神经网络和遗传算法的模糊系统的自动设计论文中英文资料对照外文翻译
传感器技术论文中英文对照资料外文翻译文献
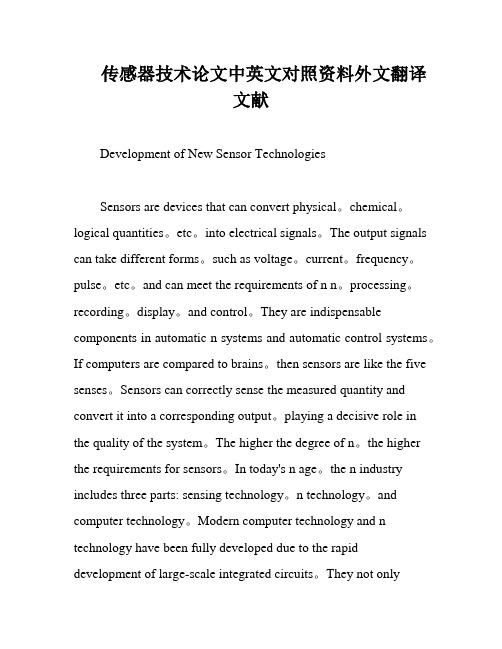
传感器技术论文中英文对照资料外文翻译文献Development of New Sensor TechnologiesSensors are devices that can convert physical。
chemical。
logical quantities。
etc。
into electrical signals。
The output signals can take different forms。
such as voltage。
current。
frequency。
pulse。
etc。
and can meet the requirements of n n。
processing。
recording。
display。
and control。
They are indispensable components in automatic n systems and automatic control systems。
If computers are compared to brains。
then sensors are like the five senses。
Sensors can correctly sense the measured quantity and convert it into a corresponding output。
playing a decisive role in the quality of the system。
The higher the degree of n。
the higher the requirements for sensors。
In today's n age。
the n industry includes three parts: sensing technology。
n technology。
and computer technology。
《神经网络与模糊系统》课程论文

《神经网络与模糊系统》课程论文题目基于深度学习的图像特征提取院〔系〕电子工程学院学号xxx专业智能信息处理年级xxx学生某某xxx指导教师xxxx2014 年12 月31日基于深度学习的图像特征提取摘要:大数据时代的降临,为深度学习理论的开展创造了良好的条件。
本文介绍了深度学习的开展背景,主要讨论了深度学习中的自编码的方法,对自编码方法实现仿真应用,在以后能应用到SAR图像上进展自动特征提取,最后阐述该理论的目前遇到的困难。
关键词:深度学习autoencoder convolution pooling一引言深度学习是机器学习研究中的一个新的领域,其核心思想在于模拟人脑的层级抽象结构,通过无监视的方式分析大规模数据,开掘大数据中蕴藏的有价值信息。
深度学习应大数据而生,给大数据提供了一个深度思考的大脑。
自2006年以来,深度学习在学术界持续升温。
斯坦福大学、纽约大学、加拿大蒙特利尔大学等成为研究深度学习的重镇。
2010年,美国国防部DARPA计划首次资助深度学习项目,参与方有斯坦福大学、纽约大学和NEC美国研究院。
支持深度学习的一个重要依据,就是脑神经系统确实具有丰富的层次结构。
一个最著名的例子就是Hubel-Wiesel模型,由于揭示了视觉神经的机理而曾获得诺贝尔医学与生理学奖。
除了仿生学的角度,目前深度学习的理论研究还根本处于起步阶段,但在应用领域已显现出巨大能量。
2011年以来,微软研究院和Google的语音识别研究人员先后采用DNN技术降低语音识别错误率20%~30%,是语音识别领域十多年来最大的突破性进展。
2012年,DNN技术在图像识别领域取得惊人的效果,在ImageNet评测上将错误率从26%降低到15%。
在这一年,DNN还被应用于制药公司的Druge Activity问题,并获得世界最好成绩,这一重要成果被《纽约时报》报道。
今天Google、微软、百度等知名的拥有大数据的高科技公司争相投入资源,占领深度学习的技术制高点,正是因为它们都看到了在大数据时代,更加复杂且更加强大的深度模型能深刻揭示海量数据里所承载的复杂而丰富的信息,并对未来或未知事件做更精准的。
模糊逻辑中英文对照外文翻译文献

模糊逻辑中英文对照外文翻译文献(文档含英文原文和中文翻译)译文:模糊逻辑欢迎进入模糊逻辑的精彩世界,你可以用新科学有力地实现一些东西。
在你的技术与管理技能的领域中,增加了基于模糊逻辑分析和控制的能力,你就可以实现除此之外的其他人与物无法做到的事情。
以下就是模糊逻辑的基础知识:随着系统复杂性的增加,对系统精确的阐述变得越来越难,最终变得无法阐述。
于是,终于到达了一个只有靠人类发明的模糊逻辑才能解决的复杂程度。
模糊逻辑用于系统的分析和控制设计,因为它可以缩短工程发展的时间;有时,在一些高度复杂的系统中,这是唯一可以解决问题的方法。
虽然,我们经常认为控制是和控制一个物理系统有关系的,但是,扎德博士最初设计这个概念的时候本意并非如此。
实际上,模糊逻辑适用于生物,经济,市场营销和其他大而复杂的系统。
模糊这个词最早出现在扎德博士于1962年在一个工程学权威刊物上发表论文中。
1963年,扎德博士成为加州大学伯克利分校电气工程学院院长。
那就意味着达到了电气工程领域的顶尖。
扎德博士认为模糊控制是那时的热点,不是以后的热点,更不应该受到轻视。
目前已经有了成千上万基于模糊逻辑的产品,从聚焦照相机到可以根据衣服脏度自我控制洗涤方式的洗衣机等。
如果你在美国,你会很容易找到基于模糊的系统。
想一想,当通用汽车告诉大众,她生产的汽车其反刹车是根据模糊逻辑而造成的时候,那会对其销售造成多么大的影响。
以下的章节包括:1)介绍处于商业等各个领域的人们他们如果从模糊逻辑演变而来的利益中得到好处,以及帮助大家理解模糊逻辑是怎么工作的。
2)提供模糊逻辑是怎么工作的一种指导,只有人们知道了这一点,才能运用它用于做一些对自己有利的事情。
这本书就是一个指导,因此尽管你不是电气领域的专家,你也可以运用模糊逻辑。
需要指出的是有一些针对模糊逻辑的相反观点和批评。
一个人应该学会观察反面的各个观点,从而得出自己的观点。
我个人认为,身为被表扬以及因写关于模糊逻辑论文而受到赞赏的作者,他会认为,在这个领域中的这种批评有点过激。
遗传算法中英文对照外文翻译文献
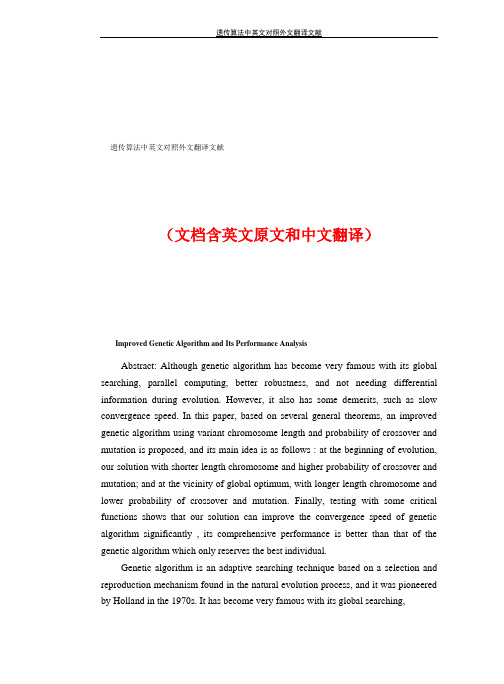
遗传算法中英文对照外文翻译文献遗传算法中英文对照外文翻译文献(文档含英文原文和中文翻译)Improved Genetic Algorithm and Its Performance AnalysisAbstract: Although genetic algorithm has become very famous with its global searching, parallel computing, better robustness, and not needing differential information during evolution. However, it also has some demerits, such as slow convergence speed. In this paper, based on several general theorems, an improved genetic algorithm using variant chromosome length and probability of crossover and mutation is proposed, and its main idea is as follows : at the beginning of evolution, our solution with shorter length chromosome and higher probability of crossover and mutation; and at the vicinity of global optimum, with longer length chromosome and lower probability of crossover and mutation. Finally, testing with some critical functions shows that our solution can improve the convergence speed of genetic algorithm significantly , its comprehensive performance is better than that of the genetic algorithm which only reserves the best individual.Genetic algorithm is an adaptive searching technique based on a selection and reproduction mechanism found in the natural evolution process, and it was pioneered by Holland in the 1970s. It has become very famous with its global searching,________________________________ 遗传算法中英文对照外文翻译文献 ________________________________ parallel computing, better robustness, and not needing differential information during evolution. However, it also has some demerits, such as poor local searching, premature converging, as well as slow convergence speed. In recent years, these problems have been studied.In this paper, an improved genetic algorithm with variant chromosome length andvariant probability is proposed. Testing with some critical functions shows that it can improve the convergence speed significantly, and its comprehensive performance is better than that of the genetic algorithm which only reserves the best individual.In section 1, our new approach is proposed. Through optimization examples, insection 2, the efficiency of our algorithm is compared with the genetic algorithm which only reserves the best individual. And section 3 gives out the conclusions. Finally, some proofs of relative theorems are collected and presented in appendix.1 Description of the algorithm1.1 Some theoremsBefore proposing our approach, we give out some general theorems (see appendix)as follows: Let us assume there is just one variable (multivariable can be divided into many sections, one section for one variable) x £ [ a, b ] , x £ R, and chromosome length with binary encoding is 1.Theorem 1 Minimal resolution of chromosome isb 一 a2l — 1Theorem 3 Mathematical expectation Ec(x) of chromosome searching stepwith one-point crossover iswhere Pc is the probability of crossover.Theorem 4 Mathematical expectation Em ( x ) of chromosome searching step with bit mutation isE m ( x ) = ( b- a) P m 遗传算法中英文对照外文翻译文献Theorem 2 wi = 2l -1 2 i -1 Weight value of the ith bit of chromosome is(i = 1,2,・・・l )E *)= P c1.2 Mechanism of algorithmDuring evolutionary process, we presume that value domains of variable are fixed, and the probability of crossover is a constant, so from Theorem 1 and 3, we know that the longer chromosome length is, the smaller searching step of chromosome, and the higher resolution; and vice versa. Meanwhile, crossover probability is in direct proportion to searching step. From Theorem 4, changing the length of chromosome does not affect searching step of mutation, while mutation probability is also in direct proportion to searching step.At the beginning of evolution, shorter length chromosome( can be too shorter, otherwise it is harmful to population diversity ) and higher probability of crossover and mutation increases searching step, which can carry out greater domain searching, and avoid falling into local optimum. While at the vicinity of global optimum, longer length chromosome and lower probability of crossover and mutation will decrease searching step, and longer length chromosome also improves resolution of mutation, which avoid wandering near the global optimum, and speeds up algorithm converging.Finally, it should be pointed out that chromosome length changing keeps individual fitness unchanged, hence it does not affect select ion ( with roulette wheel selection) .2.3 Description of the algorithmOwing to basic genetic algorithm not converging on the global optimum, while the genetic algorithm which reserves the best individual at current generation can, our approach adopts this policy. During evolutionary process, we track cumulative average of individual average fitness up to current generation. It is written as1 X G x(t)= G f vg (t)t=1where G is the current evolutionary generation, 'avg is individual average fitness.When the cumulative average fitness increases to k times ( k> 1, k £ R) of initial individual average fitness, we change chromosome length to m times ( m is a positive integer ) of itself , and reduce probability of crossover and mutation, which_______________________________ 遗传算法中英文对照外文翻译文献________________________________can improve individual resolution and reduce searching step, and speed up algorithm converging. The procedure is as follows:Step 1 Initialize population, and calculate individual average fitness f avg0, and set change parameter flag. Flag equal to 1.Step 2 Based on reserving the best individual of current generation, carry out selection, regeneration, crossover and mutation, and calculate cumulative average of individual average fitness up to current generation 'avg ;f avgStep 3 If f vgg0 三k and Flag equals 1, increase chromosome length to m times of itself, and reduce probability of crossover and mutation, and set Flag equal to 0; otherwise continue evolving.Step 4 If end condition is satisfied, stop; otherwise go to Step 2.2 Test and analysisWe adopt the following two critical functions to test our approach, and compare it with the genetic algorithm which only reserves the best individual:sin 2 弋 x2 + y2 - 0.5 [1 + 0.01( 2 + y 2)]x, y G [-5,5]f (x, y) = 4 - (x2 + 2y2 - 0.3cos(3n x) - 0.4cos(4n y))x, y G [-1,1]22. 