车牌识别英文文献1翻译
车牌倾斜校正 英文原文及翻译
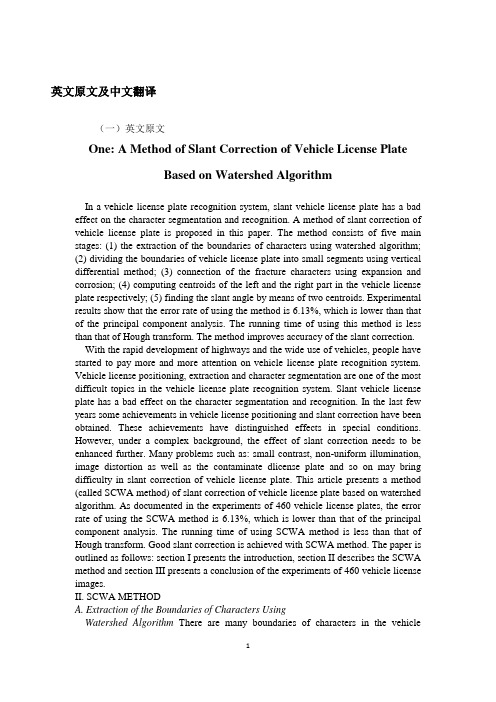
英文原文及中文翻译(一)英文原文One: A Method of Slant Correction of Vehicle License PlateBased on Watershed AlgorithmIn a vehicle license plate recognition system, slant vehicle license plate has a bad effect on the character segmentation and recognition. A method of slant correction of vehicle license plate is proposed in this paper. The method consists of five main stages: (1) the extraction of the boundaries of characters using watershed algorithm;(2) dividing the boundaries of vehicle license plate into small segments using verticaldifferential method; (3) connection of the fracture characters using expansion and corrosion; (4) computing centroids of the left and the right part in the vehicle license plate respectively; (5) finding the slant angle by means of two centroids. Experimental results show that the error rate of using the method is 6.13%, which is lower than that of the principal component analysis. The running time of using this method is less than that of Hough transform. The method improves accuracy of the slant correction.With the rapid development of highways and the wide use of vehicles, people have started to pay more and more attention on vehicle license plate recognition system.Vehicle license positioning, extraction and character segmentation are one of the most difficult topics in the vehicle license plate recognition system. Slant vehicle license plate has a bad effect on the character segmentation and recognition. In the last few years some achievements in vehicle license positioning and slant correction have been obtained. These achievements have distinguished effects in special conditions.However, under a complex background, the effect of slant correction needs to be enhanced further. Many problems such as: small contrast, non-uniform illumination, image distortion as well as the contaminate dlicense plate and so on may bring difficulty in slant correction of vehicle license plate. This article presents a method (called SCWA method) of slant correction of vehicle license plate based on watershed algorithm. As documented in the experiments of 460 vehicle license plates, the error rate of using the SCWA method is 6.13%, which is lower than that of the principal component analysis. The running time of using SCWA method is less than that of Hough transform. Good slant correction is achieved with SCWA method. The paper is outlined as follows: section I presents the introduction, section II describes the SCWA method and section III presents a conclusion of the experiments of 460 vehicle license images.II. SCWA METHODA. Extraction of the Boundaries of Characters UsingWatershed Algorithm There are many boundaries of characters in the vehiclelicense plate. These characters are very important to slant correction of vehicle license plate.The steps of extraction of the boundaries of characters are as follow:1) Produce gradient image The watershed algorithm is sensitive to noise and has excessive segmentation. In order to avoid these problems,we apply prewitt operator to produce gradient image of vehicle license.The prewitt operator is as follow:where H1 is x direction border, H2 is y direction border, gradient magnitude is:Watershed segmentation on gradient imageThe gradient magnitude of the gradient image of the vehicle license plate is considered as a topographic surface for the watershed transformation. The idea of watershed segmentation can be viewed as a landscape immersed in a lake; catchment basins will be filled up with water starting at each local minimum. Dams must be built in order to avoid the merging of catchment basins. The water shed lines are defined by the catchment basins divided by the dam at the highest level. As a result, watershed lines can separate individual catchment basins in the landscape. The result of watershed segmentation is shown in Figure 1. The watershed segmentation is as follow: Assume that G is a simple connected graph, the distance between pixel x and pixel y in G graph is the minimal route from pixel x to pixel y, min ( ) h I refers to minimal gradient magnitude in license image I when the altitude is h, hmin and hmax denote minimum and maximum in gradient magnitude domain DI respectively, h value changes from hmin to hmax.Watershed segmentation orders gradient magnitudes according to increase and then scans from hmin to hmax according to width preferential algorithm.Step 1. These pixels whose gradient magnitude is h are marked with a flag sign. The pixels which are marked with a flag sign are put into first-in-first-out queue.Step 2. A pixel P is got from the queue. Assume that P’ around pixel P is the same flag region as P. P’ and P are merged if the distance between P’ and P is smaller than the current distance.Step 3. P' is put into first-in-first-out queue if the distance between P' and the marked regions is not computed. P' distance is that the current distance adds 1.Step 4. The current distance adds 1 when the computation of current distance has finished.Step 5. Go to step 2 if the queue is not empty.Step 6. Sign a new mark for these pixels which are not handled from step 2 to step 4 and which are min ( ) h I .B. Dividing the Boundaries of Vehicle License Plate into Small Segments UsingVertical Differential Method Respecting the more intensive density of the verticaledge than the level edge of vehicle license plate region and the regular characteristics of characters spacing of vehicle license plate, we divide the boundaries of vehicle license plate into small segments using vertical differential method(shown in Fig.2).where I(i,j) is a matrix of the vehicle license plate image, G is a border matrix.C. Connection of the Fracture Characters Using Expansion and Corrosion Operation The boundaries of vehicle license plate are divided into small