SDAM A New data Aggregation Approach for Wireless Sensor Networks
stata中nmissing命令的用法 -回复

stata中nmissing命令的用法-回复Stata中的nmissing命令用于计算变量中缺失值的数量。
缺失值是缺少观测值的数据点,它们可能是由于数据采集过程中的错误、非回答、遗漏或其他原因导致的。
缺失值的存在可能会影响数据的准确性和统计分析的可靠性。
nmissing命令可以帮助我们快速确定变量中的缺失值数量,并以此来衡量数据的完整性。
下面的步骤将详细介绍如何在Stata中使用nmissing命令,以及该命令的一些选项和应用示例。
步骤1:加载数据集首先,我们需要在Stata中加载包含要分析的变量的数据集。
可以使用以下命令加载数据集:use "数据集文件路径\数据集文件名.dta"请注意,"数据集文件路径\数据集文件名.dta"是你的数据集存储的路径和文件名。
步骤2:检查变量名和缺失值数量在使用nmissing命令之前,我们需要确定要分析的变量名称。
可以使用以下命令列出数据集中所有的变量名称:describe这将显示数据集中所有变量的相关信息,包括名称、数据类型和存储格式。
找到你感兴趣的变量名称,以便对其进行缺失值计数。
步骤3:使用nmissing命令一旦我们确定了要分析的变量名称,就可以使用nmissing命令来计算其缺失值数量。
以下是nmissing命令的基本语法:nmissing 变量名称例如,如果要计算名为"age"的变量中的缺失值数量,可以使用以下命令:nmissing age执行此命令后,Stata将显示变量"age" 中的缺失值的数量。
步骤4:nmissing命令的选项nmissing命令还提供了一些选项,可以根据需要对缺失值进行分组和计数。
以下是nmissing命令的常用选项:- by(varlist): 可以使用by选项将数据按照一个或多个变量进行分组,并计算各组中缺失值的数量。
例如,使用by选项可以计算来自不同城市或性别的年龄变量中的缺失值数量。
les AS gR学生手册英文

Deploying with Oracle JDeveloper
To deploy an application with JDeveloper, perform the following steps: 1. Create the deployment profile. 2. Configure the deployment profile. 3. Create an application server connection to the target
第十六页,共33页,
Planning the Deployment Process
The deployment process includes:
1. Packaging code as J2EE applications or modules
2. Selecting a parent application
including those in a cluster
第十页,共33页,
Deploying with admin_client.jar
The admin_client.jar tool: • Is a command-line utility • Is executed by using the following basic command:
– defaultDataSource to select the data source used by the application for management of data by EJB entities
– dataSourcesPath to specify a application-specific data sources
Oracle Database Administration 2019 认证说明书
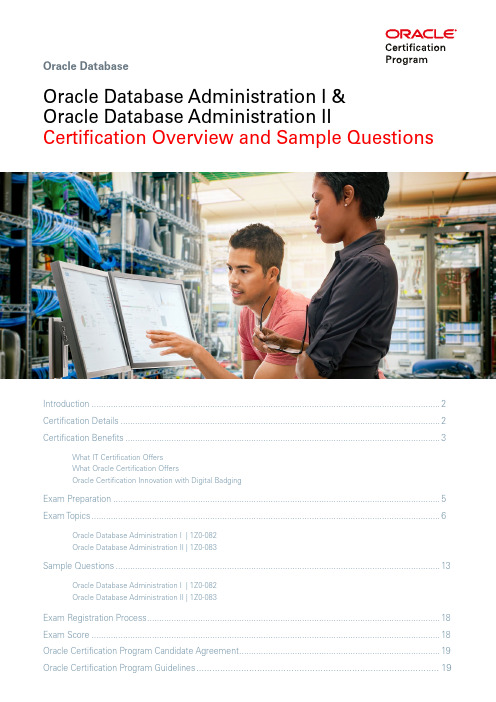
Introduction ................................................................................................................................................2Certification Details ....................................................................................................................................2Certification Benefits . (3)What IT Certification Offers What Oracle Certification OffersOracle Certification Innovation with Digital BadgingExam Preparation .......................................................................................................................................5Exam Topics (6)Oracle Database Administration I | 1Z0-082Oracle Database Administration II | 1Z0-083Sample Questions (13)Oracle Database Administration I | 1Z0-082Oracle Database Administration II | 1Z0-083Exam Registration Process .........................................................................................................................18Exam Score ................................................................................................................................................18Oracle Certification Program Candidate Agreement ...................................................................................19Oracle Certification Program Guidelines .. (19)Oracle Database Administration I & Oracle Database Administration IICertification Overview and Sample QuestionsOracle DatabaseIntroductionPreparing to earn the Oracle Database Administration 2019 Certified Professional certification helps candidates gain the skills and knowledge to install, patch and upgrade Oracle Database and Oracle Grid Infrastructure for a standalone server, create and manage a backup and recovery strategy using Recovery Manager (RMAN), use RMAN for Database duplication and transportation, diagnose failures using RMAN, and manage all aspects of Multitenant container databases, pluggable databases and application containers including creation, cloning, security, transportation and backup and recovery. The Administration I exam and recommended training focus on fundamental Database Administration topics such as understanding the database architecture, managing database instances, managing users, roles and privileges, and managing storage that lay the foundation for a Database Administrator entry-level job role. Additionally, the Admin I exam assumes knowledge of SQL.The Administration II exam and associated recommended training presents advanced topics such as multi-tenancy, backup and recovery, deploying, patching, and upgrading.Certification BenefitsWhat Oracle Certification OffersBy becoming a certified Oracle Database Administrator Professional , you demonstrate the full skill set needed to perform day to day administration of the Oracle Database.Preparing to take the Oracle Database certification exam broadens your knowledge and skills by exposing you to a wide array of important database features, functions and tasks. Oracle Database certification preparation teaches you how to perform complex, hands-on activities through labs, study and practice.Additionally, Oracle certification exams validate your capabilities using real-world, scenario-based questions that assess and challenge your ability to think and perform.What IT Certification OffersRecognitionof having required skillsExperienced a Greater Demandfor Their SkillsReceived Positive Impact onProfessional Imagethrough new skillsOpportunitiesSaid Certification was a Key Factor in Recent Raiseby peers and managementConfidence and RespectJanuary 2018 issue of Certification Magazine’s annual salary survey The kind of longevity suggests that earning and maintaining a certification can keep you moving for-ward in your career, perhaps indefinitely.73%65%71%January 2019 issue of Certification Magazine’s annual salary survey January 2019 issue of Certification Magazine’s annual salary surveyCertification that Signifies Y our Readiness to Perform Earned badges represent recognized skills and capabilitiesDisplay Y our Oracle Certification BadgeY ou‘ve earned it. Get the recognition you deserve.Modern Representation of Skills Tied to Real Time Job Markets View from your profile and apply to jobs that are matched to your skills; based on location, title, employers or salary rangeDisplay Y our