毕业论文外文翻译-利用人工神经网络和绕组的传递函数定位变压器的匝间短路故障
基于神经网络的变压器故障诊断
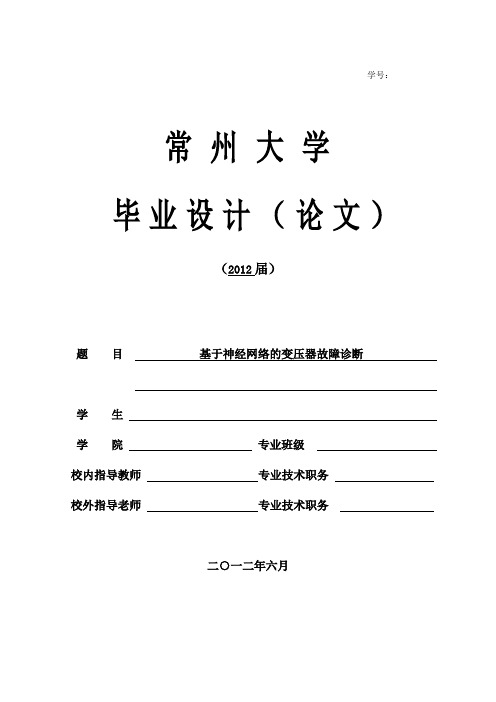
学号:常州大学毕业设计(论文)(2012届)题目基于神经网络的变压器故障诊断学生学院专业班级校内指导教师专业技术职务校外指导老师专业技术职务二○一二年六月摘要现代设备技术水平不断提高,生产率、自动化要求越来越高,相应地,故障也随之增加。
变压器作为电力系统中非常复杂而且非常重要的设备,其工作状态对电力系统、企事业单位生产及居民生活具有十分重要的影响。
如何提前对变压器故障进行预测和在故障发生后迅速判断故障原因是提高工作效率、减少经济损失的一个重要途径。
因此研究变压器故障诊断对保证系统安全、可靠、经济运行,提高经济效益具有重要意义。
概率神经网络(probabilistic neural networks)结构简单、训练简洁,利用概率神经网络模型的强大的非线性分类能力,将故障样本空间映射到故障模式空间中,可形成一个具有较强容错能力和结构自适应能力的诊断网络系统,从而提高故障诊断的准确率。
本文在对油中溶解气体分析法进行深入分析后,以改良三比值法为基础,建立基于概率神经网络的故障诊断模型。
然后,选取23组变压器故障原始样本数据对概率神经网络模型进行“学习”训练,获得了具有预测诊断功能的网络模型;选取10组变压器在线监测数据作为测试数据,并查看了训练数据网络的分类效果图和预测数据网络的分类效果图,结果只有两个样本判断错误,即只有两种变压器的故障类型判断错误,验证了基于概率神经网络在变压器故障预测诊断处理中的有效性。
关键词故障诊断概率神经网络变压器油中溶解气体分析THE STUDY OF POWER TRANSFORMER FAULT DIAGNOSIS BASED ON ARTIFICIAL NEURAL NETWORKAbstractWith the technical level of modern facility improves continually, the fault probability increases greatly. Power transformer has a very significant influence to power system, enterprise s production and people s life. How to forecast transformer s fault ahead and find the fault reason quickly after the fault is a good way to increase work efficiency and lighten the economy losing.Probabilistic neural network has the advantages of simple structure, simple training, the use of a probabilistic neural network model for strong nonlinear classification, fault sample space is mapped to a fault in the pattern space, can form a strong fault tolerant ability and structure of adaptive diagnosis system, so as to improve the accuracy of fault diagnosis. Based on the dissolved gas in oil analysis in-depth analysis, in order to improve the ratio of three as the basis, establish the fault diagnosis based on probabilistic neural network model. Then, select 23 group of transformer fault original sample data on the probabilistic neural network model of" learning" training, obtain the predictive diagnosis of functional network model; select 10 group of transformer on-line monitoring data as test data, and show the training data network classification effect diagram and the predicted data network classification effect chart, only the results of a sample of two errors of judgment, that only two transformer fault type judgement error, verification based on probabilistic neural network in transformer fault forecast and diagnosis treatment effectiveness.Keywords fault diagnosis, probability neural networks(PNN),power transformer,Dissolved Oas Analysis(DGA)摘要 (1)目录 (3)1 绪论 (4)1.1 国内外发展状况 (4)1.2 研究目的和意义 (4)1.3 论文工作介绍 (5)2 变压器故障诊断 (6)2.1 诊断工程概述 (6)2.2 故障诊断运作机理研究 (6)2.3 变压器故障诊断系统相关背景 (7)2.4 变压器故障诊断方法 (8)3 神经网络 (12)3.1 神经网络概述 (12)3.2神经网络的应用 (12)3.3神经网络的发展方向 (13)3.4 神经网络的结构 (14)3.5 概率神经网络概述 (16)3.6 概率神经网络与BP网络的比较选择 (17)4 基于概率神经网络的变压器故障诊断研究 (19)4.1 仿真环境简介 (19)4.2故障诊断模型建立 (20)4.3 基于概率神经网络的变压器故障诊断实现 (21)4.4 仿真结果及讨论 (22)5 总结 (25)参考文献 (26)致谢 (27)故障诊断(FD,Fault Diagnosis)始于机械设备故障诊断。
关于变压器保护的20xx字英语文献
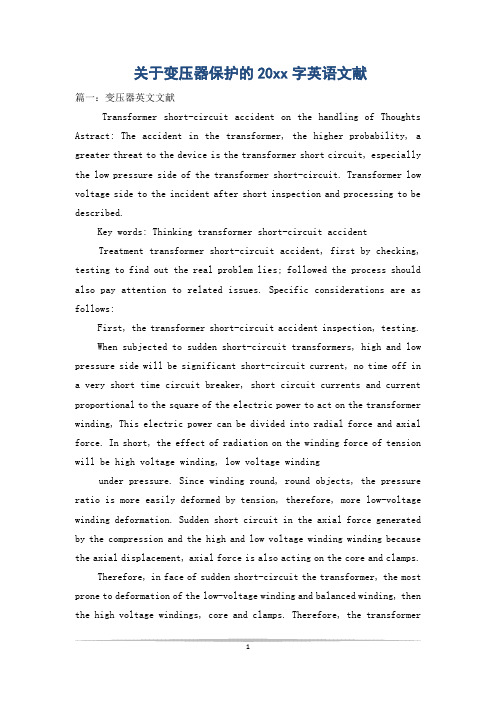
关于变压器保护的20xx字英语文献篇一:变压器英文文献Transformer short-circuit accident on the handling of Thoughts Astract: The accident in the transformer, the higher probability, a greater threat to the device is the transformer short circuit, especially the low pressure side of the transformer short-circuit. Transformer low voltage side to the incident after short inspection and processing to be described.Key words: Thinking transformer short-circuit accidentTreatment transformer short-circuit accident, first by checking, testing to find out the real problem lies; followed the process should also pay attention to related issues. Specific considerations are as follows:First, the transformer short-circuit accident inspection, testing. When subjected to sudden short-circuit transformers, high and low pressure side will be significant short-circuit current, no time off in a very short time circuit breaker, short circuit currents and current proportional to the