segments using the vertical differential method(shown in Fig. 2). The white area of less than 10 points is set to background-color in order to eliminate the boundaries of vehicle license plate. The fracture characters are connected by using expansion and corrosion operation. The erosion operation is defined as:The expansion operation is defined as:where I is a matrix of the vehicle license plate image, B is structuring element set. D. Computing Ccentroids of the Left and the Right Partin the Vehicle License Plate RespectivelyAssume that I is an image of vehicle license plate which contains m×n pixels, Sum_x1 and Sum_y1 is the sum of X coordinate value and Y coordinate value of the white pixel of left part in the image I respectively, Sum_x2 and Sum_y2 is the sum of X coordinate value and Y coordinate value of the white pixel of right part in the image I respectively.Assume that num1 and num2 is the number of pixels ofthe left and right part in the image I respectively, (centX1,centY1) and (centX2,centY2) is the centroids of the left part and the right part in the image I respectively.E. Finding the Slant Angle by Means of Two CentroidsThe connection of two centroids constitutes a main axes of the license plate. The angle between the main axes and the horizontal is θ(shown in Fig. 3).The angle of θ of counterclockwise rotation is:The transformation matrix of counter-clockwise rotation is:The angle of θ of clockwise rotation is:The result of slant correction of vehicle license plate is shown in Figure 4.Figure 3. The angle between the main axis of License plates and horizontal line. (a)angle of θ of counterclockwise rotation;(b) the angle of θ of clockwise rotation.Figure 4. Slant correction of vehicle license plateIII. CONCLUSIONSFor testing the MWF algorithm, the experiment of 460vehicle license plate images is carried on. The error rate of slant correction of vehicle license plate using the different methods is 6.13% (SCWA method) and 10.25% (PCA method). Comparison of the results of SCWA method and PCA method is shown in Figure 5.The conclusion is that the SCWA method is more effective than the PCA method. The running time using this method is less than that one of Hough transform. Our future work will be to test rigorously the SCWA method over a wide variety of images and improve further accuracy of the slant correction of vehicle license.Figure5. Comparison of the results of SCWA method and PCA method. (a) the original Slant Vehicle License Plate; (b) slant correction of vehicle license plate using PCA method. (c) slant correction of vehicle license plate using SCWA method. Two:A Method of Slant Correction of Vehicle License PlateBased on Hough Transform and Mathematics MorphologyIn a real Vehicle License Plate Recognition System, the license images obtained by vidicon are usually slantwise. The slant of vehicle licenses will do harm to the Character Segment and Recognition. The paper advances a new method combining Hough Transform and Mathematics Morphology by the analysis of the vehicle licenses’ slant pattern and the interference characteristics. Compared with the conventional methods, it overcomes the perplexity that too many disturbed lines and imperfect detection criterions. The experimental results show that the proposed method can improve the accuracy of the slant correction. It is confirmed that the noise immunity of the method is excellent, and the performance is robust. The correctionrate of the newly developed algorithm has reached over 95%.The typical steps involved in a video-based Vehicle License Plate Recognition System are Obtaining Image, Plate Location, Character Segment and Character Recognition. The obtained license image is usually slantwise and not a normal rectangle because of the CCD vidicon’s perspective warps. The slant of Vehicle Licenses will do harm to the Character Segment and Recognition, and it will affect the accuracy and reliability of the whole system. Therefore, it is necessary to do slant correction before character recognition. According to the analysis, there are several characteristics of the slant license image. The information comprised in the image is complex, and quite a number of information is the interference. The slant of the license mainly reflects on the horizontal warp. At present, the existing researches in Slant Correction have been developed on the basis of Hough Transform. Hough Transform can detect the plate’s frame lines, obtain the incline information and realize the correction. (1) Combining with Edge Detection, viz. doing edge detection firstly before Hough Transform processing. This method is liable to infection by the non-frame lines, and the veracity is not good. (2) The Longest Line Detection method (Yen, 1995). Its idea in nature is detecting the slant angle of the longest straight line to correct the plate. This method demands a high integrality of the frame lines. However, the plates in real can hardly satisfy the demands on account of the external disturbance, and the effect is also not good. This paper proposes a new approach combining Hough Transform and Mathematics Morphology. The steps for slant correction can be summed up as the following: At first, binarize the image of the vehicle license, than using Mathematics Morphology methods to exact the framework of it; Then, do erosion operation to filter the portrait lines which interfere with the slant correction; At last, use Hough Transform and knowledge reasoning to detect the transverse parallel lines, reckon the slant angle of the vehicle license, and design the rotation algorithm adapted for the situation that the rotated information region will become larger.Available Lines Picking-up based on Mathematics MorphologyThe straight line detection using the method of Hough Transform is subject to interference from non-straight line