AchievementA secure way to display and share your certification achievement • Industry Recognized • Credible • Role Based• Product Focused Across Database, Applications, Cloud, Systems, Middleware and Java• Globally one of the top 10 certifica-tion programs availableOracle Certification Innovation with Digital Badging Use Your Badge to Apply for JobsBenefitsOracle Certification Signifies a Candidate’s Readiness to Perform2019 Oracle Certified Professional Oracle Database AdministratorBoost Y our Professional ImageLearn MoreExam PreparationBy passing these exams, a certified individual proves fluency in and solid understanding of the skills required to be an Oracle Database Administrator.Recommendations to successfully prepare for Oracle Database Administration I | 1Z0-082 and Oracle Database Administration II | 1Z0-083 exams are:Attend Recommended Oracle T rainingThe courses below are currently available and are terrific tools to help you prepare not only for your exams, but also for your job as an Oracle Database Administrator.The new Oracle Database Administration Learning Subscription also helps you prepare for these exams with 24/7 access to continually updated training and hands-on labs and integrated certification.Recommended for 1Z0-082• O racle Database: Administration Workshop • Oracle Database: Introduction to SQL Recommended for 1Z0-083• O racle Database: Deploy, Patch and Upgrade Workshop• O racle Database: Backup and Recovery Workshop • O racle Database: Managing Multitenant Architecture• Oracle Database Administration: Workshop • O racle Database 19c: New Features for Administrators• O racle Database 18c: New Features forAdministrators (for 10g and 11g OCAs and OCPs)• O racle Database 12c R2: New Features for 12c R1 Administrators (12c R1 OCAs and OCPs)• O racle Database 11g: New Features for Administrators (for 10g OCAs and OCPs)The following topics are covered in the Oracle Database: Administration Workshop course.The following topics are covered in theOracle Database: Introduction to SQL course.The following topics are covered in the Oracle Database: Managing Multitenant Architecture Ed 1 course.The following topics are covered in the Oracle Database: Backup and Recovery Workshop course.The following topics are covered in the Oracle Database: Deploy, Patch and Upgrade Workshop course.The following topics are covered in the Oracle Database 19c: New Features for Administrators course.The following topics are covered in the Oracle Database: Administration Workshop course1. Which two statements are true about the Oracle Database server architecture?A. An Oracle Database server process represents the state of a user’s login to an instance.B. An Oracle Database server process is always associated with a session.C. Each server process has its own User Global Area (UGA).D. A connection represents the state of a user’s login to an instance.E. The entire data dictionary is always cached in the large pool.2. W hich two statements are true about the Oracle Database server during and immediatelyafter SHUTDOWN IMMEDIATE?A. New connection requests made to the database instance are refused.B. Uncommitted transactions are rolled back automatically.C. All existing connections to the database instance remain connected until all transactions eitherroll back or commit.D. Uncommitted transactions are allowed to continue to the next COMMIT.E. All existing transactions are aborted immediately.3. Which three statements are true about Oracle database block space management?A. A row can be migrated to a block in a different extent than the extent containing the originalblock.B. An insert statement can result in a migrated row.C. An update statement cannot cause chained rows to occur.D. A row can be migrated to a block in the same extent as the extent containing the originalblock.E. An insert statement can result in a chained row.1 2. 3.4. A n Oracle Database server session has an uncommitted transaction in progress whichupdated 5000 rows in one table.In which two situations does the transaction complete, thereby committing the updates?A. When a DDL statement is executed successfully by same user in a different session.B. When a DDL statement is executed successfully by the user in the same session.C. When a DML statement is executed successfully by same user in a different session.D. When a DML statement is executed successfully by the user in the same session.E. When a DBA issues a successful SHUTDOWN NORMAL statement and the sessionterminates normally.5.Which two statements are true about indexes and their administration in an Oracle database?A. An index can be scanned to satisfy a query without the indexed table being accessed.B. A non-unique index can be converted to a unique index using a Data Definition Language(DDL) command.C. A descending index is a type of bitmapped index.D. An invisible index is maintained when a Data Manipulation Language (DML) command is per-formed on its underlying table.E. An index is always created by scanning the key columns from the underlying table.6. Which two statements are true about sequences in a single instance Oracle database?A. Sequences that start with 1 and increment by 1 can never have gaps.B. A sequence can issue the same number more than once.C. Sequence numbers that are allocated require a COMMIT statement to make the allocationpermanent.D. A sequence can provide numeric values for more than one column or table.E. The data dictionary is always updated each time a sequence number is allocated.4. 5. 