square of the electric power to act on the transformer winding, This electric power can be divided into radial force and axial force. In short, the effect of radiation on the winding force of tension will be high voltage winding, low voltage windingunder pressure. Since winding round, round objects, the pressure ratio is more easily deformed by tension, therefore, more low-voltage winding deformation. Sudden short circuit in the axial force generated by the compression and the high and low voltage winding winding because the axial displacement, axial force is also acting on the core and clamps. Therefore, in face of sudden short-circuit the transformer, the most prone to deformation of the low-voltage winding and balanced winding, then the high voltage windings, core and clamps. Therefore, the transformershort-circuit accident, the inspection is to check the main winding, core, clamps and other parts.First, the winding of the inspection and testWhen the transformer short-circuit in the electric power under the action of winding the same time by pressing, pulling, bending and other forces acting, concealment caused by the failure of its strong, is not easy to check and repair, so the short circuit fault should focus on checking winding situation.(A) of the transformer DC resistance measurementAccording to the transformer DC resistance measurements to check the winding DC resistance unbalance and compared with previous measurements, can effectively examine the transformer winding damage. For example, a low voltage transformer short-circuit side after the accident to the DC resistance ofC increased by about 10% of new shares which may be winding to determine the situation, and finally winding hanging out, it was discovered off one phase winding C shares.(2) measurement of transformer winding capacitance.By the winding capacitance between the windings, and the cake layer capacitance and the winding-fat capacitor. This capacitor and the winding and core and in the gap, winding and core of the gap between the windings, the gap between cake layers and on. When the winding deformation, the general was “S”-shaped bend, which leads to winding on the core of the gap distance smaller, winding capacitance to ground will be larger, but the smaller the gap, the greater the capacitance changes, so winding electrical capacity can indirectly reflect the degree of winding deformation.(3) check after hanging hood.After lifting the transformer cover, check out the transformer ifinternal molten slag or copper slag or aluminum pieces of paper, high-density cable, you can determine the occurrence of a greater degree of winding deformation and off shares, etc. In addition, the shift from the winding Pad or off, clips and other bits, the pressure screw displacement can also determine the extent of the damage the windings. 2, core and clamps checks.Transformer core should have sufficient mechanical strength. Core of the mechanical strength is by all clamping on the core strength of their connections to guarantee. When the electric power generated when the winding, winding clamping axial force of the reaction will be offset, if the clamps, pull strength of less than the axial force plate when the clamps, pull board and the winding will be damaged. Therefore, we should carefully check the core, clamps, pull the state board and its connections.(1) Check the yoke iron core chips have ran up and down situation.(2) should be measured through the core and the core of the insulation resistance of the screw, check whether the damaged wearing coat-core screw; check the drawing board, drawing board connector for damage.