information. Therefore, Mathematics Morphology is employed to pick up the available lines in advance.Image ThinningGenerally speaking, image thinning is getting rid of some points in the original image but holding the former shape of the objective region. Thinning is the variant of the erosion manipulation in nature. The course of t hinning is to decide a point’s remove-or-reserve according to its 8 neighborhood points continually.Image ErosionBecause the longitudinal lines in the thinned image will interfere to the extraction of the available slantwise information, the erosion manipulation is applied and the structure elementG=[0]1×n =[g1, g2, ……, g n] gi=0, i=1, ……, nis chosen. It is considered that the width of the thinned framework is single element, and the detected lines are longer and parallel. If the chosen value of n in formula (4) is big, the framework lines might be eroded. Therefore, the 1×3 horizontal structure element is selected. The discrimination rule is: The current point will be eroded in the case of that there is one background point in the three (itself, its former point and its after point).Slant Information Extracting and Slant CorrectionHough Transform is an important method to detect and describe the linetype object, and the accuracy is quite high. It can be used to detect the lines in the license image which is thinned and eroded, and then gain the incline information then we can correct it to use traditional Hough Transform which we are so familiar with.(二)中文翻译一:基于分水岭式算法的车牌图像倾斜校正在车辆牌照自动识别系统中,车牌倾斜对车牌的分割和识别有很大的影响。
车牌识别外文文献翻译中英文

外文文献翻译(含:英文原文及中文译文)文献出处:Gao Q, Wang X, Xie G. License Plate Recognition Based On Prior Knowledge[C]// IEEE International Conference on Automation and Logistics. IEEE, 2007:2964-2968.英文原文License Plate Recognition Based On Prior KnowledgeQian Gao, Xinnian Wang and Gongfu XieAbstract - In this paper, a new algorithm based on improved BP (back propagation) neural network for Chinese vehicle license plate recognition (LPR) is described. The proposed approach provides a solution for the vehicle license plates (VLP) which were degraded severely. What it remarkably differs from the traditional methods is the application of prior knowledge of license plate to the procedure of location, segmentation and recognition. Color collocation is used to locate the license plate in the image. Dimensions of each character are constant, which is used to segment the character of VLPs. The Layout of the Chinese VLP is an important feature, which is used to construct a classifier for recognizing. The experimental results show that the improved algorithm is effective under the condition that the license plates were degraded severely.Index Terms - License plate recognition, prior knowledge, vehiclelicense plates, neural network.I. INTRODUCTIONV ehicle License-Plate (VLP) recognition is a very interesting but difficult problem. It is important in a number of applications such as weight-and-speed-limit, red traffic infringement, road surveys and park security [1]. VLP recognition system consists of the plate location, the characters segmentation, and the characters recognition. These tasks become more sophisticated when dealing with plate images taken in various inclined angles or under various lighting, weather condition and cleanliness of the plate. Because this problem is usually used in real-time systems, it requires not only accuracy but also fast processing. Most existing VLP recognition methods [2], [3], [4], [5] reduce the complexity and increase the recognition rate by using some specific features of local VLPs and establishing some constrains on the position, distance from the camera to vehicles, and the inclined angles. In addition, neural network was used to increase the recognition rate [6], [7] but the traditional recognition methods seldom consider the prior knowledge of the local VLPs. In this paper, we proposed a new improved learning method of BP algorithm based on specific features of Chinese VLPs. The proposed algorithm overcomes the low speed convergence of BP neural network [8] and remarkable increases the recognition rate especially under the condition that the license plate images were degrade severely.II. SPECIFIC FEA TURES OF CHINESE VLPSA. DimensionsAccording to the guideline for vehicle inspection [9], all license plates must be rectangular and have the dimensions and have all 7 characters written in a single line. Under practical environments, the distance from the camera to vehicles and the inclined angles are constant, so all characters of the license plate have a fixed width, and the distance between the medium axes of two adjoining characters is fixed and the ratio between width and height is nearly constant. Those features can be used to locate the plate and segment the individual character. B. Color collocation of the plateThere are four kinds of color collocation for the Chinese vehicle license plate .These color collocations are shown in table I.TABLE IMoreover, military vehicle and police wagon plates contain a red character which belongs to a specific character set. This feature can be used to improve the recognition rate.C. Layout of the Chinese VLPSThe criterion of the vehicle license plate defines the characters layout of Chinese license plate. All standard license plates contain Chinese characters, numbers and letters which are shown in Fig.1. The first one is a Chinese character which is an abbreviation of Chineseprovinces. The second one is a letter ranging from A to Z except the letter I. The third and fourth ones are letters or numbers. The fifth to seventh ones are numbers ranging from 0 to 9 only. However the first or the seventh ones may be red characters in special plates (as shown in Fig.1). After segmentation process the individual character is extracted. Taking advantage of the layout and color collocation prior knowledge, the individual character will enter one of the classes: abbreviations of Chinese provinces set, letters set, letters or numbers set, number set, special characters set.