6.7. E xamine the description of the SALES table:Name Null? Type---------------------------- -------- --------------PRODUCT_ID NOT NULL NUMBER(10)CUSTOMER_ID NOT NULL NUMBER(10)TIME_ID NOT NULL DATECHANNEL_ID NOT NULL NUMBER(5)PROMO_ID NOT NULL NUMBER(5)QUANTITY_SOLD NOT NULL NUMBER(10,2)PRICE NUMBER(10,2)AMOUNT_SOLD NOT NULL NUMBER(10,2)The SALES table has 55,000 rows.Examine this statement:CREATE TABLE mysales (prod_id, cust_id, quantity_sold, price)ASSELECT product_id, customer_id, quantity_sold, priceFROM salesWHERE 1 = 2;Which two statements are true?A. MYSALES is created with no rows.B. MYSALES will have no constraints defined regardless of which constraints might be de-fined on SALES.C. MYSALES has NOT NULL constraints on any selected columns which had that constraintin the SALES table.D. MYSALES is created with 2 rows.E. MYSALES is created with 1 row.71. Which three are true about an application container?A. It always contains multiple applications.B. Two or more application PDBs in the same application container can share access to tables.C. It can have new application PDBs created by copying PDB$SEED.D. T wo or more application PDBs in the same application container can be given exclusive accessto some tables.E. It always has a new application PDBs created by copying PDB$SEED.F. It always contains a single application.2. RMAN has just been connected to a target database and the recovery catalog database.In which two cases would an automatic partial resynchronization occur between this target database’s control file and the RMAN recovery catalog?A. When any control file metadata for data file backups or image copies is now older thanCONTROL_FILE_RECORD_KEEP_TIME.B. When a new data file is added to a tablespace in a registered target database.C. When a backup of the current SPFILE is created.D. When the target is first registered.E. When any control file metadata for archive log backups or image copies is now older thanCONTROL_FILE_RECORD_KEEP_TIME.3. Which two are true about Oracle Grid Infrastructure for a Standalone Server?A. Oracle Restart can be used without using ASM for databases.B. Oracle Restart can attempt to restart a failed ASM instance automatically.C. It must be installed before Oracle Database software is installed.D. It must be installed after Oracle Database software is installed.E. It allows ASM binaries to be installed without installing Oracle Restart.F. It allows Oracle Restart binaries to be installed without installing ASM.1 2. 3.4. W hich two are true about creating container databases (CDBs) and pluggable databases (PDBs) inOracle 19c and later releases?A. A CDB can be duplicated using the Database Configuration Assistant (DBCA) in silent mode.B. A CDB can be duplicated using Recovery Manager (RMAN) with no configuration requiredbefore starting the duplication.C. A PDB snapshot must be a full copy of a source PDB.D. A PDB snapshot can be a sparse copy of a source PDB.E. A CDB can be duplicated only by using the Database Configuration Assistant (DBCA).5. Which two are true about the Oracle Optimizer?A. It requires system statistics when generating SQL execution plans.B. It always generates an index access operation when a statement filters on an indexed columnwith an equality operator.C. It ignores stale object statistics in the Data Dictionary.D. It can automatically re-optimize execution plans that were detected to be sub-optimal whenexecuting.E. It can re-write a statement internally in order to generate a more optimal plan.4. 5.Exam Registration ProcessOracle exams are delivered through the independent company Pearson VUE. Create a Pearson VUE loginOracle Certification Program Candidate AgreementIn order to take your Oracle certification, you will need to agree to the Oracle Certification Program Candidate Agreement. Please review this document by going here.Oracle Certification Program GuidelinesLearn more about Oracle Certification policies here.This certification overview and sample questions were created in June 2019. The content is subject to change,please always check the web site for the most recent information regarding certifications and related exams: /certification。
数据管理参考手册说明书

Contents Intro..................................Introduction to data management reference manualData management............................Introduction to data management commands append............................................................Append datasets assert..........................................................Verify truth of claim assertnested...................................................Verify variables nestedbcal...............................................Business calendarfile manipulation by........................................Repeat Stata command on subsets of the data cd................................................................Change directory pare two datasets changeeol.....................................Convert end-of-line characters of textfile checksum..................................................Calculate checksum offile clear................................................................Clear memory clonevar......................................................Clone existing variable codebook.....................................................Describe data contents collapse............................................Make dataset of summary statistics pare two variables press data in memory contract....................................Make dataset of frequencies and percentages copy....................................................Copyfile from disk or URL corr2data...............................Create dataset with specified correlation structure count.................................Count observations satisfying specified conditions cross...................................Form every pairwise combination of two datasets Data types.............................................Quick reference for data types datasignature.....................................Determine whether data have changed Datetime............................................Date and time values and variables Datetime business calendars.........................................Business calendars Datetime business calendars creation...........................Business calendars creation Datetime conversion....................................Converting strings to Stata dates Datetime display formats.............................Display formats for dates and times Datetime durations................................Obtaining