(3) because of short circuit in the transformer, the plate and the clamps mayoccur between the displacement pressure of the nail plate and the yoke pulled off the ground connection, or over-current chip burning, so the plate for the winding, in addition to check the pressure screw, plate damaged, we must also check the winding and the pressure on the yoke screw and the ground connection is reliable.3, the analysis of transformer oil and gas.After suffering the impact of transformer short-circuit in the gas relay large amounts of gas may accumulate, it can be taken after the accident in the transformer gas relay the gas and oil inside the transformer for laboratory analysis, to determine the nature of theincident.Second, the transformer short-circuit fault handling precautions. 1, pieces of insulation should be replaced to ensure the performance of insulators.When dealing with the replacement of insulation parts should be tested for performance, and meet the requirements before use. In particular insulation on the lead frame wood attention should be paid. Wood should be placed before instal lation of about 80 ℃ hot transformer oil immersion period of time to ensure that the insulation of wood. 2, transformer oil filling the transformer insulation test should be conducted after 24 hours of rest.Because some of the moisture in the insulation pieces soak in the hot oil a longer time, the water will spread to the surface of insulation, if the fuelinjection after the test check the insulation defects often do not come out. For example, a 31.5MVA the 110kV transformer low voltage side of thereplacement of the treatment of a stent kV copper block, transformer filling of all the normal tests, 10kV low voltage side of the core, clamps, and insulation resis tance is reduced to about 1MΩ. Cover by hanging after examination, found that the stent 10kV copper block insulation is very low. Therefore, the transformer insulation test should be conducted 24 hours after the grease still more reliable.3, the core back to the equipment should be noted that the sharp corners.Installed in the back yoke, attention should be angular core chip, and timely measurement of oil duct insulation, in particular, pay attention to the oil channel at the chip corners, to prevent overlap resulting core chip multi-point grounding. For example, one of the 220kV120MVA transformers, replacementof the low pressure side of the winding back yoke installed, due to back loaded in the chip did not pay attention to sharp corners, and no timely measure the oil duct insulation, after installation of insulation to measure the oil channel 0Finally, take a long time to find the core chip, due to short circuit the oil channel sharp corners.关于处理变压器短路事故的几点思考摘要:在变压器事故中,发生概率较高、对设备威胁较大的就是变压器短路事故,特别是变压器低压侧发生短路。
基于人工神经网络的电力系统故障诊断技术研究
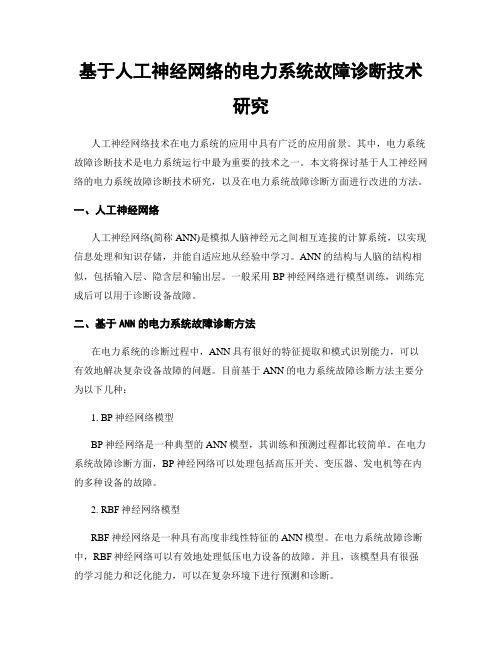
基于人工神经网络的电力系统故障诊断技术研究人工神经网络技术在电力系统的应用中具有广泛的应用前景。
其中,电力系统故障诊断技术是电力系统运行中最为重要的技术之一。
本文将探讨基于人工神经网络的电力系统故障诊断技术研究,以及在电力系统故障诊断方面进行改进的方法。
一、人工神经网络人工神经网络(简称ANN)是模拟人脑神经元之间相互连接的计算系统,以实现信息处理和知识存储,并能自适应地从经验中学习。
ANN的结构与人脑的结构相似,包括输入层、隐含层和输出层。
一般采用BP神经网络进行模型训练,训练完成后可以用于诊断设备故障。
二、基于ANN的电力系统故障诊断方法在电力系统的诊断过程中,ANN具有很好的特征提取和模式识别能力,可以有效地解决复杂设备故障的问题。
目前基于ANN的电力系统故障诊断方法主要分为以下几种:1. BP神经网络模型BP神经网络是一种典型的ANN模型,其训练和预测过程都比较简单。
在电力系统故障诊断方面,BP神经网络可以处理包括高压开关、变压器、发电机等在内的多种设备的故障。
2. RBF神经网络模型RBF神经网络是一种具有高度非线性特征的ANN模型。
在电力系统故障诊断中,RBF神经网络可以有效地处理低压电力设备的故障。
并且,该模型具有很强的学习能力和泛化能力,可以在复杂环境下进行预测和诊断。
3. SOM神经网络模型SOM神经网络是一种具有很强的自组织特征的ANN模型。
在电力系统故障诊断中,SOM神经网络主要用于电力监控系统中,可以对设备的状态进行实时监测和处理。
三、改进基于ANN的电力系统故障诊断方法无论是BP神经网络、RBF神经网络还是SOM神经网络,都存在着一些缺点和不足。
为了使其在电力系统故障诊断方面发挥更大的作用,需要进行改进。
当前,主要有如下改进方法:1. 搭建深度神经网络模型深度神经网络(Deep Neural Network)可以通过多层隐藏层来提高模型的非线性拟合能力。
在应用于电力系统故障诊断时,搭建深度神经网络模型可以提高模型的准确率和诊断精度。
毕业设计(论文)-基于BP神经网络的电路故障诊断
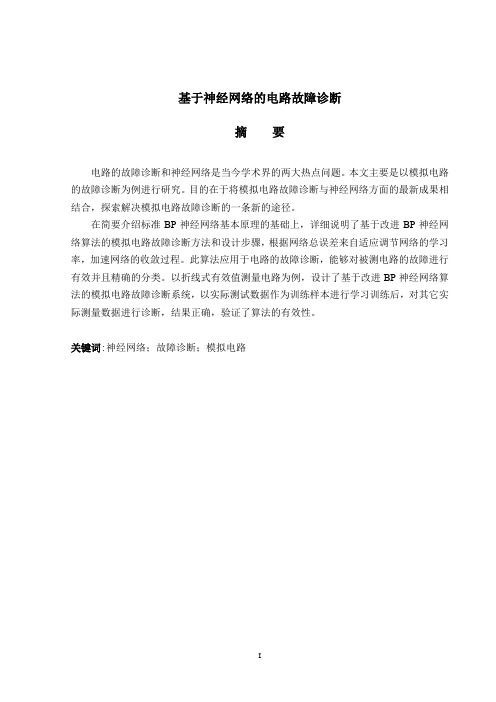
模拟电路故障诊断是微电子技术中的一个重要课题,同时也是网络理论的一个重要课,模拟电路故障诊断方法主要有以下三种:
1.3模拟电路故障诊断的意义
模拟电路广泛应用于军工、通讯、自动控制、测量仪表、家用电器等各个方面。随着大规模模拟集成电路的发展,模拟电路的复杂度和密集度不断增长,对模拟电路运行可靠性的要求更为严格。就模拟电路生产工厂而言,也要求能诊断出故障以便分析原因,改进工艺以提高成品合格率。对某些用于重要设备的模拟电路,还要求能进行故障预测,也就是对模拟电路在正常工作时的响应作持续不断的监测,以确定哪些元件将要失效,以便在模拟电路故障发生前将那些将要失效的元件替换掉,以避免故障发生。所有这些,通常的人工诊断技术已无法满足需要。因而,电路故障的自动诊断成为一个急待要解决的问题,自动故障诊断的关键在于诊断程序的产生,而诊断程序产生的中心问题是电路故障诊断理论。因此,模拟电路故障诊断的研究引起世界各国电路理论工作者的高度重视。
