Upper limits on the size of a primordial black hole
Pushingthelimits
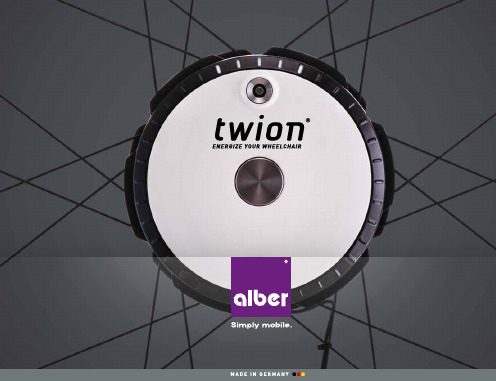
3
FEEL THE SPEED.
Faster and further: The dynamic motors accelerate the wheelchair up to 6 km/h (optionally 10 km/h*). So you can really get going and enjoy the ride. The efficient energy recovery system feeds energy back into the batteries during braking, providing a 10 % range extension for greater freedom.
So it‘s even more logical that Alber also offers its service digitally. Simply download the twion Mobility App at no cost from Google Play Store onto all android-capable smartphones and use the all-round service at any time. For example, if service is required the diagnostic checklist can help to localise the error in the wheels and, if necessary, set up direct contact to our Service Team.
D Fender Manual
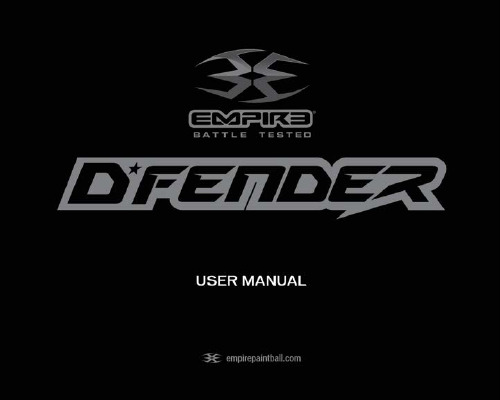
WARNING: Never carry your paintball marker uncased when not on a playing field. The non-playing public and law enforcement personnel may not be able to distinguish be-tween a paintball marker and a firearm. For your own safety and to protect the image of the sport, always carry your marker in a suitable marker case or in the box in whichit was shipped.CAUTION: Paintballs may remain in the feed tube system after the loadersection is empty. Always check for paintballs in the feed tube and breech toprevent accidental discharge!3.1 3.23.3 3.4adapter). The D*Fender’s ASA is located3.5 WARNING:Insert the threaded end of the air cylinder• Remember compressed air and nitrogen systems cylinders can be extremelydangerous if misused or improperly handled. Use only cylinders meetingD.O.T., TC or regionally defined specifications.• Never disassemble your tank or tank regulator. Only a qualified and trainedtechnician should perform work on your tank and tank regulator.• Never add any lubricants or greases into the fill adapter on yourtank regulator.WARNING: Before switching your D*Fender marker On. Make sure it ispointed in a safe direction. Everyonewithin firing range should always use paintball approved eye and face protectionin the presence of live paintball mark-ers. Make sure the marker is set to “SafeMode”, before following the steps below.ing device. Remember that the ultimate safety device is you, the operator. 4.1FLASH FREQUENCY FlickerFast Flash Slow Flash Solid Glow Breech Sensor is ON, Ball is sensed Breech Sensor is ON, No Ball is sensed Breech Sensor is turned OFF Breech Sensor is malfunctioning/eyes dirty BREECH SENSOR STATUS LED SAFE COLORBlue GreenBattery level is good Replace batteryBATTERY LEVELPOSITION 321Safe Green or Blue Safe Red Semi-Auto Amber Burst Purple Full-AutoLED COLOR FIRING MODEWARNING: Check the velocity every time prior to using your D*Fender marker. The velocity can be adjusted via the Velocity Adjuster (fig 5.1) which is accessible through either side of the marker. Adjust the velocity with a 3/32” hex wrench by inserting it into the adjuster holes and rotating.velocity is achieved. A paintball specificincrease the input pressure, and outwardWARNING: Do not increase regulator pressure over 200psi or it will damage the marker.5.15.2The (2) second set screw adjusts the rearctivation point. For best results,the activation point should be set right inthe middle of the total Trigger movementIf any of the set screws are over adjusted5.31. Start with the Ramp in the OFF position(fully toward the back of barrel, Fig 5.4).Go to your fields shooting range or other 5.4LEDSolid AquaSolid PurpleSolid WhiteFlickering RedFlickering Green Solid AmberSolid BlueSolid GreenSolid RedFiring Mode Max Rate of Fire Dwell Trigger De-bounce Ball In Place Time Ramp Start Ramp Sustain Burst Shot Count Calibrate Selector SETTING # OF BLINKS 54321Semi Auto Burst Ramp Full Auto Select Fire FIRING MODEPOSITION 321Safe Green or Blue SafeRed Semi-Auto Amber Burst PurpleFull-AutoLED COLOR FIRING MODE BLINKS VALUE VALUE BLINKS # OF BPS BPS # OF 513.5614.0714.5413.0312.5212.0111.58.088.599.0109.51110.01210.51311.014BLINKS VALUE VALUE BLINKS # OF BPS BPS # OF 1920.01819.51719.01618.51518.015.02115.52216.02316.52417.0252017.5BLINKS IN MS IN MS BLINKS # OF DWELL DWELL # OF 59.069.57810.048.538.027.517.03.093.5104.0114.5125.0135.5146.06.515the user to lock the settings mode, which WARNING: Once you are done using your D*Fender it is extremely important thatyou fully unload the marker of all paintballs and compressed air. Never look down the barrel unless it’s removed from the marker.6.1CAUTION: Paintballs may remain in the feed tube system after the loadersection is empty. Always check for paintballs in the feed tube and breech to prevent accidental discharge!WARNING: Before attempting to perform any disassembly or maintenance on your D*Fender marker, make sure that all paintballs and air sources have been removed from the marker and that the regulator gauge reads 0 psi. Install a barrel blocking device, move the Selector Switch to the Safe position and powerthe marker Off.WARNING: Do not rinse the D*Fender under water, as you may damage the marker’s electronics. WARNING: Once the Side covers are removed the Eyes and Detents may be loose and can fall out of the internal assembly.Buttons and slide the Top Rail toward the rearof the marker about an inch (2.5 cm) and lift8.1WARNING: Before removing the Quick Strip Pins from marker. Confirm the marker is degassed and the gauge reads 0 psi.Push the pins with finger or hex wrench fromOnce the Quick Strip Pins are removed, thecurved end plugs correctly into the feed tubeWARNING:• Before the Internal Assembly can be removed, the Side Covers, Top Rail, Quick Strip Pins, Barrel, and Feed Ramp must be removed first.• Before removing the Internal Assembly, make sure the Eyes are still properly within the Body to prevent damaging the eye harness. Using two hands place one finger in the8.28.38.4WARNING: The Internal Assembly must be removed prior to splitting the shells. See page 15 for removal instructions.WARNING:Never carry your Marker uncased when not on a playing field. The non-playing public and law enforcement personnel may not be able to distinguish between a paintball marker and firearm. For your own safety and to protect the image of the sport, always carry your Marker in a suitable marker case or in the box in which it was shipped. When you are finished using your Marker it is important that you prepare it for storage. This will not only serve to increase the life of the marker, but will assure optimum performance on your next outing.unauthorized persons.CAUTION: Paintballs may remain in the feed tube system after the loader section is empty. Always check for paintballs in the feed tube and breech to prevent accidental discharge!11. DIAGRAMS/PARTS LISTDIAGRAM #PART DESCRIPTION SKU 1STANDARD ALU BARREL72720 2ALU BARREL INSERT- SIZE .69011523 2ALU BARREL INSERT- SIZE .68511522 2ALU BARREL INSERT- SIZE .68011521 3O-RRING BUNA-N70 DUR-018 (.739 ID)72508 4O-RING BUNA-N 70 DUR 1MM CS X 19.5MM ID72488 5APEX BARREL72721 6O-RING BUNA-N 70 DUR -021 (.926 ID)11506 7APEX WIRE SPRING11504 8APEX BODY INSERT TOP11500 9APEX HOUSING TUBE11502 10APEX ADJUSTER11505 11APEX DEFLECTOR11503 12APEX BODY INSERT BOTTOM11501 13MAIN SPRING17535 14BOLT RUBBER TIP17533 15BOLT17532 16O-RING BUNA-N 70 DUR -017 (.676 ID)17534 17BOLT GUIDE72722 18O-RING BUNA-N 70 DUR 2.5MM CS X 23.0MM ID72340 19POPPET SEAL17629 20POPPET ASSEMBLY WITH SPRING17539 21POPPET SPRING17623 22VELOCITY ADJUSTER72723 23O-RING BUNA-N 70 DUR 2.4MM CS X 10.80MM ID17538 24O-RING URETHANE 90 DUR 2MM CS X 10MM ID 17540 25DOWEL PIN .125 DIA X .37572724 26O-RING BUNA-N 70 DUR 1.5MM CS X 12MM ID 17537 27SCREW BHCS 6-32 X .250 (SMALL HEAD)17652 28RELEASE BLOCK72725 29SPRING .120OD X 0.50FL .020WD RATE 18.LB/IN72584 30RELEASE BUTTON72726 31BEARING BALL 1/8 DIA 72585 32AIR TRANSFER GASKET17530 33MANIFOLD7272735O-RING BUNA-N 70 DUR 0.8MM CS X 12.0MM ID17553 36AIR TRANSFER TUBE MALE17551 37SOLENOID 17528 38CHECK VALVE17531 39MAIN CURCUIT BOARD72728 40EYE HARNESS72729 41BALL DETENT72577 42BODY72730 43SCREW SET 10-32 X .375 CP72654 44REGULATOR DISK17591 45REGULATOR PISTON72360 46REGULATOR PISTON RETURN SPRING72395 47O-RING BUNA-N 70 DUR -008 (.176 ID)10761 48TUBE LOWER72731 49O-RING BUNA-N 70 DUR -012 (.364 ID)72490 50SCREW SET 10-32 X 1.250 CUP POINT72732 51REGULATOR MOUNT72733 52REGULATOR FILTER COVER72359 53O-RING BUNA-N 70 DUR 1.5MM CS X 6.5MM ID72509 54REGULATOR FILTER72358 55REGULATOR BODY72734 56REGULATOR SEAL72364 57O-RING URETHANE 70 DUR -012 (.364 ID)10257 58REGULATOR ON/OFF PIN72372 59REGULATOR NUT72599 60O-RING BUNA-N 70 DUR -006 (.114 ID)72489 61REGULATOR SEAL RETAINER72652 62PIN REGULATOR72363 63REGULATOR LEVER72613 64PIN WEDGE72614 65REGULATOR CAM SPRING72369 66SCREW SHSS 3/16 DIA 3/8 LG 8-32 x .25072512 67300PSI EMPIRE GAUGE72375 68REGULATOR OPP COMPLETE17597 69MAIN REGULATOR SPRING72608 70REGULATOR CAP7261572O-RING BUNA-N 70 DUR -020 (.864 ID)40920 73CATCH CUP72736 74BEARING .250 ID X .500 OD X .125 THK38803 75E-RING 38823 76SPROCKET 31074 77GEAR BOX COVER38496 78SCREW PHCS 4-40 X .625 38827 79SENSOR COVER38497 80DRIVE SENSOR72737 81O-RING BUNA-N 70 DUR -028 (1.375 ID)38820 82PULLEY GEAR38837 83MOTOR WITH WIRING HARNESS72738 84GEAR PIN - DOWEL PIN .078 DIA X .50038828 85DRIVE SHAFT72739 86NUT HEX 4-4038805 87CARRIER72740 88SCREW THCS 6-32 X .37538982 89SPRING .180OD X 1.500FL .026WD RATE 8.0LB/IN72741 90BUTTON RELEASE72742 91TOP RAIL72743 92RIGHT SHELL72744 93SIDE RAIL ADAPTER72745 94SIDE RAIL72746 95SCREW SHCS 6-32 X .437572747 96CONNECTER BOARD SCREW W/ WASHER72779 97HARNESS CIRCUIT BOARD (COMPLETE)72749 98DOOR RETAINER38938 99SPRING .114OD X .31FL .014WD RATE 9.6LB/IN38939 100DOOR SWITCH38937 101RIGHT SIDE COVER72750 102LID SPRING31025 103STANDARD LID72751 104QUICK LOAD LID 72752 105MAGNET 8MM DIA .X 4MM31009 106MAGNET 8MM DIA X 6MM38475 107BATTERY DOOR72753109HOLDER BATTERY AA38804 110BATTERY HARNESS HOLDER17823 111BATTERY HARNESS17715 112FEED RAMP BACK72755 113TRIGGER72756 114SCREW SET 6-32 X .250 FLAT POINT17523 115SPRING .180OD X .310FL .012WD RATE 3.0LB/IN 72757 116BEARING .156 ID X .3125 OD X .155572381 117SCREW SHSS 5/32 DIA 5/16 LG 6-32 X .13872758 118FEED RAMP FRONT72759 119SPRING PIN .0625 DIA X 1.00072760 120FEED RAMP TOP72761 121SCREW SHCS 6-32 X .312572762 122LID RETAINER72763 123SCREW SHCS 6-32 X .75017815 124TRIGGER GUARD72764 125SELECTOR CIRCUIT BOARD72765 126SWITCH SHAFT INNER WITH MAGNET72766 127SELECTOR SPRING72767 128NUT HEX 6-32 .25 WIDE x .092 THK72768 129FOREGRIP RH72769 130FOREGRIP LH72770 131SCREW SHCS 6-32 x .87572771 132COMPLETE SHELL SPRING PIN (SHORT)72772 133LEFT SIDE COVER72773 134POWER BUTTON17712 135LIGHT PIPE17710 136SCREW FHCS 4-40 X .50017650 137SELECTOR SWITCH OUTER72774 138SCREW BHCS 6-32 X .25017653 139SCREW FHCS 6-32 X .75072775 140SLING MOUNT72776 141LEFT SHELL 72777 142COMPLETE SHELL SPRING PIN (LONG)71933 143RUBBER GRIP17717 144O-RING BUNA-N 70 DUR 1.0mm CSx6.0mm ID72546。