1 Analysis of convergenceDuring function testing, we carry out the following policies: roulette wheel select ion, one point crossover, bit mutation, and the size of population is 60, l is chromosome length, Pc and Pm are the probability of crossover and mutation respectively. And we randomly select four genetic algorithms reserving best individual with various fixed chromosome length and probability of crossover and mutation to compare with our approach. Tab. 1 gives the average converging generation in 100 tests.In our approach, we adopt initial parameter l0= 10, Pc0= 0.3, Pm0= 0.1 and k= 1.2, when changing parameter condition is satisfied, we adjust parameters to l= 30, Pc= 0.1, Pm= 0.01.From Tab. 1, we know that our approach improves convergence speed of genetic algorithm significantly and it accords with above analysis.2.2 Analysis of online and offline performanceQuantitative evaluation methods of genetic algorithm are proposed by Dejong, including online and offline performance. The former tests dynamic performance; and the latter evaluates convergence performance. To better analyze online and offline performance of testing function, w e multiply fitness of each individual by 10, and we give a curve of 4 000 and 1 000 generations for fl and f2, respectively.(a) onlineFig. 1 Online and offline performance of fl(a) online (b) onlineFig. 2 Online and offline performance of f2From Fig. 1 and Fig. 2, we know that online performance of our approach is just little worse than that of the fourth case, but it is much better than that of the second, third and fifth case, whose online performances are nearly the same. At the same time, offline performance of our approach is better than that of other four cases.3 ConclusionIn this paper, based on some general theorems, an improved genetic algorithmusing variant chromosome length and probability of crossover and mutation is proposed. Testing with some critical functions shows that it can improve convergence speed of genetic algorithm significantly, and its comprehensive performance is better than that of the genetic algorithm which only reserves the best individual.AppendixWith the supposed conditions of section 1, we know that the validation of Theorem 1 and Theorem 2 are obvious.Theorem 3 Mathematical expectation Ec(x) of chromosome searching step with one point crossover isb - a PEc(x) = 21 cwhere Pc is the probability of crossover.Proof As shown in Fig. A1, we assume that crossover happens on the kth locus, i. e. parent,s locus from k to l do not change, and genes on the locus from 1 to k are exchanged.During crossover, change probability of genes on the locus from 1 to k is 2 (“1” to “0” or “0” to “1”). So, after crossover, mathematical expectation of chromosome searching step on locus from 1 to k is1 chromosome is equal, namely l Pc. Therefore, after crossover, mathematical expectation of chromosome searching step isE (x ) = T 1 -• P • E (x ) c l c ckk =1Substituting Eq. ( A1) into Eq. ( A2) , we obtain 尸 11 b - a p b - a p • (b - a ) 1 E (x ) = T • P • — •• (2k -1) = 7c • • [(2z -1) ― l ] = ——— (1 一 )c l c 2 21 — 121 21 — 1 21 21 —1 k =1 lb - a _where l is large,-——-口 0, so E (x ) 口 -——P2l — 1 c 21 c 遗传算法中英文对照外文翻译文献 厂 / 、 T 1 T 1 b — a - 1E (x )="—w ="一• ---------- • 2 j -1 二 •ck2 j 2 21 -1 2j =1 j =1 Furthermore, probability of taking • (2k -1) place crossover on each locus ofFig. A1 One point crossoverTheorem 4 Mathematical expectation E m(")of chromosome searching step with bit mutation E m (x)—(b a)* P m, where Pm is the probability of mutation.Proof Mutation probability of genes on each locus of chromosome is equal, say Pm, therefore, mathematical expectation of mutation searching step is一i i - b —a b b- aE (x) = P w = P•—a«2i-1 = P•—a q2,-1)= (b- a) •m m i m 21 -1 m 2 i -1 mi=1 i=1一种新的改进遗传算法及其性能分析摘要:虽然遗传算法以其全局搜索、并行计算、更好的健壮性以及在进化过程中不需要求导而著称,但是它仍然有一定的缺陷,比如收敛速度慢。
蜂窝系统切换技术论文中英文资料对照外文翻译文献

蜂窝系统切换技术论文中英文资料对照外文翻译文献一、英文原文:Handoff in Cellular SystemsNishith D. Tripathi, NortelJeffrey H. Reed and Hugh F. VanLandinghamMPRG, Virginia TechCellular SystemDeployment ScenariosThe radio propagation environment and related handoff challenges are different in different cellular structures. A handoff algorithm with fixed parameters cannot perform well in different system environments. Specific characteristics of the communication systems should be taken into account while designing handoff algorithms. Several basic cellular structures (e.g., macrocells, microcells, and overlay systems) and special architectures (e.g., underlays, multichannel bandwidth systems,and evolutionary architectures) are described next. Integrated cordless and cellular systems, integrated cellular systems, and integrated terrestrial and satellite systems are also described.MacrocellsMacrocell radii are in several kilometers. Due to the low cellcrossing rate, centralized handoff is possible despite the large number of MSs the MSC has to manage. The signal quality in the uplink and downlink is approximately the same. The transition region between the BSs is large; handoff schemes should allow some delay to avoid flip-flopping. However, the delay should beshort enough to preserve the signal quality because the interference increases as the MS penetrates the new cell. This cell penetration is called cell dragging. Macrocells have relatively gentle path loss characteristics . The averaging interval (i.e., the time period used to average the signal strength variations) should be long enough to get rid of fadingfluctuations. First- and second-generation cellular systems provide wide-area coverage even in cities using macrocells .Typically, a BS transceiver in a macrocell transmits high output power with the antenna mounted several meters high on a tower to illuminate a large area.MicrocellsSome capacity improvement techniques (e.g., larger bandwidths, improved methods for speech coding, channel coding,and modulation) will not be sufficient to satisfy the required service demand. The use of microcells is considered the single most effective means of increasing the capacity of cellular systems.Microcells increase capacity, but radio resource management becomes more difficult. Microcells can be classified as one-, two-, or threedimensional,depending on whether they are along a road or a highway, covering an area such as a number of adjacent roads,or located in multilevel buildings, respectively . Microcells can be classified as hot spots (service areas with a higher traffic density or areas that are covered poorly), downtown clustered microcells (contiguous areas serving pedestrians and mobiles), and in-building 3-D cells (serving office buildings and pedestrians).Typically, a BS transceiver in a microcell transmits low output power with the antenna mounted at lamppost level (approximately 5 m above ground).The MS also transmits low power, which leads to longer battery life. Since BS antennas have lower heights compared to the surrounding buildings, RF signals propagate mostly along the streets.The antenna may cover 100–200 m in each street direction, serving a few city blocks. This propagation environment has low time dispersion, which allows high data rates.Microcells are more sensitive to the traffic and interference than macrocells due to short-term variations (e.g., traffic and interferencevariations),medium/long-term alterations (e.g., new buildings), and incremental growth of the radio network (e.g., new BSs) . The number of handoffs per cell is increased by an order of magnitude, and the time available to make a handoff is decreased. Using an umbrella cell is one way to reduce the handoff rate. Due to the increase in the microcell boundary crossings and expected high