现代社会中,电子设备或系统广泛应用于各个科学技术领域、工业生产部门以及人们的日常生活中,电子设备的可靠性直接影响着生产的效率、系统、设备及人类的生命安全。随着电子设备使用的日趋广泛,不论是在设备的生产阶段还是应用阶段,都对电路的故障诊断提出了迫切的要求,要求人们研究新的有效的诊断技术,进一步提高电子设备的可靠性,设备诊断技术引入生产现场已三十多年。最初,设备较为简单,维修人员主要靠感觉器官、简单仪表和个人经验就能胜任故障的诊断和排除工作,即为传统的诊断技术。随着科学技术的不断发展,动力机械设备越来越复杂化、精密化、系统化和自动化,同时价格也越来越昂贵,设备在现代工业生产中的作用和影响越来越大,生产的主体也逐渐由人力向设备转移,与设备有关的费用越来越高,传统的诊断方法已远远不能适应。机器运行中发生的任何故障或失效不仅会引起严重后果,造成重大的经济损失,甚至还可能导致灾难性的人员伤亡和恶劣的社会影响。
基于神经网络的变压器故障检测毕业论文
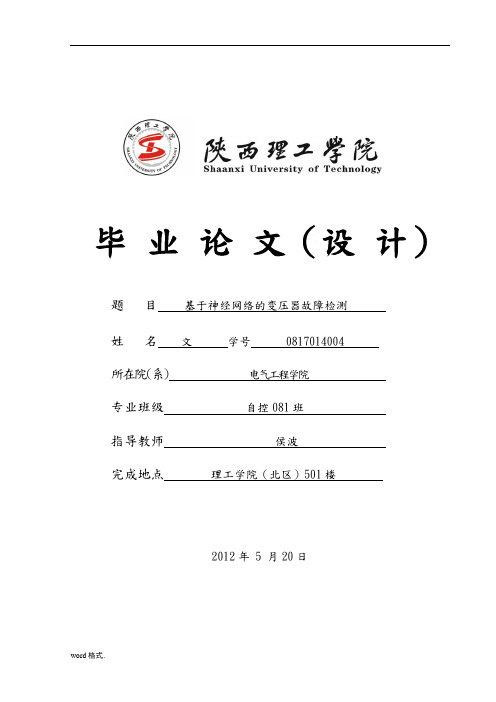
毕业论文(设计)题目基于神经网络的变压器故障检测姓名文学号 0817014004所在院(系)电气工程学院专业班级自控081班指导教师侯波完成地点理工学院(北区)501楼2012年 5 月20日基于神经网络的变压器故障检测文(理工学院电气工程学院自动化专业081班, 723003)指导教师:侯波[摘要]:电力变压器作为电力系统中最为重要的设备之一,对电力系统安全、可靠、优质、经济的运行起着决定性作用,因而,必须尽量减少变压器故障的产生。
电力变压器故障检测对电力系统的经济安全运行有着重要的意义。
油中溶解气体法,是最有效的发现和检测变压器故障的方法之一。
神经网络对外界具有很强的模式识别分类能力和联想记忆能力,因此神经网络可以用于变压器故障检测。
基于神经网络的以变压器油中溶解气体为特征量的故障检测方法为变压器故障检测提供了新的途径。
本文将采用三种不同的神经网络(BP网络、RBF网络、支持向量机)应用于变压器故障检测中,分别介绍这几种网络的基本结构和原理,并进行模型设计和仿真。
[关键词]:变压器故障检测神经网络 BP算法 RBF算法支持向量机Based on neural network of transformer fault detectionAuthor:Yang wen(Grade 08, Class 01,Major Automation,Department of Electrical Engineering ,Shaanxi University of Technology ,Hanzhong ,723003,Shaanxi )Tutor :Hou BoAbstract :as the most important part of the power system equipment,the power transformer to the safety of the electricity system, reliable and high quality, and the operation of the economy plays a decisive role, therefore, we must try to reduce the of transformer faults. Power transformer of electric power system fault detection of the economic security has important significances. The dissolved gas method, is one the most effective and found that one of the ways to detect transformer faults. Neural network has a strong pattern recognition classification ability and associative memory ability to the outside world, so neural network can be used for the transformer fault detection. Based on neural network to gases dissolved in transformer oil for the characteristic features of fault detection method for transformer fault detection offers a new way. Therefore.This article will use three different neural network (BP network, RBF network, support vector machine) used in transformer fault detection, are introduced the basic structure of the network and the principle and design and simulation model.key words : transformer ,fault detection ,neural network ,BP algorithm ,RBF algorithm ,support vector machine.目录1 绪论 (1)1.1课题研究的目的和意义 (1)1.2国外发展状况 (1)1.3变压器故障种类 (1)1.4目前变压器故障诊断的主要方法 (3)1.5本文研究的主要容 (4)2 基于神经网络的变压器故障检测机理和基本理论 (5)2.1 故障诊断技术 (5)2.2神经网络 (5)2.3 变压器故障与油中溶解气体的关系 (7)3 基于BP神经网络的变压器故障检测模型 (9)3.1 BP网络 (9)3.2 BP网络模型设计 (13)3.2.1 BP网络参数的确定 (13)3.2.2基于BP神经网络变压器故障检测模型 (15)3.2.3数据归一化处理 (15)3.3 系统仿真,训练与测试 (16)3.3.1网络训练 (16)3.3.2网络测试 (18)4 基于RBF神经网络的变压器故障检测模型 (20)4.1 RBF网络 (20)4.1.1 RBF网络概述 (20)4.1.2 RBF网络原理 (20)4.2 RBF网络模型设计 (21)4.2.1 RBF网络模型 (21)4.2.2 RBF网络参数的选取 (22)4.2.3 RBF网络训练方法的确定 (22)4.3 仿真结果 (22)5 基于支持向量机的变压器故障检测模型 (24)5 .1 支持向量机(SVM) (24)5 .1.1 支持向量机(SVM)基本理论 (24)5.1.2 支持向量机在故障诊断中的应用现状 (28)5.1.3 基于支持向量机变压器故障多分类算法 (28)5.2 变压器故障特征诊断模型设计 (29)5.2.1 变压器故障特征诊断参数选取 (29)5.2.2 故障诊断流程 (29)5.3系统仿真 (29)5.3.1 故障模型训练和参数寻优 (29)5.3.2 测试结果与分析 (33)6 结论与展望 (35)致 (36)参考文献 (37)英文文献 (39)1 绪论1 .1课题研究的目的和意义现代设备技术水平不断提高,生产率、自动化要求越来越高,相应地,故障也随之增加。
基于BP神经网络的变压器故障诊断研究毕业设计
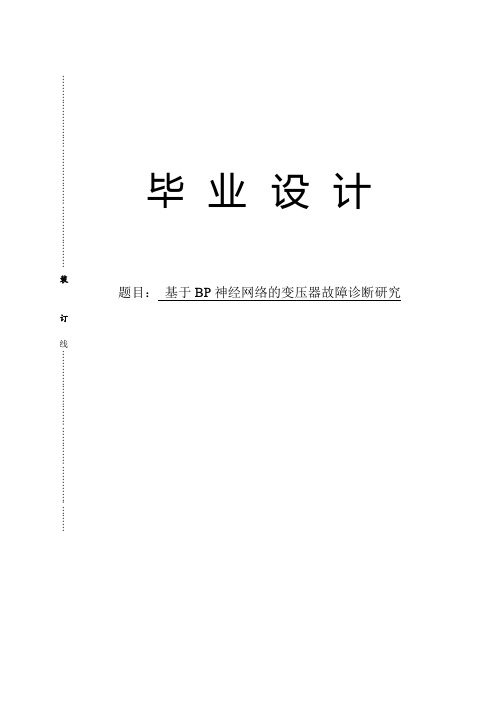
……………………. ………………. …………………毕业设计装题目:基于BP神经网络的变压器故障诊断研究订线……………….……. …………. …………. ………毕业设计(论文)原创性声明和使用授权说明原创性声明本人郑重承诺:所呈交的毕业设计(论文),是我个人在指导教师的指导下进行的研究工作及取得的成果。
尽我所知,除文中特别加以标注和致谢的地方外,不包含其他人或组织已经发表或公布过的研究成果,也不包含我为获得及其它教育机构的学位或学历而使用过的材料。
对本研究提供过帮助和做出过贡献的个人或集体,均已在文中作了明确的说明并表示了谢意。
作者签名:日期:指导教师签名:日期:使用授权说明本人完全了解大学关于收集、保存、使用毕业设计(论文)的规定,即:按照学校要求提交毕业设计(论文)的印刷本和电子版本;学校有权保存毕业设计(论文)的印刷本和电子版,并提供目录检索与阅览服务;学校可以采用影印、缩印、数字化或其它复制手段保存论文;在不以赢利为目的前提下,学校可以公布论文的部分或全部内容。
作者签名:日期:学位论文原创性声明本人郑重声明:所呈交的论文是本人在导师的指导下独立进行研究所取得的研究成果。
除了文中特别加以标注引用的内容外,本论文不包含任何其他个人或集体已经发表或撰写的成果作品。
对本文的研究做出重要贡献的个人和集体,均已在文中以明确方式标明。
本人完全意识到本声明的法律后果由本人承担。