and working with durations Datetime relative dates................Obtaining dates and date information from other dates Datetime values from other software...........Date and time conversion from other software describe..........................................Describe data in memory or in afile destring.......................Convert string variables to numeric variables and vice versa dir...............................................................Displayfilenames drawnorm..............................Draw sample from multivariate normal distribution drop..................................................Drop variables or observations pactly list variables with specified properties duplicates....................................Report,tag,or drop duplicate observations dyngen....................................Dynamically generate new values of variables edit..............................................Browse or edit data with Data Editor egen.........................................................Extensions to generate encode.......................................Encode string into numeric and vice versaiii Contents erase..............................................................Erase a diskfile expand.......................................................Duplicate observations expandcl..............................................Duplicate clustered observations export...........................................Overview of exporting data from Stata filefilter.......................................Convert ASCII or binary patterns in afile fillin.........................................................Rectangularize dataset format....................................................Set variables’output format fralias..............................................Alias variables from linked frames frames intro...................................................Introduction to frames frames................................................................Data frames frame change.................................Change identity of current(working)frame frame copy..................................................Make a copy of a frame frame create.....................................................Create a new frame frame drop................................................Drop frames from memory frame prefix...............................................The frame prefix command frame put..........................Copy selected variables or observations to a new frame frame pwf....................................Display name of current(working)frame frame rename..................................................Rename existing frame frames describe..................................Describe frames in memory or in afile frames dir......................................Display names of all frames in memory frames reset.............................................Drop all frames from memory frames save..............................................Save a set of frames on disk frames use.............................................Load a set of frames from disk frget................................................Copy variables from linked frame frlink.................................................................Link frames frunalias.........................................Change storage type of alias variables generate..........................................Create or change contents of variable gsort..................................................Ascending and descending sort hexdump...........................................Display hexadecimal report onfile icd...................................................Introduction to ICD commands icd9.....................................................ICD-9-CM diagnosis codes icd9p....................................................ICD-9-CM procedure codes icd10.......................................................ICD-10diagnosis codes icd10cm.................................................ICD-10-CM diagnosis codes icd10pcs................................................ICD-10-PCS procedure codes import...........................................Overview of importing data into Stata import dbase............................................Import and export dBasefiles import delimited...................................Import and export delimited text data import excel.............................................Import and export Excelfiles import fred.............................Import data from Federal Reserve Economic Data import haver................................Import data from Haver Analytics databases import sas.........................................................Import SASfiles import sasxport5..................Import and export data in SAS XPORT Version5format import sasxport8..................Import and export data in SAS XPORT Version8format import spss.............................................Import and export SPSSfiles infile(fixed format).......................Import text data infixed format with a dictionary infile(free format).........................................Import unformatted text data infix(fixed format).....................................Import text data infixed format input......................................................Enter data from keyboardContents iii insobs.....................................................Add or insert observations inspect.....................................Display simple summary of data’s attributes ipolate.........................................Linearly interpolate(extrapolate)values isid......................................................Check for unique identifiersjdbc...........................Load,write,or view data from a database with a Java API joinby.....................................Form all pairwise combinations within groupslabel.............................................................Manipulate labels label bels for variables and values in multiple languages bel utilities list.........................................................List