trafficloads, a higher degree of decentralization of the handoff process becomes necessary.Microcells encounter a propagation phenomenon called the corner effect. The corner effect is characterized by a sudden large drop (e.g., 20–30 dB) in signal strength (e.g., at 10–20 m distance) when a mobile turns around a corner.The corner effect is due to the loss of the line of sight (LOS) component from the serving BS to the MS. The corner effect demands a faster handoff and can change the signal quality very fast. The corner effect is hard to predict. A long measurement averaging interval is not desirable due to the corner effect. Moving obstacles can temporarily hinder the path between a BS and an MS,which resembles the corner effect. Reference studies the properties of symmetrical cell plans in a Manhattan-type environment. Cell plans affect signal-to-interference ratio (SIR) performance in the uplink and downlink significantly. Symmetrical cell plans have four nearest co-channel BSs located at the same distance. Such cell plans can be classified into half-square (HS), full-square (FS), and rectangular (R) cell plans. These cell plans are described next.Half-Square Cell Plan—This cell planplaces BSs with omnidirectional antennas at each intersection, and each BS covers half a block in all four directions. This cell plan avoids the street corner effect and provides the highest capacity. This cell plan has only LOS handoffs. Figure 2 shows an example of a half-square cell plan in a microcellular system.Full-Square Cell Plan —There is a BSwith an omnidirectional antenna located at every other intersection, and each BS coversa block in all four directions. It is possible for an MS to experience the street corner effect for this cell plan. The FS cell plan can have LOS or NLOS handoffs. Figure 3 shows an example of a fullsquare cell plan in a microcellular system.Rectangular Cell Plan —Each BS covers a fraction of either a horizontal or vertical street with the BS located in the middle of the cell. This cell plan can easily be adapted to market penetration. Fewer BSs with high transmit power can be used initially. As user density increases, new BSs can be added with reduced transmit power from appropriate BSs.The street corner effect is possible for this cell plan. The R cell plan can have LOS or NLOS handoffs. Figure 4 shows an example of a rectangular cell plan in a microcellular system. Macrocell/Microcell Overlays Congestion of certain microcells, the lack of service of microcells in some areas, and high speed of some users are some reasons for higher handoff rates and signaling load for microcells. To alleviate some of these problems, a mixed-cell architecture (called an overlay/underlay system) consisting of largesizemacrocells (called umbrella cells or overlay cells) and small-size microcells(called underlay cells) can be used. Figure 5 illustrates an overlay system.The macrocell/microcell overlay architecture provides a balance between maximizing the number of users per unit area and minimizing the network control load associated with handoff. Macrocells provide wide-area coverage beyond microcell service areas and ensure better intercell handoff.Microcells provide capacity due to greater frequency reuse and cover areas with high traffic density (called hot spots). Examples of hot spots include an airport,a railway station, or a parking lot. In less congested areas (e.g., areas beyond a city center or outside the main streets of a city) traffic demand is not very high, and macrocells can provide adequate coverage in such areas. Macrocells also serve highspeed MSs and the areas not covered by microcells (e.g., dueto lack of channels or the MS being out of the microcell range). Also, after the microcellular system is used to its fullest extent, the overflow traffic can be routed to macrocells.One of the important issues for the overlay/underlay system is the determination of optimum distribution of channels in the macrocells and microcells.Reference evaluates four approaches to sharing the available spectrum between the two tiers. Approach 1 uses TDMA for microcell and CDMA for macrocell. Approach 2 uses CDMA for microcell and TDMA for macrocell. Approach 3 uses TDMA in both tiers, while approach 4 uses orthogonal frequency channels in both tiers.The overlay/underlay system has several advantages over a pure microcell system:• The BSs are required only in high traffic load areas. Since it is not necessary to cover the whole service area with microcells,infrastructure costs are saved.• The number of ha ndoffs in an overlay system is much less than in a microcell system because fast-moving vehicles can be connected to the overlay macrocell.• Both calling from an MS and location registration can easily be done through the microcell system.There are several classes of umbrella cells. In one class, orthogonal channels are distributed between microcells and macrocells.In another class, microcells use channels that are temporarily unused by macrocells. In yet another class,microcells reuse the channels already assigned to macrocells and use slightly higher transmit power levels to counteract the interference from the macrocells.Within the overlay/underlay system environment, four types of handovers need to be managed[19]: microcell to microcell, microcell to macrocell, macrocell to macrocell, and macrocell to microcell.Reference describes combined cell splitting and overlaying. Reuse of channels in the two cells is done by establishing an overlaid small cell served by the same cell site as the large cell. Small cells reuse the split cell’s channels because of the large distance between the split cell and the small inner cell, while the large cell cannot reuse these channels. Overlaid cells are approximately 50 percent more spectrally efficient than segmenting (the process of distributing the channels among the small- and largesize cells to avoid interference).A practical approach for implementation of a microcell system overlaid with an existing macrocell system is proposed in . This reference introduces channel segregation (a self-organized dynamic channel assignment)and automatic transmit power control to obviate the need to design channel assignment and transmit power control for the microcell system. The available channels are reused automatically between microcells and macrocells. A slight increase of transmit power for the microcell system compensates for the macrocell-to-microcell interference.Simulation results indicate that the local traffic is accommodated by the microcells laid under macrocells without any significant channel management effort. The methodology of the Global System for Mobile Communications (GSM)-based system is extended to the macrocell/microcell overlay system in. The use of random frequency hopping and adaptive frequency planning is recommended,and different issues related to handoff and frequency planning for an overlay system are discussed. Four strategies are designed to determine a suitable cell for a user for an overlay system. Two strategies are based on the dwell time (the time for which a call can be maintained in a cell without handoff), and the other two strategies are based on user speed estimation. A speed estimation technique based on dwell times is also proposed.A CDMA cellular system can provide full connectivity between the microcells and the overlaying macrocells without capacity degradation. Reference analyzes several factors that determine the cell size, the soft handoff (SHO) zone, and the capacity of the cell clusters. Several techniques for overlay-underlay cell clustering are also outlined. Application of CDMA to microcell/macrocell overlay have the following major advantages:• A heterogeneous environment can be illuminated uniformly by using a distributed antenna (with a series of radiators with different propagation delays) while still maintaining a high-quality signal.