蛋白质Geneontology-KEGG分析软件David使用方法介绍
DAVID 使用

DAVID KnowledgebaseWhy Do We Propose DAVID Gene Concept?Due to the complex and distributed nature of biological research,our current knowledge is spread over many redundant databases maintained by independent groups.One gene could have different identifiers within one,or many,databases. Similarly,the biological terms associated with different gene identifiers for the same gene could be collected in different levels across different databases.Most gene functional annotation databases are in a gene-associated format, i.e.annotation contents usually associate with corresponding gene or protein identifiers.Such a format provides an opportunity to integrate heterogeneous annotation resources through their common gene identifiers.However,there are dozens of types of gene or protein sequence identifiers that are redundant across several independent groups,such as GenBank Accession;GenBank ID;RefSeq Accession;PIR ID;PIR Accession;UniProt ID;UniProt Accession; Affymetrix Probe ID;etc.The major challenge of integration comes from the weak cross-reference of different types of gene identifiers used by different functional annotation databases.Figure:The poor coverage and overlap of different types of protein identifiers across independent resources.As examples,four popular types of protein identifiers(PIR ID,UniProt Accession,RefSeq Protein,and GenPept Accession)are only covered partially by NCBI Entrez Gene(EG),UniProt UniRef100(UP),and PIR NRef100(NF).The DAVID gene collects and integrates all of them for better coverage and integration.DAVID Gene Concept:DAVID gene is a secondary gene cluster used to hold all different types of gene IDs belonging to the same gene.Each unique gene has a unique DAVID gene ID.DAVID Gene is conceptially equivalent to Entrez Gene,but with much broader data coverage cross most,if not all,of well known bioinformatics systems.How is DAVID Gene Constructed?An Example:A DAVID gene constructed by a single-linkage algorithmFigure:Two UniRef100clusters,two NRef100clusters,and one Entrez Gene cluster were systematically found sharing one or more protein identifiers with each other.The single linkage rule can further iteratively agglomerate them as a whole into one DAVID Genegene.Thus,for this particular example of tyrosine-protein phosphatase non-receptor type21(PTPN21),the resulting DAVID Gene is able to integrate all gene/protein identifiers more comprehensivelyas compared to each original gene cluster.Results:The process collects~50million individual gene/protein identifiers representing22identifier types,which are eventually agglomerated into over3.7million DAVID genes,for over90,000species.How Are Annotations Assigned to DAVID Gene?DAVID Knowledgebase:After the annotations are assigned to DAVID Genes,the annotations plus DAVID Genes are calledDAVID Knowledgebase.Figure:Under DAVID Gene Concept,most major types of gene identifiers can be translated to a corresponding DAVID gene identifier.Thus,as long as annotation data are in gene-associated format,the heterogeneous annotation contents have a much better chance of being integrated by the common DAVID gene identifier,thus improving theintegration of annotation contents as a whole.Results:The DAVID Knowledgebase collects a wide range of annotation contents from dozens of databases including: Gene Ontology;Protein Domains;Bio-pathways;Gene Expression;Disease Association;PubMed;Protein-Protein interactions;Affymetrix;Gene General Features;NCI Thesaurus;Panther Family;and more.Hypothetical Illustration of DAVID Knowledgebase centralized by DAVID genesFigure:Illustration of the heterogeneous functional annotation sources integrated by DAVID genes.As long as they are in a gene-associated format,any functional annotation data sources can be linked by the common DAVID genes. Thus,a large collection of heterogeneous annotation sources can be integrated and fully cross-referenced.The Gene ID Type Converage in DAVID KnowledgebaseMore than20types of gene identifers were comprehensively collected by DAVID KnowledgebaseAnnotation Content Coverage in DAVID KnowledgebaseThe wide-range collection of heterogeneous functional annotations in the DAVID Knowledgebase.Over40functional categories from dozens of independent public sources(databases)are collected and integrated into the DAVIDKnowledgebaseDAVID Knowledgebase is Organized into Pairwise Text files.An Example:to query data from pairwise text formated files in DAVID KnowledgebaseThe DAVID Knowledgebase in a simple pairwise text format centralized by DAVID gene identifiers.Each independent annotation source and gene identifier system is separated into independent files in the same pairwise format of“did-to-annotation.”For this example,a user starts with Affymetrix identifier(affy_id)207849_at(IL2).The first step is to obtain the corresponding DAVID gene identifier(2864938).Then,with this DID(red),the annotation terms of interest(underlined)in different source files (OMIM,SMART,Pfam,GO Molecular Function,KEGG Pathway,BioCart Pathway,etc.)canbe queried sequentially.The Web Interface to Query the DAVID KnowledgebaseFrom genes to annotations。
零基础的小白如何自己做GOKEGG分析?