values of variables lookfor....................................Search for string in variable names and labelsmemory.......................................................Memory management merge..............................................................Merge datasets Missing values.......................................Quick reference for missing values mkdir.............................................................Create directory mvencode.........................Change missing values to numeric values and vice versa notes............................................................Place notes in data obs.....................................Increase the number of observations in a dataset odbc.....................................Load,write,or view data from ODBC sources order.....................................................Reorder variables in dataset outfile...................................................Export dataset in text format pctile............................................Create variable containing percentiles putmata....................................Put Stata variables into Mata and vice versa range.....................................................Generate numerical range recast................................................Change storage type of variable recode...................................................Recode categorical variables rename............................................................Rename variable rename group..............................................Rename groups of variables reshape..............................Convert data from wide to long form and vice versa rmdir............................................................Remove directory sample........................................................Draw random sample save.............................................................Save Stata dataset separate....................................................Create separate variables shell.............................................Temporarily invoke operating system snapshot..............................................Save and restore data snapshots sort.....................................................................Sort data split..................................................Split string variables into parts splitsample.............................................Split data into random samples stack...................................................................Stack data statsby..................................Collect statistics for a command across a by list e shipped dataset type.......................................................Display contents of afile unicode............................................................Unicode utilities unicode nguage-specific Unicode collators unicode convertfile...........................Low-levelfile conversion between encodingsiv Contentsunicode encoding...........................................Unicode encoding utilities unicode locale.................................................Unicode locale utilities unicode translate............................................Translatefiles to Unicode use.............................................................Load Stata datasetvarmanage...........................Manage variable labels,formats,and other properties vl............................................................Manage variable lists vl create....................................Create and modify user-defined variable lists vl drop................................Drop variable lists or variables from variable lists vl list...................................................List contents of variable lists vl rebuild......................................................Rebuild variable lists vl set................................................Set system-defined variable listse dataset from Stata websitexpose...........................................Interchange observations and variableszipfipress and uncompressfiles and directories in zip archive formatGlossary.........................................................................Subject and author index...........................................................Contents v Stata,Stata Press,and Mata are registered trademarks of StataCorp LLC.Stata andStata Press are registered trademarks with the World Intellectual Property Organization®of the United Nations.Other brand and product names are registered trademarks ortrademarks of their respective companies.Copyright c 1985–2023StataCorp LLC,College Station,TX,USA.All rights reserved.。
teradata开窗函数 -回复
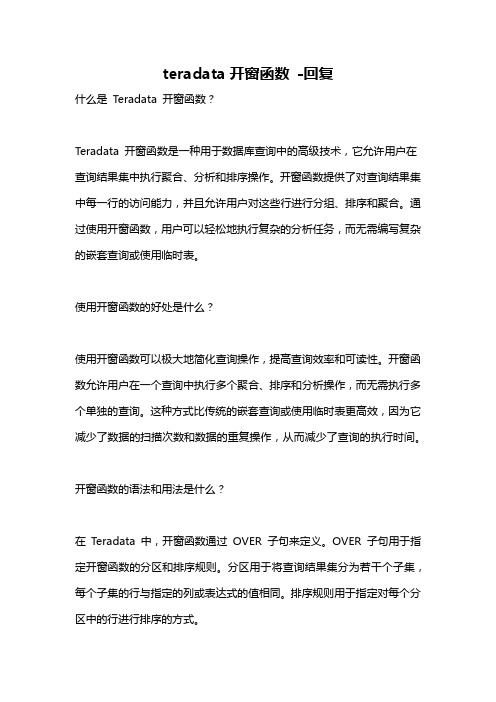
teradata开窗函数-回复什么是Teradata 开窗函数?Teradata 开窗函数是一种用于数据库查询中的高级技术,它允许用户在查询结果集中执行聚合、分析和排序操作。
开窗函数提供了对查询结果集中每一行的访问能力,并且允许用户对这些行进行分组、排序和聚合。
通过使用开窗函数,用户可以轻松地执行复杂的分析任务,而无需编写复杂的嵌套查询或使用临时表。
使用开窗函数的好处是什么?使用开窗函数可以极大地简化查询操作,提高查询效率和可读性。
开窗函数允许用户在一个查询中执行多个聚合、排序和分析操作,而无需执行多个单独的查询。
这种方式比传统的嵌套查询或使用临时表更高效,因为它减少了数据的扫描次数和数据的重复操作,从而减少了查询的执行时间。
开窗函数的语法和用法是什么?在Teradata 中,开窗函数通过OVER 子句来定义。
OVER 子句用于指定开窗函数的分区和排序规则。
分区用于将查询结果集分为若干个子集,每个子集的行与指定的列或表达式的值相同。
排序规则用于指定对每个分区中的行进行排序的方式。
在OVER 子句中,可以使用以下关键字和函数:- PARTITION BY:用于指定分区的列或表达式。
每个不同的值将创建一个新的分区。
- ORDER BY:用于指定分区中的行的排序规则。
可以指定多个列或表达式,并按升序或降序进行排序。
- ROWS BETWEEN:用于指定如何在分区中定义开窗的范围。
可以指定从某一行开始和某一行结束,或者指定从分区的第一行到某一行、从某一行到分区的最后一行、从分区的第一行到最后一行等。
开窗函数的常用类型有哪些?Teradata 提供了多种开窗函数类型,可以满足不同的分析需求。
常用的开窗函数类型包括:- 聚合函数:如SUM、COUNT、AVG、MIN、MAX 等。
聚合函数会对每个分区中的行进行聚合操作,并返回一个聚合结果。
- 分析函数:如RANK、DENSE_RANK、ROW_NUMBER 等。
虚拟变量的引入

REPORT
CATALOG
DATE
ANALYSIS
SUMMAR Y
02
虚拟变量的创建方法
手动创建
确定变量范围
根据研究需求,确定需要引入的虚拟变量及其取值范 围。
创建变量矩阵
根据确定的取值范围,创建相应的变量矩阵,用于表 示各个虚拟变量。
赋值编码
为矩阵中的各个元素进行赋值编码,以表示不同的虚 拟变量取值。
2. 交互作用
虚拟变量可以用于表示两个或多个分类变量之间的交互作用。例如,在回归分析中,可以引入交互项来研究不同类别 之间的相互作用对因变量的影响。
3. 多因素分析
虚拟变量在多因素分析中非常有用,例如在回归分析、方差分析、聚类分析等中。通过引入虚拟变量, 可以研究不同类别之间的差异和交互作用。
虚拟变量的应用场景
虚拟变量的引入会增加模型的复杂度,使得模型更难以解释和理解。
多重共线性问题
如果虚拟变量之间存在多重共线性关系,会导致回归系数不稳定, 影响模型的准确性。
过度拟合问题
过度引入虚拟变量可能导致模型过度拟合,使得模型在训练数据上 表现良好,但在实际应用中表现较差。
如何选择合适的虚拟变量
考虑变量的实际意义 在选择虚拟变量时,应考虑变量 的实际意义,确保选择的变量能 够反映研究问题的实际情况。