• SHO obviates the need for complex frequency planning.Reference studies the feasibility of a CDMA overlay that can share the 1850–1990 MHz personal communications services (PCS) band with existing microwave signals (transmitted by utility companies and state agencies). The results of several field tests demonstrate the application of such an overlay for the PCS band. The issue of use of a CDMA microcell underlay for an existing analog macrocell is the focus of. It is shown that high capacity can be achieved in a microcell at the expense of a slight degradation in macrocell performance.Reference finds that transmit and receive notch filters should be used at the microcell BSs. It shows that key parameters for such an overlay are the powers of the CDMA BS and MS transmitters relative to the macrocell BSs and the MSs served by the macrocells. Reference [25] studies spectrum management in an overlay system. A new cell selection method is proposed, which uses the history of microcell sojourn times. A procedure to determine an optimum velocity threshold for the proposed method is also outlined. A systematic approach to optimal frequency spectrum management is described.Special Architectures There are several special cellular architectures that try to improve spectral efficiency without a large increase in infrastructure costs. Some ofthese structures, discussed here, include an underlay/overlay system (which is different from the overlay/underlay system described earlier) and a multichannel bandwidth system. Many cellular systems are expected to evolve from a macrocellular system to an overlay/underlay system. A study that focuses on such evolution is described in [26].A Multiple-Channel-Bandwidth System—Multiple channel bandwidths can be used within a cell to improve spectral efficiency.In a multiple-channel-bandwidth system (MCBS), a cell has two or three ring-shaped regions with different bandwidth channels [28]. Figure 7 shows an MCBS. Assume that 30 kHz is the normal bandwidth for a signal.Now, for a three-ring MCBS, 30 kHz channels can be used in the outermost ring, 15 kHz channels in the middle ring, and 7.5 kHz channels in the innermost ring. The areas of these rings can be determined based on the expected traffic conditions.Thus, instead of using 30 kHz channels throughout the cell, different bandwidth channels (e.g., 15 kHz and 7.5 kHz) can be used to increase the number of channels in a cell. The MCBS uses the fact that a wide-bandwidth channel requires a lower carrier-to-interference ratio (C/I) than a narrow-bandwidth channel for the same voice quality. For example, C/I requirements for 30 kHz,15 kHz, and 7.5 kHz channel bandwidths are 18 dB, 24 dB, and 30 dB, respectively, based on subjective voice quality tests [28]. If the transmit power at a cell cite is the same for all the bandwidths, a wide channel can serve a large cell while a narrow channel can serve a relatively small cell. Moreover, since a wide channel can tolerate a higher level of co-channel interference (CCI), it can afford a smaller D/R ratio (the ratio of co-channel distance to cell radius). Thus, in the MCBS more channels become available due to multiple-bandwidth signals, and frequency can be reused more closely in a given service region due to different C/I requirements.Integrated Wireless SystemsIntegrated wireless systems are exemplified by integrated cordless and cellular systems, integrated cellular systems, and integrated terrestrial and satellite systems. Such integrated systems combine the features of individual wireless systems to achieve the goals of improved mobility and low cost.Integrated Terrestrial Systems —Terrestrial intersystem handoff may be between two cellular systems or between a cellular system and a cordless telephone system. Examples of systems that need intersystem handoffs include GSM–Digital European Cordless Telephone (DECT), CDMA in macrocells, and TDMA in microcells. When a call initiated in a cellular system controlled by an MSC enters a system controlled by another MSC, intersystem handoff is required to continue the call [29]. In this case one MSC makes a handoff request to another MSC to save the call. The MSCs need to have software for intersystem handoff if intersystem handoff is to be implemented. Compatibility between the concerned MSCs needs to be considered, too.There are several possible outcomes of an intersystem handoff [29]:• A long-distance call becomes a local call when an MS becomes a roamer.• A long-distance call becomes a local call when a roamer becomes a home mobile unit.• A local call becomes a long distance call when a home mobile unit becomes a roamer.• A local call becomes a long-distance call when a roamer becomes a home mobile unit. There is a growing trend toward service portability across dissimilar systems such as GSM and DECT [30]. For example,it is nice to have intersystem handoff between cordless and cellular coverage. Cost-effective handoff algorithms for such scenarios represent a significant research area. This article outlines different approaches to achieving intersystem handoff. Simulation results arepresented for handoff between GSM and DECT/Wide Access Communications System (WACS). The paper shows that a minor adjustment to the DECT specification can greatly simplify the implementation of an MS capable of intersystem handoff between GSM and DECT.Integrated Terrestrial and Satellite Systems—In an integrated cellular/satellite system, the advantages of satellites and cellular systems can be combined. Satellites can provide widearea coverage, completion of coverage, immediate service, and additional capacity (by handling overflow traffic). A cellular system can provide a high-capacity economical system. Some of the issues involved in an integrated system are discussed in [31]. In particular, the procedures of GSM are examined for their application to the integrated systems.The future public land mobile telecommunication system (FPLMTS) will provide a personal telephone system that enables a person with a handheld terminal to reach anywhere in the world [32]. The FPLMTS will include low Earth orbit (LEO) or geostationary Earth orbit (GEO) satellites as well as terrestrial cellular systems. When an MS is inside the coverage area of a terrestrial cellular system, the BS will act as a relay station and provide a link between the MS and the satellite.When an MS is outside the terrestrial system coverage area, it will have a direct communication link with the satellite.Different issues such as system architecture, call handling, performance analysis of the access, and transmission protocols are discussed in [32]. The two handoff scenarios in an integrated system are described below.Handoff from the Land Mobile Satellite System to the TerrestrialSystem —While operating, the MS monitors the satellite link and evaluates the link performance. The received signal strengths (RSSs) are averaged (e.g., over a 30 s time period) to minimize signal strength variations. If the RSS falls below a certain threshold N consecutive times (e.g., N = 3), the MS begins measuring RSS from the terrestrial cellular system.If the terrestrial signals are strong enough, handoff is made to the terrestrial system, provided that the terrestrial system can serve the MS.Handoff from the Terrestrial System to the Land Mobile Satellite System —When an MS is getting service from the terrestrial system, the BS sends an acknowledge request(called page) at predefined intervals to ensure that the MS is still inside the coverage area. If an acknowledge request signal from the MS (called page response) is not received at the BS for N consecutive times, it is handed off to the land mobile satellite system (LMSS).Reference [33] focuses on personalcommunication systems with hierarchical overlays that incorporate terrestrial and satellite systems. The lowest level in the hierarchy is formed by microcells. Macrocells overlay microcells and form the middle level in the hierarchy. Satellite beams overlay macrocells and constitute the topmost hierarchy level. Two types of subscribers are considered, satellite-only and dual cellular/satellite. Call attempts from satellite-only subscribers are served by satellite systems, while call attempts from dual subscribers are first directed to the serving terrestrial systems with the satellites taking care of the overflow traffic. An analytical model for teletraffic performance is developed, and performance measures such as traffic distribution, blocking probability, and forced termination probability are evaluated for low-speed and high-speed users.Handoff Evaluation MechanismsThree basic mechanisms used to evaluate the performance of handoff algorithms include the analytical, simulation, and emulation approaches. These mechanisms are described here. The Analytical Approach This approach can quickly give a preliminary idea about the performance of some handoff algorithms for simplified handoff scenarios. This approach is valid only under specified constraints (e.g., assumptions about the RSS profiles). Actual handoff procedures are quite complicated and are not memoryless.This makes the analytical approach less realistic. For real-world situations, this approach is complex and mathematically intractable. Some of the analytical approaches appearing in the literature are briefly touched on below.二、英文翻译:蜂窝系统切换技术蜂窝系统部署方案其无线电传播环境和相关切换的难度是在于针对不同的单元结构。
