基于python的小波阈值去噪算法
小波分析的语音信号噪声消除方法
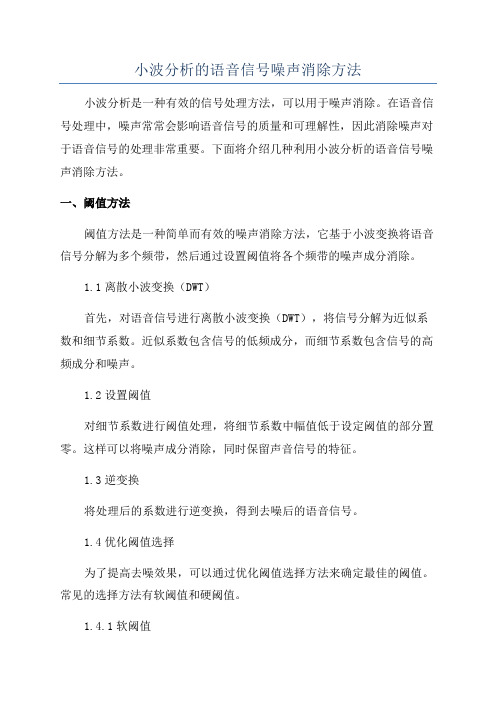
小波分析的语音信号噪声消除方法小波分析是一种有效的信号处理方法,可以用于噪声消除。
在语音信号处理中,噪声常常会影响语音信号的质量和可理解性,因此消除噪声对于语音信号的处理非常重要。
下面将介绍几种利用小波分析的语音信号噪声消除方法。
一、阈值方法阈值方法是一种简单而有效的噪声消除方法,它基于小波变换将语音信号分解为多个频带,然后通过设置阈值将各个频带的噪声成分消除。
1.1离散小波变换(DWT)首先,对语音信号进行离散小波变换(DWT),将信号分解为近似系数和细节系数。
近似系数包含信号的低频成分,而细节系数包含信号的高频成分和噪声。
1.2设置阈值对细节系数进行阈值处理,将细节系数中幅值低于设定阈值的部分置零。
这样可以将噪声成分消除,同时保留声音信号的特征。
1.3逆变换将处理后的系数进行逆变换,得到去噪后的语音信号。
1.4优化阈值选择为了提高去噪效果,可以通过优化阈值选择方法来确定最佳的阈值。
常见的选择方法有软阈值和硬阈值。
1.4.1软阈值软阈值将细节系数进行映射,对于小于阈值的细节系数,将其幅值缩小到零。
这样可以在抑制噪声的同时保留语音信号的细节。
1.4.2硬阈值硬阈值将细节系数进行二值化处理,对于小于阈值的细节系数,将其置零。
这样可以更彻底地消除噪声,但可能会损失一些语音信号的细节。
二、小波包变换小波包变换是对离散小波变换的改进和扩展,可以提供更好的频带分析。
在语音信号噪声消除中,小波包变换可以用于更精细的频带选择和噪声消除。
2.1小波包分解将语音信号进行小波包分解,得到多层的近似系数和细节系数。
2.2频带选择根据噪声和语音信号在不同频带上的能量分布特性,选择合适的频带对语音信号进行噪声消除。
2.3阈值处理对选定的频带进行阈值处理,将噪声成分消除。
2.4逆变换对处理后的系数进行逆变换,得到去噪后的语音信号。
三、小波域滤波小波域滤波是一种基于小波变换的滤波方法,通过选择合适的小波函数和滤波器来实现噪声消除。
基于小波变换的阈值图像去噪方法

关键词:图像去噪 小波变换 Radon 变换 脊波变换 阈值
山东科技大学硕士学位论文
目录
Abstract
Ima ge is an important carrier of the information as well as an important channel of acceeding informations. However, the ima ges are polluted by the noise or interferred by other non-target signa ls to different extents in ever y process of ima ge acquisition, transmission, and access. In order to obtain the ima ge informations more accurately, noise ima ge need to be denoised. Wavelet analysis is a new kind of frontier area. It has been attented extensively in signa l and ima ge de-noising while the wavelet analysis theor y is improving daily. This paper ma inly research on applica tion of the theor y of wavelet in ima ge de-noising,the ma in contents is as follows: In the previous three chapters of this paper, we introduce the status of ima ge de-noising ,the basic theor y of the waveletr analysis and the common ima ge-denoising algor ithms based on the wavelet transform. And we conclude the analysis