面板数据分位数回归的一个简单方法
面板数据的分位回归方法及其模拟研究三

面板数据的分位回归方法及其模拟研究(三)罗幼喜田茂再2012-10-15 10:50:32 来源:《统计研究》(京)2010年10期第81~87页四、真实数据分析我们以2004-2008年我国各地区城镇居民人均可支配收入x(元)和消费支出y(元)的面板数据为例,利用上述提出的分位回归方法对近5年来我国城镇居民收入消费模式进行建模分析,探讨居民人均可支配收入x对其消费支出y的影响,数据来源于《中国统计年鉴(2005-2009)》。
通过对总体数据作散点图容易看到y与x之间有比较明显的线性关系,所以可以考虑采用线性模型来刻画。
而通过横向散点图可以看到各地区y与x的斜率变化不大,但截距却有明显的不同,即各地区平均边际消费倾向差异不大,但自发消费存在着明显差异;从纵向散点图可以看到各年度y与x的关系基本相同,无论是斜率还是截距都没有发生太大变化,所以可以认为不存在时期效应。
首先考虑直接利用混合数据建立简单线性模型:LS估计的结果如表6。
表6LS估计值及显著性检验虽然从表6的结果来看,模型和参数都通过了显著性检验,可决系数也比较高,但残差分析图显示方差并不相等,而且残差值波动比较大,拟合效果并不好,这有可能是由于LS估计没有照顾到各地区可能存在的个体差异而引起的。
另外91号数据(广东省2008年)、92号数据(广东省2007年)和129号数据(西藏2005年)残差表现异常,这也使得上述β的LS估计在这些异常点的强影响下可能错估了平均边际消费水平。
考虑带个体固定效应的模型:从第三节的蒙特卡洛模拟结果来看,当模型判断正确时,FE估计是能够极大地改进LS估计的,对参数β的估计也具有较高的精度和稳定性,所以我们首先用FE估计法对参数进行估计,结果如下:表7FE估计值及显著性检验从表7结果来看,F值显著增加,模型的拟合优度也提高了很多(当然这也有部分原因是由于我们加入了新的解释变量而引起的)β的估计值也高度显著,残差分析图显示残差方差异常的情况消失了,而且残差呈现正态分布。
分位数回归方法及应用PPT18页
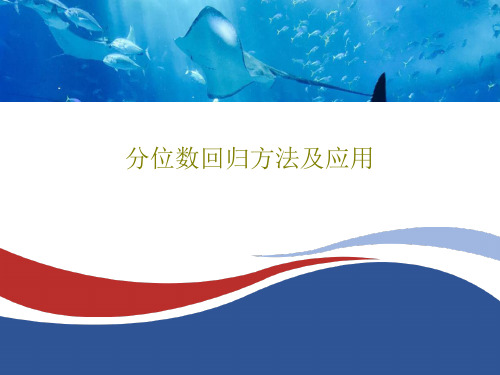
▪
26、要使整个人生都过得舒适、愉快,这是不可能的,因为人类必须具备一种能应付逆境的态度。——卢梭
▪
Байду номын сангаас
27、只有把抱怨环境的心情,化为上进的力量,才是成功的保证。——罗曼·罗兰
▪
28、知之者不如好之者,好之者不如乐之者。——孔子
▪
29、勇猛、大胆和坚定的决心能够抵得上武器的精良。——达·芬奇
▪
30、意志是一个强壮的盲人,倚靠在明眼的跛子肩上。——叔本华
谢谢!