judgingthepositionofthebigdipperandpolaris
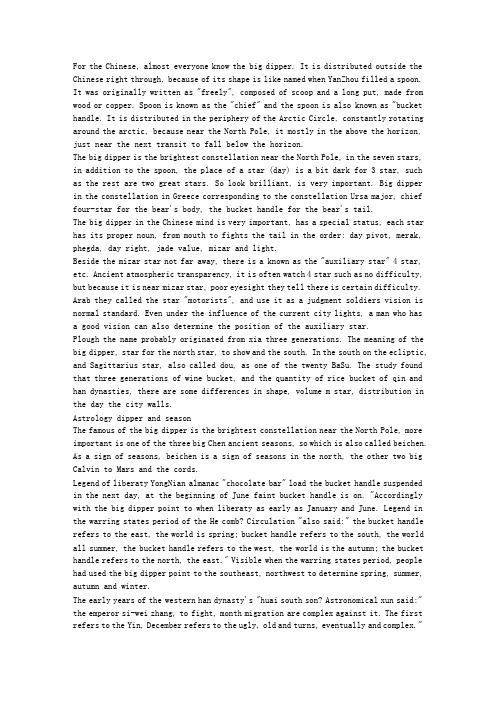
For the Chinese, almost everyone know the big dipper. It is distributed outside the Chinese right through, because of its shape is like named when YanZhou filled a spoon. It was originally written as "freely", composed of scoop and a long put, made from wood or copper. Spoon is known as the "chief" and the spoon is also known as "bucket handle. It is distributed in the periphery of the Arctic Circle, constantly rotating around the arctic, because near the North Pole, it mostly in the above the horizon, just near the next transit to fall below the horizon.The big dipper is the brightest constellation near the North Pole, in the seven stars, in addition to the spoon, the place of a star (day) is a bit dark for 3 star, such as the rest are two great stars. So look brilliant, is very important. Big dipper in the constellation in Greece corresponding to the constellation Ursa major, chief four-star for the bear's body, the bucket handle for the bear's tail.The big dipper in the Chinese mind is very important, has a special status, each star has its proper noun, from mouth to fights the tail in the order: day pivot, merak, phegda, day right, jade value, mizar and light.Beside the mizar star not far away, there is a known as the "auxiliary star" 4 star, etc. Ancient atmospheric transparency, it is often watch 4 star such as no difficulty, but because it is near mizar star, poor eyesight they tell there is certain difficulty. Arab they called the star "motorists", and use it as a judgment soldiers vision is normal standard. Even under the influence of the current city lights, a man who has a good vision can also determine the position of the auxiliary star.Plough the name probably originated from xia three generations. The meaning of the big dipper, star for the north star, to show and the south. In the south on the ecliptic, and Sagittarius star, also called dou, as one of the twenty BaSu. The study found that three generations of wine bucket, and the quantity of rice bucket of qin and han dynasties, there are some differences in shape, volume m star, distribution in the day the city walls.Astrology dipper and seasonThe famous of the big dipper is the brightest constellation near the North Pole, more important is one of the three big Chen ancient seasons, so which is also called beichen. As a sign of seasons, beichen is a sign of seasons in the north, the other two big Calvin to Mars and the cords.Legend of liberaty YongNian almanac "chocolate bar" load the bucket handle suspended in the next day, at the beginning of June faint bucket handle is on. "Accordingly with the big dipper point to when liberaty as early as January and June. Legend in the warring states period of the He comb? Circulation "also said:" the bucket handle refers to the east, the world is spring; bucket handle refers to the south, the world all summer, the bucket handle refers to the west, the world is the autumn; the bucket handle refers to the north, the east." Visible when the warring states period, people had used the big dipper point to the southeast, northwest to determine spring, summer, autumn and winter.The early years of the western han dynasty's "huai south son? Astronomical xun said:" the emperor si-wei zhang, to fight, month migration are complex against it. The first refers to the Yin, December refers to the ugly, old and turns, eventually and complex."Visible in the early western han dynasty have beidou seasons, the method of development to the bucket handle to Yin bases such as twelve azimuth, determine such as January and February 12 months.Stone-working of "historical records? Day GuanShu" are concluded: "the fight for the emperor, the central, near the suburbs. The Yin and Yang, built four, all five lines, section c, the age, summed up in the bucket." Speech day emperor sit on composed of beidou carriage circuit, line is a week for a year, and thus distinguish between Yin and Yang are two half of the year, points out the four seasons and five times, line degree of the throttle and the sun can be determined. Therefore, people use the orientation of the big dipper, it is of great significance to determine farming calendar. This is why the ancients then attaches great importance to the plough. Fight for emperor and mizar attached the story of the starIn the shandong Wu Liang shrine in the murals of the stone carving, carrying a "fight for emperor car" figure, in the middle of the figure engraved with the big dipper stars, star mizar, and with a little star. Little star beside a winged fairy, he clearly is the symbol of auxiliary star.But he did not say what all is two stars. , "said sung? Day chinese-speaking's star 8 bi xing, right at the first seven stars, see; 9 yue fu, stars in star left 6, common." Song Zhi bi star, was missing, it is difficult to say. And 9 star for the auxiliary mizar star, is not clear. All the two stars, when outside scoops side."Historical records? Day GuanShu" said: "scoop side has two stars, one for the spear, obtrusive; outside for a shield, days back." Set solution lead jin burning yue: ", far from big dipper, two, in the south, a XuanGe." By "the Book of Jin? Day chinese-speaking" and so on the map, the XuanGe between wave light and obtrusive, visible "day GuanShu" wrong "and". Beidou nine star star for XuanGe 8, 9 star to be seen.As a big dipper season nine star indicates a positive, "huainan zi? When xun" has the following records:Meng Chun moon, be seen refers to Yin;Month of air, ostentatiously refers to the frame;Chen JiChun moon, be seen finger;......JiDong moon, be seen ugly."When the training with loud star at different points to 12 months to determine time, indicates the beidou star has used its nine points to determine the function of time. "Training" when using the ostentatious, rather than XuanGe or point of light, star is 9 obtrusive rather than its star can be determined. Because if use beidou nine star judge season, could not rule out 9 star with 8 star. When the training records, further confirmed the flaunting for beidou nine star.。
fazizogluomerg@csucsbedu Lower Bounds on Communication Loads and Optimal Placements in Toru
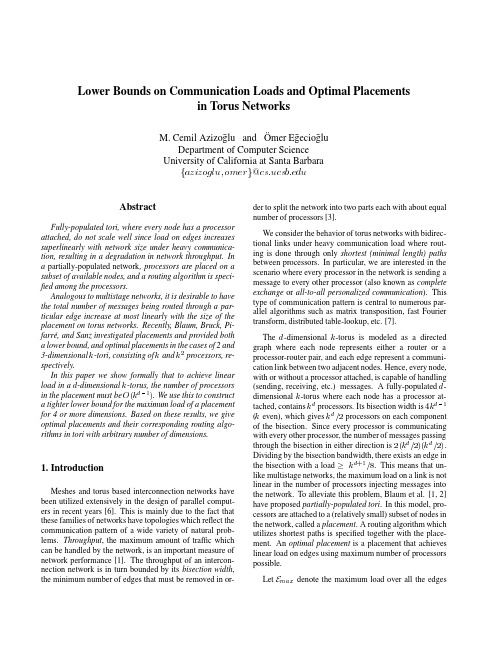
Lower Bounds on Communication Loads and Optimal Placementsin Torus NetworksM.Cemil Azizo¯g lu and¨Omer E¯g ecio¯g luDepartment of Computer ScienceUniversity of California at Santa BarbaraAbstractFully-populated tori,where every node has a processor attached,do not scale well since load on edges increases superlinearly with network size under heavy communica-tion,resulting in a degradation in network throughput.In a partially-populated network,processors are placed on asubset of available nodes,and a routing algorithm is speci-fied among the processors.Analogous to multistage networks,it is desirable to have the total number of messages being routed through a par-ticular edge increase at most linearly with the size of theplacement on torus networks.Recently,Blaum,Bruck,Pi-farr´e,and Sanz investigated placements and provided botha lower bound,and optimal placements in the cases of2and 3-dimensional-tori,consisting of and processors,re-spectively.In this paper we show formally that to achieve linear load in a-dimensional-torus,the number of processorsin the placement must be.We use this to construct a tighter lower bound for the maximum load of a placementfor4or more dimensions.Based on these results,we give optimal placements and their corresponding routing algo-rithms in tori with arbitrary number of dimensions.1.IntroductionMeshes and torus based interconnection networks have been utilized extensively in the design of parallel comput-ers in recent years[6].This is mainly due to the fact that these families of networks have topologies which reflect the communication pattern of a wide variety of natural prob-lems.Throughput,the maximum amount of traffic which can be handled by the network,is an important measure of network performance[1].The throughput of an intercon-nection network is in turn bounded by its bisection width, the minimum number of edges that must be removed in or-der to split the network into two parts each with about equal number of processors[3].We consider the behavior of torus networks with bidirec-tional links under heavy communication load where rout-ing is done through only shortest(minimal length)pathsbetween processors.In particular,we are interested in the scenario where every processor in the network is sending amessage to every other processor(also known as complete exchange or all-to-all personalized communication).This type of communication pattern is central to numerous par-allel algorithms such as matrix transposition,fast Fourier transform,distributed table-lookup,etc.