(a)Typical layout(b) Special characterFig.1 The layout of the Chinese license plateIII. THE PROPOSED ALGORITHMThis algorithm consists of four modules: VLP location, character segmentation, character classification and character recognition. The main steps of the flowchart of LPR system are shown in Fig. 2.Firstly the license plate is located in an input image and characters are segmented. Then every individual character image enters the classifier to decide which class it belongs to, and finally the BP network decides which character the character image represents.A. Preprocessing the license plate1) VLP LocationThis process sufficiently utilizes the color feature such as color collocation, color centers and distribution in the plate region, which are described in section II. These color features can be used to eliminate the disturbance o f the fake plate ’ s regions. The flowchart of the plate location is shown in Fig. 3.Fig.3 The flowchart of the plate location algorithmThe regions which structure and texture similar to the vehicle plate are extracted. The process is described as followed:Here, the Gaussian variance is set to be less than W/3 (W is the character stroke width), so 1P gets its maximum value M at the center of the stroke. After convolution, binarization is performed according to a threshold which equals T * M (T<0.5). Median filter is used to preserve the edge gradient and eliminate isolated noise of the binary image. An N * N rectangle median filter is set, and N represents the odd integer mostly close to W.Morphology closing operation can be used to extract the candidate region. The confidence degree of candidate region for being a license plate is verified according to the aspect ratio and areas. Here, the aspect ratio is set between 1.5 and 4 for the reason of inclination. The prior knowledge of color collocation is used to locate plate region exactly. The locating process of the license plate is shown in Fig. 4.2) Character segmentationThis part presents an algorithm for character segmentation based on prior knowledge, using character width, fixed number of characters, the ratio of height to width of a character, and so on. The flowchart of the character segmentation is shown in Fig. 5.Firstly, preprocess the license the plate image, such as uneven illumination correction, contrast enhancement, incline correction and edge enhancement operations; secondly, eliminating space mark which appears between the second character and the third character; thirdly, merging the segmented fragments of the characters. In China, all standard license plates contain only 7 characters (see Fig. 1). If the number of segmented characters is larger than seven, the merging process must be performed. Table II shows the merging process. Finally, extracting the individual character’ image based on the number and the width of the character. Fig. 6 shows the segmentation results. (a) The incline and broken plate image, (b) the incline and distort plate image, (c)the serious fade plate image, (d) the smut license plate image.where Nf is the number of character segments, MaxF is the number of the license plate, and i is the index of each character segment.The medium point of each segmented character is determined by:(3)where 1i Sis the initial coordinates for the character segment, and 2i S is thefinal coordinate for the character segment. The d istance between two consecutive medium points is calculated by:(4)Fig.6 The segmentation resultsB. Using specific prior knowledge for recognitionThe layout of the Chinese VLP is an important feature (as described in the section II), which can be used to construct a classifier for recognizing. The recognizing procedure adopted conjugate gradient descent fast learning method, which is an improved learning method of BP neural network[10]. Conjugate gradient descent, which employs a series of line searches in weight or parameter space. One picks the first descent direction and moves along that direction until the minimum in error is reached. The second descent direction is then computed: this direction the “ conjugate direction” is the one along which the gr adient does not change its direction will not “ spoil ” the contribution from the previous descent iterations. This algorithm adopted topology 625-35-N as shown in Fig. 7. The size of input value is 625 (25*25 ) and initial weights are with random values, desired output values have the same feature with the input values.As Fig. 7 shows, there is a three-layer network which contains working signal feed forward operation and reverse propagation of error processes. The target parameter is t and the length of network outputvectors is n. Sigmoid is the nonlinear transfer function, weights are initialized with random values, and changed in a direction that will reduce the errors.The algorithm was trained with 1000 images of different background and illumination most of which were degrade severely. After preprocessing process, the individual characters are stored. All characters used for training and testing have the same size (25*25 ).The integrated process for license plate recognition consists of the following steps:1) Feature extractingThe feature vectors from separated character images have direct effects on the recognition rate. Many methods can be used to extract feature of the image samples, e.g. statistics of data at vertical direction, edge and shape, framework and all pixels values. Based on extensive experiments, all pixels values method is used to construct feature vectors. Each character was reshaped into a column of 625 rows’ feature vector. These feature vectors are divided into two categories which can be used for training process and testing process.2) Training modelThe layout of the Chinese VLP is an important feature, which can be used to construct a classifier for training, so five categories are divided. The training process of numbers is shown in Fig. 8.As Fig. 8 shows, firstly the classifier decides the class of the inputfeature vector, and then the feature vector enters the neural network correspondingly. After the training process the optimum parameters of the net are stored for recognition. The training and testing process is summarized in Fig. 9.