18
分位数回归方法及应用
16、人民应该为法律而战斗,就像为 了城墙 而战斗 一样。 ——赫 拉克利 特 17、人类对于不公正的行为加以指责 ,并非 因为他 们愿意 做出这 种行为 ,而是 惟恐自 己会成 为这种 行为的 牺牲者 。—— 柏拉图 18、制定法律法令,就是为了不让强 者做什 么事都 横行霸 道。— —奥维 德 19、法律是社会的习惯和思想的结晶 。—— 托·伍·威尔逊 20、人们嘴上挂着的法律,其真实含 义是财 富。— —爱献 生
分位数回归 分样本回归

分位数回归分样本回归一、基本概念分位数回归是一种回归分析方法,与传统的OLS回归不同,它能够揭示自变量对因变量在不同分位数上的影响。
在传统OLS回归中,我们只能得到因变量均值对自变量的影响,而分位数回归则能提供更加全面的信息。
分位数是指将一组数据按大小顺序排列后,划分为若干等份,每一份包含相同比例的数据。
例如,25%分位数就是将数据分为四份,每一份包含25%的数据。
二、应用场景分位数回归在经济学、社会科学等领域具有广泛的应用。
它能够帮助研究人员了解不同自变量对因变量在不同分位数上的影响程度,从而更好地理解数据背后的规律。
例如,在研究收入不平等问题时,传统OLS回归只能告诉我们收入水平对平均收入的影响,而分位数回归可以告诉我们不同收入水平下收入的分布情况,从而更全面地了解收入分配状况。
三、分位数回归分析步骤进行分位数回归分析一般需要以下几个步骤:1. 数据准备:收集所需的自变量和因变量数据,并进行必要的数据清洗和变量转换。
2. 模型设定:根据研究问题和数据特点,选择适当的分位数回归模型。
常用的模型包括线性分位数回归模型和非线性分位数回归模型。
3. 估计模型参数:利用最小二乘法或其他合适的方法,对模型进行估计,并得到各个分位数上的回归系数。
4. 模型诊断:对估计结果进行检验,包括残差分析、异方差性检验等,以确保模型的可靠性和有效性。
5. 结果解释:根据估计结果,解释自变量对因变量在不同分位数上的影响情况。
可以通过绘制分位数回归曲线或计算边际效应来展示结果。
四、分位数回归的优势和局限性分位数回归具有以下优势:1. 揭示了因变量在不同分位数上的影响情况,提供了更加全面的信息。
2. 对于非线性关系的建模更加灵活,适用性广泛。
然而,分位数回归也存在一些局限性:1. 对于数据量较小的样本,分位数回归可能会产生估计不准确的问题。
2. 分位数回归依赖于分位数的选择,不同的分位数选择可能会导致不同的结果。
五、总结分位数回归是一种重要的回归分析方法,能够揭示自变量对因变量在不同分位数上的影响。
【实证方法】分位数回归(QuantileRegression)
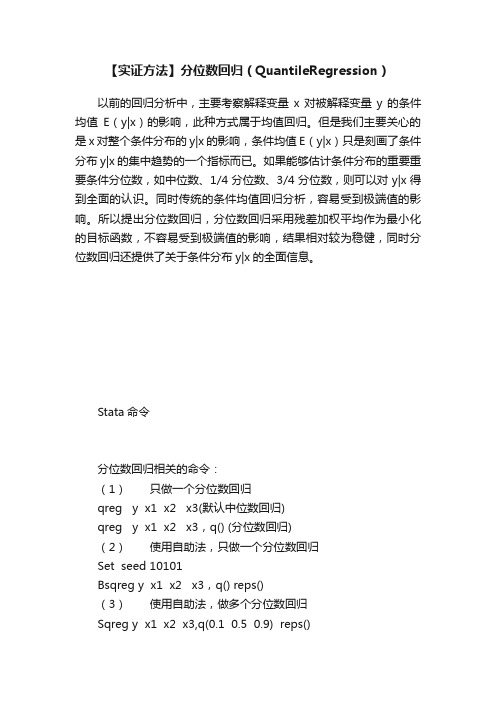
【实证方法】分位数回归(QuantileRegression)
以前的回归分析中,主要考察解释变量x对被解释变量y的条件均值E(y|x)的影响,此种方式属于均值回归。
但是我们主要关心的是x对整个条件分布的y|x的影响,条件均值E(y|x)只是刻画了条件分布y|x的集中趋势的一个指标而已。