1. 市场调查
在市场调查中,常常需要了解不同人群的特征和行为。虚 拟变量可以用于表示不同的群体或类别,例如性别、年龄 段、职业等。
2. 社会科学研究
在社会科学研究中,常常需要研究不同群体之间的差异和 交互作用。虚拟变量可以用于表示不同的社会群体或状态, 例如种族、宗教、教育程度等。
3. 生物统计学
网络安全管理员中级工练习题库(含答案)

网络安全管理员中级工练习题库(含答案)一、单选题(共40题,每题1分,共40分)1、关于机房建设物理位置的选择,下列选项中正确的是( )A、大楼中部B、大楼顶楼C、地下室D、一楼正确答案:A2、关于IDS和IPS,说法正确的是( )A、IDS部署在网络边界,IPS部署在网络内部B、IDS适用于加密和交换环境,IPS不适用C、用户需要对IDS日志定期查看,IPS不需要D、IDS部署在网络内部,IPS部署在网络边界正确答案:D3、双绞线与避雷引下线之间的最小平行净距为( )。
A、600mmB、800mmC、1000mmD、400mm正确答案:C4、在任务管理器的( )选项卡中可以查看CPU和内存使用情况。
A、“应用程序”选项卡B、“进程”选项卡C、“性能”选项卡D、“联网”选项卡正确答案:C5、1000BASE-T标准规定网卡与HUB之间的非屏蔽双绞线长度最大为( )。
A、50米B、100米C、200米D、500米正确答案:B6、网络中使用光缆的优点是什么?( )A、能够实现的传输速率比同轴电缆或双绞线都高B、容易安装C、是一个工业标准,在任何电气商店都能买到D、便宜正确答案:A7、根据《中国南方电网有限责任公司信息系统运行维护管理办法(2014年)》,缺陷指信息系统发生的( ),这些异常或隐患将影响信息系统安全可靠运行、性能、寿命或服务质量。
A、故障B、安全隐患C、异常或存在的隐患(包括信息安全漏洞)D、事件正确答案:C8、应配备集中运行监控系统实现对所有服务器、网络设备、安全设备、数据库、中间件、应用系统的( )。
A、单独控制B、集中测试C、集中监控D、分散监控正确答案:C9、以下( )标准是信息安全管理国际标准。
A、ISO9000-2000B、SSE-CMMC、ISO27000D、ISO15408正确答案:C10、不能防范ARP欺骗攻击的是( )A、使用静态路由表B、使用ARP防火墙软件C、使用防ARP欺骗的交换机D、主动查询IP和MAC地址正确答案:A11、在数据库系统中,当数据库的模式改变时,用户程序可以不做改变,这是数据的( )。
Avamar与Networker备份的共性与区别

– 备份模块——备份什么 ➢Client、DB Agent、Email Agent 、SAP Agent ……
– 备份介质——往哪里备份 ➢TAPE、Disk、VTL……
– 备份架构——用什么方式备份 ➢Lan-Base、Lan-Free、Server-Free
份”,可用于即时恢复 • 在不过度增加 CPU 利用率的情况
下保持高效的整合率
传统备份每周移动 ~200%
应用程序 操作系统
应用程序 操作系统
VMware 虚拟化层 X86 体系结构
CPU
内存
NIC
磁盘
Avamar 每周移动 ~2%
App
App
App
App
App
App
OS
OS
OS
OS
OS
OS
App
App
• 促进了快速、经济高效的部署和更高的投资回报 • 支持 VMotion 以提高部署灵活性 • 每台 VMware ESX 服务器最多可配置两个 Avamar
Virtual Edition for VMware 虚拟工具以实现可扩展性
9
Avamar 针对 VMware 进行了优化
• 客户系统级备份
Avamar Data Store
灵活的部署选项
• Avamar Data Store
– 消除重复数据后的磁盘容量可扩展至 52 TB – 相当于多达数 PB 的累积传统磁盘或磁带备份存储* – 备份介质需求减少至 1/20 到 1/50 – 通过 RAIN 体系结构、RAID、每日完整性检查和冗余的电源、
ABH
- 1、下载文档前请自行甄别文档内容的完整性,平台不提供额外的编辑、内容补充、找答案等附加服务。
- 2、"仅部分预览"的文档,不可在线预览部分如存在完整性等问题,可反馈申请退款(可完整预览的文档不适用该条件!)。
- 3、如文档侵犯您的权益,请联系客服反馈,我们会尽快为您处理(人工客服工作时间:9:00-18:30)。
SDAM: A New data Aggregation Approach for Wireless Sensor NetworksHang Qin Software Engineering State KeyLaboratoryWuhan UniversityWuhan 430072, Chinahangqin100@Hui WangSoftware Engineering State KeyLaboratoryWuhan UniversityWuhan 430072, ChinaHuaibei ZhouInternational School of SoftwareWuhan UniversityWuhan 430072, ChinaAbstract—In this paper, a novel scalable scheme, Scalable Data Aggregation Monitoring (SDAM), is proposed f or ef f icient datagathering with aggregation (fusion) in Wireless Sensor Networks (WSN). Di f f erent f rom existing schemes, SDAM not only optimizes data transmission cost, but also incorporates the unction of data f usion, which is crucial f or emerging sensor networks with data management and high availability requirements. Employing a randomized algorithm that allows f usion points to be chosen by nodes’ data amount, SDAM can achieve high availability or sensor nodes, which results the optimal solution f or nowadays system setup. Simulation results demonstrate that SDAM scheme can re lect the node status promptly, and save the network throughput of sensor nodes. Therefore, the lifetime of WSN is significantly extended.Keywords-sensor networks, data aggregation, zone monitoring, timestampI.I NTRODUCTIONWSN is an accumulation of sensors interconnected by wireless communication channels. Under the control of the network, every sensor node is a small device that can collect data from surrounding area, communicate with each other, and carry out computation. Long distance communication is normally achieved in a multi-hop manner. Thanks to recent advances in remote monitoring system, such networks are progressing rapidly, and are expected to be popular in applications such as environment monitoring, intrusion detection, and earthquake warning.Nonetheless, existing strategies miss one vital measures in the optimization space for routing correlated data, namely, the data aggregation cost, which may not be negligible for certain applications. As the result, WSN monitoring field parameters need to seek simple functions (for instance, average, maximum, or minimum) with cost-effectiveness. Meanwhile other sensor nodes may require complex operations for data aggregation. In terms of network availability for monitoring algorithm, the sensor data fusion has been shown in the same order of data communication. Partitioning and recombining information are demonstrated as two aggregation process examples in experiment.II.B ACKGROUND AND R ELATED W ORK WSN have acquired much attention because of the potential application of the technology. Numerous data communication protocols have been proposed lately, such as: EPAS (Energy-Efficient Protocol for Aggregator Selection ) [1], TAG (Tiny AGgregation)[2], TTDD (Two-Tier Data Dissemination)[3], GRAB(GRAdient Broadcast)[4], MF ST (Minimum F usion Steiner Tree)[5]. Three types of data collections in WSN are discussed: (1)Event-based data, such as intrusion detection or object tracking, are accumulated when an event at a particular locale within the deployment region comes. The event is strengthened by detecting sensors with local agreement, and presented to the control authority.(2)Global state-based data, such as temperature or humidity, are accumulated by sensor nodes all over the deployment area and transmitted to the sink. (3)Focused state-based data is accumulated in response to a query sent to selected sensors requesting relevant data. Our interest here is in focused state-based data.III.S YSTEM M ODEL AND SDAM D ESIGNA.Problem Formulation and Sensor Node’s StateTransformationIn this paper, aggregation organizer determines the status of node machine from the data packet content and interval sent out by sensor node[6][7], and the conceivable node machine status may be: Down,Shift, Activate and Pause. When the data packet of node machine is received by aggregating mechanism, the aggregation organizer can search a new buffer region in the shared memory area to save this node’s information. Down is aggregation organizer has not received the data packet after waiting for a lot of time. At this time sensor node is closed down or has a fault, and