(a) Training process(b)Testing processFig.9 The recognition process3) Recognizing modelAfter training process there are five nets which were completely trained and the optimum parameters were stored. The untrained feature vectors are used to test the net, the performance of the recognition system is shown in Table III. The license plate recognition system is characterized by the recognition rate which is defined by equation (5).Recognition rate =(number of correctly read characters)/ (number of found characters) (5)IV. COMPARISON OF THE RECOGNITION RA TE WITH OTHER METHODSIn order to evaluate the proposed algorithm, two groups of experiments were conducted. One group is to compare the proposed method with the BP based recognition method [11]. The result is shown in table IV. The other group is to compare the proposed method with themethod based on SVM [12].The result is shown in table V. The same training and test data set are used. The comparison results show that the proposed method performs better than the BP neural network and SVM counterpart.V. CONCLUSIONIn this paper, we adopt a new improved learning method of BP algorithm based on specific features of Chinese VLPs. Color collocation and dimension are used in the preprocessing procedure, which makes location and segmentation more accurate. The Layout of the Chinese VLP is an important feature, which is used to construct a classifier for recognizing and makes the system performs well on scratch and inclined plate images. Experimental results show that the proposed method reduces the error rate and consumes less time. However, it still has a few errors when dealing with specially bad quality plates and characters similar to others. This often takes place among these characters (especially letter and number): 3—8 4—A 8—B D—0.In order to improve the incorrect recognizing problem we try to add template-based model [13] at the end of the neural network.中文译文基于先验知识的车牌识别Qian Gao, Xinnian Wang and Gongfu Xie摘要- 本文介绍了一种基于改进的BP(反向传播)神经网络的中国车牌识别(LPR)算法。
有关车牌的介绍(英语)(双语)

The cost of a car license plate hit a record high at an auction in Shanghai, skyrocketing to more than 90,000 yuan ($14,480), despite municipal government efforts at regulating the market.
1994 Base price
The auction by tender
2000 No base price
Ve automobiles and
the imported ones
2004 Limit to buy the license plate 2008 The new car can just use the new license plate
The Shanghai government said that the price of a secondhand car plate should not exceed the auction price of new car plates, a month before March's car plate auction on Saturday, blaming the rising price of secondhand plates for pushing up the new prices.
文献综述车牌识别

文献综述1前言近几年来,随着汽车的数量猛增,智能型交通体系(ITS——Intel ligent Tran sporta tion S ystem)便成为未来交通监管系统的主要发展趋势,所谓智能交通系统是在较完善的基础设施(包括道路、港口、机场和通信)之上将先进的信息技术、通信技术、控制技术、传感器、计算机技术和系统综合技术有效的集成,并应用于地面运输系统,从而建立起在大范围内发挥作用的,实时、准确、高效的运输系统[1~2]。
行驶车辆的车牌实时识别尤其是智能运输系统研究的重要组成部分。
车牌识别系统是对公路上配置的摄像头拍摄的照片进行数字图像处理与分析,综合应用大量的图像处理最新成果和数学形态学方法对汽车图像进行平滑、二值化、模糊处理、边缘检测、图像分割、开运算、比运算、区域标识等,利用多种手段以提取车牌区域,进而达到对汽车牌照的精确定位并最终完成对汽车牌照的识别。
车牌识别系统的用途很多,如高速公路电子收费站、公路流量控制、公路稽查、失窃车辆查询、监测黑牌机动车、监控违章车辆的电子警察等公路监管场合,以及停车场车辆管理、出入控制等需要车牌认证的场合都要应用车牌识别系统,尤其在高速公路收费系统中,实现不停车收费技术可提高公路系统的运行效率,由此可见车牌识别系统具有不可替代的作用,因此对车牌识别技术的研究和应用系统的开发具有重要的现实意义。
2 车牌识别技术研究现状车牌识别系统要综合应用多种手段提取车牌区域,对汽车牌照的精确定位并最终完成对汽车牌照的识别。
因此车牌识别系统要应对多种复杂环境,如车流量高峰期、照射反光、车牌污染等。
利用模拟人脑智能A NN,在识别车牌时能进行联想记忆与推理,能够较好地解决字符残缺不完整而无法识别的问题。
实时的车牌识别系统 中英文
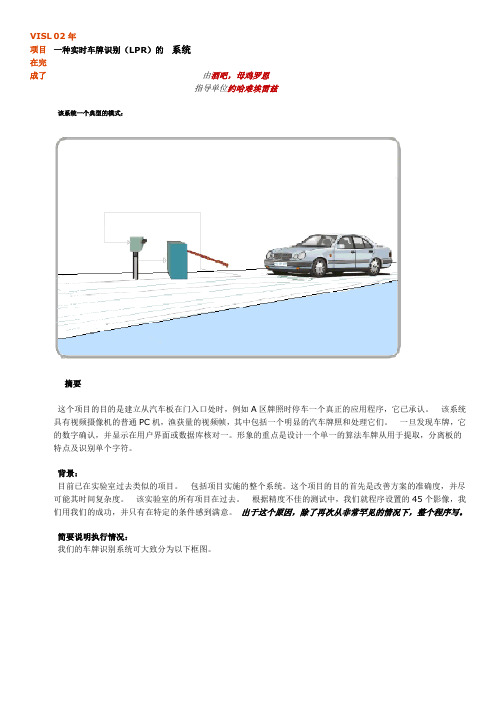
VISL 项目在完成了02年一种实时车牌识别(LPR)的系统由酒吧,母鸡罗恩指导单位约哈难埃雷兹该系统一个典型的模式:摘要这个项目的目的是建立从汽车板在门入口处时,例如A区牌照时停车一个真正的应用程序,它已承认。
该系统具有视频摄像机的普通PC机,渔获量的视频帧,其中包括一个明显的汽车牌照和处理它们。
一旦发现车牌,它的数字确认,并显示在用户界面或数据库核对一。
形象的重点是设计一个单一的算法车牌从用于提取,分离板的特点及识别单个字符。
背景:目前已在实验室过去类似的项目。
包括项目实施的整个系统。
这个项目的目的首先是改善方案的准确度,并尽可能其时间复杂度。
该实验室的所有项目在过去。
根据精度不佳的测试中,我们就程序设置的45个影像,我们用我们的成功,并只有在特定的条件感到满意。
出于这个原因,除了再次从非常罕见的情况下,整个程序写。
简要说明执行情况:我们的车牌识别系统可大致分为以下框图。
框图全球系统。
另外这个进程可以被看作是减少或地方的牌照抑制有害信息从携带信息的信号,这里是一个视频序列包含大量无关信息的特点,形式抽象符号的研究。
光学字符识别(OCR)已采用神经网络技术,采用神经元在输出层的前馈网络的3层,200个神经元在20输入层,中间神经元在10层,。
我们保留了神经网络数据集图像用在项目的先例,其中包括238位第我们的算法的详细步骤说明如下图:框图程序的子系统。
这里介绍捕获帧的一个给定的产出上面所述的主要步骤:示例捕获帧黄色区域捕获的帧过滤捕获帧地区扩张黄色车牌区域确定氡角度的变换板的使用改进的LP地区调整唱片轮廓-列和图调整唱片轮廓-线条和图唱片作物灰度唱片唱片二值化,均衡使用自适应阈值二进制唱片归唱片确定使用的LP水平轮廓图像总和先决行归唱片轮廓调节字符分割使用的山峰到山谷方法扩张型数位影像调整数字图像水平轮廓-线和图调整的数字图像轮廓调整大小的数字图像OCR的数字识别的神经网络方法工具该方案实施开发了基于Matlab。
英语作文车牌号格式
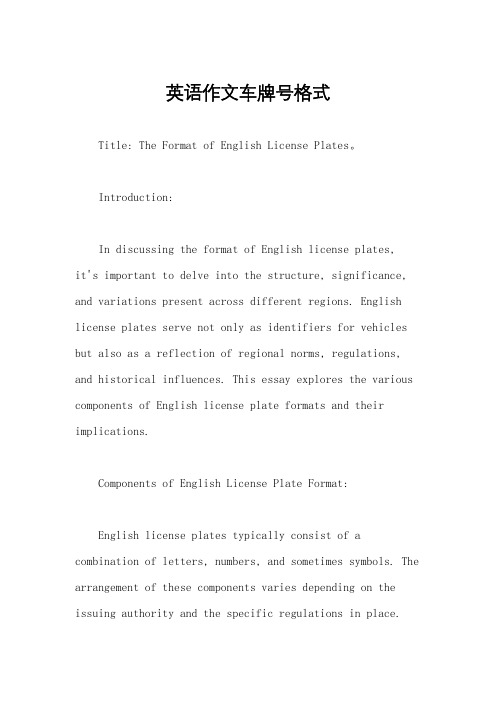
英语作文车牌号格式Title: The Format of English License Plates。
