communication Strategy for Cooperative Robots,Proc.of IEEE/RSJ Intl.Conference on Inteligent Robots and Systems ,pp.114–119,1998.[3]H.Yanco,L.A.Stein,An Adaptive Communication Protocol for Cooperating Mobile Robots,From Animals to Animats 2,pp.478–485,1993.[4]A.Billard,G.Hayes,Learning to Communicate Through Imitation in Autonomous Robots,Artificial Neural Networks -ICANN’97,pp.763–768,1997.[5]Walburga V on Raffler-Engel (Editor):Aspects of Nonverbal Communication,Loyola Pr,1979.[6]M.Hoshino,H.Asama,K.Kawabata,Y .Kunii and I.Endo:Communication Learning forCooperation among Autonomous Robots,Proceedings of the IEEE International Conference on Industrial Electronics,Control &Instrumentation ,pp.2111–2116,2000.[7]Richard S.Sutton and Andrew G.Barto:Reinforcement Learning:An Introduction (AdaptiveComputation and Machine Learning),The MIT Press,1998.[8]Steven J.Bradtke and Michael O.Duff:Reinforcement Learning Methods for Continuous-Time Markov Decision Problems,In G.Tesauro and D.Touretzky and T.Leen,editors,Ad-vances in Neural Information Processing Systems ,V ol.7,pp.393–400,The MIT Press,1995.[9]Satinder P.Singh and Tommi Jaakkola and Michael I.Jordan:Learning Without State-Estimation in Partially Observable Markovian Decision Processes,International Conference on Machine Learning ,pp.284–292,1994.。