and comparison about the three common methods of ima ge de-noising based on wavelet transform. In the forth chapter,beca use the algor ithm of ima ge de-noising based on orthogona l wavelet transform should make the Gibbs phenomenon and the common threshold usua lly cause the tendency of over strangled ing , we draw out the method of adaptive threshold ima ge denoising based on stationary wavelet transform .We give out the adeptive threshold by correcting the common threshold based on different scale and sub-band direction because the signa l and noise have different propagating character istics. We show that this algor ithm is reasonable and effective . In the fifth chapter, we introduce the rid gelet transform against the optima l basis of zerodimensiona l singula r objective function rather tha n the optima l basis of multi-dimensiona l objective function. Actually ridgelet is obtained by participating an orientation parpameter. The function of basis can describe the multi-dimensiona l singula r signa l along linear or hyperpla ne.We use ridgelet transform for ima ge denoising because the linear singula r of ima ge is express ed by less rid gelet coefficients.But noise do not have so significa nt coefficients.So we can obtain better effect by proposing the method of adaptive threshold ima ge de-noising based on rid gelet transform.We improve the common threshold according to the theory that the noise gradually weakened as the level of decomposition. Finally, we verify the effectiveness of this algor ithm by exper iments ,especia lly to the ima ge with features of linear singula rities.
Python小波变换去噪的原理解析

Python⼩波变换去噪的原理解析⼀.⼩波去噪的原理信号产⽣的⼩波系数含有信号的重要信息,将信号经⼩波分解后⼩波系数较⼤,噪声的⼩波系数较⼩,并且噪声的⼩波系数要⼩于信号的⼩波系数,通过选取⼀个合适的阀值,⼤于阀值的⼩波系数被认为是有信号产⽣的,应予以保留,⼩于阀值的则认为是噪声产⽣的,置为零从⽽达到去噪的⽬的。
⼩波阀值去噪的基本问题包括三个⽅⾯:⼩波基的选择,阀值的选择,阀值函数的选择。
(1) ⼩波基的选择:通常我们希望所选取的⼩波满⾜以下条件:正交性、⾼消失矩、紧⽀性、对称性或反对称性。