作者签名:日期:年月日学位论文版权使用授权书本学位论文作者完全了解学校有关保留、使用学位论文的规定,同意学校保留并向国家有关部门或机构送交论文的复印件和电子版,允许论文被查阅和借阅。
本人授权大学可以将本学位论文的全部或部分内容编入有关数据库进行检索,可以采用影印、缩印或扫描等复制手段保存和汇编本学位论文。
涉密论文按学校规定处理。
作者签名:日期:年月日导师签名:日期:年月日注意事项1.设计(论文)的内容包括:1)封面(按教务处制定的标准封面格式制作)2)原创性声明3)中文摘要(300字左右)、关键词4)外文摘要、关键词5)目次页(附件不统一编入)6)论文主体部分:引言(或绪论)、正文、结论7)参考文献8)致谢9)附录(对论文支持必要时)2.论文字数要求:理工类设计(论文)正文字数不少于1万字(不包括图纸、程序清单等),文科类论文正文字数不少于1.2万字。
神经网络在变压器故障诊断中的应用分析
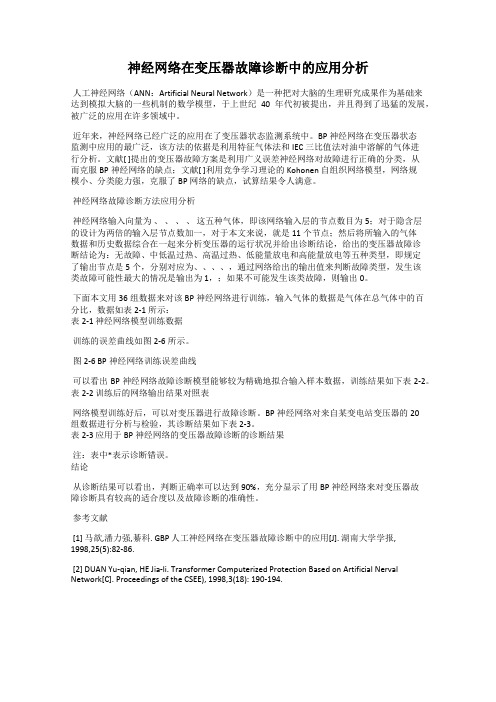
神经网络在变压器故障诊断中的应用分析人工神经网络(ANN:Artificial Neural Network)是一种把对大脑的生理研究成果作为基础来达到模拟大脑的一些机制的数学模型,于上世纪40年代初被提出,并且得到了迅猛的发展,被广泛的应用在许多领域中。
近年来,神经网络已经广泛的应用在了变压器状态监测系统中。
BP神经网络在变压器状态监测中应用的最广泛,该方法的依据是利用特征气体法和IEC三比值法对油中溶解的气体进行分析。
文献[ ]提出的变压器故障方案是利用广义误差神经网络对故障进行正确的分类,从而克服BP神经网络的缺点;文献[ ]利用竞争学习理论的Kohonen 自组织网络模型,网络规模小、分类能力强,克服了BP网络的缺点,试算结果令人满意。
神经网络故障诊断方法应用分析神经网络输入向量为、、、、这五种气体,即该网络输入层的节点数目为5;对于隐含层的设计为两倍的输入层节点数加一,对于本文来说,就是11个节点;然后将所输入的气体数据和历史数据综合在一起来分析变压器的运行状况并给出诊断结论,给出的变压器故障诊断结论为:无故障、中低温过热、高温过热、低能量放电和高能量放电等五种类型,即规定了输出节点是5个,分别对应为、、、、,通过网络给出的输出值来判断故障类型,发生该类故障可能性最大的情况是输出为1,;如果不可能发生该类故障,则输出0。
下面本文用36组数据来对该BP神经网络进行训练,输入气体的数据是气体在总气体中的百分比,数据如表2-1所示:表2-1 神经网络模型训练数据训练的误差曲线如图2-6所示。
图2-6 BP神经网络训练误差曲线可以看出BP神经网络故障诊断模型能够较为精确地拟合输入样本数据,训练结果如下表2-2。
表2-2 训练后的网络输出结果对照表网络模型训练好后,可以对变压器进行故障诊断。
BP神经网络对来自某变电站变压器的20组数据进行分析与检验,其诊断结果如下表2-3。
表2-3 应用于BP神经网络的变压器故障诊断的诊断结果注:表中*表示诊断错误。
基于BP人工神经网络的变压器故障诊断
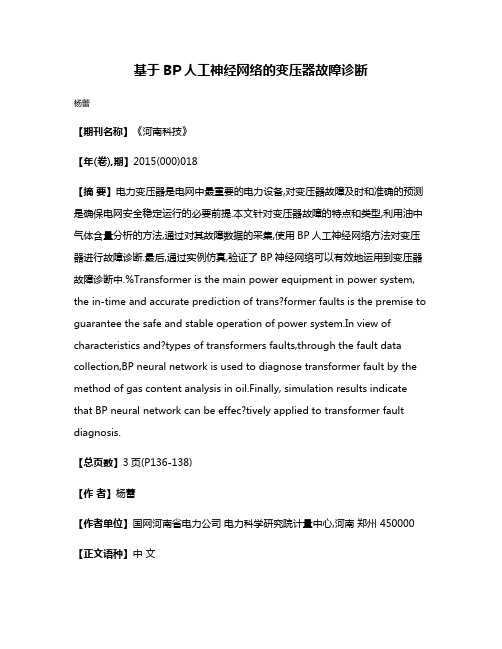
基于BP人工神经网络的变压器故障诊断杨蕾【期刊名称】《河南科技》【年(卷),期】2015(000)018【摘要】电力变压器是电网中最重要的电力设备,对变压器故障及时和准确的预测是确保电网安全稳定运行的必要前提.本文针对变压器故障的特点和类型,利用油中气体含量分析的方法,通过对其故障数据的采集,使用BP人工神经网络方法对变压器进行故障诊断.最后,通过实例仿真,验证了BP神经网络可以有效地运用到变压器故障诊断中.%Transformer is the main power equipment in power system, the in-time and accurate prediction of trans?former faults is the premise to guarantee the safe and stable operation of power system.In view of characteristics and?types of transformers faults,through the fault data collection,BP neural network is used to diagnose transformer fault by the method of gas content analysis in oil.Finally, simulation results indicate that BP neural network can be effec?tively applied to transformer fault diagnosis.【总页数】3页(P136-138)【作者】杨蕾【作者单位】国网河南省电力公司电力科学研究院计量中心,河南郑州 450000【正文语种】中文【中图分类】TP183;TM407【相关文献】1.基于BP人工神经网络的电力变压器故障诊断研究 [J], 谭子兵;黄秀超;钟建伟2.基于BP人工神经网络平潭海域赤潮叶绿素a浓度模型演算研究 [J], 许阳春; 张明峰; 苏玉萍; 洪颐; 苏金洙; 陈晶晶3.GBP人工神经网络在变压器故障诊断中的应用 [J], 马歆;潘力强;綦科4.基于BP人工神经网络喷射成形7055铝合金的本构模型 [J], 罗锐;曹赟;邱宇;崔树刚;周皓天;周易名;袁飞;张肖佩佩;程晓农5.基于BP人工神经网络的快速工程估算研究综述 [J], 韩思淼;黄剑因版权原因,仅展示原文概要,查看原文内容请购买。
- 1、下载文档前请自行甄别文档内容的完整性,平台不提供额外的编辑、内容补充、找答案等附加服务。
- 2、"仅部分预览"的文档,不可在线预览部分如存在完整性等问题,可反馈申请退款(可完整预览的文档不适用该条件!)。
- 3、如文档侵犯您的权益,请联系客服反馈,我们会尽快为您处理(人工客服工作时间:9:00-18:30)。
中文翻译利用人工神经网络和绕组的传递函数定位变压器的匝间短路故障Mohsen Faridi Ebrahim Rahimpour摘要:为了用自动化的程序定位在变压器绕组匝间故障,提出了一种叫人工神经网络(ANN)的方法。
为此,我们定制了一个特制的配电变压器,通过测试其绕组来验证该方法的可靠性。
这使这个实验设计合理,决定用任意两个相邻短路故障作为研究对象。
利用低电压脉冲(LVI)测试绕组在正常和故障的条件的频率响应。
从频率响应中提取特征数据用于检验和测试人工神经网络ANN。
结果表明,该方法能够准确在绕组匝间故障的位置。
关键词:匝间短路,变压器绕组;传递函数;神经网络。
变压器被认为是电网中最重要的设备之一。
变压器故障降低电力网络的可靠性,同时也对变压器的运行造成灾害性的损坏。
所以提高可靠性和诊断其故障将有效减少支损失。
为了在早期阶段发现变压器匝间短路故障,有必要开发合适的方法来检测它们。
在这之前,虽然有采用了很多方法,但显然还没有出现一种可靠的方法来检测它们的故障。
导言配电变压器的匝间故障是一种常见的故障。
到目前为止,已经有很多不同的方法被用来检测这种故障。
包括传递函数计算,[1 - 2],[3]有限元分析,小波分析[4],变压器电流序列分析,[5][6]和漏磁通密度计算。
在本文中,提出的神经网络(ANN)是一个基于传递函数的测量来检测匝间故障。
为此,定制一个特制配电变压器,变压器的绕组是人工模拟匝间短路故障。
然后测量故障之前和故障之后的传递函数,并用人工神经网络ANN定位匝间短路位置。
问题分析在配电变压器匝间故障是主要是由不规范操作和绕组热点温升过高引起。
这些可能导致导体的绝缘损坏。
因此在绕组匝间短路的故障主要可能是在高压绕组的外层或者高压绕组(HV)和低压绕组(LV)的顶部或者低部。
在配电变压器匝间故障定位的传统方法是应用一个测试电压绕组,然后逐渐增加其大小,直到绝缘缺陷的处变得碳化。
然后,缺陷位置可以观察到。
但是这种方法是破坏性的,并可能导致损害相邻匝。
因此有必要找到无损定位匝间短路故障的方法。
根据绕组匝间短路故障的模型参数的影响和传递函数的测量和分析来诊断匝间短路时合理的。
在这个研究上电压转移和转移导纳函数是用来定位匝间短路故障。
测试绕组的特性在这个工作电压转移和转移导纳函数是用来定位匝间短路故障图1显示了专门准备的绕组20/0.4KV,原理图50 kva变压器用于进行实验测试。
层类型高压绕组由15层。
到第31个接头,更有可能变成匝间短路故障。
因为短路选取两个接头产生匝间短路缺陷。
考虑到选取的邻近两个匝间的距离,在查查寻过程中找到84匝人工匝间短路模型。
图1检测电路和测量系统两个不同的频率响应测量的目的故障定位。
图2显示了测量的测试电路转移导纳函数。
在这个电路低压(LV)绕组短路的和中性的高压绕组接地通过50Ω阻力。
一连串的低压脉冲用于激励高压侧线圈。
高压侧电压及其中性电流测量在时间域分别作为输入和输出信号。
利用傅里叶变换计算测量信号的导纳来得出传递函数。
Io和Vi分别是接地电流和外加电压。