零基础的小白如何自己做GOKEGG分析?不会R语言,不会python,只会实验的生信小白如何面对自己的测序数据呢?今天就从最简单的GO term分析说起。
并且手把手教你在线做GO分析!let go!GO是Gene ontology的缩写,是一系列用来描述基因、基因产物特性的语义(terms)。
这些语义主要分为三种:细胞组份(Cellular Component,简称GO-CC),用于描述基因产物在细胞中的位置,如内质网,核或蛋白酶体等;分子功能(Molecular Function,简称GO-MF),大部分指的是单个基因产物的功能,如结合活性或催化活性等。
生物学途径/过程(biological process,简称GO-BP),多是指具有多个步骤的有序的生物过程,如细胞生长、分化和维持、凋亡以及信号传导等过程。
Pathway指代谢通路,对差异基因进行pathway分析,可以知道实验条件下哪些代谢通路发生显著改变。
KEGG(Kyoto Encyclopedia of Genes and Genomes),是一个系统分析基因产物在细胞中代谢途径的数据库,是一种最常用的代谢通路分析。
接下来,就安利一个不用安装分析软件不必有生信分析理论基础就可以在线完成的GO分析。
如下图,DAVID网站/home.jsp。
第一步,准备你的数据。
将需要做富集分析的差异基因或靶基因以基因名称为list保存为txt文档或者excel中。
第二步,网站分析。
1:打开网站/home.jsp如下图示,点击start analysis;2:上传数据出现下图所示页面,步骤进行:点击上传后,会弹出下图所示的一个对话框,点击确定即可。
3:分析数据出现以下界面,然后,在新界面里第一步点击clean all,第二步选择GO ONTOLOGY and PATHWAY 对应的前方+的下拉框。
出现,如下的新界面:在新界面里第一步依次勾选箭头所示的红字选项。
差异蛋白go和kegg分析流程

差异蛋白go和kegg分析流程下载温馨提示:该文档是我店铺精心编制而成,希望大家下载以后,能够帮助大家解决实际的问题。
文档下载后可定制随意修改,请根据实际需要进行相应的调整和使用,谢谢!并且,本店铺为大家提供各种各样类型的实用资料,如教育随笔、日记赏析、句子摘抄、古诗大全、经典美文、话题作文、工作总结、词语解析、文案摘录、其他资料等等,如想了解不同资料格式和写法,敬请关注!Download tips: This document is carefully compiled by theeditor. I hope that after you download them,they can help yousolve practical problems. The document can be customized andmodified after downloading,please adjust and use it according toactual needs, thank you!In addition, our shop provides you with various types ofpractical materials,such as educational essays, diaryappreciation,sentence excerpts,ancient poems,classic articles,topic composition,work summary,word parsing,copy excerpts,other materials and so on,want to know different data formats andwriting methods,please pay attention!差异蛋白 GO 和 KEGG 分析是生物信息学中常用的方法,用于研究蛋白质在生物过程、细胞组分和分子功能方面的差异,以及它们在代谢通路中的作用。
DAVIDMetascape:专注于基因功能注释和富集通路分析的网站
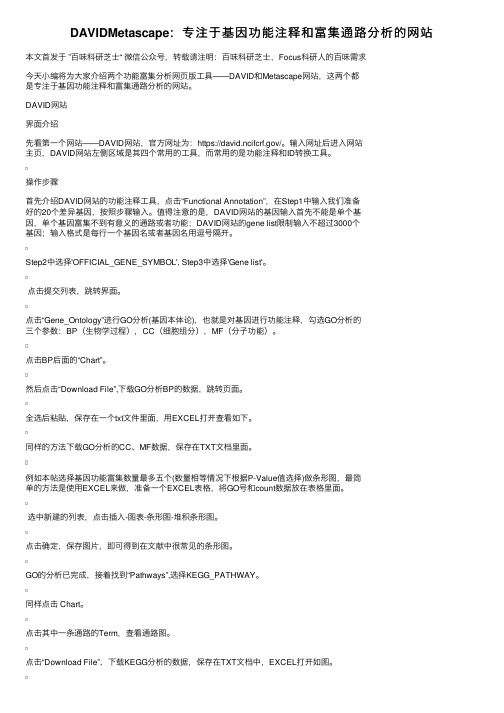
DAVIDMetascape:专注于基因功能注释和富集通路分析的⽹站本⽂⾸发于 ”百味科研芝⼠“ 微信公众号,转载请注明:百味科研芝⼠,Focus科研⼈的百味需求今天⼩编将为⼤家介绍两个功能富集分析⽹页版⼯具——DAVID和Metascape⽹站,这两个都是专注于基因功能注释和富集通路分析的⽹站。
DAVID⽹站界⾯介绍先看第⼀个⽹站——DAVID⽹站,官⽅⽹址为:https:///。
输⼊⽹址后进⼊⽹站主页,DAVID⽹站左侧区域是其四个常⽤的⼯具,⽽常⽤的是功能注释和ID转换⼯具。
操作步骤⾸先介绍DAVID⽹站的功能注释⼯具,点击“Functional Annotation”,在Step1中输⼊我们准备好的20个差异基因,按照步骤输⼊。
