人工智能 贝叶斯网络.ppt
合集下载
人工智能 贝叶斯网络
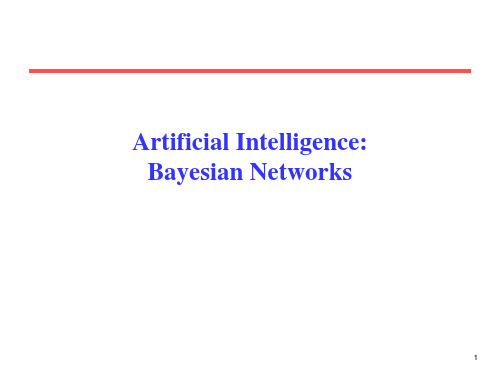
– Roots (sources) of the DAG that have no parents are given prior probabilities.
P(B) .001
Burglary
Earthquake
B E P(A)
P(E) .002
T
T
F T F
.95
.94 .29 .001 A P(M) .70 .01 4
11
Bayes Net Inference
• Given known values for some evidence variables, determine the posterior probability of some query variables. • Example: Given that John calls, what is the probability that there is a Burglary?
Alarm
A T F P(J) .90 .05
T F F
JohnCalls
MaryCalls
T F
CPT Comments
• Probability of false not given since rows must add to 1. • Example requires 10 parameters rather than 25–1 = 31 for specifying the full joint distribution. • Number of parameters in the CPT for a node is exponential in the number of parents (fan-in).
– For example, Burglary and Earthquake should be considered independent since they both cause Alarm.
P(B) .001
Burglary
Earthquake
B E P(A)
P(E) .002
T
T
F T F
.95
.94 .29 .001 A P(M) .70 .01 4
11
Bayes Net Inference
• Given known values for some evidence variables, determine the posterior probability of some query variables. • Example: Given that John calls, what is the probability that there is a Burglary?
Alarm
A T F P(J) .90 .05
T F F
JohnCalls
MaryCalls
T F
CPT Comments
• Probability of false not given since rows must add to 1. • Example requires 10 parameters rather than 25–1 = 31 for specifying the full joint distribution. • Number of parameters in the CPT for a node is exponential in the number of parents (fan-in).
– For example, Burglary and Earthquake should be considered independent since they both cause Alarm.
贝叶斯网络
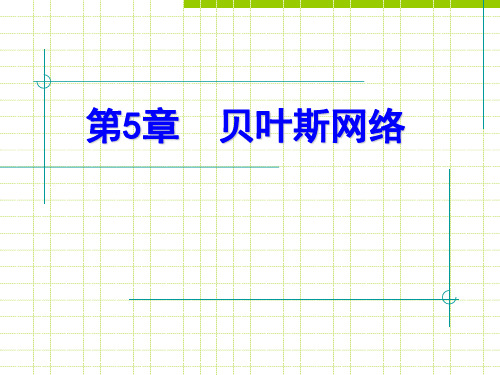
(40-9)
贝叶斯网络中的独立关系
•利用变量间的条件独立关系可以将联合概率分布分解成多个复杂度较低的 概率分布,从而降低模型复杂度,提高推理效率。 •例如:由链规则可以把联合概率分布P(A, B, E, J, M)改写为: 独立参数:1+2+4+8+16=31