Introduction:In discussing the format of English license plates,it's important to delve into the structure, significance, and variations present across different regions. English license plates serve not only as identifiers for vehicles but also as a reflection of regional norms, regulations, and historical influences. This essay explores the various components of English license plate formats and their implications.Components of English License Plate Format:English license plates typically consist of a combination of letters, numbers, and sometimes symbols. The arrangement of these components varies depending on the issuing authority and the specific regulations in place.Generally, the format follows a pattern that facilitates easy identification and registration of vehicles.1. Letters:Letters on English license plates often serve as an identifier for the region or authority issuing the plate. These letters may be initials, abbreviations, or alphanumeric codes representing specific geographic areas or administrative divisions. For example, in the United Kingdom, the first two letters on a license plate indicate the region of registration, such as "AB" for Aberdeen or "LD" for London.2. Numbers:Numbers on license plates serve to further distinguish vehicles within a particular region. These numbers may be randomly assigned or follow a sequential pattern depending on the registration system in place. In some cases, the numbers may hold significance beyond mere identification, such as indicating the year of registration or a specificcategory of vehicles.3. Symbols:Symbols on license plates can convey additional information or serve decorative purposes. Common symbols include hyphens, spaces, and emblematic icons representing the issuing authority or country. These symbols may be strategically placed within the license plate format to enhance aesthetics or comply with regulatory standards.Variations in License Plate Formats:While certain aspects of license plate formats remain consistent across English-speaking regions, there are notable variations influenced by historical, cultural, and regulatory factors. These variations contribute to the diversity of license plate designs and formats observed globally.1. Regional Differences:Different regions within English-speaking countries may adopt distinct license plate formats to reflect local preferences or administrative structures. For instance, the format used in the United States differs from that of the United Kingdom, with variations in the arrangement of letters, numbers, and symbols.2. Specialized Plates:In addition to standard license plates, English-speaking countries often offer specialized plates for specific purposes or vehicle categories. These plates may feature unique formats, colors, or symbols to denote eligibility for privileges such as disability parking permits, vanity plates, or commemorative designs.3. Evolution Over Time:License plate formats have evolved over time in response to technological advancements, changing regulations, and societal trends. Modern license plates may incorporate features such as reflective materials, digitalprinting, or embedded chips for enhanced security and visibility.Conclusion:In conclusion, the format of English license plates encompasses a combination of letters, numbers, and symbols designed to facilitate vehicle identification and registration. While certain components remain consistent, variations in format and design reflect regional differences, specialized requirements, and evolving standards. Understanding the structure and significance of license plate formats is essential for navigating the roadways and interpreting vehicular information accurately.。
车牌识别某英文翻译
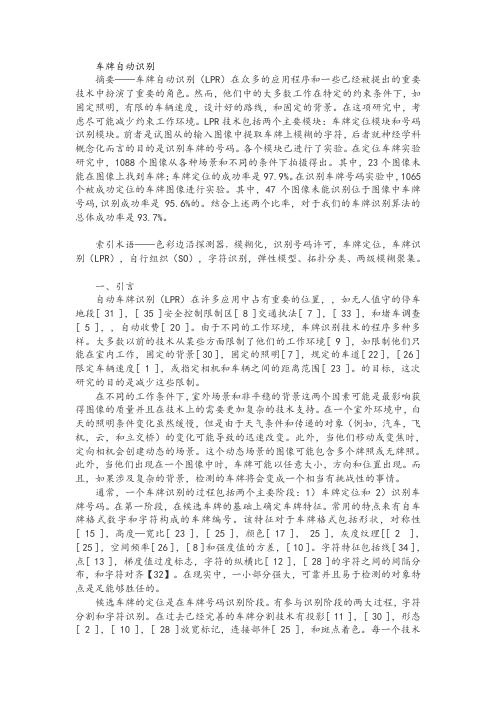
车牌自动识别摘要——车牌自动识别(LPR)在众多的应用程序和一些已经被提出的重要技术中扮演了重要的角色。
然而,他们中的大多数工作在特定的约束条件下,如固定照明,有限的车辆速度,设计好的路线,和固定的背景。
在这项研究中,考虑尽可能减少约束工作环境。
LPR技术包括两个主要模块:车牌定位模块和号码识别模块。
前者是试图从的输入图像中提取车牌上模糊的字符,后者就神经学科概念化而言的目的是识别车牌的号码。
各个模块已进行了实验。
在定位车牌实验研究中,1088个图像从各种场景和不同的条件下拍摄得出。
其中,23个图像未能在图像上找到车牌;车牌定位的成功率是97.9%。
在识别车牌号码实验中,1065个被成功定位的车牌图像进行实验。
其中,47个图像未能识别位于图像中车牌号码,识别成功率是95.6%的。
结合上述两个比率,对于我们的车牌识别算法的总体成功率是93.7%。
索引术语——色彩边沿探测器,模糊化,识别号码许可,车牌定位,车牌识别(LPR),自行组织(SO),字符识别,弹性模型、拓扑分类、两级模糊聚集。
一、引言自动车牌识别(LPR)在许多应用中占有重要的位置,,如无人值守的停车地段[ 31 ],[ 35 ]安全控制限制区[ 8 ]交通执法[ 7 ],[ 33 ],和堵车调查[ 5 ],,自动收费[ 20 ]。
由于不同的工作环境,车牌识别技术的程序多种多样。
大多数以前的技术从某些方面限制了他们的工作环境[ 9 ],如限制他们只能在室内工作,固定的背景[ 30 ],固定的照明[ 7 ],规定的车道[ 22 ],[ 26 ]限定车辆速度[ 1 ],或指定相机和车辆之间的距离范围[ 23 ]。
的目标,这次研究的目的是减少这些限制。
在不同的工作条件下,室外场景和非平稳的背景这两个因素可能是最影响获得图像的质量并且在技术上的需要更加复杂的技术支持。
在一个室外环境中,白天的照明条件变化虽然缓慢,但是由于天气条件和传递的对象(例如,汽车,飞机,云,和立交桥)的变化可能导致的迅速改变。
车牌识别外文翻译

中英文翻译A configurable method for multi-style license platerecognitionAutomatic license plate recognition (LPR) has been a practical technique in the past decades. Numerous applications, such as automatic toll collection, criminal pursuit and traffic law enforcement , have been benefited from it . Although some novel techniques, for example RFID (radio frequency identification), WSN (wireless sensor network), etc., have been proposed for car ID identification, LPR on image data is still an indispensable technique in current intelligent transportation systems for its convenience and low cost. LPR is generally divided into three steps: license plate detection, character segmentation and character recognition. The detection step roughly classifies LP and non-LP regions, the segmentation step separates the symbols/characters from each other in one LP so that only accurate outline of each image block of characters is left for the recognition, and the recognition step finally converts greylevel image block into characters/symbols by predefined recognition models. Although LPR technique has a long research history, it is still driven forward by various arising demands, the most frequent one of which is the variation of LP styles, for example:(1) Appearance variation caused by the change of image capturingconditions.(2)Style variation from one nation to another.