值得注意的是,DAVID⽹站的基因输⼊⾸先不能是单个基因,单个基因富集不到有意义的通路或者功能;DAVID⽹站的gene list限制输⼊不超过3000个基因;输⼊格式是每⾏⼀个基因名或者基因名⽤逗号隔开。
Step2中选择'OFFICIAL_GENE_SYMBOL', Step3中选择'Gene list'。
点击提交列表,跳转界⾯。
点击“Gene_Ontology”进⾏GO分析(基因本体论),也就是对基因进⾏功能注释,勾选GO分析的三个参数:BP(⽣物学过程),CC(细胞组分),MF(分⼦功能)。
点击BP后⾯的“Chart”。
然后点击“Download File”,下载GO分析BP的数据,跳转页⾯。
全选后粘贴,保存在⼀个txt⽂件⾥⾯,⽤EXCEL打开查看如下。
同样的⽅法下载GO分析的CC、MF数据,保存在TXT⽂档⾥⾯。
例如本帖选择基因功能富集数量最多五个(数量相等情况下根据P-Value值选择)做条形图,最简单的⽅法是使⽤EXCEL来做,准备⼀个EXCEL表格,将GO号和count数据放在表格⾥⾯。
选中新建的列表,点击插⼊-图表-条形图-堆积条形图。
DAVID使用方法介绍

DAVID使用说明文档一、DAVID简介DA VID (the Database for Annotation,Visualization and Integrated Discovery)的网址是/。
DA VID是一个生物信息数据库,整合了生物学数据和分析工具,为大规模的基因或蛋白列表(成百上千个基因ID或者蛋白ID列表)提供系统综合的生物功能注释信息,帮助用户从中提取生物学信息。
DA VID这个工具在2003年发布,目前版本是v6.7。
和其他类似的分析工具,如GoMiner,GOstat等一样,都是将输入列表中的基因关联到生物学注释上,进而从统计的层面,在数千个关联的注释中,找出最显著富集的生物学注释。
最主要是功能注释和信息链接。
二、分析工具:DAVID需要用户提供感兴趣的基因列表,在基因背景下,使用提供的分析工具,提取该列表中含有的生物信息。
这里说的基因列表和背景文件的选取对结果至关重要。
1.基因列表:这个基因列表可能是上游的生物信息分析产生的基因ID列表。
对于富集分析而言,一般情况下,大量的基因组成的列表有更高的统计意义,对富集程度高的特殊Terms有更高的敏感度。
富集分析产生的p-value在相同或者数量相同的基因列表中具有可比性。
DAVID对于基因列表的格式要求为每行一个基因ID或者是基因ID用逗号分隔开。
基因列表的质量会直接影响到分析结果。
这里定性给出好的基因列表应该具有的特点,一个好的基因列表至少要满足以下的大部分的要求:(1)包含与研究目的相关的大部分重要的基因(如标识基因)。
(2)基因的数量不能太多或者太少,一般是100至10000这个数量级。
(3)大部分基因可以较好的通过统计筛选,例如,在控制组和对照组样品间选择显著差异表达基因时,使用的t-test标准:fold changes >=2 && P-values <=0.05。
(4)大部分是上下调的基因都涉及到特定的某一生物过程,而不是随机的散布到所有可能的生物过程中。
kegg与go通路数据库介绍功能富集软件介绍

42
GO组成
GO提供了一系列的语义(terms)用来描述基因、基因 产物的特性。分三类:
1. 细胞组分(Cellular Component):用于描述亚细胞 结构、位置和大分子复 合物,如细胞核、端粒等; 2. 分子功能(Molecular Function):用于描述基因、 基因产物个体的功能,如酶活性,分子结合等;
41
GO 简介
GO (gene ontology)是基因本体联合会(Gene Ontology Consortium)所建立的数据库,旨在建立一个适用于各种 物种的,对在不同数据库中的基因和蛋白质产物进行限定 和一致性描述的,并能随着研究不断深入而更新的语义词 汇标准。 该数据库最初是由1998年对三个模式生物数据库的整合开 始:the FlyBase (果蝇数据库),the Saccharomyces Genome Database(酵母基因组数据库SGD) 和 the Mouse Genome Informatics(小鼠基因组数据库MGI)。随后,GO 不断发展扩大,现在已是包含多种动物、植物、微生物的 数据库。
2
下载数据
预处理的数据: E-GEOD 18842.processed.1.zip 原始数据: E-GEOD-18842.raw.1.zip E-GEOD-18842.raw.2.zip E-GEOD-18842.raw.3.zip 样本信息: E-GEOD-18842.sdrf.txt 平台信息: A-AFFY-44.adf.txt
3
芯片数据预处理步骤
1. 背景校正(Background Correction); 2. 标准化(Normalization); 3. 合并(Summary).