– E与B相互独立, 即P(E|B)=P(E) – 给定A时,J与B和E相互独立, 即P(J|B, E, A)=P(J|A) – 给定A时,M与J、B和E都相互独立,即P(M|J, A, B, E)=P(M|A)
– 条件独立 – 因果影响独立 – 环境独立
(40-11)
贝叶斯网络中的独立关系
(一)条件独立
•贝叶斯网络的网络结构表达节点间的条件独立关系。 •三种局部结构
– 顺连 (serial connection) – 分连(diverging connection) – 汇连(converging connection)
(40-15)
贝叶斯网络中的独立关系
(四)环境独立(context independence)
•环境独立是指在特定环境下才成立的条件独立关系。 •一个环境是一组变量及其取值的组合。设环境中涉及变量的集合用 C表示, C的一种取值用c表示,则C=c表示一个环境。 •定义5.8 设X,Y,Z,C是4个两两交空的变量集合,如果 P(X, Y, Z, C=c)>0 且 P(X|Y, Z, C=c)= P(X| Z, C=c) 则称X, Y在环境C=c下关于Z条件独立。若Z为空,则称X, Y在环境C=c下 环境独立。
得到联合概率边缘化分布:
再按照条件概率定义,得到
(40-8)
不确定性推理与联合概率分布
贝叶斯网络全解 共64页
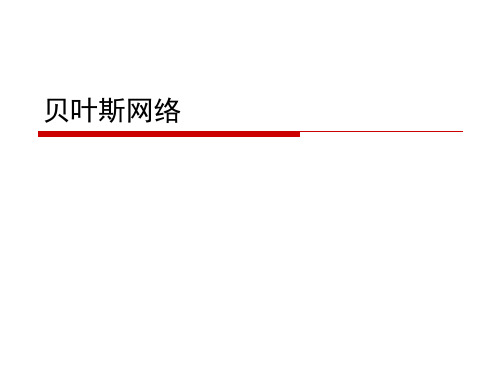
意结点到B中任意结点的路径,若要求A,B条件独 立,则需要所有的路径都被阻断(blocked),即满足 下列两个前提之一:
A和B的“head-to-tail型”和“tail-to-tail型”路径都通过C; A和B的“head-to-head型”路径不通过C以及C的子孙;
32
有向分离的举例
每个结点在给定其直接前驱时,条件独立于其非后继。
稍后详细解释此结论
18
一个简单的贝叶斯网络
19
全连接贝叶斯网络
每一对结点之间都有边连接
20
一个“正常”的贝叶斯网络
有些边缺失 直观上:
x1和x2独立 x6和x7在x4给定的条件下独立
x1,x2,…x7的联合分布:
21
BN(G, Θ) G:有向无环图 G的结点:随机变量 G的边:结点间的有向依赖 Θ:所有条件概率分布的参数集合 结点X的条件概率:P(X|parent(X))
思考:需要多少参数才能确定上述网络呢? 每个结点所需参数的个数:结点的parent数目是M,结点和 parent的可取值数目都是K:KM*(K-1) 为什么? 考察结点的parent对该结点形成了多少种情况(条件分布)
贝叶斯网络(Bayesian Network),又称有向无环图模 型(directed acyclic graphical model),是一种概率图 模型,借由有向无环图(Directed Acyclic Graphs, DAG)中得知一组随机变量{X1,X2...Xn}及其n组条 件概率分布(Conditional Probability Distributions, CPD)的性质。
Gas和Radio是独立的吗?给定Battery呢? Ignition呢?Starts呢?Moves呢?(答:IIIDD)
A和B的“head-to-tail型”和“tail-to-tail型”路径都通过C; A和B的“head-to-head型”路径不通过C以及C的子孙;
32
有向分离的举例
每个结点在给定其直接前驱时,条件独立于其非后继。
稍后详细解释此结论
18
一个简单的贝叶斯网络
19
全连接贝叶斯网络
每一对结点之间都有边连接
20
一个“正常”的贝叶斯网络
有些边缺失 直观上:
x1和x2独立 x6和x7在x4给定的条件下独立
x1,x2,…x7的联合分布:
21
BN(G, Θ) G:有向无环图 G的结点:随机变量 G的边:结点间的有向依赖 Θ:所有条件概率分布的参数集合 结点X的条件概率:P(X|parent(X))