[7].The-dimensional-torus is modeled as a directed graph where each node represents either a router or a processor-router pair,and each edge represent a communi-cation link between two adjacent nodes.Hence,every node, with or without a processor attached,is capable of handling(sending,receiving,etc.)messages.A fully-populated-dimensional-torus where each node has a processor at-tached,contains processors.Its bisection width is(even),which gives processors on each component of the bisection.Since every processor is communicating with every other processor,the number of messages passingthrough the bisection in either direction is. Dividing by the bisection bandwidth,there exists an edge in the bisection with a load.This means that un-like multistage networks,the maximum load on a link is not linear in the number of processors injecting messages into the network.To alleviate this problem,Blaum et al.[1,2] have proposed partially-populated tori.In this model,pro-cessors are attached to a(relatively small)subset of nodes in the network,called a placement.A routing algorithm which utilizes shortest paths is specified together with the place-ment.An optimal placement is a placement that achieves linear load on edges using maximum number of processors possible.Let denote the maximum load over all the edgesfor the placement.Blaum et al.give the lower bound(1) If is constrained to be of the form,then they also give placements of sizes for and for,together with routing algorithms.These placements are optimal in the sense that the two lower bounds are actually achieved by the placements.How do we justify that in general a maximal size place-ment that can achieve linear load is?If the place-ment has size for some constant,then mimicking the case of the fully-populated-dimensional-torus,This seems to imply that linear load is at least possible for .This is a faulty argument however,as we do not know a priori that number of edges needed to split into two equal size pieces is the same as the bisection width of the whole torus.This may push the size of an optimal placement above or below.In this paper,we introduce the concept of bisection width with respect to a placement,and use its properties to prove that in a-dimensional-torus,the size of an opti-mal placement is.Given a placement of max-imal size,we also prove that there exists an edge separator of size which splits the torus into two components with processors of on each side.This gives a lower bound of the form(2) for maximum load.In(2)is a constant independent of. This is a tighter lower bound for the load for large parame-ters than the lower bound(1).Finally,we give optimal placements achieving the lower bound(2)and their corresponding routing algorithms in tori with arbitrary number of dimensions.2.Preliminaries and Problem DefinitionIn this section,we formalize the terminology used and give necessary definitions.Definition1Torus:The-dimensional-torus is a di-rected graph,with vertex setwhere denotes the integers modulo,and edge setsuch that1(mod k),andforhas a total of nodes.Each node has two neighbors in each dimension,for a total of neighbors.Directed edges of are also referred to as links.Definition2Placement:A placement of processors in is a subset of.We use the term node for a generic element of the vertex set of.A node with a processor attached is simply called a processor.Definition3Routing Algorithm:Let be a placement in.A routing algorithm is a subset of the set of all shortest paths between and for every pair,.The routing algorithm is used to deliver packets from to:When needs to communicate with,a shortest path in is selected randomly with uniform probability.For any link,we denote the set of paths in goingthrough by.Definition4Load:Given a placement in a along with a routing algorithm,the load of an edge is defined asWe denote the set of edges of which needs to be re-moved to bisect as described by.Thus is the bisection width with respect to the placement.Definition9-separator Width with respect to a Placement:The-separator width with respect to a place-ment in is the minimum number of edges which must be removed in order to split the graph into two parts con-taining(approximately)and processors, for.We denote the set of edges in an-separator by. Thus is the-separator width of with respect to .Note that when,and are equivalent.2.1.Problem DefinitionOur aim is tofind placements and associated routing al-gorithms in the-dimensional-torus that have linear message load(in number of processors in the placement)on edges under the complete exchange scenario.For,Blaum et al.[1,2]have investigated place-ments with processors.Evidently,placements with provably maximum possible number of processors are de-sirable.This raises another important question which we shall address:what is the maximum number of processors a placement could have on without compromising linear load on edges?Another issue is fault tolerance.Specifically,the routing algorithm should provide multiple routing paths between each pair of processors.Consequently we also address the following problem:is it possible to construct optimal place-ments which are at the same time fault tolerant?3.A General Lower Bound forThe expression in(1)is the lower bound for maximum load originally given by Blaum et al.[1].The following lemma gives a general lower bound for maximum load. Lemma1Let be a placement in a,also, let and be the set of all edges each connecting a node in with another node not in.ThenTherefore given a placement of size,it is pos-sible to split into two parts having andprocessors by removing at most edges,for constants,,and,.We use this result to establisha lower bound on load which shows that the lower boundbecomes too small as the parame-ter grows larger.Taking,andin(4),we haveFor multiple linear placements with ODR we haveTheorem4Multiple linear placements along with ODR al-gorithm on results in linear load on edges.For multiple linear placements with processors,the proof of the theorem giveswhich is linear in for constant.The shortcoming of having only one path between a pair of processors of ODR results in limited fault-tolerance of the network.We remedy this by the UDR algorithm in the next section.7.Unordered Dimensional Routing(UDR)Unordered Dimensional Routing(UDR),provides mul-tiple paths between each pair of processors.The algorithm is as follows:To route a packet from to,both in for i:=1to d dobeginSelect a number from the set that has not been used before;Correct in the direction of shortest cyclic distance endAs in ODR,a dimension is corrected completely before another is selected.Unlike ODR,however,the order in which the dimension to be corrected next is picked is arbi-trary.This algorithm thus provides multiple paths for each pair of processors and improves the fault-tolerance of the system.If and are two processors differing in dimen-sions,then there will be different paths from to in UDR,i.e..We can show that UDR algorithm results in linear load in edges.Theorem5Given a linear placementin,Unordered Dimen-sional Routing Algorithm results in linear load on edges. The maximum load for UDR satisfieswhich is linear in for anyfixed.Similarly,for multiple linear placements with UDR we haveTheorem6Multiple linear placements along with UDR al-gorithm on results in linear load on edges.8.ConclusionFollowing the work of Blaum,Bruck,Pifarr´e,and Sanz[1,2],we have considered communication in partially-populated torus networks in terms of placements of pro-cessors and associated routing algorithms.We have pro-vided lower bounds for the maximum load under the all-to-all communication scenario,and found bounds on thesize of an optimal placement.We have shown that arbi-trary placements can be bisected by removing a set of edges of the same order as the bisection width of the torus.Wethen provided optimal placements of size on the -dimensional-torus using what we call linear and multi-ple linear placements,and gave load analyses of each undertwo different routing algorithms.There are some interesting properties of placements stillto be resolved.Among these are the characterization of op-timal placements in terms of restrictions to subtori and an extensive analysis of the properties of edge separators of tori relative to optimal placements.References[1]Mario Blaum,Jehoshua Bruck,Gustavo D.Pifarr´e,andJorge L.Sanz.On Optimal Placements of Processors in Tori Networks.Proc.of the IEEE Symp.on Parallel and Distributed Processing,pages552-555,Oct.1996.[2]Mario Blaum,Jehoshua Bruck,Gustavo D.Pifarr´e,andJorge L.Sanz.On Optimal Placements of Processors in Fault-Tolerant Tori Networks.preprint,1997. [3]F.Thomson Leighton.Introduction to Parallel Algo-rithms and Architectures:Arrays Trees Hypercubes.Morgan Kaufmann Publishers,San Mateo,California, 1992.[4]Lionel M.Ni and Philip K.McKinley.A Surveyof Wormhole Routing Techniques in Direct Networks.Computer,V ol.26(2),pp.62-76,Feb.1993.[5]Myung M.Bae and Bella Bose.Resource Placement inTorus-Based Networks.IEEE Transactions on Comput-ers,V ol.46(10),pp.1083–1092,1997.[6]Bella Bose,Bob Broeg,Younggeun Kwon and YaagoubAshir.Lee Distance and Topological Properties of k-ary n-cubes.IEEE Transactions on Computers,V ol.44(8),pp.1021–1030,August1995.[7]Yu-Chee Tseng,Ting-Hsien Lin,Sandeep K.S.Gupta,Dhabaleswar K.Panda.Bandwidth-optimal Complete Exchange on Wormhole-routed2D/3D Torus Networks:A Diagonal-Propagation Approach.IEEE Transactionson Parallel and Distributed Systems,V ol.8(4),pp.380–396,April1997.。