基因组学中的基因注释方法教程
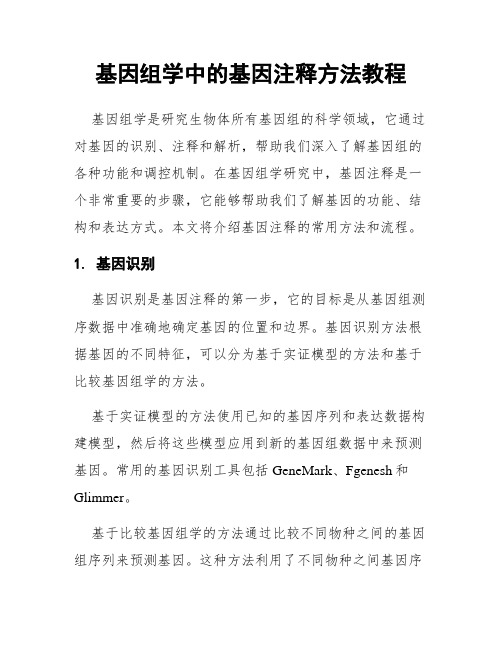
基因组学中的基因注释方法教程基因组学是研究生物体所有基因组的科学领域,它通过对基因的识别、注释和解析,帮助我们深入了解基因组的各种功能和调控机制。
在基因组学研究中,基因注释是一个非常重要的步骤,它能够帮助我们了解基因的功能、结构和表达方式。
本文将介绍基因注释的常用方法和流程。
1. 基因识别基因识别是基因注释的第一步,它的目标是从基因组测序数据中准确地确定基因的位置和边界。
基因识别方法根据基因的不同特征,可以分为基于实证模型的方法和基于比较基因组学的方法。
基于实证模型的方法使用已知的基因序列和表达数据构建模型,然后将这些模型应用到新的基因组数据中来预测基因。
常用的基因识别工具包括GeneMark、Fgenesh和Glimmer。
基于比较基因组学的方法通过比较不同物种之间的基因组序列来预测基因。
这种方法利用了不同物种之间基因序列的保守性,即功能相似的基因在不同物种之间会有相似的序列。
常用的基因识别工具包括Genewise、Exonerate和BLAST。
2. 基因结构预测基因结构预测是基因注释的重要环节,它的目标是确定基因的内含子、外显子和启动子区域等结构。
基因结构预测方法可以根据基因的序列和序列间相互作用信息来进行,常用的方法包括组学特征法、同源比对法和重叠法。
组学特征法通过分析基因组序列的物理和化学特性来预测基因结构。
这种方法利用基因的启动子、剪接位点、终止位点等特征来推测基因的结构。
常用的组学特征法包括激活一个氨基甘氨酸激酶(AUG)起始的编码区域(CDS)的请求(ATG)、剪接位点的提取和启动子的分析。
同源比对法通过比较不同物种之间的基因序列来推测基因的结构。
这种方法依赖于功能相似的基因在不同物种之间存在的序列保守性。
常用的同源比对工具包括Exonerate、BLAT和AUGUSTUS。
重叠法通过将碱基序列分割成多个片段(称为k-mers)并将它们与参考序列进行比对,来预测基因的结构。
这种方法利用重叠的片段来确定基因的边界和内含子和外显子的位置。
KEGG使用经验精品总结

KEGG使用经验精品总结KEGG(Kyoto Encyclopedia of Genes and Genomes)是一个综合性的数据库资源,旨在提供基因、基因组、化学物质和疾病等生物信息的综合分析平台。
在使用KEGG的过程中,我积累了一些经验和技巧,现总结如下:首先,在使用KEGG前,我们需要了解KEGG的内容和结构,以便更好地利用它来进行生物信息学分析。
KEGG主要包括四个主要数据库:基因数据库(包括基因组、序列、蛋白质等信息)、化学数据库(包括化合物、反应等信息)、组织和细胞数据库(包括组织和细胞的功能以及相关代谢通路)、人类疾病数据库(包括疾病的代谢通路及相关基因)。
熟悉这些数据库的内容和相互关系,有助于我们更好地理解和分析生物信息。
其次,使用KEGG进行基因或基因组的分析时,需要了解和掌握KEGG的工具和资源,如“BLAST KEGG”、“KEGG Mapper”等。
在基因或序列时,我们可以使用BLAST KEGG来进行相似序列的和比较,以获得更多有关该基因或序列的信息。
使用KEGG Mapper可以将基因或基因组与KEGG数据库中的通路或代谢网路进行关联分析,检查它们在生物过程中的功能和相互作用。
第三,使用KEGG时,要了解并熟悉KEGG的ID和命名规则,如基因的K号(K number)、基因家族(KO)等。
基因的K number是KEGG用于将基因与代谢通路进行关联的重要标识符。
在进行基因或基因组的分析时,我们可以通过查询基因或序列的K number,进一步了解它们在KEGG数据库中的相关功能和通路信息。
此外,了解基因家族的KO号也有助于我们了解该基因的分类和功能。
第四,使用KEGG时,还要善于使用可视化工具和图形表示方法,如KEGG地图、代谢通路图等。
KEGG地图是将基因、蛋白质、代谢物或化合物等按照空间或功能关系绘制成图形的一种方法,能够直观地展示生物多样性和复杂的相互作用关系。
- 1、下载文档前请自行甄别文档内容的完整性,平台不提供额外的编辑、内容补充、找答案等附加服务。
- 2、"仅部分预览"的文档,不可在线预览部分如存在完整性等问题,可反馈申请退款(可完整预览的文档不适用该条件!)。
- 3、如文档侵犯您的权益,请联系客服反馈,我们会尽快为您处理(人工客服工作时间:9:00-18:30)。
Linear or redundant chart report of annotation terms for all selected annotation categories above
Clustered or non-redundant chart report of annotation terms for all selected annotation categories above
involved in this annotation category
2 View and select
annotation categories of your interests
3 Single chart report
only for this Annotation categories
Table report for all Selected annotation categories