遗传算法中英文对照外文翻译文献
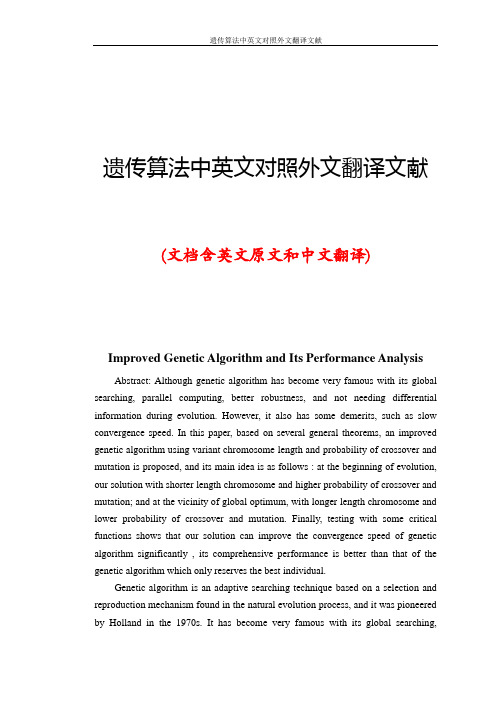
遗传算法中英文对照外文翻译文献(文档含英文原文和中文翻译)Improved Genetic Algorithm and Its Performance Analysis Abstract: Although genetic algorithm has become very famous with its global searching, parallel computing, better robustness, and not needing differential information during evolution. However, it also has some demerits, such as slow convergence speed. In this paper, based on several general theorems, an improved genetic algorithm using variant chromosome length and probability of crossover and mutation is proposed, and its main idea is as follows : at the beginning of evolution, our solution with shorter length chromosome and higher probability of crossover and mutation; and at the vicinity of global optimum, with longer length chromosome and lower probability of crossover and mutation. Finally, testing with some critical functions shows that our solution can improve the convergence speed of genetic algorithm significantly , its comprehensive performance is better than that of the genetic algorithm which only reserves the best individual.Genetic algorithm is an adaptive searching technique based on a selection and reproduction mechanism found in the natural evolution process, and it was pioneered by Holland in the 1970s. It has become very famous with its global searching,parallel computing, better robustness, and not needing differential information during evolution. However, it also has some demerits, such as poor local searching, premature converging, as well as slow convergence speed. In recent years, these problems have been studied.In this paper, an improved genetic algorithm with variant chromosome length and variant probability is proposed. Testing with some critical functions shows that it can improve the convergence speed significantly, and its comprehensive performance is better than that of the genetic algorithm which only reserves the best individual.In section 1, our new approach is proposed. Through optimization examples, in section 2, the efficiency of our algorithm is compared with the genetic algorithm which only reserves the best individual. And section 3 gives out the conclusions. Finally, some proofs of relative theorems are collected and presented in appendix.1 Description of the algorithm1.1 Some theoremsBefore proposing our approach, we give out some general theorems (see appendix) as follows: Let us assume there is just one variable (multivariable can be divided into many sections, one section for one variable) x ∈ [ a, b ] , x ∈ R, and chromosome length with binary encoding is 1.Theorem 1 Minimal resolution of chromosome is s =12l --a b Theorem 2 Weight value of the ith bit of chromosome isw i =12l --a b 12-i ( i = 1,2,…l ) Theorem 3 Mathematical expectation Ec(x) of chromosome searching step with one-point crossover isE c (x) = la b 2-P c where Pc is the probability of crossover.Theorem 4 Mathematical expectation Em ( x ) of chromosome searching step with bit mutation isE m ( x ) = ( b- a) P m1. 2 Mechanism of algorithmDuring evolutionary process, we presume that value domains of variable are fixed, and the probability of crossover is a constant, so from Theorem 1 and 3, we know that the longer chromosome length is, the smaller searching step of chromosome, and the higher resolution; and vice versa. Meanwhile, crossover probability is in direct proportion to searching step. From Theorem 4, changing the length of chromosome does not affect searching step of mutation, while mutation probability is also in direct proportion to searching step.At the beginning of evolution, shorter length chromosome( can be too shorter, otherwise it is harmful to population diversity ) and higher probability of crossover and mutation increases searching step, which can carry out greater domain searching, and avoid falling into local optimum. While at the vicinity of global optimum, longer length chromosome and lower probability of crossover and mutation will decrease searching step, and longer length chromosome also improves resolution of mutation, which avoid wandering near the global optimum, and speeds up algorithm converging.Finally, it should be pointed out that chromosome length changing keeps individual fitness unchanged, hence it does not affect select ion ( with roulette wheel selection) .1. 3 Description of the algorithmOwing to basic genetic algorithm not converging on the global optimum, while the genetic algorithm which reserves the best individual at current generation can, our approach adopts this policy. During evolutionary process, we track cumulative average of individual average fitness up to current generation. It is written as X(t) = G 1∑=G t avg f1(t)where G is the current evolutionary generation,avg f is individual averagefitness. When the cumulative average fitness increases to k times ( k> 1, k ∈ R) of initial individual average fitness, we change chromosome length to m times ( m is a positive integer ) of itself , and reduce probability of crossover and mutation, whichcan improve individual resolution and reduce searching step, and speed up algorithm converging. The procedure is as follows:Step 1 Initialize population, and calculate individual average fitness0avg f ,and set change parameter flag. Flag equal to 1.Step 2 Based on reserving the best individual of current generation, carry out selection, regeneration, crossover and mutation, and calculate cumulative average of individual average fitness up to current generationavg f ;Step 3 If 0avg avgf f ≥k and Flag equals 1, increase chromosome length to m times of itself, and reduce probability of crossover and mutation, and set Flag equal to 0; otherwise continue evolving.Step 4 If end condition is satisfied, stop; otherwise go to Step 2.2 Test and analysisWe adopt the following two critical functions to test our approach, and compare it with the genetic algorithm which only reserves the best individual: ()]01.01[5.0sin 5.0),(2222221y x y x y x f ++-+-= ]5,5[ ∈,-y x))4cos(4.0)3cos(3.02(4),(222y x y x y x f ππ--+-= ]1,1[ ∈,-y x2. 1 Analysis of convergenceDuring function testing, we carry out the following policies: roulette wheel select ion, one point crossover, bit mutation, and the size of population is 60, l is chromosome length, Pc and Pm are the probability of crossover and mutation respectively. And we randomly select four genetic algorithms reserving best individual with various fixed chromosome length and probability of crossover and mutation to compare with our approach. Tab. 1 gives the average converging generation in 100 tests.In our approach, we adopt initial parameter l0= 10, Pc0= 0.3, Pm0= 0.1 and k=1.2, when changing parameter condition is satisfied, we adjust parameters to l= 30, Pc= 0.1, Pm= 0.01.From Tab. 1, we know that our approach improves convergence speed of genetic algorithm significantly and it accords with above analysis.2. 2 Analysis of online and offline performanceQuantitative evaluation methods of genetic algorithm are proposed by Dejong, including online and offline performance. The former tests dynamic performance; and the latter evaluates convergence performance. To better analyze online and offline performance of testing function, w e multiply fitness of each individual by 10, and we give a curve of 4 000 and 1 000 generations for f1 and f2, respectively.(a) online (b) onlineFig. 1 Online and offline performance of f1(a) online (b) onlineFig. 