如果能够估计条件分布的重要重要条件分位数,如中位数、1/4分位数、3/4分位数,则可以对y|x得到全面的认识。
同时传统的条件均值回归分析,容易受到极端值的影响。
所以提出分位数回归,分位数回归采用残差加权平均作为最小化的目标函数,不容易受到极端值的影响,结果相对较为稳健,同时分位数回归还提供了关于条件分布y|x的全面信息。
Stata命令
分位数回归相关的命令:
(1)只做一个分位数回归
qreg y x1 x2 x3(默认中位数回归)
qreg y x1 x2 x3,q() (分位数回归)
(2)使用自助法,只做一个分位数回归
Set seed 10101
Bsqreg y x1 x2 x3,q() reps()
(3)使用自助法,做多个分位数回归
Sqreg y x1 x2 x3,q(0.1 0.5 0.9) reps()
检验系数是否相等
Test [q10=q50=q90]:x1 (4)图形比较
安装grqreg命令
Set seed 10101
Bsqreg y x1 x2 x3,reps() q() Grqreg ,cons ci ols olsci
例证。
我国碳金融市场中碳交易价格的影响因素分析——基于面板分位数模型
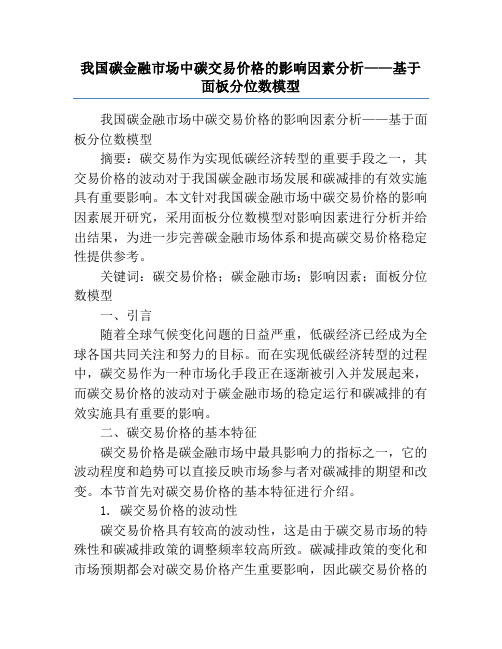
我国碳金融市场中碳交易价格的影响因素分析——基于面板分位数模型我国碳金融市场中碳交易价格的影响因素分析——基于面板分位数模型摘要:碳交易作为实现低碳经济转型的重要手段之一,其交易价格的波动对于我国碳金融市场发展和碳减排的有效实施具有重要影响。
本文针对我国碳金融市场中碳交易价格的影响因素展开研究,采用面板分位数模型对影响因素进行分析并给出结果,为进一步完善碳金融市场体系和提高碳交易价格稳定性提供参考。
关键词:碳交易价格;碳金融市场;影响因素;面板分位数模型一、引言随着全球气候变化问题的日益严重,低碳经济已经成为全球各国共同关注和努力的目标。
而在实现低碳经济转型的过程中,碳交易作为一种市场化手段正在逐渐被引入并发展起来,而碳交易价格的波动对于碳金融市场的稳定运行和碳减排的有效实施具有重要的影响。
二、碳交易价格的基本特征碳交易价格是碳金融市场中最具影响力的指标之一,它的波动程度和趋势可以直接反映市场参与者对碳减排的期望和改变。
本节首先对碳交易价格的基本特征进行介绍。
1. 碳交易价格的波动性碳交易价格具有较高的波动性,这是由于碳交易市场的特殊性和碳减排政策的调整频率较高所致。
碳减排政策的变化和市场预期都会对碳交易价格产生重要影响,因此碳交易价格的波动也是合理的。
2. 碳交易价格的季节性变化碳交易价格在不同季节会出现不同程度的变化。
一方面,季节性能源需求的变化会导致碳交易价格在不同季节呈现出不同的走势。
另一方面,碳减排政策的执行和监管也会随着季节的变化而有所差异,从而对碳交易价格产生影响。
三、碳交易价格的影响因素碳交易价格的波动和变化受到诸多因素的影响。
本节主要分析了一些重要的影响因素,并通过面板分位数模型进行量化和分析。
1. 宏观经济因素宏观经济因素对碳交易价格具有重要的影响作用。
经济增长水平、人均收入水平、产业结构等因素都会对碳交易价格产生影响。