思考:需要多少参数才能确定上述网络呢? 每个结点所需参数的个数:结点的parent数目是M,结点和 parent的可取值数目都是K:KM*(K-1) 为什么? 考察结点的parent对该结点形成了多少种情况(条件分布)
贝叶斯网络(Bayesian Network),又称有向无环图模 型(directed acyclic graphical model),是一种概率图 模型,借由有向无环图(Directed Acyclic Graphs, DAG)中得知一组随机变量{X1,X2...Xn}及其n组条 件概率分布(Conditional Probability Distributions, CPD)的性质。
Gas和Radio是独立的吗?给定Battery呢? Ignition呢?Starts呢?Moves呢?(答:IIIDD)
第7章贝叶斯网络.ppt
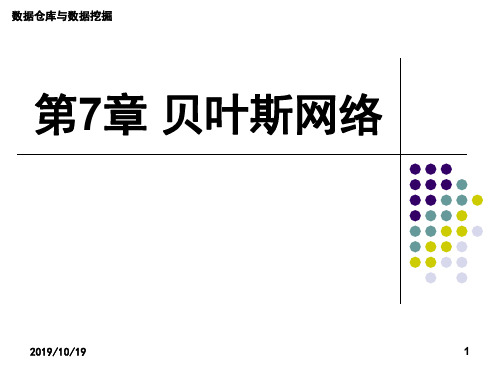
计算已知参加晚会的情况下,第二天早晨呼吸有 酒精味的概率。
P(+SA)=P(+HO)P(+SA|+HO)+P(-HO)P(+SA|-HO)
计算已知参加晚会的情况下,头疼发生的概率。
2019/10/19
数据仓库与数据挖掘
15
7.4.2 贝叶斯网络的预测算法
输入:给定贝叶斯网络B(包括网络结构m个节点以及某些节点间的连线、原因 节点到中间节点的条件概率或联合条件概率),给定若干个原因节点发生与 否的事实向量F(或者称为证据向量);给定待预测的某个节点t。
2019/10/19
数据仓库与数据挖掘
11
7.3.3 贝叶斯网络的3个主要议题
贝叶斯网络预测:从起因推测一个结果的理论, 也称为由顶向下的推理。目的是由原因推导出结 果。
贝叶斯网络诊断:从结果推测一个起因的推理, 也称为由底至上的推理。目的是在已知结果时, 找出产生该结果的原因。
贝叶斯网络学习:由先验的贝叶斯网络得到后验 贝叶斯网络的过程。
13
7.4.1 概率和条件概率数据
P(PT)
P(BT)
P(HO|PT)
PT=True
True False
0.200 0.800
0.001 0.999
True False
0.700 0.300
PT=False 0
1.000
左表给出了事件发生的概率:PT发生 的概率是0.2,不发生的概率是0.8
右表给出了事件发生的条件概率:PT 发生时,HO发生的概率是0.7
概率分布,并把节点n标记为已处理; (5)重复步骤(2)-(4)共m次。此时,节点t的概率分布就是它的发生/不发
AI-05-15-贝叶斯网络-----人工智能课程--浙江大学研究生PPT课件
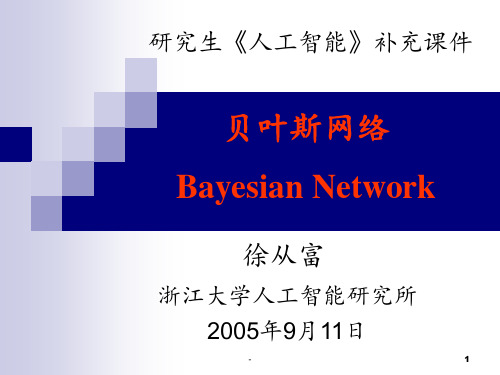
(C) 0.50
工作压力 大(W)
U P(W)
t 0.90 f 0.05
学校政策 (U)
C P(U) t 0.95 f 0.01
身体状况 差(B)
U P(B) t 0.30 f 0.01
W B P(A)
过劳死 (D)
t t 0.335 t f 0.30
f t 0.05
-
f f 0.00
26
已知:一个事件e = {学校政策U = true, and 工作压力大 = true},
-
28
多连通网络及其CPT: P(C) 0.50 Cloudy
C P(S) t 0.10 f 0.50
Sprinkler
Rain
C P(R) t 0.80 f 0.20
Wet Grass
S R P(W) t t 0.99 t f 0.90 f t 0.90 f f 0.00
-
29
等价的联合树及其CPT:
A. 贝叶斯网络的由来 B. 贝叶斯网络的定义 C. 贝叶斯网络的别名 D. 独立和条件独立 E. 贝叶斯网络示例
-
3
A. 贝叶斯网络的由来
全联合概率计算复杂性十分巨大
朴素贝叶斯太过简单
现实需要一种自然、有效的方式来捕捉 和推理——不确定性知识
变量之间的独立性和条件独立性可大大 减少为了定义全联合概率分布所需的概 率数目
“因果模型”比“诊断模型”需要更少的数 据,且这些数据也更容易得到
-
12
贝叶斯网络中的条件独立关系:
给定父节点,一个节点与它的非后代节点是 条件独立的