但事实上具有上述性质的⼩波是不可能存在的,因为⼩波是对称或反对称的只有Haar⼩波,并且⾼消失矩与紧⽀性是⼀对⽭盾,所以在应⽤的时候⼀般选取具有紧⽀的⼩波以及根据信号的特征来选取较为合适的⼩波。
(2) 阀值的选择:直接影响去噪效果的⼀个重要因素就是阀值的选取,不同的阀值选取将有不同的去噪效果。
⽬前主要有通⽤阀值(VisuShrink)、SureShrink阀值、Minimax阀值、BayesShrink阀值等。
(3) 阀值函数的选择:阀值函数是修正⼩波系数的规则,不同的反之函数体现了不同的处理⼩波系数的策略。
最常⽤的阀值函数有两种:⼀种是硬阀值函数,另⼀种是软阀值函数。
还有⼀种介于软、硬阀值函数之间的Garrote函数。
另外,对于去噪效果好坏的评价,常⽤信号的信噪⽐(SNR)与估计信号同原始信号的均⽅根误差(RMSE)来判断。
⼆,在python中使⽤⼩波分析进⾏阈值去噪声,使⽤pywt.threshold函数#coding=gbk#使⽤⼩波分析进⾏阈值去噪声,使⽤pywt.thresholdimport pywtimport numpy as npimport pandas as pdimport matplotlib.pyplot as pltimport mathdata = np.linspace(1, 10, 10)print(data)# [ 1. 2. 3. 4. 5. 6. 7. 8. 9. 10.]# pywt.threshold(data, value, mode, substitute) mode 模式有4种,soft, hard, greater, less; substitute是替换值可以点进函数⾥看,data/np.abs(data) * np.maximum(np.abs(data) - value, 0) data_soft = pywt.threshold(data=data, value=6, mode='soft', substitute=12)print(data_soft)# [12. 12. 12. 12. 12. 0. 1. 2. 3. 4.] 将⼩于6 的值设置为12,⼤于等于6 的值全部减去6data_hard = pywt.threshold(data=data, value=6, mode='hard', substitute=12)print(data_hard)# [12. 12. 12. 12. 12. 6. 7. 8. 9. 10.] 将⼩于6 的值设置为12,其余的值不变data_greater = pywt.threshold(data, 6, 'greater', 12)print(data_greater)# [12. 12. 12. 12. 12. 6. 7. 8. 9. 10.] 将⼩于6 的值设置为12,⼤于等于阈值的值不变化data_less = pywt.threshold(data, 6, 'less', 12)print(data_less)# [ 1. 2. 3. 4. 5. 6. 12. 12. 12. 12.] 将⼤于6 的值设置为12,⼩于等于阈值的值不变三,在python中使⽤ecg⼼电信号进⾏⼩波去噪实验#-*-coding:utf-8-*-import matplotlib.pyplot as pltimport pywtimport mathimport numpy as np#get Dataecg=pywt.data.ecg() #⽣成⼼电信号index=[]data=[]coffs=[]for i in range(len(ecg)-1):X=float(i)Y=float(ecg[i])index.append(X)data.append(Y)#create wavelet object and define parametersw=pywt.Wavelet('db8')#选⽤Daubechies8⼩波maxlev=pywt.dwt_max_level(len(data),w.dec_len)print("maximum level is"+str(maxlev))threshold=0 #Threshold for filtering#Decompose into wavelet components,to the level selected:coffs=pywt.wavedec(data,'db8',level=maxlev) #将信号进⾏⼩波分解for