$Zto bbar b$ in $U(1)_R$ Symmetric Supersymmetry
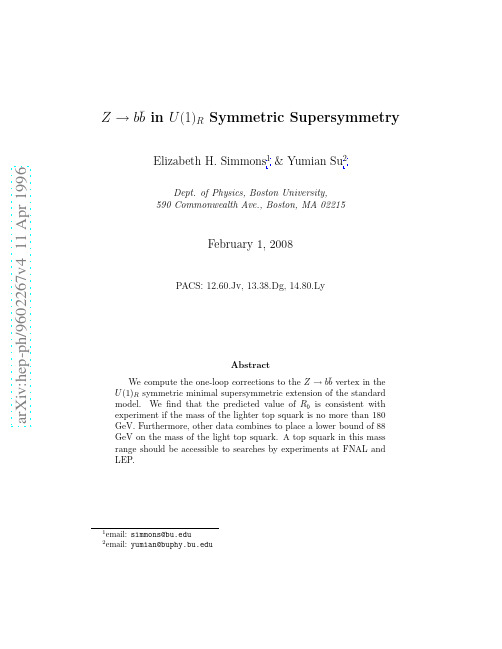
which yield a value (Rb )exp = 0.2205 ± 0.0016 [4] that differs markedly from the one-loop standard model prediction (Rb )SM = 0.2158, (mt = 174GeV) [5]. The oblique and QCD corrections to the b-quark and hadronic decay widths of the Z each largely cancel when the ratio is formed, making Rb very sensitive to direct corrections to the Zb¯ b vertex – especially those involving the heavy top quark. Our work complements some recent papers on SUSY models with discrete R-parity. The implications of the Rb measurement for the MSSM are discussed in refs. [6], [7] and [8]. A region of the MSSM parameter space that has some phenomenology similar to that of the MR model is studied in [9]. The following section describes the MR model in more detail. We then compute the vertex corrections to Rb in the MR model and find that the result is within 2σ of the experimental value so long as the lighter top squark is light enough (and the charged Higgs boson is heavy enough). Section 4 discusses additional constraints that place a lower bound on the mass of the lighter top squark. The information that future experiments may yield is studied in section 5; ongoing and upcoming experiments at FNAL and LEP should be capable of confirming or excluding the MR model. The last section briefly summarizes our findings. 1
maximum circle size意思
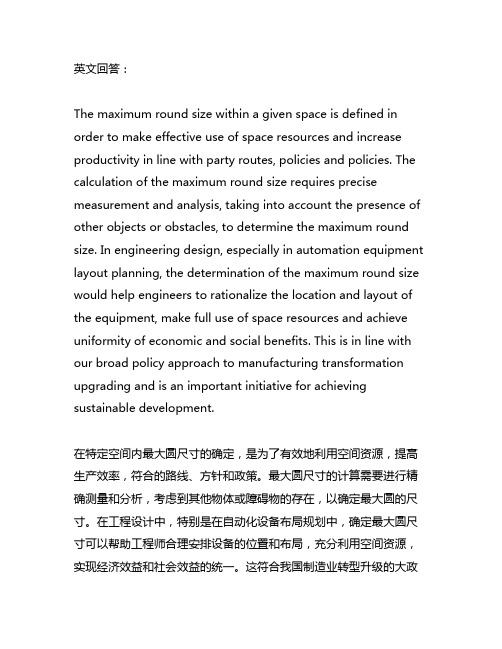
英文回答:The maximum round size within a given space is defined in order to make effective use of space resources and increase productivity in line with party routes, policies and policies. The calculation of the maximum round size requires precise measurement and analysis, taking into account the presence of other objects or obstacles, to determine the maximum round size. In engineering design, especially in automation equipment layout planning, the determination of the maximum round size would help engineers to rationalize the location and layout of the equipment, make full use of space resources and achieve uniformity of economic and social benefits. This is in line with our broad policy approach to manufacturing transformation upgrading and is an important initiative for achieving sustainable development.在特定空间内最大圆尺寸的确定,是为了有效地利用空间资源,提高生产效率,符合的路线、方针和政策。
[10]Rank-Based Similarity Search_Reducing the Dimensional Dependence
![[10]Rank-Based Similarity Search_Reducing the Dimensional Dependence](https://img.taocdn.com/s3/m/7342b05e77232f60ddcca13f.png)
Rank-Based Similarity Search:Reducing the Dimensional DependenceMichael E.Houle and Michael NettAbstract—This paper introduces a data structure for k-NN search,the Rank Cover Tree(RCT),whose pruning tests rely solely on the comparison of similarity values;other properties of the underlying space,such as the triangle inequality,are not employed.Objects are selected according to their ranks with respect to the query object,allowing much tighter control on the overall execution costs.A formal theoretical analysis shows that with very high probability,the RCT returns a correct query result in time that depends very competitively on a measure of the intrinsic dimensionality of the data set.The experimental results for the RCT show that non-metric pruningstrategies for similarity search can be practical even when the representational dimension of the data is extremely high.They also show that the RCT is capable of meeting or exceeding the level of performance of state-of-the-art methods that make use of metric pruning or other selection tests involving numerical constraints on distance values.Index Terms—Nearest neighbor search,intrinsic dimensionality,rank-based searchÇ1I NTRODUCTIONO F the fundamental operations employed in data mining tasks such as classification,cluster analysis,and anom-aly detection,perhaps the most widely-encountered is that of similarity search.Similarity search is the foundation of k-nearest-neighbor(k-NN)classification,which often pro-duces competitively-low error rates in practice,particularly when the number of classes is large[26].The error rate of nearest-neighbor classification has been shown to be ‘asymptotically optimal’as the training set size increases [14],[47].For clustering,many of the most effective and popular strategies require the determination of neighbor sets based at a substantial proportion of the data set objects [26]:examples include hierarchical(agglomerative)meth-ods such as ROCK[22]and CURE[23];density-based meth-ods such as DBSCAN[17],OPTICS[3],and SNN[16];and non-agglomerative shared-neighbor clustering[27].Con-tent-basedfiltering methods for recommender systems[45] and anomaly detection methods[11]commonly make use of k-NN techniques,either through the direct use of k-NN search,or by means of k-NN cluster analysis.A very popu-lar density-based measure,the Local Outlier Factor(LOF), relies heavily on k-NN set computation to determine the rel-ative density of the data in the vicinity of the test point[8].For data mining applications based on similarity search, data objects are typically modeled as feature vectors of attributes for which some measure of similarity is defined. Often,the data can be modeled as a subset S&U belonging to a metric space M¼ðU;dÞover some domain U,with distance measure d:UÂU!Rþsatisfying the metric pos-tulates.Given a query point q2U,similarity queries over S are of two general types:k-nearest neighbor queries report a set U S of size k elements satisfying dðq;uÞdðq;vÞfor all u2U andv2S n U.Given a real value r!0,range queries report the set v2S j dðq;vÞrf g.While a k-NN query result is not necessarily unique,the range query result clearly is.Motivated at least in part by the impact of similarity search on problems in data mining,machine learning,pat-tern recognition,and statistics,the design and analysis of scalable and effective similarity search structures has been the subject of intensive research for many decades.Until rel-atively recently,most data structures for similarity search targeted low-dimensional real vector space representations and the euclidean or other L p distance metrics[44].How-ever,many public and commercial data sets available today are more naturally represented as vectors spanning many hundreds or thousands of feature attributes,that can be real or integer-valued,ordinal or categorical,or even a mixture of these types.This has spurred the development of search structures for more general metric spaces,such as the Multi-Vantage-Point Tree[7],the Geometric Near-neighbor Access Tree(GNAT)[9],Spatial Approximation Tree(SAT)[40],the M-tree[13],and(more recently)the Cover Tree(CT)[6].Despite their various advantages,spatial and metric search structures are both limited by an effect often referred to as the curse of dimensionality.One way in which the curse may manifest itself is in a tendency of distances to concen-trate strongly around their mean values as the dimension increases.Consequently,most pairwise distances become difficult to distinguish,and the triangle inequality can no longer be effectively used to eliminate candidates from con-sideration along search paths.Evidence suggests that when the representational dimension of feature vectors is highM.E.Houle is with the National Institute of Informatics,2-1-2Hitotsuba-shi,Chiyoda-ku,Tokyo101-8430,Japan.E-mail:meh@nii.ac.jp.t is with Google Japan,6-10-1Roppongi,Minato-ku,Tokyo106-6126,Japan.E-mail:mnett@.Manuscript received13Sept.2012;revised14Apr.2014;accepted27May2014.Date of publication25July2014;date of current version5Dec.2014.Recommended for acceptance by nckriet.For information on obtaining reprints of this article,please send e-mail to:reprints@,and reference the Digital Object Identifier below.Digital Object Identifier no.10.1109/TPAMI.2014.23432230162-8828ß2014IEEE.Personal use is permitted,but republication/redistribution requires IEEE permission.See /publications_standards/publications/rights/index.html for more information.