aggregation organizer will inform this status to the administrator. Shift shows sensor node has been closed down for a long time, and aggregation organizer can shift this node machine from theshared memory area for the saving of shared memory area. Activate defines sensor node can work normally and aggregation organizer can activate sensor node’s previous data. Pause describes aggregation organizer can not receive sensor node’s data packet in the certain time, it represents sensor node is busy, fault or failure, and aggregation organizer can wait for the data packet continuously. Figure 1 depicts theaggregation states model of the above states:Figure 1. Aggregation States Model of Sensor NodeB.Finite Automata of Sensor Node’s State TransitionGive for the formal approach of sensor network nodes, the node machine’s states transformation diagram is enhanced in this section. In Figure 2, the start status and the terminal status are added. Ready depicts the initialization of aggregation organizer, double circles show the final state in this status diagram and one state transformation procedure of node machine. Thus the Deterministic Finite Automata (DF A) [6] can be constructed from sensor nodes’ state transformation diagram:Figure2. Transition diagram of DFA to node via aggregationDef inition 3.1DFA node =(T, Q, δ, F, S ). T = {p, d, s, r }, pdefines having not received data packet in t p senonds, d stands for having not received data packet in t d seconds, r depicts having not received data packet in t s seconds, r shows receive one data packet. Q is the state set, Q = {R, S , D, P, A }, R stands for the ready status, S stands for shift, D stands for Down, P stands for pause, A stands for activate. And T is transaction set. δstands for a state transformation function:Q ×T → Q , and the state transformation formula can be showed in Table 1. F is the final states set, F = {S }, and F ⊆Q .Table 1. the sensor nodes’ transformation aggregation rule r p d s R A S A D A S P A D A A PThe sensor nodes’ one action set from the above equation set can be got, this action depicts the process from start state to failure state, or any states from the start status to the shutting down status. And this set can be described by the regular expression: R node =r(r + pr + pdr + pdsr)* pds . Any sentence produced by the regular expression R node is one action of node machine, such as rpds depicts the failure for some reason after the sensor nodes send one information packet to the monitoring mechanism. Therefore finite automata DFA node and regular expression R node provide the formal description for the research of sensor nodes’ state transformation.C.SDAM Algorithm DesignIn this Section, the data aggregation algorithm is proposed as below.Epoch 1COUNT packet is the number of 8K data packets, LENGTH total is the overall length of the data to be sent, LENGTH data is the length of data to be hold in one packet, MOD is the arithmetic operation, << is the shift left operation, packet is one data packet, TS is timestamp, and sequence is the current sending packet serial number. Whereas LENGTH total MOD LENGTH data = 0, all the data can be hold in the COUNT packet data packets:1.COUNT packet ←LENGTH total / LENGTH data ,TS ←current system time, LENGTH data ← 8K – the length of information packet header, id ← 0;2.if LENGTH total MOD LENGTH data = 0, then cover← (1 << COUNT packet ) – 1, else cover ← (1 << (COUNT packet + 1)) – 1;3.if COUNT packet = 0, then goto 7;4.run distribute( ), packet_cover ← cover, packet_id← 1 << id, packet_timestamp ←TS ,LEGTH packet ← 8K5.copy LENGTH data data to the information message atthe beginning of data’s id ×LENGTH data offset address, send information packet;6.id ← id + 1, if id = COUNT packet ,then goto 4;7.if LENGTH total MOD LENGTH data = 0, then goto 10;8.packet_cover ←cover, packet_id ← id,packet_timestamp ←TS ,LEGTH packet ←LENGTH total MOD LENGTH data + the length of information packet;9.copy LENGTH data data to the information message atthe beginning of data’s id ×LENGTH data offset address, send information packet;10.return .Epoch 2:packet is the information packets received by aggregation organizer, TS is the timestamp when aggregation organizer receives the former information packet, and the initialization is TS ← 0.Aggregation organizer allocates 256K buffer region to save the received data, LENGTH data is he length of data to be hold in one packet, | is the arithmetic OR operation. The current packet will be discarded when it is older than the former packet:1.if packet_timestamp < TS ,goto 5;2.copy the packet data with the length of packet.LENGTH data to the buffer region at the position of corresponding offset address;3.run election( ), if packet_timestamp > TS ,thencover ← 0, TS ←packet_timestamp; 4.cover ← cover | packet_id;5.if cover = packet_cover, then clean up theinformation in the buffer region, and save it in the shared memory area; 6.return .IV.T RANSMISSION AND P ACKET S ALVAGING A NALYSIS OFSDMAPerformance of this sensor monitoring system can be evaluated from two aspects: the response time and the sensor network bandwidth in occupancy. In order to describe the performance of remote monitoring system more accurately, it is modeled as: Definition 4.1 Node machine performance 4-tuple PF= (F com ,V max , TP, LT ), F com (Communication F requency) is the bandwidth of communication, which is the number of sensor nodes’ request from monitoring node to sensor nodes per second, V max is the transmission speed maximum of node machine sensor network device, TP (Throughput Percentage) describes the percentage of sensor network bandwidth in theresponding process of node machine, LT (Latency Time)defines the monitoring request time of node machine responsemonitoring time. Therefore the responding time LT is the sumof sensor network communication time and the sensor nodes data aggregation time, and LT = T agg +T com . Sensor network throughput TP is closely related with the communicationquantity, communication frequency and the maximum