图2.测试导纳的电路图图3.测量转移电压的电路图图4.试验设备在图3的测试电路测量电压传递函数。
在这个电路中,接线柱的高压和低压绕组接地直接和脉冲应用于高压终端。
电压转移函数计算使用的傅里叶变换测量高压和低压电压:V0和Vi是对低压绕组LV的电压传输和高压绕组的应用。
应用低压脉冲有10个ns倍上升。
输入和输出信号使用数字示波器测量500 msam /秒和105样本/记录。
完整的测试电路安排图4所示。
在图5中,转移导纳的测量输入和输出信号和传输电压测试电路圈所示的正常状况。
测量信号在测试电路第2和第4匝,第26匝和30匝的短路转身是短路如图6所示。
计算传递函数图6所示的信号提出了图7到10。
图,5正常情况下的绕组的信号输出测量第2和第4之间还有第26和第30匝之间的短路故障情况下的输入和输出信号图7. 测量在正常运行时第26和第30匝之间的短路故障的传递导纳图8. 测量在正常运行时第2和第4匝之间的短路故障的传递导纳图9. 绕组的传递电压在正常情况下和第26 和第30匝之间的短路故障。
图10. 绕组的传递电压在正常情况下和第2 和第4匝之间的短路故障。
人工神经网络智能算法可以学习之后一系列的训练模式和分类出新模式。
为了缺陷定位,两个相似的人工神经网络的具有反向学习算法得到应用。
16主导极点的振幅再计算电压转移和转移导纳函数作为这两个网络作为输入。
模拟匝间故障的结果,被分为两个组,一组用于训练网络,另一组用于测试。
这样,42匝间故障信息被用来测试ANN和其他42个错误被用来测试它。
所以,在训练阶段,两个42×16和42×1矩阵每个网络的应用作为输入和预期的输出。
用试错法,人工神经网络结构包括16,4和1神经元的输入,隐层和输出层,分别独立的。
得出人功智能网络(ANN)故障能准确合理的定位匝间短路故障。
图11.用ANN神经网络测试转移电压的结果图12.用ANN神经网络测试传递导纳的结果用人工神经网络定位图11和图12显示的输出ANN的输入训练数据集和测试数据集分别传输电压和传输导纳函数。
可以看到,ANN学习模式和也能成功定位故障。
最大、最小和平均误差测试数据传输电压输入1.949,0.0065,0。
分别为825。
这些值在应用的情况传输导纳函数的输入是2.352,0.2502,0。
分别为7302。
也在这两种情况下,训练网络能够准确定位缺陷在95%缺陷位置的测试数据集。
结论在这篇文章中,,提出了基于神经网络来定位匝间短路故障得方法。
这个网络使用特征值法提取传输电压和传输导纳函数的数据。
利用人工模拟变压器匝间短路故障的试验数据来训练ANN神经网络的定位故障。
结果由此证明利用神经网络ANN定位匝间的可行性。
Localization of Turn-to-Turn Fault in TransformersUsing Artificial Neural Networks and WindingTransfer FunctionMohsen FaridiIslamic Azad University, Khodabandeh BranchKhodabandeh, IranEbrahim RahimpourABB AG, Power TransformersBad Honnef, GermanyAbstract—To automate the procedure of localizing turn-to-turn faults in transformers windings, a method is proposed by employing of Artificial Neural Networks (ANN). For this purpose, a specially made distribution transformer winding is used as a test object to approve the capability of proposed method. This winding is appropriately designed to perform short circuit faults between any two desired adjacent turns. Then the frequency response of winding in both healthy and faulty conditions is measured using the Low Voltage Impulse (LVI) test. Extracted features from frequency responses are used to train and test the proposed ANN. The results show that this method is able to determine the location of turn-to-turn fault in winding.Keywords-component;Turn-to-Turn Fault;TransformerWinding; Transfer Function; Neural NetworkI I NTRODUCTIONTransformers are supposed to be one of the most important equipments in power networks. Transformer failures not only reduce these networks reliability, but also cause catastrophic damages to their active parts. So any efforts to increase their reliability and diagnosing their faults would effectively reduce expenditures. Regarding to importance of recognition and detection of transformer internal faults in their early stages of appearance, it is necessary to develop suitable methods to detect them. Many works already have been performed in this context before, but clearly it has not been introduced any reliable method to detect their faults yet.Turn-to-turn fault is a common cause of distribution transformers failures. Up to now, many different methods have been used to detect this fault such as transfer function calculation [1-2], finite element analysis [3], wavelet analysis [4], transformer current sequence analysis [5] and leakage factor calculation method [6]. In this paper, an Artificial Neural Network (ANN) based on transfer function measurement is proposed to detect turn-to-turn faults. For this purpose, a special made winding of a distribution transformer is manufactured to simulate artificial turn-to-turn faults. Thendifferent transfer functions of this winding are measured before and after implementing defects and then their extracted features applied to ANN to localize defect site.II.P ROBLEM D EFINITIONTurn-to-turn faults in distribution transformers are caused mainly by careless transportation and excessive temperature rise in hot spots of windings. These might lead to damages to conductor’s insulation. Therefore the main probable sites for turn-to-turn