i in range(1,len(coffs)):coffs[i]=pywt.threshold(coffs[i],threshold*max(coeffs[i]))datarec=pywt.waverec(coffs,'db8')#将信号进⾏⼩波重构mintime=0maxtime=mintime+len(data)print(mintime,maxtime)plt.figure()plt.subplot(3,1,1)plt.plot(index[mintime:maxtime], data[mintime:maxtime])plt.xlabel('time (s)')plt.ylabel('microvolts (uV)')plt.title("Raw signal")plt.subplot(3, 1, 2)plt.plot(index[mintime:maxtime], datarec[mintime:maxtime])plt.xlabel('time (s)')plt.ylabel('microvolts (uV)')plt.title("De-noised signal using wavelet techniques")plt.subplot(3, 1, 3)plt.plot(index[mintime:maxtime],data[mintime:maxtime]-datarec[mintime:maxtime])plt.xlabel('time (s)')plt.ylabel('error (uV)')plt.tight_layout()plt.show()到此这篇关于Python⼩波变换去噪的⽂章就介绍到这了,更多相关Python⼩波去噪内容请搜索以前的⽂章或继续浏览下⾯的相关⽂章希望⼤家以后多多⽀持!。
小波阈值去噪算法
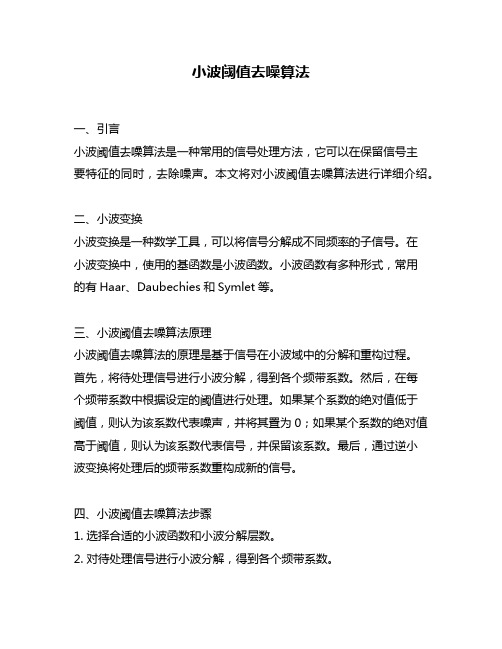
小波阈值去噪算法一、引言小波阈值去噪算法是一种常用的信号处理方法,它可以在保留信号主要特征的同时,去除噪声。
本文将对小波阈值去噪算法进行详细介绍。
二、小波变换小波变换是一种数学工具,可以将信号分解成不同频率的子信号。
在小波变换中,使用的基函数是小波函数。
小波函数有多种形式,常用的有Haar、Daubechies和Symlet等。
三、小波阈值去噪算法原理小波阈值去噪算法的原理是基于信号在小波域中的分解和重构过程。
首先,将待处理信号进行小波分解,得到各个频带系数。
然后,在每个频带系数中根据设定的阈值进行处理。
如果某个系数的绝对值低于阈值,则认为该系数代表噪声,并将其置为0;如果某个系数的绝对值高于阈值,则认为该系数代表信号,并保留该系数。
最后,通过逆小波变换将处理后的频带系数重构成新的信号。
四、小波阈值去噪算法步骤1. 选择合适的小波函数和小波分解层数。
2. 对待处理信号进行小波分解,得到各个频带系数。
3. 根据设定的阈值对每个频带系数进行处理。
4. 通过逆小波变换将处理后的频带系数重构成新的信号。
五、小波阈值去噪算法实现1. 选择合适的小波函数和小波分解层数。
常用的小波函数有Haar、Daubechies和Symlet等,选择不同的小波函数会影响到去噪效果。
一般来说,选择Daubechies或Symlet等多项式型小波函数效果较好。
选择合适的小波分解层数需要根据信号特点和噪声水平进行调整,通常在3~5之间。
2. 对待处理信号进行小波分解,得到各个频带系数。
可以使用MATLAB等软件实现。
3. 根据设定的阈值对每个频带系数进行处理。
阈值可以根据经验或者实验结果进行调整。
一般来说,可以选择软阈值或硬阈值方法进行处理。
4. 通过逆小波变换将处理后的频带系数重构成新的信号。
六、总结小波阈值去噪算法是一种常用的信号处理方法,可以在保留信号主要特征的同时,去除噪声。
实现小波阈值去噪算法需要选择合适的小波函数和小波分解层数,并对每个频带系数进行阈值处理。
基于小波阈值的图像去噪-毕业论文
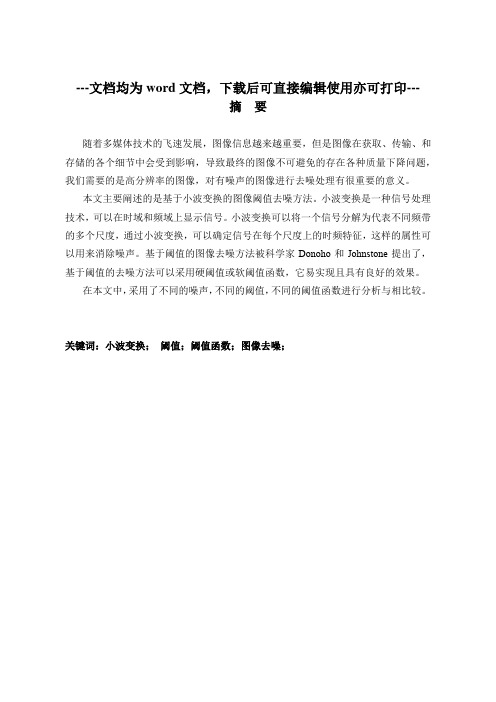
---文档均为word文档,下载后可直接编辑使用亦可打印---摘要随着多媒体技术的飞速发展,图像信息越来越重要,但是图像在获取、传输、和存储的各个细节中会受到影响,导致最终的图像不可避免的存在各种质量下降问题,我们需要的是高分辨率的图像,对有噪声的图像进行去噪处理有很重要的意义。