(3)Style variation when the government releases new LP format. Wesummed them up into four factors, namely rotation angle,line number, character type and format, after comprehensive analyses of multi-style LP characteristics on real data. Generally speaking, any change of the above four factors can result in the change of LP style or appearance and then affect the detection, segmentation or recognition algorithms. If one LP has a large rotation angle, the segmentation and recognition algorithms for horizontal LP may not work. If there are more than one character lines in one LP, additional line separation algorithm is needed before a segmentation process. With the variation of character types when we apply the method from one nation to another, the ability to re-define the recognition models is needed. What is more, the change of LP styles requires the method to adjust by itself so that the segmented and recognized character candidates can match best with an LP format.Several methods have been proposed for multi-national LPs or multiformat LPs in the past years while few of them comprehensively address the style adaptation problem in terms of the abovementioned factors. Some of them only claim the ability of processing multinational LPs by redefining the detection and segmentation rules or recognition models.In this paper, we propose a configurable LPR method which is adaptable from one style to another, particularly from one nation to another, by defining the four factors as parameters.1Users can constrain the scope of a parameter and at the same time the method will adjust itself so that the recognition can be faster and more accurate. Similar to existing LPR techniques, we also provide details of detection, segmentation and recognition algorithms. The difference is that we emphasize on the configurable framework for LPR and the extensibility of the proposed method for multistyle LPs instead of the performance of each algorithm.In the past decades, many methods have been proposed for LPR that contains detection, segmentation and recognition algorithms. In the following paragraphs, these algorithms and LPR methods based on them are briefly reviewed.LP detection algorithms can be mainly classified into three classes according to the features used, namely edgebased algorithms, colorbased algorithms and texture-based algorithms. The most commonly used method for LP detection is certainly the combinations of edge detection and mathematical morphology .In these methods, gradient (edges) is first extracted from the image and then a spatial analysis by morphology is applied to connect the edges into LP regions. Another way is counting edges on the image rows to find out regions of dense edges or to describe the dense edges in LP regions by a Hough transformation .Edge analysis is the most straightforward method with low computation complexity and good extensibility. Compared with edgebased algorithms, colorbased algorithms depend more on the application conditions. Since LPs in a nation often have several2predefined colors, researchers have defined color models to segment region of interests as the LP regions .This kind of method can be affected a lot by lighting conditions. To win both high recall and low false positive rates, texture classification has been used for LP detection. In Ref.Kim et al. used an SVM to train texture classifiers to detect image block that contains LP pixels.In Ref. the authors used Gabor filters to extract texture features in multiscales and multiorientations to describe the texture properties of LP regions. In Ref. Zhang used X and Y derivative features,grey-value variance and Adaboost classifier to classify LP and non-LP regions in an image.In Refs. wavelet feature analysis is applied to identify LP regions. Despite the good performance of these methods the computation complexity will limit their usability. In addition, texture-based algorithms may be affected by multi-lingual factors.Multi-line LP segmentation algorithms can also be classified into three classes, namely algorithms based on projection,binarization and global optimization. In the projection algorithms, gradient or color projection on vertical orientation will be calculated at first. The “valleys”on the projection result are regarded as the space between characters and used to segment characters from each other.Segmented regions are further processed by vertical projection to obtain precise bounding boxes of the LP characters. Since simple segmentation methods are easily affected by the rotation of LP, segmenting the skewed LP becomes a key issue to be solved. In the binarization algorithms, global or local methods are often used3to obtain foreground from background and then region connection operation is used to obtain character regions. In the most recent work, local threshold determination and slide window technique are developed to improve the segmentation performance. In the global optimization algorithms, the goal is not to obtain good segmentation result for independent characters but to obtain a compromise of character spatial arrangement and single character recognition result. Hidden Markov chain has been used to formulate the dynamic segmentation of characters in LP. The advantage of the algorithm is that the global optimization will improve the robustness to noise. And the disadvantage is that precise format definition is necessary before a segmentation process.Character and symbol recognition algorithms in LPR can be categorized into learning-based ones and template matching ones. For the former one, artificial neural network (ANN) is the mostly used method since it is proved to be able to obtain very good recognition result given a large training set. An important factor in training an ANN recognition model for LP is to build reasonable network structure with good features. SVM-based method is also adopted in LPR to obtain good recognition performance with even few training samples. Recently, cascade classifier method is also used for LP recognition. Template matching is another widely used algorithm. Generally, researchers need to build template images by hand for the LP characters and symbols. They can assign larger weights for the important points, for example, the corner points, in the4template to emphasize the different characteristics of the characters. Invariance of feature points is also considered in the template matching method to improve the robustness. The disadvantage is that it is difficult to define new template by the users who