给定一个节点的父节点、子节点以及子节点 的父节点——马尔可夫覆盖(Markov blanket), 这个节点和网络中的所有其它节点是条件独 立的
工作压力 大(W)
U P(W)
t 0.90 f 0.05
学校政策 (U)
C P(U) t 0.95 f 0.01
身体状况 差(B)
U P(B) t 0.30 f 0.01
W B P(A)
过劳死 (D)
t t 0.335 t f 0.30
f t 0.05
-
f f 0.00
26
已知:一个事件e = {学校政策U = true, and 工作压力大 = true},
-
28
多连通网络及其CPT: P(C) 0.50 Cloudy
C P(S) t 0.10 f 0.50
Sprinkler
Rain
C P(R) t 0.80 f 0.20
Wet Grass
S R P(W) t t 0.99 t f 0.90 f t 0.90 f f 0.00
-
29
等价的联合树及其CPT:
A. 贝叶斯网络的由来 B. 贝叶斯网络的定义 C. 贝叶斯网络的别名 D. 独立和条件独立 E. 贝叶斯网络示例
-
3
A. 贝叶斯网络的由来
全联合概率计算复杂性十分巨大
朴素贝叶斯太过简单
现实需要一种自然、有效的方式来捕捉 和推理——不确定性知识
变量之间的独立性和条件独立性可大大 减少为了定义全联合概率分布所需的概 率数目
“因果模型”比“诊断模型”需要更少的数 据,且这些数据也更容易得到
-
12
贝叶斯网络中的条件独立关系:
给定父节点,一个节点与它的非后代节点是 条件独立的
给定一个节点的父节点、子节点以及子节点 的父节点——马尔可夫覆盖(Markov blanket), 这个节点和网络中的所有其它节点是条件独 立的
第8章贝叶斯网导论【本科研究生通用机器学习课程精品PPT系列】
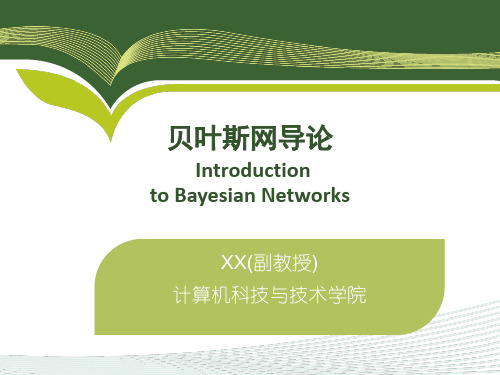
Burglary 独立假设2
独立假设2 Earthquake
Alarm
Alarm
JohnCalls
MaryCalls
1.5解决方案
•合并独立假设1和独立假设2,可得:P(John| Burglary, Earthquake, Alarm)=P(John| Alarm)
合并独立假设1和2
Burglary
P(E e) P( X ) 是 X 的先验分布, P(X | E e) 是 X 的后验分布, P(E e | X ) 称为 X 的似然函数。 P(E e) 是一个归一化常数
后验分布正比于先验分布和似然函数的乘积。
1.3几个重要原理
链规则(chain rule)
利用变量间条件独立性
1.3不确定性推理与联合概率分布
n n 9.1E-1
1.3不确定性推理与联合概率分布
从联合概率分布 P(Burglary,Earthquake, Alarm,John,Mary)出发,先计算边缘分布
P(Burglary, Mary)
P(Burglary, Earthquake, Alarm, John, Mary)
Earthquake, Alarm,John
0.000115
0.61
P(Burglary y, Mary y) P(Burglary n, Mary y) 0.000115 0.000075
1.4存在的问题
直接使用联合分布进行不确定性推理的困难很明显,即它的复杂度
极高。上图中有 5 个二值随机变量,整个联合分布包含25 1 31 个独
n n 2.8E-4 n
n
y
n n 2.9E-5
y
n
人工智能贝叶斯网络.ppt

• Directed Acyclic Graph (DAG)
– Nodes are random variables – Edges indicate causal influences
Burglary
Earthquake
Alarm
JohnCalls
MaryCalls
3
Conditional Probability Tables
– Bayesian Networks: Directed acyclic graphs that indicate causal structure.