通过分析宏观经济变量与碳交易价格的相关性,可以更好地理解宏观经济对碳交易价格的影响。
我国农业保险发展影响因素的实证检验——基于面板数据分位数回归方法
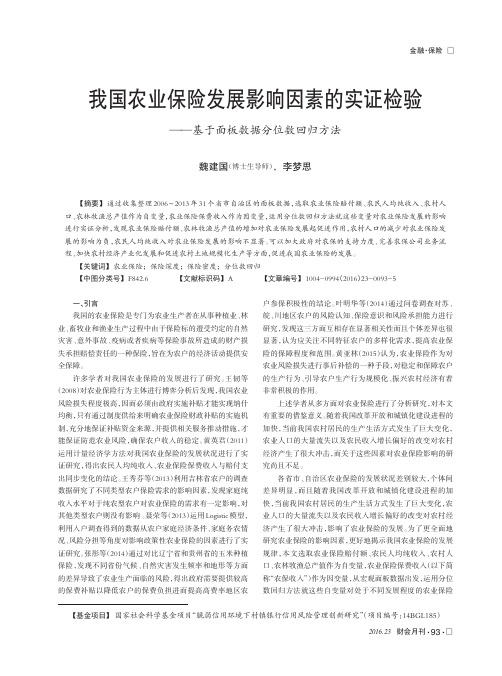
一、引言我国的农业保险是专门为农业生产者在从事种植业、林业、畜牧业和渔业生产过程中由于保险标的遭受约定的自然灾害、意外事故、疫病或者疾病等保险事故所造成的财产损失承担赔偿责任的一种保险,旨在为农户的经济活动提供安全保障。
许多学者对我国农业保险的发展进行了研究。
王韧等(2008)对农业保险行为主体进行博弈分析后发现,我国农业风险损失程度极高,因而必须由政府实施补贴才能实现纳什均衡,只有通过制度供给来明确农业保险财政补贴的实施机制,充分地保证补贴资金来源,并提供相关服务推动措施,才能保证防范农业风险,确保农户收入的稳定。
黄英君(2011)运用计量经济学方法对我国农业保险的发展状况进行了实证研究,得出农民人均纯收入、农业保险保费收入与赔付支出同步变化的结论。
王秀芬等(2013)利用吉林省农户的调查数据研究了不同类型农户保险需求的影响因素,发现家庭纯收入水平对于纯农型农户对农业保险的需求有一定影响,对其他类型农户则没有影响。
聂荣等(2013)运用Logistic 模型,利用入户调查得到的数据从农户家庭经济条件、家庭务农情况、风险分担等角度对影响政策性农业保险的因素进行了实证研究。
张彤等(2014)通过对比辽宁省和贵州省的玉米种植保险,发现不同省份气候、自然灾害发生频率和地形等方面的差异导致了农业生产面临的风险,得出政府需要提供较高的保费补贴以降低农户的保费负担进而提高高费率地区农户参保积极性的结论。
叶明华等(2014)通过问卷调查对苏、皖、川地区农户的风险认知、保险意识和风险承担能力进行研究,发现这三方面互相存在显著相关性而且个体差异也很显著,认为应关注不同特征农户的多样化需求,提高农业保险的保障程度和范围。
黄亚林(2015)认为,农业保险作为对农业风险损失进行事后补偿的一种手段,对稳定和保障农户的生产行为、引导农户生产行为规模化、振兴农村经济有着非常积极的作用。
上述学者从多方面对农业保险进行了分析研究,对本文有重要的借鉴意义。
分位数回归及应用简介

分位数回归及应用简介分位数回归(Quantile Regression)是一种预测模型,与传统的最小二乘法回归(OLS regression)不同,它不仅可以估计数据的均值,还可以估计数据分布的其他分位数。
这种方法在处理不同分位数下的潜在差异时非常有用,因为它可以提供理解和预测在不同条件下的数据变化情况。
最小二乘法回归通过最小化预测值与实际值的平方差,给出一个数据分布的均值估计。
然而,由于数据的分布可能是非对称的,存在异常值或极端值,使用最小二乘法回归的均值估计可能不准确。
在这种情况下,分位数回归是一种更好的方法,因为它可以估计多个分位数,包括中位数(50%分位数)和极值(例如90%或95%分位数)。
分位数回归可以通过最小化损失函数来估计模型参数,常用的损失函数是加权绝对值损失函数。
这个损失函数对应的优化问题可以使用线性规划或非线性规划的方法求解。