have no professional knowledge on pattern recognition, which will restrict the application of the algorithm.Based on the abovementioned algorithms, lots of LPR methods have been developed. However, these methods aremainly developed for specific nation or special LP formats. In Ref. the authors focus on recognizing Greek LPs by proposing new segmentation and recognition algorithms. The characters on LPs are alphanumerics with several fixed formats. In Ref. Zhang et al. developed a learning-based method for LP detection and character recognition. Their method is mainly for LPs of Korean styles. In Ref. optical character recognition (OCR) technique are integrated into LPR to develop general LPR method, while the performance of OCR may drop when facing LPs of poor image quality since it is difficult to discriminate real character from candidates without format supervision. This method can only select candidates of best recognition results as LP characters without recovery process. Wang et al. developed a method to recognize LPR with various viewing angles. Skew factor is considered in their method. In Ref. the authors proposed an automatic LPR method which can treat the cases of changes of illumination, vehicle speed, routes and backgrounds, which was realized by developing new detection and segmentation algorithms with robustness to the5illumination and image blurring. The performance of the method is encouraging while the authors do not present the recognition result in multination or multistyle conditions. In Ref. the authors propose an LPR method in multinational environment with character segmentation and format independent recognition. Since no recognition information is used in character segmentation, false segmented characters from background noise may be produced. What is more, the recognition method is not a learning-based method, which will limit its extensibility. In Ref. Mecocci et al. propose a generative recognition method. Generative models (GM) are proposed to produce many synthetic characters whose statistical variability is equivalent (for each class) to that showed by real samples. Thus a suitable statistical description of a large set of characters can be obtained by using only a limited set of images. As a result, the extension ability of character recognition is improved. This method mainly concerns the character recognition extensibility instead of whole LPR method.From the review we can see that LPR method in multistyle LPR with multinational application is not fully considered. Lots of existing LPR methods can work very well in a special application condition while the performance will drop sharply when they are extended from one condition to another, or from several styles to others.多类型车牌识别配置的方法自动车牌识别(LPR)在过去的几十年中的实用技术。
- 1、下载文档前请自行甄别文档内容的完整性,平台不提供额外的编辑、内容补充、找答案等附加服务。
- 2、"仅部分预览"的文档,不可在线预览部分如存在完整性等问题,可反馈申请退款(可完整预览的文档不适用该条件!)。
- 3、如文档侵犯您的权益,请联系客服反馈,我们会尽快为您处理(人工客服工作时间:9:00-18:30)。
提出的模型
本文的主要目的是要开发一个系统可以从没有虚假质量的复杂的现场图像中提取车牌号码,相机和车牌之间的距离,其中的相对车牌已被抓获在相机等。
对车牌识别系统概述见图1,在车辆图片由相机拍摄后,它会被传递到预先处理单位由系统作进一步处理。
其主要功能是消除图像采集子系统所造成的噪声,提升图像的其他两个子系统使用的功效。
图像将被板提取模块扫描以找到车辆的车牌。
下一阶段是对于车牌中字符的分割。
最后每个字符将传递给光学字符识别(OCR)模块来进行识别确定,最终结果将是ASCII字符和车牌号码。
3.1.预处理
输入图像的最初处理是为了提高其质量,并为系统的下一阶段执行作准备。
首先,该系统使用的NTSC标准的方法将RGB图像转换为灰度图像。
G=0.299*R+0.587*G+0.114*B
第二步,用中值滤波(5x5)对灰度图像进行处理,以消除噪音,同时也能保持图像的清晰度。
中值滤波是一种非线性滤波器,它用各像素5x5邻里的计算得出中位值来取代该像素的值。
3.2.板块提取
板块提取处理包含五个不同的阶段进行,如图2所示;在这里每个阶段执行灰度图像分割过程以消除不属于车牌区域的多余的像素。
例如,水平定位阶段是负责寻找水平部分可能包含一个车牌。
在下面的讨论中,每一个阶段都会被细细展开讨论。
马来西亚车牌由一排白色字符在黑色的背景底色,所以我们可以说,车牌区域的特点是从一排黑色过渡到一排白色,反之亦然,这样转换被称为“边缘”。
从车牌字符到其背景在色彩强度上总的变化叫做边缘的强度。
最强边缘值,能够在从一个黑色像素过渡到一个白色像素或从白色像素变为黑色像素情况下被找到。
在理想的情况下,马来西亚的车牌是白色的字符绘制在黑色的背景上,因此这种搭配产生了高强度边缘值,用于查找出可能的板区域。
在本文中我们将使用Sobel算子来查找边缘。
Sobel运算在图片上执行一个2-D空间梯度测量。
通常它是用来寻找近似绝对的梯度幅度对在每一个点在输入的灰度图像上。
该Sobel
边缘检测器使用一对3x3卷积板块,一个在x方向(列)上按梯度检测,另一个在y方向(行)按梯度检测。
实际索贝尔Sobel面具如下:
图4说明了一个边缘检测过程中使用Sobel算子。
较淡的区域表示更强板块边缘的情况下牌照。
在创建的边缘图像后,系统会搜索有高边缘值的区域,这些区域中最有可能包含车
牌。
要做到这一点,系统将为边缘图像建立一个水平投影。
一个图像的投影轮廓是一个紧凑的空间表示像素内容分布。
水平投影轮廓被定义为每行上像素值之和的矢量。
图5显示了图表示水平投影图像的边缘,图像波峰表示为强边缘区域,可用于提取最有可能的水平位置车牌。
该系统将标记所有的对应的水平段相当于标记边缘图像的投影轮廓的波峰。
提取过程的下一步是在板中寻找车牌的垂直位置。
对于这部分,通常使用的方法为研究统计板的图片的直方图。
但这些方法并不适用于马来西亚的车牌,为两个原因:
1.字符数在各板上不同。
2.有些板的特点为字符写在两行。
我们提出了一个简单的方法用的边缘图像来定位图像板的垂直坐标。
该算法开始每个像素内的具体位置窗口。
通过每一个窗口水平段来滑动窗口和总结窗口内的边缘值,然后通过
窗口的面积划分结果得到的平均倾斜值。
对于每一个水平段,该算法为以后处理在进入下一个新的矢量时将存储每个窗口的结果值,我们称这个矢量为HDV,公式为:
这里i=0,1...(ImW- M+1),G(x,y)是边缘图像,W是滑动窗口,A w是滑动窗口的区域。
ImW是图像的宽度,M是滑动窗口的宽度。
图6说明了横向密度矢量的计算过程,由于窗口滑过模板的矢量值的平面密度(HDV)图逐渐增大,直到达到图像的波峰,然后再下降,在HDV图的波峰峰点时滑动窗口与车牌进行匹配。
图6. HDV的计算
此后,该算法能找到所有的波峰在HDV图形中的每个水平段。
一个波峰如果大于设定的阀值就假定属于一个车牌。
图7显示了一个例子为在这一过程中提取的垂直位置车牌随着每个HDV的图的水平段。
图7. 车牌垂直位置的例子
在垂直位置处理过程以后的下一步骤是是清理阶段。
这执行过程分为三个主要阶段,它们是:
1.两排板的的分隔处被错误地检测为一个段。
2.从多余的背景中将车牌孤立出来。
3.斜角的检测和纠正。
图8.两个板块出现在同一的水平段中
分离出来的包含有两行字符的车牌将通过二值图像一个水平投影来进行确切的提取和检测一个波谷作为一个特殊的阀值。
如被检测到,这一部分将被视为两排板,将被切割成两个独立部分,如下图9所示:
图9.车牌的水平投影
从多余的背景中分离出来的车牌使用一投影轮廓,反映了白色像素在每一列和每一行中的数目。
在大多数情况下,预测的纵向的和水平白色像素数量揭示了该板块的实际位置。
预测的一个例子如图10所示。
图10. 车牌的水平和垂直投影
要查找车牌的倾斜角度,我们所使用的算法描述在[1]中。
计算的倾斜角是通过Hough 变换找到车牌图像中的最明显的线和其角度,最后的车牌图像将被旋转的如图11所示。
图11. 偏斜角的纠正
定位过程的最后一步,是核查阶段。
该阶段的输入内容是前面几步中所有的可能包含有车牌的区域。
因此,这个阶段的主要目标是过滤掉不属于车牌的区域。
我们将遵循以基础规则为的分类做法是,如果下列条件之一满足则该区域将被删除:
1.该区域的高宽比(宽度/高度)小于/大于某阀值。
2.该区域的面积的是小于某一阀值。
3.每一像素的平均倾斜度小于/大于某一阀值。
4.有效的字符数为小于3或大于9。
5.区域密度小于/大于某一阀值(密度=对象区域面积÷所有区域面积)。
所有的阈值
决定于实验测试数据。
3.3.字符分割
为了简化字符识别的过程, 最好板块划分为不同的图像,每个包含一个分离的字符。
有一些广泛使用的字符分离方法,这些方法可用于几乎所有的可靠的车牌识别系统系统中。
这些方法有:静态式[4],垂直投影[8]和连接的组件。
前两种方法不能用来分割马来西亚车牌,因为每个车牌没有一个固定的字符数目。
本文中以下步骤是用来提取车牌的字符:
1.在可用的灰度值的范围内(0~255)内,扩大图像的对比度。
2.用Otsu方法[10]对车牌图像按所规定的阀值进行分界。
3.搜索图像中的连通区域,每个连通域用一个特殊的标志进行标记来区分图像中两个不同的连通域。
如图12所示。
4.从以前的步骤中调整每个字符到标准的高度和宽度(20*10)用于进行下面的识别处理。
图12. 提取车牌字符
3.4.字符识别
人工神经网络用于字符识别。
我们使用的多层感知神经网络器(MLP神经网络)测试时用反向传播算法。
在学习阶段,数据库的字符构成表示在MLP神经网络的输入层相应的输出与理想的输出作比较。
在网络输出时,为了获取响应尽可能接近到理想输出的权重而反复修改。
输入层由135个输入神经元组成,一个隐藏层为有40个神经元有登录传递功能。
输出级有36神经元,每个输出给定的概率输入相应的字符。