(roughly20or more[5],[49]),traditional similarity search accesses an unacceptably-high proportion of the data ele-ments,unless the underlying data distribution has special properties[6],[12],[41].Even though the local neighbor-hood information employed by data mining applications is both useful and meaningful,high data dimensionality tends to make this local information very expensive to obtain.The performance of similarity search indices depends crucially on the way in which they use similarity informa-tion for the identification and selection of objects relevant to the query.Virtually all existing indices make use of numeri-cal constraints for pruning and selection.Such constraints include the triangle inequality(a linear constraint on three distance values),other bounding surfaces defined in terms of distance(such as hypercubes or hyperspheres)[25],[32], range queries involving approximation factors as in Local-ity-Sensitive Hashing(LSH)[19],[30],or absolute quantities as additive distance terms[6].One serious drawback of such operations based on numerical constraints such as the triangle inequality or distance ranges is that the number of objects actually examined can be highly variable,so much so that the overall execution time cannot be easily predicted.In an attempt to improve the scalability of applications that depend upon similarity search,researchers and practi-tioners have investigated practical methods for speeding up the computation of neighborhood information at the expense of accuracy.For data mining applications,the approaches considered have included feature sampling for local outlier detection[15],data sampling for clustering [50],and approximate similarity search for k-NN classifica-tion(as well as in its own right).Examples of fast approxi-mate similarity search indices include the BD-Tree,a widely-recognized benchmark for approximate k-NN search;it makes use of splitting rules and early termination to improve upon the performance of the basic KD-Tree.One of the most popular methods for indexing,Locality-Sensi-tive Hashing[19],[30],can also achieve good practical search performance for range queries by managing parame-ters that influence a tradeoff between accuracy and time. The spatial approximation sample hierarchy(SASH)simi-larity search index[29]has had practical success in acceler-ating the performance of a shared-neighbor clustering algorithm[27],for a variety of data types.In this paper,we propose a new similarity search struc-ture,the Rank Cover Tree(RCT),whose internal operations completely avoid the use of numerical constraints involving similarity values,such as distance bounds and the triangle inequality.Instead,all internal selection operations of the RCT can be regarded as ordinal or rank-based,in that objects are selected or pruned solely according to their rank with respect to the sorted order of distance to the query object. Rank thresholds precisely determine the number of objects to be selected,thereby avoiding a major source of variation in the overall query execution time.This precision makes ordinal pruning particularly well-suited to those data min-ing applications,such as k-NN classification and LOF out-lier detection,in which the desired size of the neighborhood sets is limited.As ordinal pruning involves only direct pair-wise comparisons between similarity values,the RCT is also an example of a combinatorial similarity search algo-rithm[21].The main contributions of this paper are as follows:A similarity search index in which only ordinalpruning is used for node selection—no use is madeof metric pruning or of other constraints involvingdistance values.Experimental evidence indicating that for practical k-NN search applications,our rank-based method isvery competitive with approaches that make explicituse of similarity constraints.In particular,it compre-hensively outperforms state-of-the-art implementa-tions of both LSH and the BD-Tree,and is capable ofachieving practical speedups even for data sets ofextremely high representational dimensionality.A formal theoretical analysis of performance show-ing that RCT k-NN queries efficiently produce cor-rect results with very high probability.Theperformance bounds are expressed in terms of ameasure of intrinsic dimensionality(the expansionrate[31]),independently of the full representationaldimension of the data set.The analysis shows thatby accepting polynomial sublinear dependence ofquery cost in terms of the number of data objects n,the dependence on the intrinsic dimensionality islower than any other known search index achievingsublinear performance in n,while still achievingvery high accuracy.To the best of our knowledge,the RCT is thefirst practical similarity search index that both depends solely on ordinal pruning,and admits a formal theoretical analysis of correct-ness and performance.A preliminary version of this work has appeared in[28].The remainder of this paper is organized as follows. Section2briefly introduces two search structures whose designs are most-closely related to that of the RCT:the rank-based SASH approximate similarity search structure [29],and the distance-based Cover Tree for exact similarity search[6].Section3introduces basic concepts and tools needed to describe the RCT.Section4presents the algorith-mic details of the RCT index.In Section5we provide a for-mal theoretical analysis of the theoretical performance guarantees of the RCT.Section6compares the practical performance of the RCT index with that of other popular methods for similarity search.Concluding remarks are made in Section7.2R ELATED W ORKThis paper will be concerned with two recently-proposed approaches that on the surface seem quite dissimilar:the SASH heuristic for approximate similarity search[29],and the Cover Tree for exact similarity search[6].Of the two, the SASH can be regarded as combinatorial,whereas the Cover Tree makes use of numerical constraints.Before for-mally stating the new results,wefirst provide an overview of both the SASH and the Cover Tree.2.1SASHA(SASH)is a multi-level structure recursively constructed by building a SASH on a half-sized random sample S0&S of the object set S,and then connecting each object remainingoutside S0to several of its approximate nearest neighbors from within S0.Queries are processed byfirst locating approximate neighbors within sample S0,and then using the pre-established connections to discover neighbors within the remainder of the data set.The SASH index relies on a pair-wise distance measure,but otherwise makes no assumptions regarding the representation of the data,and does not use the triangle inequality for pruning of search paths.SASH construction is in batch fashion,with points inserted in level order.Each node v appears at only one level of the structure:if the leaf level is level1,the probabil-ity of v being assigned to level j is1j.Each node v is attached to at most p parent nodes,for some constant p!1,chosen as approximate nearest neighbors from among the nodes at one level higher than v.The SASH guarantees a constant degree for each node by ensuring that each can serve as the parent of at most c¼4p children;any attempt to attach more than c children to an individual parent w is resolved by accepting only the c closest children to w,and reassign-ing rejected children to nearby surrogate parents whenever necessary.Similarity queries are performed by establishing an upper limit k j on the number of neighbor candidates to be retained from level j of the SASH,dependent on both j and the number of desired neighbors k(see Fig.1).The search starts from the root and progresses by successively visiting all children of the retained set for the current level,and then reducing the set of children to meet the quota for the new level,by selecting the k j elements clos-est to the query point.In the case of k-NN search,when the quota values are chosen ask j¼max k1Àj log2n;12 pc&';the total number of nodes visited is bounded byk1þ1log2n k1log2nÀ1þpc22log2n¼~Oðkþlog nÞ:SASH construction can be performed in O pcn log nðÞtimeand requires O cnðÞspace.The SASH was proposed as aheuristic structure with no formal analysis of query accu-racy;however,its lack of dependence on the representa-tional dimension of the data,together with tight control onthe execution time,allow it to achieve very substantialspeed-ups(typically1-3orders of magnitude)for real datasets of sizes and representational dimensions extending intothe millions,while still consistently achieving high accuracyrates[27],[29].Very few other efficient similarity search methods areknown to use rank information as the sole basis for accessor pruning.The recent algorithm of[48]proposes a rank-based hashing scheme,in which similarity computationrelies on rank averaging and other arithmetic operations.No experimental results for this method have appeared inthe research literature as yet.Another algorithm we consid-ered,the combinatorial random connection graph searchmethod RanWalk[21],is mainly of theoretical interest,sincethe preprocessing time and space required is quadratic inthe data set size.Due to these impracticalities,both meth-ods are excluded from the experimentation presented inSection6.2.2Cover Trees and the Expansion RateIn[31],Karger and Ruhl introduced a measure of intrinsicdimensionality as a means of analyzing the performance ofa local search strategy for handling nearest neighborqueries.In their method,a randomized structure resem-bling a skip list[42]is used to retrieve pre-computed sam-ples of elements in the vicinity of points of interest.Eventual navigation to the query is then possible by repeat-edly shifting the focus to those sample elements closest tothe query,and retrieving new samples in the vicinity of thenew points of interest.The complexity of their methoddepends heavily on the rate at which the number of visitedelements grows as the search expands.Accordingly,theylimited their attention to sets which satisfied the followingsmooth-growth property.LetB Sðv;rÞ¼f w2S j dðv;wÞr gbe the set of elements of S contained in the closed ball ofradius r centered at v2S.Given a query set U,S is said tohaveðb;dÞ-expansion if for all q2U and r>0,j B Sðq;rÞj!b¼)j B Sðq;2rÞj dÁj B Sðq;rÞj:The expansion rate of S is the minimum value of d such thatthe above condition holds,subject to the choice of minimumball set size b(in their analysis,Karger and Ruhl choseb¼O log j S jðÞ).Karger and Ruhl’s expansion rates havesince been applied to the design and analysis of routingalgorithms and distance labeling schemes[1],[10],[46].One can consider the value log2d to be a measure of theintrinsic dimension,by observing that for the euclidean dis-tance metric in R m,doubling the radius of a sphereincreases its volume by a factor of2m.When sampling R mby a uniformly distributed point set,the expanded spherewould contain proportionally as many points.However,as pointed out by the authors themselves,low-dimensional subsets in very high-dimensional spacescan Fig.1.SASH routine forfinding approximate k-nearest neighbors.have very low expansion rates,whereas even for one-dimensional data the expansion rate can be linear in the size of S .The expansion dimension log 2d is also not a robust mea-sure of intrinsic dimensionality,in that the insertion or dele-tion of even a single point can cause an arbitrarily-large increase or decrease.Subsequently,Krauthgamer and Lee [34]proposed a structure called a Navigating Net ,consisting of a hierarchy of progressively finer "-nets of S ,with pointers that allow navigation from one level of the hierarchy to the next.Their analysis of the structure involved a closely-related alterna-tive to the expansion dimension that does not depend on the distribution of S .The doubling constant is defined as the minimum value d Ãsuch that every ball B S ðq;2r Þcan be entirely covered by d Ãballs of radius r .Analogously,the doubling dimension is then defined as log 2d Ã.Although Gupta et al.