DAVID Gene Functional classification
The Functional Classification Tool generates a gene-to-gene similarity matrix based shared functional annotation using over 75,000 terms from 14 functional annotation sources. The novel clustering algorithms classifies highly related genes into functionally related groups.
than 0.9 are considered excellent.
Parameter Panel
Gene Clusters Identified by
DAVID
User’s gene IDs & Names
1
3 Percentage,e.g.44/163
3 Genes from the list
DAVID Gene ID Conversion Tool (DGCT)
If a significant portion (>20%) of input gene IDs fail to bemapped to an internal DAVID ID, a specially designed module, the DAVID Gene ID Conversion Tool, will start up to help map such IDs.
DAVID Gene Name Batch Viewer
1. The gene name batch viewer is able to quickly attach meaning to a list of gene IDs by rapidly translating them into their corresponding gene names.
Identify enriched biological themes, particularly GO terms Discover enriched functional-related gene groups Cluster redundant annotation terms Visualize genes on BioCarta & KEGG pathway maps Display related many-genes-to-many-terms on 2-D view. Search for other functionally related genes not in the list List interacting proteins Explore gene names in batch Link gene-disease associations Highlight protein functional domains and motifs Redirect to related literatures Convert gene identifiers from one type to another.
2. before proceeding to analysis with other more comprehensive analytic tools, investigators can quickly glance at the gene names to further gain insight abstions.
DAVID
Database for Annotation, Visualization and Integrated Discovery
DAVID provides a comprehensive set of functional annotation tools for investigators to understand biological meaning behind large list of genes
Gene List Manager
DAVID Analytic Modules
Any given gene is associating with a set of annotationterms. If genes share similar set of those terms, they are most likely involved in similar biologicalmechanisms. The algorithm adopts kappa statistics to quantitatively measure the degree of the agreement how genes share the similar annotation terms. Kappa result ranges from 0 to 1. The higher the value of Kappa, the stronger the agreement. Kappa more than 0.7 typically indicates that agreement of two genes are strong. Kappa values greater