2 Online and offline performance of f2From Fig. 1 and Fig. 2, we know that online performance of our approach is just little worse than that of the fourth case, but it is much better than that of the second, third and fifth case, whose online performances are nearly the same. At the same time, offline performance of our approach is better than that of other four cases.3 ConclusionIn this paper, based on some general theorems, an improved genetic algorithmusing variant chromosome length and probability of crossover and mutation is proposed. Testing with some critical functions shows that it can improve convergence speed of genetic algorithm significantly, and its comprehensive performance is better than that of the genetic algorithm which only reserves the best individual.AppendixWith the supposed conditions of section 1, we know that the validation of Theorem 1 and Theorem 2 are obvious.Theorem 3 Mathematical expectation Ec(x) of chromosome searching step with one point crossover is Ec(x) = c P l a b 2-where Pc is the probability of crossover.Proof As shown in Fig. A1, we assume that crossover happens on the kth locus, i. e. parent’s locus from k to l do not change, and genes on the locus from 1 to k are exchanged.During crossover, change probability of genes on the locus from 1 to k is 21(“1” to “0” or “0” to “1”). So, after crossover, mathematical expectation of chromosome searching step on locus from 1 to k is)12(12212122121)(111-•--•=•--•==-==∑∑k l j k j l j kj ck a b a b w x E Furthermore, probability of taking place crossover on each locus of chromosome is equal, namely l 1Pc. Therefore, after crossover, mathematical expectation of chromosome searching step is)(1)(11x E P lx E ck c l k c ••=∑-= Substituting Eq. ( A1) into Eq. ( A2) , we obtain )1211(2)(])12[(122)12(12211)(11---•=--•--•=-•--•••=∑-=l c i l c k l c l k c l a b P l a b l P a b P lx E where l is large, 012≈-l l , so )(x E c c P l a b 2-≈Fig. A1 One point crossoverTheorem 4 Mathematical expectation)(x E m of chromosome searching step with bit mutation m m P a b x E •-=)()(, where Pm is the probability of mutation.Proof Mutation probability of genes on each locus of chromosome is equal, say Pm, therefore, mathematical expectation of mutation searching step isE m (x )=P m ·w i =i =1l åP m ·b -a 2l -1·2i -1=i =1l åP m ·b -a 2i -1·(2i -1)=(b -a )·P m一种新的改进遗传算法及其性能分析摘要:虽然遗传算法以其全局搜索、并行计算、更好的健壮性以及在进化过程中不需要求导而著称,但是它仍然有一定的缺陷,比如收敛速度慢。
外文翻译---人工神经网络
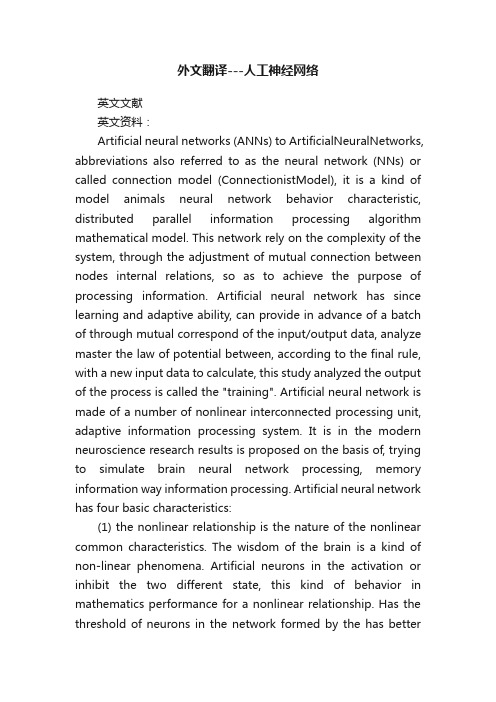
外文翻译---人工神经网络英文文献英文资料:Artificial neural networks (ANNs) to ArtificialNeuralNetworks, abbreviations also referred to as the neural network (NNs) or called connection model (ConnectionistModel), it is a kind of model animals neural network behavior characteristic, distributed parallel information processing algorithm mathematical model. This network rely on the complexity of the system, through the adjustment of mutual connection between nodes internal relations, so as to achieve the purpose of processing information. Artificial neural network has since learning and adaptive ability, can provide in advance of a batch of through mutual correspond of the input/output data, analyze master the law of potential between, according to the final rule, with a new input data to calculate, this study analyzed the output of the process is called the "training". Artificial neural network is made of a number of nonlinear interconnected processing unit, adaptive information processing system. It is in the modern neuroscience research results is proposed on the basis of, trying to simulate brain neural network processing, memory information way information processing. Artificial neural network has four basic characteristics:(1) the nonlinear relationship is the nature of the nonlinear common characteristics. The wisdom of the brain is a kind of non-linear phenomena. Artificial neurons in the activation or inhibit the two different state, this kind of behavior in mathematics performance for a nonlinear relationship. Has the threshold of neurons in the network formed by the has betterproperties, can improve the fault tolerance and storage capacity.(2) the limitations a neural network by DuoGe neurons widely usually connected to. A system of the overall behavior depends not only on the characteristics of single neurons, and may mainly by the unit the interaction between the, connected to the. Through a large number of connection between units simulation of the brain limitations. Associative memory is a typical example of limitations.(3) very qualitative artificial neural network is adaptive, self-organizing, learning ability. Neural network not only handling information can have all sorts of change, and in the treatment of the information at the same time, the nonlinear dynamic system itself is changing. Often by iterative process description of the power system evolution.(4) the convexity a system evolution direction, in certain conditions will depend on a particular state function. For example energy function, it is corresponding to the extreme value of the system stable state. The convexity refers to the function extreme value, it has DuoGe DuoGe system has a stable equilibrium state, this will cause the system to the diversity of evolution.Artificial neural network, the unit can mean different neurons process of the object, such as characteristics, letters, concept, or some meaningful abstract model. The type of network processing unit is divided into three categories: input unit, output unit and hidden units. Input unit accept outside the world of signal and data; Output unit of output system processing results; Hidden unit is in input and output unit, not between by external observation unit. The system The connections between neurons right value reflect the connection between the unit strength, information processing and embodied in the network said theprocessing unit in the connections. Artificial neural network is a kind of the procedures, and adaptability, brain style of information processing, its essence is through the network of transformation and dynamic behaviors have akind of parallel distributed information processing function, and in different levels and imitate people cranial nerve system level of information processing function. It is involved in neuroscience, thinking science, artificial intelligence, computer science, etc DuoGe field cross discipline.Artificial neural network is used the parallel distributed system, with the traditional artificial intelligence and information processing technology completely different mechanism, overcome traditional based on logic of the symbols of the artificial intelligence in the processing of intuition and unstructured information of defects, with the adaptive, self-organization and real-time characteristic of the study.Development historyIn 1943, psychologists W.S.M cCulloch and mathematical logic W.P home its established the neural network and the math model, called MP model. They put forward by MP model of the neuron network structure and formal mathematical description method, and prove the individual neurons can perform the logic function, so as to create artificial neural network research era. In 1949, the psychologist put forward the idea of synaptic contact strength variable. In the s, the artificial neural network to further development, a more perfect neural network model was put forward, including perceptron and adaptive linear elements etc. M.M insky, analyzed carefully to Perceptron as a representative of the neural network system function and limitations in 1969 after the publication of the book "Perceptron, and points out thatthe sensor can't solve problems high order predicate. Their arguments greatly influenced the research into the neural network, and at that time serial computer and the achievement of the artificial intelligence, covering up development new computer and new ways of artificial intelligence and the necessity and urgency, make artificial neural network of research at a low. During this time, some of the artificial neural network of the researchers remains committed to this study, presented to meet resonance theory (ART nets), self-organizing mapping, cognitive machine network, but the neural network theory study mathematics. The research for neural network of research and development has laid a foundation. In 1982, the California institute of J.J.H physicists opfield Hopfield neural grid model proposed, and introduces "calculation energy" concept, gives