faults in windings are those turns which are located in outer layers of High Voltage (HV) winding or in top or bottom of both Low V oltage (LV) and HV windings.The conventional method for turn-to-turn fault localization in distribution transformers is to apply a test voltage to their windings and then increase its magnitude gradually until the insulation of defect site becomes carbonized. Afterwards, defect location could be discriminated by visualinspections. But this method is destructive and may result in damages to adjacent turns. Therefore it is favorable to find a non-destructive method to localize such defects.Due to the affect of turn-to-turn faults in windings model parameters, transfer function measurement and analysis is supposed to be suitable for their detection and localization. In this work the transfer voltage and the transfer admittance functions are employed to localize turn-to-turn fault.III.T EST W INDING C HARACHTERISTICSFig. 1 shows the schematic diagram of specially prepared winding of 20/0.4KV, 50KV A transformer which is used to perform experimental tests. The layer type HV winding consists of 15 layers. Up to 31 joints are extracted from those turns which are more likely to be subjected to turn-to-turn faults. By short circuiting two extracted joints turn-to-turn defects are generated. Considering the proximity of those turnswhich are sampled out, totally 84 states of artificial turn-to-turn defects are simulated in this research work.Figure 1.Schematic diagram of test winding turs and joints.IV. T EST C IRCUITS AND M EASURING S YSTEMTwo different frequency responses are measured for the aim of fault localization. Fig. 2 shows the test circuit for measuring transfer admittance function. In this circuit the Low Voltage (LV) winding is short circuited and the neutral of HV winding is grounde d via a 50Ω resistance. A train of low voltage impulses is applied to HV terminal to excite the winding. The HV terminal voltage and its neutral currents are measured in time domain as input and output signals respectively. The admittance transfer function is calculated using Fourier Transforms of measured signals as follows:FFT ( I o)TFAdmitt anceFFT (V i)Where I o and V i are the ground current of HV winding and applied voltage respectively.In Fig. 3 the test circuit for measuring the transfer voltage function is shown. In this circuit, the neutral terminal of both HV and LV windings is grounded directly and the impulse is applied to HV terminal. The transfer voltage function is calculated using the Fourier Transforms of measured HV and LV voltages:TFFFT (V o)TransferVoltageFFT (V i)Where V o and V i are the transferred voltage to LV winding and applied voltage to HV winding respectively.JJJ.TEST WINDING CHARACHTERISTICSFig. 1 shows the schematic diagram of specially prepared winding of 20/0.4KV, 50KVA transformer which is used to perform experimental tests. The layer type HV winding consists of 15 layers. Up to 31 joints are extracted from those turns which are more likely to be subjected to turn-to-turn faults. By short circuiting two extracted joints turn-to-turn defects are generated. Considering the proximity of those turnsFigure 2.Test circuit diagram for transfer admittance function measurement.Figure 3.Test circuit diagram for transfer voltage function measurement.The applied low voltage impulses have 10ns rise times. Input and output signals are measured using a digital oscilloscope with 500Msam/sec and 105 samples per record. The complete test circuit arrangement is shown in Fig. 4.V.M EASUREMENTS R ESULTSIn Fig. 5, the measured input and output signals of both transfer admittance and transfer voltage test circuits in healthy condition of winding are shown. The measured signals in both test circuits when the 2nd and 4th turns as well as 26th and 30th turns are short circuited are depicted in Fig. 6. The calculated