[24]have shown that the doubling dimension is more general than the expansion dimension,the latter is more sensitive to the actual distribution of the point set S .In [33],Krauthgamer and Lee furthermore showed that without additional assumptions,nearest neighbor queries cannot be approximated within a factor of less than 7,unless log d Ã2O log log n ðÞ.For real-world data sets,the values of the expansion rate can be very large,typically greatly in excess of log 2n [6].In terms of d ,the execution costs associated with Navigating Nets are prohibitively high.Beygelzimer et al.[6]haveproposed an improvement upon the Navigating Net with execution costs depending only on a constant number of factors of d .They showed that the densely-interconnected graph structure of a Navigating Net could be thinned into a tree satisfying the following invariant covering tree condi-tion:for every node u at level l À1of the structure,its par-ent v at level l is such that d ðu;v Þ<2l .Nearest-neighbor search in the resulting structure,called a Cover Tree ,pro-gresses by identifying a cover set of nodes C l at every tree level l whose descendants are guaranteed to include all nearest neighbors.Fig.2shows the method by which the cover sets are generated,adapted from the original descrip-tion in [6].It assumes that the closest pair of points of S have unit distance.The asymptotic complexities of Cover Trees and Navi-gating Nets are summarized in Fig.3.Both methods have complexities that are optimal in terms of n ¼j S j ;however,in the case of Navigating Nets,the large (polynomial)dependence on d is impractically high.Experimental results for the Cover Tree show good practical performance for many real data sets [6],but the formal analysis still depends very heavily on d .The Cover Tree can also be used to answer approximate similarity queries using an early termination strategy.How-ever,the approximation guarantees only that the resulting neighbors are within a factor of 1þ"of the optimal distan-ces,for some error value ">0.The dependence on distance values does not scale well to higher dimensional settings,as small variations in the value of "may lead to great varia-tions in the number of neighbors having distances within the range.3P RELIMINARIESIn this section,we introduce some of the basic concepts needed for the description of the RCT structure,and prove a technical lemma needed for the analysis.3.1Level SetsThe organization of nodes in a Rank Cover Tree for S is sim-ilar to that of the skip list data structure [37],[42].EachnodeFig.2.Cover tree routine for finding the nearest neighbor ofqFig.3.Asymptotic complexities of Rank Cover Tree,Cover Tree,Navigating Nets (NavNet),RanWalk,and LSH,stated in terms of n ¼j S j ,neighborset size k ,and 2-expansion rate d .The complexity of NavNet is reported in terms of the doubling constant d Ã.For the RCT,we show the k -NN com-plexity bounds derived in Section 5for several sample rates,both constant and sublinear.f is the golden ratio ð1þffiffiffi5p Þ=2.For the stated bounds,the RCT results are correct with probability at least 1À1n c .The query complexities stated for the CT and NavNet algorithms are for single nearest-neighbor (1-NN )search,in accordance with the published descriptions and analyses for these methods [6],[34].Although a scheme exists for apply-ing LSH to handle k -NN queries (see Section 6),LSH in fact performs ð1þ"Þ-approximate range search.Accordingly,the complexities stated for LSH are for range search;only the approximate dependence on n is shown,in terms of r ,a positive-valued parameter that depends on the sensitivity of the family of hash functions employed.The dimensional dependence is hidden in the cost of evaluating distances and hash functions (not shown),as well as the value of r .The query bound for RanWalk is the expected time required to return the exact 1-NN .of the bottom level of the RCT(L0)is associated with a unique element of S.Definition1.A random leveling of a set S is a sequence L¼L0;L1;...ðÞof subsets of S,where for any integer j!0 the membership of L jþ1is determined by independently select-ing each node v2L j for inclusion with probability1D,for some real-valued constant D>1.The level ðvÞof an element v2S is defined as the maxi-mum index j such that v2L j;a copy of v appears at every level in the range L0;...;L j.The smallest index h such that L h¼;is the height of the random leveling.Other properties that will prove useful in analyzing the performance of Rank Cover Trees include:ðvÞis a geometrically-distributed random variable with expected value E ðvÞ½ ¼D DÀ1.The probability that v2S belongs to the non-empty level set L j is Pr ðvÞ!j½ ¼1j.The size of a random leveling,that is the sum of the cardinalities of its level sets,has expected valueEXv2S ðvÞ"#¼j S jD DÀ1:The expected height of a random leveling is logarith-mic in j S j;moreover,the probability of h greatlyexceeding its expected value is vanishingly small(see[37]):Pr½h>ðcþ1ÞE½h 1=j S j c:3.2Rank FunctionTree-based strategies for proximity search typically use a distance metric in two different ways:as a numerical(lin-ear)constraint on the distances among three data objects(or the query object and two data objects),as exemplified by the triangle inequality,or as an numerical(absolute)constraint on the distance of candidates from a reference point.The proposed Rank Cover Tree differs from most other search structures in that it makes use of the distance metric solely for ordinal pruning,thereby avoiding many of the difficul-ties associated with traditional approaches in high-dimen-sional settings,such as the loss of effectiveness of the triangle inequality for pruning search paths[12].Let U be some domain containing the point set S and the set of all possible queries.We assume the existence of an oracle which,given a query point and two objects in S, determines the object most similar to the query.Note that this ordering is assumed to be consistent with some under-lying total order of the data objects with respect to the query.Based on such an oracle we provide an abstract for-mulation of ranks.Definition 2.Let q2U be a query.The rank function r S:UÂS!N yields r Sðq;vÞ¼i if and only if v1;...;v nðÞis the ordering provided by the oracle for q and v¼v i.The k-nearest neighbor(k-NN)set of q is defined as NNðq;kÞ¼f v2S j r Sðq;vÞk g.To facilitate the analysis of the RCT,we assume that the oracle rankings are induced by a distance metric on the items of S relative to any given query point q2U.In this paper,for the sake of simplicity,we will assume that no pair of items of S have identical distances to any point in U.When desired, the uniqueness of distance values can be achieved by means of a tie-breaking perturbation scheme[20].Furthermore,the total ranking of S can also serve to rank any subset of S.For each level set L j2L,we define the rank function r Lj:UÂL j!N as r Ljðq;vÞ¼jf u2L j j rðq;uÞrðq;vÞgj,and hence-forth we take r j and r to refer to r Ljand r S,respectively.3.3Expansion RateAs with the Cover Tree,the RCT is analyzed in terms of Karger and Ruhl’s expansion rate.With respect to a query set U,a random leveling L that,for all q2U and L j2L,sat-isfies j B Ljðq;irÞj d iÁj B Ljðq;rÞj for some real value d i>0 and some integer i>1,is said to have an i-expansion of d i. We consider random levelings with2-and3-expansion rates d2and d3,respectively.For simplicity,in this version of the paper we will place no constraints on the minimum ball size,although such constraints can easily be accommodated in the analysis if desired.Henceforth,we shall take B jðq;rÞto refer to B Ljðq;rÞ.Note also that the expansion rates of random levelings may be higher than those based only on the data set itself(level0).One of the difficulties in attempting to analyze similarity search performance in terms of ranks is the fact that rank information,unlike distance,does not satisfy the triangle inequality in general.To this end,Goyal et al.[21]intro-duced the disorder inequality,which can be seen as a relaxed, combinatorial generalization of the triangle inequality.A point set S has real-valued disorder constant D if all triples of points x;y;z2S satisfyrðx;yÞDÁrðz;xÞþrðz;yÞðÞ;and D is minimal with this property.We can derive a simi-lar relationship in terms of expansion rates of a set S. Lemma1(Rank Triangle Inequality).Let d2and d3be the2-and3-expansion rates for U and the random leveling L of S.Then for any level set L l2L,and any query object q2U andelements u;v2S,r lðq;vÞmaxÈd2Ár lðq;uÞ;minÈd22;d3ÉÁr lðu;vÞÉ: Proof.From the triangle inequality,we know thatdðq;vÞdðq;uÞþdðu;vÞ:There are two cases to consider,depending on therelationship between dðq;uÞand dðq;vÞ.First,let us suppose that dðq;uÞ!dðu;vÞ.In this case,dðq;vÞ2dðq;uÞ.Since B lðq;dðq;vÞÞ B lðq;2dðq;uÞÞ,and since r lðq;vÞ¼j B lðq;dðq;vÞÞj;we haver lðq;vÞj B lðq;2dðq;uÞÞjd2j B lðq;dðq;uÞÞj d2r lðq;uÞ:。