本文主要阐述的是基于小波变换的图像阈值去噪方法。
小波变换是一种信号处理技术,可以在时域和频域上显示信号。
小波变换可以将一个信号分解为代表不同频带的多个尺度,通过小波变换,可以确定信号在每个尺度上的时频特征,这样的属性可以用来消除噪声。
基于阈值的图像去噪方法被科学家Donoho和Johnstone提出了,基于阈值的去噪方法可以采用硬阈值或软阈值函数,它易实现且具有良好的效果。
在本文中,采用了不同的噪声,不同的阈值,不同的阈值函数进行分析与相比较。
关键词:小波变换;阈值;阈值函数;图像去噪;A b s t r a c tWith the rapid development of multimedia technology and network technology, image information becomes more and more important in people's work, study and life. But the image in the acquisition, transmission, and storage process sections will be affected seriously, which leads to the final image effected by all kinds of inevitable quality problems. but, which we need is the image with clearity and high resolution. Therefore, to deal with the noise of noisy images has very important meaning in practical application and life.There are a lot of methods for image de-noising. This paper mainly describes the image de-noising method based on wavelet transform. It is well known that wavelet transform is a signal processing technique which can display the signals on in both time and frequency domain. In this paper, we use several threshold based on wavelet transform to provide an enhanced approach for eliminating noise.Wavelet transforms can decompose a signal into several scales that represent different frequency band. The position of signal's instantaneous at each scale can be determined approximately by wavelet transform.Such a property can be used to denoise. Threshold-based de-noising method was proposed by Donoho. Threshold-based de-noising method is used hard-threshold or soft-threshold. It is very simple and has good performance. This paper uses the threshold techniques which applied threshold according to each band characteristic of image.In this paper, the results will be analyzed and compared for different noises, different thresholds, different threshold functions. It has a superior performance than traditional image de-noising method.Keyword:Wavelet Transform; Threshold; Threshold Function; Image De-noising第一章绪论1.1研究目的和意义当今各种信息充斥于我们的日常生活中,图像信息成为人类获取信息的重要信息,因为图像具有传输速度快,信息量大等一系列的强势[1]。