– Markov Networks: Undirected graphs that capture general dependencies.
2
Bayesian Networks
JohnCalls
MaryCalls
However, this ignores the prior probability of John calling.
12
Bayes Net Inference
• Example: Given that John calls, what is the probability that there is a Burglary?
7
Independencies in Bayes Nets
• If removing a subset of nodes S from the network renders nodes Xi and Xj disconnected, then Xi and Xj are independent given S, i.e. P(Xi | Xj, S) = P(Xi | S)
贝叶斯网络简介PPT课件
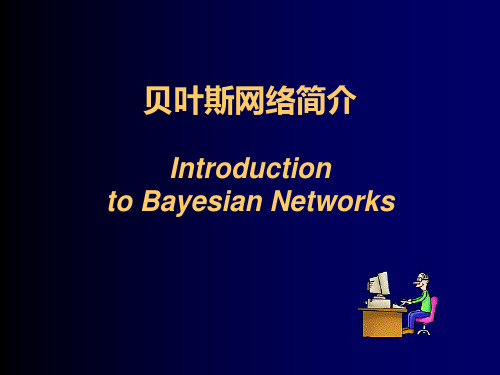
而在贝叶斯网络中,由于存在前述性质,任意随 机变量组合的联合条件概率分布被化简成
其中Parents表示xi的直接前驱节点的联合,概率 值可以从相应条件概率表中查到。
.
6
例子
P(C, S,R,W) = P(C)P(S|C)P(R|S,C)P(W|S,R,C) chain rule
= P(C)P(S|C)P(R|C)P(W|S,R,C) since
= P(C)P(S|C)P(R|C)P.(W|S,R) since
7
贝叶斯网络的构造及训练
1、确定随机变量间的拓扑关系,形成DAG 。这一步通常需要领域专家完成,而想要 建立一个好的拓扑结构,通常需要不断迭 代和改进才可以。
2、训练贝叶斯网络。这一步也就是要完成 条件概率表的构造,如果每个随机变量的 值都是可以直接观察的,方法类似于朴素 贝叶斯分类。但是通常贝叶斯网络的中存 在隐藏变量节点,那么训练方法就是比较 复杂。
4、将收敛结果作为推. 断值。
9
贝叶斯网络应用
医疗诊断,
工业,
金融分析,
计算机(微软Windows,Office),
模式识别:分类,语义理解
军事(目标识别,多目标跟踪,战争身份识别
等),
生态学,
生物信息学(贝叶斯网络在基因连锁分析中应
用),
编码学,
分类聚类,
时序数据和动态模型 .
• 用概率论处理不确定性的主要优点是保 证推理结果的正确性。
.
2
几个重要原理
• 链规则(chain rule)
P ( X 1 , X 2 ,X . n ) . P ( . X 1 ) , P ( X 2 |X 1 ) P ( X .n | . X 1 , . X 2 ,X . n ) ..,
- 1、下载文档前请自行甄别文档内容的完整性,平台不提供额外的编辑、内容补充、找答案等附加服务。
- 2、"仅部分预览"的文档,不可在线预览部分如存在完整性等问题,可反馈申请退款(可完整预览的文档不适用该条件!)。
- 3、如文档侵犯您的权益,请联系客服反馈,我们会尽快为您处理(人工客服工作时间:9:00-18:30)。
• However, this is too strict a criteria for conditional independence since two nodes will still be considered independent if their simply exists some variable that depends on both.
Artificial Intelligence: Bayesian Networks
1
Graphical Models
• If no assumption of independence is made, then an exponential number of parameters must be estimated for sound probabilistic inference.
• No realistic amount of training data is sufficient to estimate so many parameters.
• If a blanket assumption of conditional independence is made, efficient training and inference is possible, but such a strong assumption is rarely warranted.
• If removing a subset of nodes S from the network renders nodes Xi and Xj disconnected, then Xi and Xj are independent given S, i.e. P(Xi | Xj, S) = P(Xi | S)
– Bayesian Networks: Directed acyclic graphs that indicate causal structure.
– Markov Networks: Undirected graphs that capture general dependencies.