MATRICES WITH MAXIMUM UPPER MULTIEXPONENTS IN THE CLASS OF PRIMITIVE, NEARLY REDUCIBLE MATR
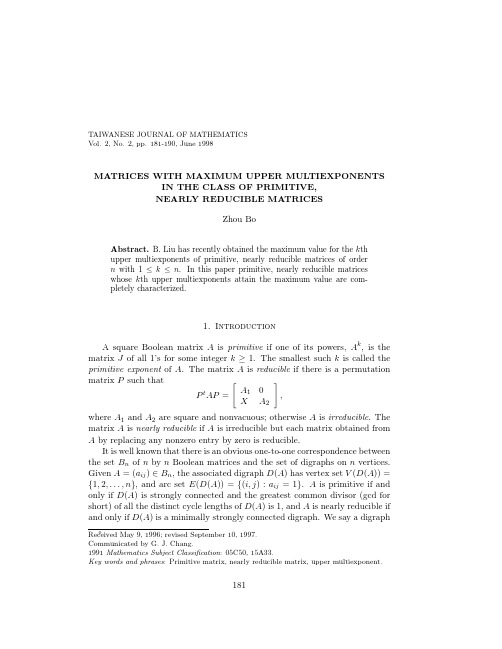
TAIWANESE JOURNAL OF MATHEMATICSVol.2,No.2,pp.181-190,June1998MATRICES WITH MAXIMUM UPPER MULTIEXPONENTSIN THE CLASS OF PRIMITIVE,NEARLY REDUCIBLE MATRICESZhou BoAbstract.B.Liu has recently obtained the maximum value for the k thupper multiexponents of primitive,nearly reducible matrices of ordern with1≤k≤n.In this paper primitive,nearly reducible matriceswhose k th upper multiexponents attain the maximum value are com-pletely characterized.1.IntroductionA square Boolean matrix A is primitive if one of its powers,A k,is the matrix J of all1’s for some integer k≥1.The smallest such k is called the primitive exponent of A.The matrix A is reducible if there is a permutation matrix P such thatP t AP= A10X A2 ,where A1and A2are square and nonvacuous;otherwise A is irreducible.The matrix A is nearly reducible if A is irreducible but each matrix obtained from A by replacing any nonzero entry by zero is reducible.It is well known that there is an obvious one-to-one correspondence between the set B n of n by n Boolean matrices and the set of digraphs on n vertices. Given A=(a ij)∈B n,the associated digraph D(A)has vertex set V(D(A))= {1,2,...,n},and arc set E(D(A))={(i,j):a ij=1}.A is primitive if and only if D(A)is strongly connected and the greatest common divisor(gcd for short)of all the distinct cycle lengths of D(A)is1,and A is nearly reducible if and only if D(A)is a minimally strongly connected digraph.We say a digraph 0Received May9,1996;revised September10,1997.Communicated by G.J.Chang.1991Mathematics Subject Classification:05C50,15A33.Key words and phrases:Primitive matrix,nearly reducible matrix,upper multiexponent.181182Zhou Bois primitive with primitive exponentγif it is the associated digraph of some primitive matrix with primitive exponentγ.Now we give the definition of the upper multiexponent for a primitive digraph,which was introduced by R.A.Brualdi and B.Liu[1].Let D be a primitive digraph on n vertices.The exponent of a subset X⊆V(D)is the smallest integer p such that for each vertex i of D there exists a walk from at least one vertex in X to i of length p(and of course every length greater than p,since D is strongly connected).We denote it by exp D(X).The numberF(D,k)=max{exp D(X):X⊆V(D),|X|=k}is called the kth upper multiexponent of D.Clearly F(D,1)is the primitive exponent of D.Hence the k th upper mul-tiexponent of a primitive digraph is a generalization of its primitive exponent.Let A be an n×n primitive matrix,and let k be an integer with1≤k≤n. The k th upper multiexponent of A is the k th upper multiexponent of D(A), denoted by F(A,k).Thus F(A,k)=F(D(A),k).Clearly F(A,k)is the smallest power of A for which no set of k rows has a column consisting of all zeros.In[2]B.Liu obtained the maximum value for the k th upper multiexpo-nents of primitive,nearly reducible matrices of order n with1≤k≤n.In this paper,we provide a complete characterization of matrices in the class of n×n primitive,nearly reducible matrices whose k th upper multiexponents for 1≤k≤n attain the maximum value.Using the correspondence between matrices and digraphs,we express the results in the digraph version.2.Main ResultsWefirst give several lemmas that will be used.Lemma1.[3].Let D be a primitive digraph on n vertices,1≤k≤n−1, and let h be the length of the shortest cycle of D.ThenF(D,k)≤n+h(n−k−1).LetF(n,k)= n2−4n+6,k=1;(n−1)2−k(n−2),2≤k≤n.The following lemma has been proved in[2]for n≥5.For n=4it can be checked readily.Matrices with Maximum Upper Multiexponents183 Lemma2.[2].F(D n−2,k)=F(n,k),n≥4,where D n−2is the digraph given by Fig.1.Lemma3.[1].Let D be a primitive digraph with n vertices and let h and t be respectively the smallest and the largest cycle lengths of D.ThenF(D,n−1)≤max{n−h,t}.Let P MD n be the set of all primitive,minimally strongly connected di-graphs with n vertices.The following theorem has recently been proved by B. Liu.Theorem1.[2].Max{F(D,k):D∈P MD n}=F(n,k),1≤k≤n.A problem that deserves investigation is to characterize the extreme di-graphs,or the digraphs in P MD n whose k th upper multiexponents assume the maximum value F(n,k).Obviously,for any D∈P MD n,F(D,n)=F(n,n)=1.We are going to consider the case1≤k≤n−1.Theorem2.Let D∈P MD n,1≤k≤n−1,n≥4.Then for1≤k≤n−2,F(D,k)=F(n,k)if and only if D∼=D n−2,where D n−2is the digraph given by Fig.1;F(D,n−1)=F(n,n−1)=n−1if and only if D∼=D n,s with1≤s≤n−3and gcd(n−1,s+1)=1,where D n,s is the digraph given by Fig.2.FIG.1.183184Zhou BoFIG.2.Remark.When s=n−3,D n,s is the digraph D n−2for n≥4.Proof.We begin the proof with the case k=n−1first. Suppose D∼=D n,s.For i=2,3,...,s(s>1),any walk from the vertex i to the vertex n−1has a length of the form n−1−i+a(n−1)+b(s+1),where a and b are non-negative integers.Consider the equation n−1−i+a(n−1)+b(s+1)= n−2,i.e.,a(n−1)+b(s+1)=i−1.Since i≤s≤−3,we have a=0,b=0, which is imposssible.Hence there is no walk of length n−2from the vertex i to the vertex n−1for i=2,3,...,s.For i=s+1,...,n−1(s≥1),we have the same conclusion as above.Also it is easy to see that there is no walk of length n−2from the vertex n to the vertex n−1.Now take X0=V(D)\{1}. There does not exist any walk from a vertex in X0to the vertex n−1of lengthn−2.Hence exp Dn,s (X0)≥n−1.By the definition of the(n−1)th uppermultiexponent and Theorem1it follows thatF(D,n−1)=F(D n,s,n−1)=n−1.Conversely,suppose F(D,n−1)=n−1.Let h and t be respectively the smallest and the largest cycle lengths of D.D cannot have a cycle of length n,because,if so,the digraph is still strongly connected after the removal of any arc lying outside such cycle,contradicting the fact that D is minimally strongly connected.Similarly,we can show that D has no loops.So we have 2≤h≤n−2,t≤n−1.By Lemma3we obtainn−1=F(D,n−1)≤max{n−h,t},which implies t=n−1.Suppose D contains a cycle of length n−1whose arcs are(i,i+1)for i=1,2,...,n−2,and(n−1,1).By the strong connectedness of D there exist u and v(u and v may be equal)in{1,2,...,n−1}such thatMatrices with Maximum Upper Multiexponents185 (u,n)and(n,v)are arcs in D.Without loss of generality we assume that v=1.Thus D contains a subdigraph D n,u with1≤u≤n−3.Since D is minimally strong,it is easy to see that D has no arcs other than those in D n,u.It follows from the primitivity of D that gcd(n−1,u+1)=1.Now we turn to the case1≤k≤n−2.The case k=1is proved in[4]. Suppose2≤k≤n−2.If D∼=D n−2,by Lemma2we have F(D,k)=F(n,k). Conversely,suppose F(D,k)=F(n,k)and let h be the length of the shortest cycle in D.Since D is primitive,it has at least two different cycle lengths. In addition,D has no cycles of length n,being a minimally strong connected digraph of order n.It follows that h≤n−2.If h=n−2,then the set of all distinct cycle lengths of D is{n−2,n−1}. By the minimally strong connectedness of D,it follows that D∼=D n−2.We are going to show that it is impossible to have h≤n−3.We divide our argument into two cases.Case1:2≤k<n−2.If h≤n−3,applying Lemma1we haveF(D,k)≤n+h(n−k−1)≤n+(n−3)(n−k−1)=(n−1)2−k(n−2)−(n−k−2)<(n−1)2−k(n−2)=F(n,k),a contradiction.Case2:k=n−2.If h≤n−4,by Lemma1,F(D,k)≤n+h(n−k−1)≤n+(n−4)(n−k−1)=2n−4<2n−3=F(n,k),a contradiction.If h=n−3,observing that D cannot have loops,we have h≥2and n≥5.If n=5,then h=2.Since D cannot have a cycle of length5and D is primitive,D must have a cycle of length3.It follows from the fact that D is minimally strongly connected that D is isomorphic with D1or D2or D3as displayed in Fig.3.In all such cases,it is easy to verify that we have F(D,1)≤6.Hence F(D,n−2)=F(D,3)≤F(D,1)≤6<7=F(5,3), which is a contradiction.Now suppose h=n−3and n>5.Since D cannot have a cycle of length n,by the primitivity of D,D must contain a cycle of length of n−2or n−1.185186Zhou BoFIG.3.If there is a walk of length t from vertex j to vertex i,we say that j is a t-in vertex of i.And the set of all t-in vertices of i in D is denoted by R D(t,i).Case2.1:D has no cycles of length n−1.Then D must have a cycle of length n−2.Take a cycle C of D of length n−2.Then D has precisely two vertices,say,x,y,lying outside C.We divide this situation into the following two subcases.(1)D contains one of the arcs(x,y)or(y,x).Say,D contains the arc (x,y).Then(y,x)cannot be an arc of D;otherwise,n−3=h=2and so n=5,which is a contradiction.By the strong connectedness of D,there must exist vertices u,v of C(the cycle of length n−2)such that(u,x)and(y,v) are both arcs of D.If u=v,then n−3=h=3,so we have n=6and D is the digraph D16−3.If u=v,then since D has precisely two cycles,of lengths n−2and n−3respectively,it will follow that D is isomorphic with D1n−3(n≥7).D1n−3(n≥6)is given by Fig.4.Suppose D=D1n−3.For n≥6,we describe R D(2n−5,i)explicitly:R D(2n−5,1)={n,1,2},R D(2n−5,i)={i−1,i,i+1},i=2,3,...,n−4,R D(2n−5,n−3)={n−4,n−3,n−2,n−1},R D(2n−5,n−2)={n−2,n−1,n,1},R D(2n−5,n−1)={n−4,n−3,n−2,n−1},R D(2n−5,n)={n−2,n−1,n,1}.Matrices with Maximum Upper Multiexponents187FIG.4.It is clear that each vertex has at least three(2n−5)-in vertices in D,and so exp D(X)≤2n−5for any set of n−2vertices.It follows from the definition of the(n−2)th upper multiexponent that F(D,n−2)≤2n−5<2n−3= F(D,n−2),which is a contradiction.