sensor network transmission ratio of sensor nodes and monitoring node, and as is show in formula (1), where S cmd and S data are the size of command packet and information packetrespectively:max()100%com cmd data F S S TP V +=× (1)De inition 4.2 Sensor aggregation monitoring performancemodel SDAM PF = (M, E, N, ATP, ALT ), and M (Medium)represents what wireless network medium the remotemonitoring system runs, M ∈{L, X, P }, L (WLAN) is thewireless local area network, X (WiMAX) is the wirelessmetropolitan area network or the Internet, P (WPAN) is thewireless personal area networks. E (Environment) describes thehardware and soft configuration environment of the whole sensor networks. Hardware environment includes the configuration of sensor nodes, monitoring nodes and wireless network connectivity, such as the number of sensor nodes, the transmission ratio of wireless network communication and the capacity of data storage device. Software environment includes the version of operating system, communication protocol and so on. N (Nodes) is the set of performance 4-tuple in the monitored sensor nodes, thus N={x | x=PF }. ATP (Average Throughput Percentage) defines the network bandwidth percentage of monitoring system in the whole wireless sensor networks. ALT (Average Latency Time) describes the average responding time of sensor nodes.max cmd datacom n Nn NS S F n TPnV ATP NN∈∈+××==¦¦ (2)()comagg n Nn Nn LT n TT ALT NN∈∈××+==¦¦ (3)As far as the average latency time ALT is concerned, it is responsible for the N ,T com and T agg in every sensor nodes. With the increasing of sensor nodes number ΊN Ί,T com inevery node is becoming larger and larger. It is because monitoring node will process more information packet from the sensor nodes, while the sensor nodes’ processing time T agg is invariable. With the increasing of sensor nodes’ number, the average responding time will decrease. As the percentage ATP of the monitoring system in the whole wireless sensor network, it is closely related with the number of sensor node VΊN Ί, the size of command packet S cmd and information packet S data, the transmission speedmaximum of wireless network device V max, and thecommunication frequency F combetween the monitoring nodesand sensor nodes. When the number of sensor nodes isincreasing, ATP is invariable practically, although theresponding time in the sensor nodes is becoming larger. It isbecause S cmd ,S data ,V max and F com are invariable. At the sametime, ATP is invariable practically when the overload of the sensor nodes system becomes larger. Due to the invariability of S cmd and S data , the change of ATP depends on F com and V max .V.S IMULATION S TUDY To verify the algorithm introduced above, a simulationprogram is run and shows following results.Suppose the surveillance region have 2500 square meters with a foursquare shape. Comparing the results of two different groups shown in Figure 3 and Figure 4, the overheadof WSN increase slowly. Moreover, if increasing theprobability of edge fails, the overhead decreases on thecontrary.When the system overload as the sensor nodes increase,the processing time T agg is becoming larger, and T com isbecoming larger even if these overloads focus on the wirelessnetwork communication. Otherwise T com will has a littlevariation, triggered by the increasing of sensor nodes overload. Therefore the average responding time becomes larger when the system overload is increasing.Number of Sensor NodesL a t e n c y T i m e (m S e c )Figure 3. Data aggregation and Latency101010101010Packet CountL a t e n c y T i m e (m S e c )Figure 4. Transmission Cost and LatencyIt is obviously the ALT is increasing with the increase of F com and the decrease of V max . This provides an approach for the administrator to reduce ALT when the wireless network overload is increasing. We can not only configure the sensornetworks monitoring system for the increase of collecting information interval and the decrease of F com , but also deploythe faster sensor networks device for the increase of V max .VI.C ONCLUSIONIn this paper, a scalable data fusion server based on WSN architecture is proposed. Techniques such as parallel data storing and distribution policy, adaptive timestamp data aggregation algorithm, are discussed extensively. Simulation results based on existing data aggregation monitoring system demonstrate the expected advantages. Also, the results will lead to further evaluation of these techniques later. In the future, the impacts on the performance of the data fusion system will be explored in details, especially it is induced by communication operation reduction. The principle of dynamic transmitting rate at aggregator to reduce the user perceived latency needs further study in depth.R EFERENCES[1] Yuanzhu, Peter Chen, and Arthur L. Liestman, “A Hierarchical Energy-Efficient F ramework for Data Aggregation in Wireless Sensor Networks”, IEEE Transactions on vehicular technology, Vol. 55, No. 3, May 2006, 789–796[2] S. Madden, M. J. Franklin, J. M. Hellerstein, and W. Hong, “TAG: A tinyaggregation service for ad-hoc sensor networks”, in Proc.5th S ymp. OSDI, Boston, MA, Dec. 2002.[3] F.Ye, H.Luo, J.Cheng, S.Lu and L.Zhang,“A two-tier data disseminationmodel for large-scale wireless sensor networks”, in Proc. MobiCom, Atlanta, GA, Sep. 2002[4] F. Ye, G. Zhong, S. Lu, and L. Zhang, “Robust data delivery protocol forlarge scale sensor networks”, in Proc. IEEE Int. Workshop IPS N, Palo Alto, CA, Apr. 2003.[5] Hong Luo, Yonghe Liu and Sajal K. Das, “Routing Correlated Data withFusion Cost in Wireless Sensor Networks”, IEEE Transactions on mobile computing , Vol. 5, No. 11, November 2006, pp. 1620–1632.[6] Harry R. Lewis and Christos H. Papadimitrious, Elements of the Theoryof Computation, Prentice Hall, Second Edition, 2006, pp.34–39.[7] Alberto Cerpa and Deborah Estrin, “ASCENT: Adaptive Self-ConfiguringSensor Networks Topologies”, in Proc. 11th Joint Conf on IEEE Computer and Communications Societies (INFOCOM), New York, NY, June 2002.。