2
Bayesian Networks
A P(J) T .90 F .05
JohnCalls
MaryCalls
A P(M) T .70 F .01
4
CPT Comments
• Probability of false not given since rows must add to 1.
• Example requires 10 parameters rather than 25–1 = 31 for specifying the full joint distribution.
• Naïve Bayes is a simple Bayes Net
Y
… X1
X2
Xn
• Priors P(Y) and conditionals P(Xi|Y) for Naïve Bayes provide CPTs for the network.
7
Independencies in Bayes Nets
n
P(x1, x2 ,... xn ) P(xi | Parents( X i )) i 1
• Example
P(J M A B E)
P(J | A)P(M | A)P(A | B E)P(B)P(E)
0.90.70.0010.9990.998 0.00062
• Therefore an inefficient approach to inference is:
• Graphical models use directed or undirected graphs over a set of random variables to explicitly specify variable dependencies and allow for less restrictive independence assumptions while limiting the number of parameters that must be estimated.
• Directed Acyclic Graph (DAG)
– Nodes are random variables – Edges indicate causal influences
Burglary
EarthquaryCalls
3
Conditional Probability Tables
– 1) Compute the joint distribution using this equation. – 2) Compute any desired conditional probability using
the joint distribution.
6
Naïve Bayes as a Bayes Net
• Each node has a conditional probability table (CPT) that gives the probability of each of its values given every possible combination of values for its parents (conditioning case).
– Roots (sources) of the DAG that have no parents are given prior probabilities.
P(B)
.001
Burglary
P(E)
Earthquake .002
Alarm
B E P(A) T T .95 T F .94 F T .29 F F .001
• Number of parameters in the CPT for a node is exponential in the number of parents (fan-in).
5
Joint Distributions for Bayes Nets
• A Bayesian Network implicitly defines a joint distribution.
Artificial Intelligence: Bayesian Networks
1
Graphical Models
• If no assumption of independence is made, then an exponential number of parameters must be estimated for sound probabilistic inference.
• No realistic amount of training data is sufficient to estimate so many parameters.
• If a blanket assumption of conditional independence is made, efficient training and inference is possible, but such a strong assumption is rarely warranted.
• If removing a subset of nodes S from the network renders nodes Xi and Xj disconnected, then Xi and Xj are independent given S, i.e. P(Xi | Xj, S) = P(Xi | S)
– Bayesian Networks: Directed acyclic graphs that indicate causal structure.
– Markov Networks: Undirected graphs that capture general dependencies.
2
Bayesian Networks
A P(J) T .90 F .05
JohnCalls
MaryCalls
A P(M) T .70 F .01
4
CPT Comments
• Probability of false not given since rows must add to 1.
• Example requires 10 parameters rather than 25–1 = 31 for specifying the full joint distribution.
• Naïve Bayes is a simple Bayes Net
Y
… X1
X2
Xn
• Priors P(Y) and conditionals P(Xi|Y) for Naïve Bayes provide CPTs for the network.
7
Independencies in Bayes Nets
n
P(x1, x2 ,... xn ) P(xi | Parents( X i )) i 1
• Example
P(J M A B E)
P(J | A)P(M | A)P(A | B E)P(B)P(E)
0.90.70.0010.9990.998 0.00062
• Therefore an inefficient approach to inference is:
• Graphical models use directed or undirected graphs over a set of random variables to explicitly specify variable dependencies and allow for less restrictive independence assumptions while limiting the number of parameters that must be estimated.
• Directed Acyclic Graph (DAG)
– Nodes are random variables – Edges indicate causal influences
Burglary
EarthquaryCalls
3
Conditional Probability Tables
– 1) Compute the joint distribution using this equation. – 2) Compute any desired conditional probability using
the joint distribution.
6
Naïve Bayes as a Bayes Net
• Each node has a conditional probability table (CPT) that gives the probability of each of its values given every possible combination of values for its parents (conditioning case).
– Roots (sources) of the DAG that have no parents are given prior probabilities.
P(B)
.001
Burglary
P(E)
Earthquake .002
Alarm
B E P(A) T T .95 T F .94 F T .29 F F .001
• Number of parameters in the CPT for a node is exponential in the number of parents (fan-in).
5
Joint Distributions for Bayes Nets
• A Bayesian Network implicitly defines a joint distribution.