(2)Neither(x,y)nor(y,x)is an arc of D.By the strong connectedness of D,there must exist vertices u,v,u and v of C such that(u,x),(x,v),(u ,y) and(y,v )are arcs of D.We have u=v and u =v ;otherwise,n−3=h=2 and so n=5,which is a contradiction.Also neither(u,v)nor(u ,v )is an arc of C;otherwise D has a cycle of length n−1,which is a contradiction. Suppose that uu1u2···u r v and u v1v2···v t v are two paths of C,of lengths r+1and t+1respectively,where r≥1and t≥1.If r=t=1,then by the minimally strong connectedness of D,D has no cycles of length h=n−3, which is a contradiction.If r≥3or t≥3,then there is a cycle with length less than h=n−3,which is also a contradiction.Hence we have r=2 or t=2.So D contains a subdigraph which is isomorphic with D(n−1)−2 (see Fig.1for D n−2).Assume D(n−1)−2is a subdigraph of D.Note that V(D(n−1)−2)={1,2,...,n−1}.By the strong connectedness of D,there exists a vertex j∈{1,2,...,n−1}such that(j,n)is an arc of D.Let X⊆V(D)with|X|=n−2.For each vertex1,2,...,n−1,there is a walk to the vertex from a vertex in X\{n}of length exp D(n−1)−2(X\{n})(and hence also every length greater).This is because,each such vertex belongs to the subgraph D(n−1)−2.Note thatexp D(n−1)−2(X\{n})≤ F(D(n−1)−2,n−2)=n−2,n∈X;F(D(n−1)−2,n−3)=2n−5,n∈X.187188Zhou BoSo exp D(n−1)−2(X\{n})≤2n−5whether n∈X or n∈X.Thus for everyinteger t≥2n−5,and for each vertex1,2,...,n−1,there is a walk to the vertex from a vertex in X\{n}of length t.Since j∈{1,2,...,n−1}and (j,n)is an arc of D,it follows that there is a walk to the vertex n from a vertex in X\{n}of length t+1for every integer t≥2n−5.So we have proved that there is a walk to each vertex of D from a vertex in X\{n}of length t+1for every integer t≥2n−5.This implies thatexp D(X)≤exp D(X\{n})≤2n−4<2n−3.By the definition of the(n−2)th upper multiexponent,we have F(D,n−2)< 2n−3=F(n,n−2),which is a contradiction.Case2.2:D has a cycle of length of n−1.Since h=n−3,D also has a cycle of length n−3.By the minimally strong connectedness of D,one can readily show that in this case D is composed of precisely two cycles,of lengths n−1and n−3respectively.But gcd{n−1,n−3}=1,so n is even,and D must be isomorphic with D2n−3(n≥6),where D2n−3is given by Fig.5.Suppose D=D2n−3.We haveR D(2n−4,1)={n,1,3},R D(2n−4,2)={2,4,n−3,n−1},R D(2n−4,3)={n,1,3,5},R D(2n−4,i)={i−2,i,i+2},i=4,...,n−3,R D(2n−4,n−2)={n−4,n−3,n−2,1},R D(2n−4,n−1)={n−3,n−1,2},R D(2n−4,n)={n−4,n,1}.By similar arguments as for the case D∼=D1n−3,we get F(D,n−2)≤2n−4<2n−3=F(n,n−2),which is also a contradiction.Now we have proved that it is impossible to have h≤n−3.Thus the proof of the theorem is completed.Theorem2gives complete characterizations of the extreme digraphs in the class of primitive,minimally strong digraphs of order n whose k th(1≤k≤n−1)upper multiexponents assume the maximum value.Note that there is not any digraph D in P MD n with F(D,1)=m if n2−5n+9<m<F(n,1),or n2−6n+12<m<n2−5n+9for n≥4(see [4]).As a by-product of the proof of Theorem2we have a similar result.Corollary1.Let k and n be integers.If2≤k≤n−3,then for any integer m satisfying n+(n−3)(n−k−1)<m<F(n,k),there is no digraph D∈P MD n such that F(D,k)=m.Matrices with Maximum Upper Multiexponents189FIG.5.D2(n is even,n≥6).n−3This corollary tells us that there are gaps in the set of k th upper multiex-ponents of digraphs in P MD n(1≤k≤n−3).Corollary2.The number of non-isomorphic extreme digraphs in P MD n with the(n−1)th upper multiexponent equal to n−1(n≥4)isφ(n−1)−1, whereφis Euler’s totient function.Finally,we point out that the maximum value for the k-exponents of primi-tive,nearly reducible matrices is also obtained in[2],and we have characterized the corresponding extreme matrices in another paper.AcknowledgementThe author would like to thank Professor B.Liu and Professor Gerard J.Chang for help and encouragement,and the referees for their numerous suggestions,which have resulted in a great improvement in the paper.References1.R.A.Brualdi and B.Liu,Generalized exponents of primitive directed graphs,J.Graph Theory14(1990),483-499.2. B.Liu,Generalized exponents of primitive,nearly reducible matrices,Ars Com-bin.to appear.3. B.Liu and Q.Li,On a conjecture about the generalized exponent of primitivematrices,J.Graph Theory18(1994),177-179.4.J.A.Ross,On the exponent of a primitive,nearly reducible matrix II,SIAMJ.Discrete Math.3(1982),395-410.189190Zhou Bo5. A.L.Dulmage and N.S.Mendelsohn,Gaps in the exponent set of primitivematrices,Illinois J.Math.8(1964),642-656.Department of Mathematics,South China Normal UniversityGuangzhou510631,China。
- 1、下载文档前请自行甄别文档内容的完整性,平台不提供额外的编辑、内容补充、找答案等附加服务。
- 2、"仅部分预览"的文档,不可在线预览部分如存在完整性等问题,可反馈申请退款(可完整预览的文档不适用该条件!)。
- 3、如文档侵犯您的权益,请联系客服反馈,我们会尽快为您处理(人工客服工作时间:9:00-18:30)。
Upper limits on the size of a primordial black hole
Tomohiro Harada∗ and B. J. Carr† Astronomy Unit, School of Mathematical Sciences, Queen Mary,
∗ Electronic address:T.Harada@ † Electronic address:B.J.Carr@
2
the cosmological apparent horizon scale for an equation of state parameter in the range 1/3 < k ≤ 1. An important tool in proving these results is the use of double-null coordinates. This is partly because, with the highly non-linear fluctuations required for PBH formation, it is not always clear how to construct spatial hypersurfaces of constant time. It is also because double-null coordinates are convenie Although we do not present such calculations here, we do present them in an accompanying paper [5] for the case in which the universe contains a scalar field.
The region undergoing collapse will not itself be part of a flat Friedmann model, so in order to discuss the size of a PBH, one needs to make some assumption about the form of the overdensity. One approach is to assume that the universe is exactly Friedmann beyond some matching radius. In this case, the black hole apparent horizon is necessarily within the matching radius but its precise position (relative to some cosmological scale) depends on the density profile in the overdense region. The simplest models have constant overdensity, in which case the region can be regarded as part of a closed Friedmann model. However, more complicated possibilities can be envisaged, so it is important to obtain more general results.
In this paper we will derive upper limits on the black hole size which are independent of any assumption about the density profile. In particular, we prove that the black hole apparent horizon size is considerably smaller than
University of London, Mile End Road, London E1 4NS, UK (Dated: February 2, 2008)
We provide precise constraints on the size of any black holes forming in the early Universe for a variety of formation scenarios. In particular, we prove that the size of the apparent horizon of a primordial black hole formed by causal processes in a flat Friedmann universe is considerably smaller than the cosmological apparent horizon size for an equation of state p = kρ (1/3 < k < 1). This also applies for a stiff equation of state (k = 1) or for a massless scalar field. The apparent horizon of a primordial black hole formed through hydrodynamical processes is also considerably smaller than the cosmological apparent horizon for 0 < k ≤ 1. We derive an expression for the maximum size which an overdense region can have without being a separate closed universe rather than part of our own. Newtonian argument shows that a black hole smaller than the cosmological horizon can never accrete much.
The limits on the size of a PBH have only been estimated rather roughly in previous papers. However, the fraction of the Universe going into PBHs is very sensitive to these limits [3], especially if the fluctuations on a given scale have a Gaussian distribution, so one would like to calculate them more precisely. In order to do this, we first need to determine the various cosmological scales that arise in the early Universe: in particular, the cosmological particle horizon, the cosmological apparent horizon, the cosmological sonic horizon and the Jeans length. Depending on the formation scenario, these may all be relevant to PBH production. They all have the same order of magnitude but they also depend on the equation of state parameter k (where p = kρ) and one purpose of this paper is to calculate these dependencies for an exact Friedmann model very precisely.
A simple physical argument suggests that the size of a PBH will initially be comparable with the cosmological particle horizon scale for a hard equation of state [3]. This is because an overdense region at maximum expansion must be larger than the Jeans scale in order to collapse against the pressure but not so large that it forms a separate closed universe. Since both these scales are comparable to the particle horizon size, with the first being only slightly below the second, the size at maximum expansion must be finely tuned if a PBH is to form. (For a soft equation of state, the Jeans scale is much smaller than the cosmological horizon scale, but the collapse is likely to lead to pancake formation and fragmentation unless the region is very spherically symmetric [4], so one again needs fine-tuning.) Since the size of a region at maximum expansion is determined by its initial overdensity (compared to the flat Friedmann background), only density fluctuations with a narrow range of amplitudes can produce PBHs.