python 小波分解去除噪声

python 小波分解去除噪声
# 重构信号 denoised_signal = pywt.waverec(coeffs, wavelet)
# 绘制原始信号、带噪声信号和去噪后的信号 import matplotlib.pyplot as plt
plt.figure(figsize=(10, 6)) plt.subplot(3, 1, 1) plt.plot(signal) plt.title('Original Signal')
plt.subplot(3, 1, 2itle('Noisy Signal')
plt.subplot(3, 1, 3) plt.plot(denoised_signal) plt.title('Denoised Signal')
plt.tight_layout() plt.show() ```
# 进行小波分解 wavelet = 'db4' # 小波基函数选择 level = 6 # 分解的层数 coeffs = pywt.wavedec(noisy_signal, wavelet, level=level)
# 对每个尺度的系数进行去噪处理 threshold = np.std(coeffs[-1]) * np.sqrt(2 * np.log(len(noisy_signal))) # 设置阈值 coeffs = [pywt.threshold(c, threshold) for c in coeffs]
python 小波分解去除噪声
在Python中,可以使用PyWavelets库来进行小波分解和去噪处理。以下是一个简单的示
例代码:
```python import pywt import numpy as np
bayer小波变换去噪算法 python实现
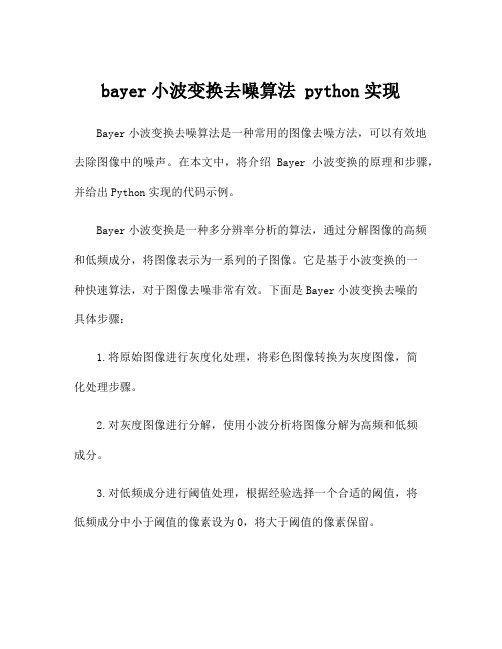
bayer小波变换去噪算法 python实现Bayer小波变换去噪算法是一种常用的图像去噪方法,可以有效地去除图像中的噪声。
在本文中,将介绍Bayer小波变换的原理和步骤,并给出Python实现的代码示例。
Bayer小波变换是一种多分辨率分析的算法,通过分解图像的高频和低频成分,将图像表示为一系列的子图像。
它是基于小波变换的一种快速算法,对于图像去噪非常有效。
下面是Bayer小波变换去噪的具体步骤:1.将原始图像进行灰度化处理,将彩色图像转换为灰度图像,简化处理步骤。
2.对灰度图像进行分解,使用小波分析将图像分解为高频和低频成分。
3.对低频成分进行阈值处理,根据经验选择一个合适的阈值,将低频成分中小于阈值的像素设为0,将大于阈值的像素保留。
4.对高频成分进行阈值处理,同样根据经验选择一个合适的阈值,将高频成分中小于阈值的像素设为0,将大于阈值的像素保留。
5.对处理后的图像进行逆小波变换,将图像恢复到原始大小。
6.重复以上步骤,直到达到期望的去噪效果。
下面是一个Bayer小波变换去噪算法的Python实现示例:```pythonimport numpy as npimport pywtimport cv2def bayer_denoising(image, threshold):#将原始图像转换为灰度图像gray_image = cv2.cvtColor(image, cv2.COLOR_BGR2GRAY)#对灰度图像进行Bayer小波变换coeffs = pywt.dwt2(gray_image, 'haar')cA, (cH, cV, cD) = coeffs#对低频成分进行阈值处理cA_thresh = pywt.threshold(cA, threshold)#对高频成分进行阈值处理cH_thresh = pywt.threshold(cH, threshold)cV_thresh = pywt.threshold(cV, threshold)cD_thresh = pywt.threshold(cD, threshold)#将阈值处理后的系数合并为一个列表coeffs_thresh = [cA_thresh, (cH_thresh, cV_thresh, cD_thresh)]#对处理后的系数进行逆变换,恢复图像大小denoised_image = pywt.idwt2(coeffs_thresh, 'haar') #将图像数据转换为uint8类型denoised_image = np.uint8(denoised_image)return denoised_image#读取图像image = cv2.imread('image.jpg')#设置阈值threshold = 30#调用Bayer小波变换去噪算法denoised_image = bayer_denoising(image, threshold) #显示原始图像和去噪后的图像cv2.imshow('Original Image', image)cv2.imshow('Denoised Image', denoised_image)cv2.waitKey(0)cv2.destroyAllWindows()```在上述代码中,首先使用`cv2.cvtColor()`函数将原始彩色图像转换为灰度图像,然后利用`pywt.dwt2()`函数对灰度图像进行Bayer小波变换。
小波去噪python代码
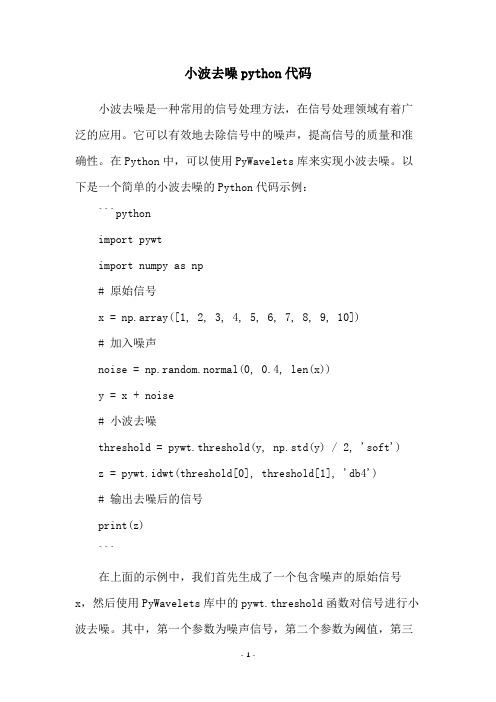
小波去噪python代码
小波去噪是一种常用的信号处理方法,在信号处理领域有着广泛的应用。
它可以有效地去除信号中的噪声,提高信号的质量和准确性。
在Python中,可以使用PyWavelets库来实现小波去噪。
以下是一个简单的小波去噪的Python代码示例:
```python
import pywt
import numpy as np
# 原始信号
x = np.array([1, 2, 3, 4, 5, 6, 7, 8, 9, 10])
# 加入噪声
noise = np.random.normal(0, 0.4, len(x))
y = x + noise
# 小波去噪
threshold = pywt.threshold(y, np.std(y) / 2, 'soft') z = pywt.idwt(threshold[0], threshold[1], 'db4')
# 输出去噪后的信号
print(z)
```
在上面的示例中,我们首先生成了一个包含噪声的原始信号x,然后使用PyWavelets库中的pywt.threshold函数对信号进行小波去噪。
其中,第一个参数为噪声信号,第二个参数为阈值,第三
个参数为阈值类型。
在本例中,我们选择了软阈值去噪方式。
最后,我们使用PyWavelets库中的pywt.idwt函数对去噪后的信号进行重构,得到了一个去噪后的信号z。
需要注意的是,在实际应用中,需要根据具体情况选择适合的小波基函数和阈值。
- 1、下载文档前请自行甄别文档内容的完整性,平台不提供额外的编辑、内容补充、找答案等附加服务。
- 2、"仅部分预览"的文档,不可在线预览部分如存在完整性等问题,可反馈申请退款(可完整预览的文档不适用该条件!)。
- 3、如文档侵犯您的权益,请联系客服反馈,我们会尽快为您处理(人工客服工作时间:9:00-18:30)。
基于python的小波阈值去噪算法
小波图像去噪原理
图像和噪声在经小波变换后具有不同的统计特性:图像本身的能量对应着幅值较大的小波系数,主要集中在低频(LL)部分;噪声能量则对应着幅值较小的小波系数,并分散在小波变换后的所有系数中。
基于此可设置一个合适的阈值门限,认为大于该阈值的小波系数的主要成份为有用的信号,给予收缩后保留;小于该阈值的小波系数,主要成份为噪声,予以置零剔除;然后经过阈值函数映射得到估计系数;最后对估计系数进行逆变换,就可以实现去噪和重建。
去噪时,通常认为低通系数含有大量的图像能量,一般不作处理,只对剩余三个高通部分进行处理。
一次阈值去噪并不能完全去除噪声,还需要对未作处理的低频部分(LL)再次进行小波分解和阈值去噪,直到实际图像与估计图像的偏差达到最小值。
但是,随着分解和去噪次数的增加,小波系数中的噪声能量越来越小,并且趋于分散,去噪的效果将逐渐降低。
一般来说,进行3-4层小波分解和去噪就可以达到满意的去噪效果。
小波阈值去噪步骤
(1)二维信号的小波分解。
选择一个小波(sym8)和小波分解的层次
N(3),然后计算信号S到第N层的分解。