transfer functions of signal which are shown in Fig. 6 are presented in Fig. 7 through 10.Figure 5.Measured input and ouput signals from winding healthy condition.Figure 6. Measured input and ouput signals when 2nd and 4th turns as well as 26th and 30th turns are short circuitted.Figure 7. Winding transfer admittance functions in healty condition and when 26th and 30th turns short circuitted.Figure 8. Winding transfer admittance functions in healty condition and when 2nd and 4th turns short circuitted.Figure 9. Winding transfer voltage functions in healty condition and when 26th and 30th turns short circuitted.VI. D EFECT L OCALIZATION U SING ANNANNs are intelligent algorithms which could learn a set of training patterns and afterwards classify new patterns. For defect localization two similar ANNs with back propagation learning algorithm are used. The amplitudes of 16 dominant poles of calculated transfer voltage and transfer admittance functions are applied to these two networks as inputs. The results obtained from simulated turn-to-turn faults, were divided to two separate groups, one for training the networks and the other for testing them. In this way, 42 turn-to-turn faultsinformation were used to train ANN and the other 42 faults were used to test it. So, in training phase, two 42×16 and 42×1 matrices were applied as input and desired outputs of each network. Using a try and error method, ANNs structures which include 16, 4 and 1 neurons in input, hidden and output layers respectively, found to be suitable for defect localization.Fig. 11 and Fig. 12 show the output of ANN for training data set of inputs as well as test data set for transfer voltage and transfer admittance functions respectively. As can be seen, the ANN was capable to learn the patterns and could successfully localize the site of defects too.The maximum, minimum and average error for test data in case of transfer voltage inputs are 1.949, 0.0065 and 0.825 respectively. These values in the case of applying inputs of transfer admittance function are 2.352, 0.2502 and 0.7302 respectively. Also in both cases the trained networks were able to localize defects accurately in 95% defect locations of test data setFigure 11. ANN test results for transfer voltage functions.Figure 12. ANN test results for transfer admittance functions.VII. CONCLUSIONIn this paper, a turn-to-turn fault localization method basedon neural network was proposed. This network uses the features extracted from both transfer voltage and transferadmittance functions. The feasibility of fault localization oftrained ANN was tested using the experimental data whichwere obtained from measurements on artificial defectssimulated on a specially manufactured winding. Resultsapprove the ability of proposed ANN in this context.ACKNOWLEDGMENTThe authors would like to thank Iran-Transfo Co. for itssupports and collaborations in tests and measurements.REFERENCES[1] E. Rahimpour, and D. Gorzin, “A New Method For Comparing theTransfer Function of Transformers in order to Detect the Location andAmount of Winding Faults. ”, Electrical Engineering, V ol.88, 2006, pp.411-416.[2] E. Rahimpour, J. Christian, , K. Feser, and H. Mohseni, “Modellierungder Transformatorwicklung zur Berechnung der Übertragungs funktionfür die Diagnose von Transformatoren. ”, Elektrie, No.54/1-2, 2000, pp.18-30.[3] H. Wang, and K. L. Bulter, “Finite Element Analysis of InternalWinding Faults in Distribution Transformers”, IEEE Trans. on PowerDel., Vol.16, No.3, 2001, pp. 422-428.[4] M. R. Rao, and B. P. Singh, “Detection and Localizat ion of InterturnFault in the HV Winding of a Power Transformer Using Wavelets. ”IEEE Trans. on Dielect. and Elect. Insul., V ol.8, No.4, 2001, pp. 652-657.[5] G. Diaz, and A. Barbon, “Currents Sequence Analysis of a Transformer五分钟搞定5000字毕业论文外文翻译,你想要的工具都在这里!在科研过程中阅读翻译外文文献是一个非常重要的环节,许多领域高水平的文献都是外文文献,借鉴一些外文文献翻译的经验是非常必要的。