the network stability judgment. In 1984, he again put forward the continuous time Hopfield neural network model for the neural computers, the study of the pioneering work, creating a neural network for associative memory and optimization calculation, the new way of a powerful impetus to the research into the neural network, in 1985, and scholars have proposed a wave ears, the study boltzmann model using statistical thermodynamics simulated annealing technology, guaranteed that the whole system tends to the stability of the points. In 1986 the cognitive microstructure study, puts forward the parallel distributed processing theory. Artificial neural network of research by each developed country, the congress of the United States to the attention of the resolution will be on jan. 5, 1990 started ten years as the decade of the brain, the international research organization called on its members will the decade of the brain into global behavior. In Japan's "real world computing(springboks claiming)" project, artificial intelligence research into an important component.Network modelArtificial neural network model of the main consideration network connection topological structure, the characteristics, the learning rule neurons. At present, nearly 40 kinds of neural network model, with back propagation network, sensor, self-organizing mapping, the Hopfieldnetwork.the computer, wave boltzmann machine, adapt to the ear resonance theory. According to the topology of the connection, the neural network model can be divided into:(1) prior to the network before each neuron accept input and output level to the next level, the network without feedback, can use a loop to no graph. This network realization from the input space to the output signal of the space transformation, it information processing power comes from simple nonlinear function of DuoCi compound. The network structure is simple, easy to realize. Against the network is a kind of typical prior to the network.(2) the feedback network between neurons in the network has feedback, can use a no to complete the graph. This neural network information processing is state of transformations, can use the dynamics system theory processing. The stability of the system with associative memory function has close relationship. The Hopfield network.the computer, wave ear boltzmann machine all belong to this type.Learning typeNeural network learning is an important content, it is through the adaptability of the realization of learning. According to the change of environment, adjust to weights, improve thebehavior of the system. The proposed by the Hebb Hebb learning rules for neural network learning algorithm to lay the foundation. Hebb rules say that learning process finally happened between neurons in the synapse, the contact strength synapses parts with before and after the activity and synaptic neuron changes. Based on this, people put forward various learning rules and algorithm, in order to adapt to the needs of different network model. Effective learning algorithm, and makes the godThe network can through the weights between adjustment, the structure of the objective world, said the formation of inner characteristics of information processing method, information storage and processing reflected in the network connection. According to the learning environment is different, the study method of the neural network can be divided into learning supervision and unsupervised learning. In the supervision and study, will the training sample data added to the network input, and the corresponding expected output and network output, in comparison to get error signal control value connection strength adjustment, the DuoCi after training to a certain convergence weights. While the sample conditions change, the study can modify weights to adapt to the new environment. Use of neural network learning supervision model is the network, the sensor etc. The learning supervision, in a given sample, in the environment of the network directly, learning and working stages become one. At this time, the change of the rules of learning to obey the weights between evolution equation of. Unsupervised learning the most simple example is Hebb learning rules. Competition rules is a learning more complex than learning supervision example, it is according to established clustering on weights adjustment. Self-organizing mapping, adapt to theresonance theory is the network and competitive learning about the typical model.Analysis methodStudy of the neural network nonlinear dynamic properties, mainly USES the dynamics system theory and nonlinear programming theory and statistical theory to analysis of the evolution process of the neural network and the nature of the attractor, explore the synergy of neural network behavior and collective computing functions, understand neural information processing mechanism. In order to discuss the neural network and fuzzy comprehensive deal of information may, the concept of chaos theory and method will play a role. The chaos is a rather difficult toprecise definition of the math concepts. In general, "chaos" it is to point to by the dynamic system of equations describe deterministic performance of the uncertain behavior, or call it sure the randomness. "Authenticity" because it by the intrinsic reason and not outside noise or interference produced, and "random" refers to the irregular, unpredictable behavior, can only use statistics method description. Chaotic dynamics of the main features of the system is the state of the sensitive dependence on the initial conditions, the chaos reflected its inherent randomness. Chaos theory is to point to describe the nonlinear dynamic behavior with chaos theory, the system of basic concept, methods, it dynamics system complex behavior understanding for his own with the outside world and for material, energy and information exchange process of the internal structure of behavior, not foreign and accidental behavior, chaos is a stationary. Chaotic dynamics system of stationary including: still, stable quantity, the periodicity, with sex and chaos of accuratesolution... Chaos rail line is overall stability and local unstable combination of results, call it strange attractor.A strange attractor has the following features: (1) some strange attractor is a attractor, but it is not a fixed point, also not periodic solution; (2) strange attractor is indivisible, and that is not divided into two and two or more to attract children. (3) it to the initial value is very sensitive, different initial value can lead to very different behavior.superiorityThe artificial neural network of characteristics and advantages, mainly in three aspects: first, self-learning. For example, only to realize image recognition that the many different image model and the corresponding should be the result of identification input artificial neural network, the network will through the self-learning function, slowly to learn to distinguish similar images. The self-learning function for the forecast has special meaning. The prospect of artificial neural network computer will provide mankind economic forecasts, market forecast, benefit forecast, the application outlook is very great. The second, with lenovo storage function. With the artificial neural network of feedback network can implement this association. Third, with high-speed looking for the optimal solution ability. Looking for a complex problem of the optimal solution, often require a lot of calculation, the use of a problem in some of the design of feedback type and artificial neural network, use the computer high-speed operation ability, may soon find the optimal solution.Research directionThe research into the neural network can be divided into the theory research and application of the two aspects of research.Theory study can be divided into the following two categories: 1, neural physiological and cognitive science research on human thinking and intelligent mechanism.2, by using the neural basis theory of research results, with mathematical method to explore more functional perfect, performance more superior neural network model, the thorough research network algorithm and performance, such as: stability and convergence, fault tolerance, robustness, etc.; The development of new network mathematical theory, such as: neural network dynamics, nonlinear neural field, etc.Application study can be divided into the following two categories:1, neural network software simulation and hardware realization of research.2, the neural network in various applications in the field of research. These areas include: pattern recognition, signal processing, knowledge engineering, expert system, optimize the combination, robot control, etc. Along with the neural network theory itself and related theory, related to the development of technology, the application of neural network will further.Development trend and research hot spotArtificial neural network characteristic of nonlinear adaptive information processing power, overcome traditional artificial intelligence method for intuitive, such as mode, speech recognition, unstructured information processing of the defects in the nerve of expert system, pattern recognition and intelligent control, combinatorial optimization, and forecast areas to be successful application. Artificial neural network and other traditional method unifies, will promote the artificial intelligence and information processing technology development. In recentyears, the artificial neural network is on the path of human cognitive simulation further development, and fuzzy system, genetic algorithm, evolution mechanism combined to form a computational intelligence, artificial intelligence is an important direction in practical application, will be developed. Information geometry will used in artificial neural network of research, to the study of the theory of the artificial neural network opens a new way. The development of the study neural computers soon, existing product to enter the market. With electronics neural computers for the development of artificial neural network to provide good conditions.Neural network in many fields has got a very good application, but the need to research is a lot. Among them, are distributed storage, parallel processing, since learning, the organization and nonlinear mapping the advantages of neural network and other technology and the integration of it follows that the hybrid method and hybrid systems, has become a hotspot. Since the other way have their respective advantages, so will the neural network with other method, and the combination of strong points, and then can get better application effect. At present this in a neural network and fuzzy logic, expert system, genetic algorithm, wavelet analysis, chaos, the rough set theory, fractal theory, theory of evidence and grey system and fusion.汉语翻译人工神经网络(ArtificialNeuralNetworks,简写为ANNs)也简称为神经网络(NNs)或称作连接模型(ConnectionistModel),它是一种模范动物神经网络行为特征,进行分布式并行信息处理的算法数学模型。
网络设计与规划中英文对照外文翻译文献
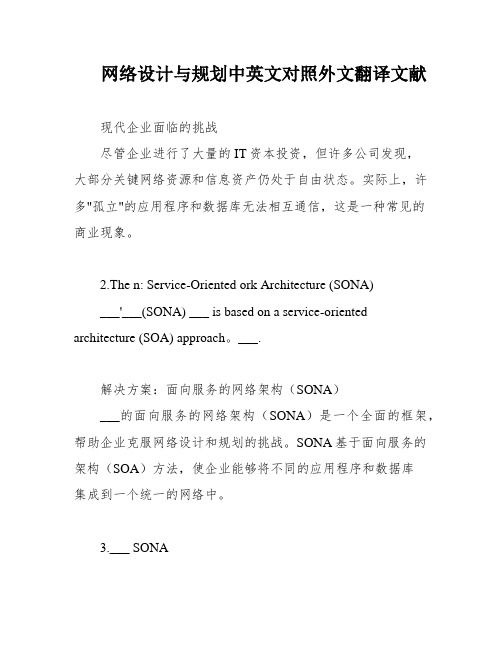
网络设计与规划中英文对照外文翻译文献现代企业面临的挑战尽管企业进行了大量的IT资本投资,但许多公司发现,大部分关键网络资源和信息资产仍处于自由状态。
实际上,许多"孤立"的应用程序和数据库无法相互通信,这是一种常见的商业现象。
2.The n: Service-Oriented ork Architecture (SONA)___'___(SONA) ___ is based on a service-oriented architecture (SOA) approach。
___.解决方案:面向服务的网络架构(SONA)___的面向服务的网络架构(SONA)是一个全面的框架,帮助企业克服网络设计和规划的挑战。
SONA基于面向服务的架构(SOA)方法,使企业能够将不同的应用程序和数据库集成到一个统一的网络中。
3.___ SONABy implementing SONA。
businesses ___ of benefits。
___。
increased security。
___。
___ security features。
such as identity and access management。
to protect critical n assets。
Finally。
___.SONA的好处通过实施SONA,企业可以获得许多好处,包括提高网络敏捷性、增加安全性和降低成本。
SONA通过提供灵活和可扩展的网络架构,使企业能够快速适应不断变化的业务需求。
此外,SONA提供了增强的安全功能,如身份和访问管理,以保护关键信息资产。
最后,SONA通过简化网络管理和减少对额外硬件和软件的需求,帮助企业降低成本。
4.nIn today's fast-paced business environment。
it is essential for ___。
secure。
and cost-effective ork architecture.结论在今天快节奏的商业环境中,企业必须拥有一个可以快速适应不断变化的业务需求的网络基础设施。
- 1、下载文档前请自行甄别文档内容的完整性,平台不提供额外的编辑、内容补充、找答案等附加服务。
- 2、"仅部分预览"的文档,不可在线预览部分如存在完整性等问题,可反馈申请退款(可完整预览的文档不适用该条件!)。
- 3、如文档侵犯您的权益,请联系客服反馈,我们会尽快为您处理(人工客服工作时间:9:00-18:30)。
基于神经网络和遗传算法的模糊系统的自动设计摘要本文介绍了基于神经网络和遗传算法的模糊系统的设计,其目的在于缩短开发时间并提高该系统的性能。
介绍一种利用神经网络来描绘的多维非线性隶属函数和调整隶属函数参数的方法。
还提及了基于遗传算法的集成并自动化三个模糊系统的设计平台。
1 前言模糊系统往往是人工手动设计。
这引起了两个问题:一是由于人工手动设计是费时间的,所以开发费用很高;二是无法保证获得最佳的解决方案。
为了缩短开发时间并提高模糊系统的性能,有两种独立的途径:开发支持工具和自动设计方法。
前者包括辅助模糊系统设计的开发环境。
许多环境已具有商业用途。
后者介绍了自动设计的技术。
尽管自动设计不能保证获得最优解,他们仍是可取的手工技巧,因为设计是引导走向和依某些标准的最优解。
有三种主要的设计决策模糊控制系统设计:(1)确定模糊规则数,(2)确定隶属度函数的形式。
(3)确定变化参数再者,必须作出另外两个决定:(4)确定输入变量的数量(5)确定论证方法(1)和(2)相互协调确定如何覆盖输入空间。
他们之间有高度的相互依赖性。
(3)用以确定TSK(Takagi-Sugeno-Kang)模式【1】中的线性方程式的系数,或确定隶属度函数以及部分的Mamdani模型【2】。
(4)符合决定最低套相关的输入变量,计算所需的目标决策或控制的价值观。
像逆向消除(4)和信息标准的技术在此设计中经常被利用。
(5)相当于决定使用哪一个模糊算子和解模糊化的方法。
虽然由数种算法和模糊推理的方法已被提出,仍没有选择他们标准。
[5]表明动态变化的推理方法,他依据这个推理环境的结果在性能和容错性高于任何固定的推理的方法。
神经网络模型(以更普遍的梯度)和基于遗传算法的神经网络(最常见的梯度的基础)和遗传算法被用于模糊系统的自动设计。
基于神经网络的方法主要是用来设计模糊隶属度函数。
这有两种主要的方法;(一)直接的多维的模糊隶属度函数的设计:该方法首先通过数据库确定规则的数目。
然后通过每个簇的等级的训练来确定隶属函数的形式。
更多细节将在第二章给出。
(二)间接的多维的模糊隶属度函数的设计:这种方法通过结合一维模糊隶属函数构建多维的模糊隶属度函数。
隶属度函数梯度技术被用于调节试图减少模糊系统的期望产量和实际生产所需的产出总量的误差。
第一种方法的优点在于它可以直接产生非线性多维的模糊隶属度函数;没有必要通过结合一维模糊隶属函数构建多维的模糊隶属度函数。
第二种方法的优点在于可通过监测模糊系统的最后性能来调整。
这两种方法都将在第二章介绍。
许多基于遗传算法的方法与方法二在本质上一样;一维隶属函数的形式利用遗传算法自动的调整。
这些方法中很多只考虑了一个或两个前面提及的设计问题。
在第三章中,我们将介绍一种三个设计问题同时考虑的方法。
2 神经网络方法2.1多维输入空间的直接的模糊分区该方法利用神经网络来实现多维的非线性隶属度函数,被称为基于NN的模糊推理。
该方法的优点在于它可以产生非线性多维的模糊隶属度函数。
在传统的模糊系统中,用于前期部分的一维隶属度函数是独立设计的,然后结合起来间接实现多维的模糊隶属度函数。
可以说,神经网络方法在由神经网络吸收的结合操作方面是传统模糊系统的一种更普遍的形式。
当输入变量是独立的时传统的间接设计方法就有问题。
例如,设计一个基于将温度和湿度作为输入的模糊系统的空调控制系统。
在模糊系统的传统设计方法中,隶属函数的温度和湿度是独立设计的。
输入空间所产生的模糊分区如图1(a)。
然而,当输入变量是独立的,如温度、湿度,模糊分区如图1(b)比较合适。
很难构建这样来自一维模糊隶属度函数的非线性分区。
由于NN-driven模糊推理直接构建多维的非线性的模糊隶属度函数,很有可能使线性分区如图1(b)。
NN-driven模糊推理的设计的有三个步骤:聚集给出的训练数据,利用神经网络的模糊分区输入空间,和设计各分区空间的随机部分。
第一步是要聚集培训资料,确定规则的数目。
这一步之前,不恰当的输入变量已经利用信息或淘汰落后指标的方法消除掉了。
逆向消除方法的任意消除n个输入变量和训练神经网络的n - 1个输入变量。
然后比较n个和n-1个变量的神经网络的性能。
如果n-1个变量的神经网络的性能与n个变量的性能相似或者更好,那么消除输入变量就被认为是无关紧要的。
然后这些数据被聚集,得到了数据的分布。
集群数量是规则的数目。
第二步是决定在第一步中得到的集群资料的簇边界;输入空间的划分并确定多维输入的隶属函数。
监督数据是由在第1步中获得的隶属度的输入数据聚类提供的。
第一个带有n输入和c输出的神经网络被准备好,其中n是输入变量的数量,c是在第一步中得到的集群数量。
为了神经网络的数据,图2中NN数量,产生于第一步提供的集群信息。
一般来说,每个输入变量被分配到其中的一个集群。
集群任务就是将输入变量和培训模式相结合。
例如,在属于集群2的四个集群和输入向量的案例中,监督的培训模式将是(0,1,0,0)。
在某些情况下,如果他/她相信一个输入的数据点应按不同的聚类,用户不得非法干预和手动建造部分监督。
举例来说,如果用户认为一个数据点同样属于一个两个班,适当的监管输出模式可能(0.5,0.5,0,0)。
这个神经网络在关于该培训资料的训练结束后,神经网络计算特定输入属于各集群向量。
因此,我们认为该神经网络通过学习获得特征的隶属度函数所有的规则,可以产生与隶属度相适应的任意的输入向量。
利用神经网络如发生器的模糊系统是NN-driven模糊推理。
第三步是随机的设计。
因为我们知道哪个集群能举出一个输入数据,我们可以使用输入数据和期望的结果训练随机的部分。
神经网络的表达可在这里,如[3,4]中所言,但是其他的方法,如数学方程或模糊变量,可以用来代替。
该模型的最关键的是神经网络的输入空间分割模糊聚类。
图2所示的一个例子NN-driven模糊推理系统。
这是一个输出由神经网络或TSK模型计算的一个单独的价值的模型。
在图乘法和加法计算加权平均值。
如果后续的部分输出模糊值,适当的t-conorm和/或解模糊化操作应该被使用。
图1.模糊划分:(a)常规(b)期望图2.NN-driven结构模糊推理实例2.2调整参数的模糊系统这个定义隶属度函数形式的参数来减少模糊系统输出和监督的数据之间的误差。
两种方法用于修改这些参数:摘要现有基于梯度方法和遗传算法。
遗传算法的方法将在下一章节讲述,基于梯度的方法将在这部分解释。
这个基于梯度的方法的程序是:(1)决定如何确定的隶属度函数的形式(2)利用梯度方法调整降低模糊系统的实际输出与期望输出的参数,通常最速下降。
隶属函数的中心的位置和宽度通常用来定义参数的形状。
Ichihashi et al. [6]and Nomura et al. [7, 8], Horikawa et al.[9][10], Ichihashi et al.[ll] and Wang et al. [12], Jang [13][14] 已经分别用三角形,结合sigmoidal、高斯,钟型隶属度函数。
他们利用最速下降法来调整模糊隶属函数参数。
图3. 神经网络调整模糊系统的参数图4. 调整模糊系统的神经网络图3显示了此方法和同构于图4. 图中的u ij在i-th 规则下输入模糊隶属函数的参数x j,而它实际上是代表一个描述隶属度函数的形式的参数向量。
也就是说,这个方法使模糊系统作为神经网络的模糊隶属度函数和通过节点执行重量和规则一样。
任何网络学习算法,例如反向传播算法,可以用来设计这种结构。
3遗传算法方法3.1遗传算法与模糊控制遗传算法是进行优化、生物激励的技术,他的运行用二进制表示,并进行繁殖,交叉和变异。
繁殖后代的权利是通过应用程序提供的一种健身价值。
遗传算法吸引人是因为他们不需要存在的衍生物,他们的同时搜索的鲁棒性很强,并能避免陷入局部最小。
SeverM的论文提出了利用自动遗传算法的模糊系统的设计方法。
大量的工作主要集中在调整的模糊隶属度函数[17]-[25]。
其他的方法使用遗传算法来确定模糊规则数[18,26]。
在[26]中,通过专家制定了一系列规则,并且遗传算法找到他们的最佳的组合。
在[18],卡尔已经开发出一种方法用于测定模糊隶属度函数和模糊规则数。
在这篇文章中,卡尔的方法首先用遗传算法按照预先定义的规则库确定规则的数目。
这个阶段后,利用遗传算法来调整模糊隶属度函数。
虽然这些方法设计的系统表现的比手工设计的系统好,它们仍可能会欠佳,因为他们一次只有一个或两个三大设计阶段。
因为这些设计阶段可能不会是独立的,所以重要的是要考虑它们同时找到全局最优解。
在下一节里,我们提出一个结合的三个主要设计阶段自动设计方法。
3.2基于遗传算法的模糊系统的综合设计这部分提出了一种利用遗传算法的自动模糊系统的设计方法, 并将三个主要设计阶段一体化:隶属函数的形式, 模糊规则数, 和规则后件同一时间内确定[27]。
当将遗传算法应用于程序上时,有两个主要步骤;(a)选择合适的基因表达,(b) 设计一个评价函数的人口排名。
在接下来的段落里,我们讨论我们的模糊系统表现及基因表达。
一个嵌入验前知识的评价函数和方法将会在接下来的章节中提及模糊系统和基因表现我们用TSK模型的模糊系统,它被广泛应用于控制问题,对地图系统的状态来控制的价值。
TSK模型在随之而来的模糊模型中的线性方程与模糊语言表达方面有别于传统的模糊系统。
例如,一个TSK模型规则的形式:如果X1是A,X2是B,那么y=w1X1+w2X2+w3;是常数其中wn最后的控制价值通过每个规则的输出和依据规则的加权的射击力量来计算。
我们利用左基地,右基地,和以往的中心点距离(第一个中心是一个绝对的位置)对三角形隶属度函数进行参数化,。
其他参数化的形状,如双曲形、高斯,钟形,或梯形可以代替。
不同于大多数方法、重叠限制不是放在在我们的系统和完整的重叠存在(见图5)。
图5. (a)隶属度函数表示法(b)可能的隶属函数一般来说,每个输入变量模糊集理论的数量与确定的模糊规则的数目相结合。
例如,一个带有m输入变量的TSK模型,每n个模糊集,将会产生n m模糊规则。
因为规则的数目直接取决于这个数目的隶属函数,消除隶属度函数对消除规则有直接的影响。
每一隶属函数需要三个参数并且每一个模糊规则需要三个参数。
因此,每个变量需要n个模糊输入的m-input-one-output系统要求3(mn+n m)参量。
这个基因表达明确包含三个成员函数的参数及前述的随机参数。
然而,规则的数,通过应用编码含蓄的边界条件和隶属函数的定位。
我们可以隐含的控制规则的数目,消除隶属函数的中心位置的范围之外的相应的包含这些内容的输入变量和规则。
例如,在单摆应用隶属度函数使用θ的中心位置大于90°都是可以避免的。