通过计算不同分位数的估计结果,可以获得数据分布的详细信息。
分位数回归有一些应用的优势。
首先,它可以提供更全面的数据估计,对于非对称或含有异常值的数据分布具有更好的预测能力。
其次,分位数估计结果可以用来比较不同分位数处的特征变量对因变量的影响程度。
例如,在收入预测模型中,分位数回归可以帮助我们比较高收入人群和低收入人群对某个特征变量的影响程度。
此外,分位数回归还可以用于分析不同条件下的潜在差异,例如预测某个特征变量在不同行业、不同地区或不同时间段的变化情况。
分位数回归的应用非常广泛。
在经济学领域,它常被用于研究收入分布、贫富差距以及社会流动性等问题。
它还可以用于金融学中的风险评估和资产定价分析,其中分位数回归可以帮助我们理解极端事件的风险程度。
此外,分位数回归还可以在医学和社会科学领域中,用于研究不同群体或个体的特征与某个健康指标或社会指标的关系。
尽管分位数回归有许多优点,但也存在一些限制。
首先,分位数回归对于数据分布的假设较少,因此可以适用于各种类型的数据。
分位数回归参数估计 -回复
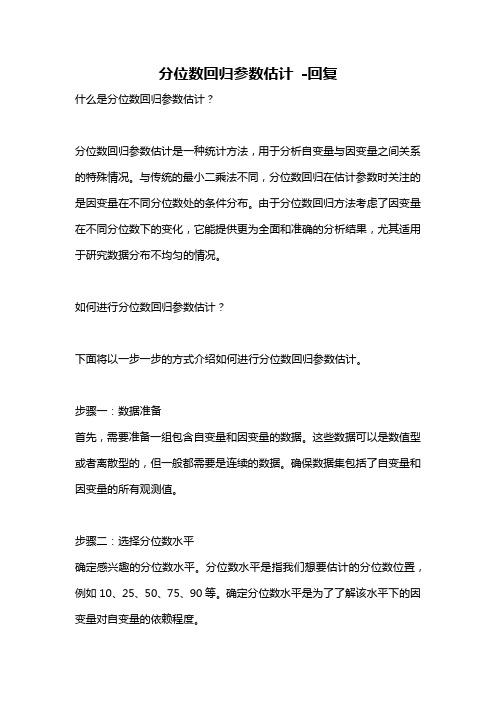
分位数回归参数估计-回复什么是分位数回归参数估计?分位数回归参数估计是一种统计方法,用于分析自变量与因变量之间关系的特殊情况。
与传统的最小二乘法不同,分位数回归在估计参数时关注的是因变量在不同分位数处的条件分布。
由于分位数回归方法考虑了因变量在不同分位数下的变化,它能提供更为全面和准确的分析结果,尤其适用于研究数据分布不均匀的情况。
如何进行分位数回归参数估计?下面将以一步一步的方式介绍如何进行分位数回归参数估计。
步骤一:数据准备首先,需要准备一组包含自变量和因变量的数据。
这些数据可以是数值型或者离散型的,但一般都需要是连续的数据。
确保数据集包括了自变量和因变量的所有观测值。
步骤二:选择分位数水平确定感兴趣的分位数水平。
分位数水平是指我们想要估计的分位数位置,例如10、25、50、75、90等。
确定分位数水平是为了了解该水平下的因变量对自变量的依赖程度。
步骤三:估计分位数回归参数通过最小化分位数损失函数来估计分位数回归参数。
分位数损失函数是通过比较实际观测值和分位数预测值的差异来衡量模型的拟合度。
一般情况下,我们使用最小绝对偏差估计法来估计分位数回归参数,这是一种鲁棒性较好的方法。
步骤四:进行推断分析在获得估计的参数后,可以进行推断分析,包括参数的显著性检验、置信区间估计等。
这些分析可以帮助我们对模型的有效性和参数的可信度进行评估。
步骤五:解释分位数回归结果最后,根据估计的分位数回归参数,解释自变量对因变量的影响。
可以分析不同分位数水平下自变量变化对因变量变化的影响程度。
这对于研究因变量分布中的特殊部分非常有用。
为什么使用分位数回归参数估计?传统的最小二乘法在估计参数时假设了因变量的条件分布是对称的,而分位数回归方法不对分布进行任何假设,能够更好地适应于现实世界中的数据分布。
因此,分位数回归方法在研究中应用广泛。
分位数回归参数估计能够帮助我们了解自变量对因变量的影响在不同分位数下的差异。
这对于研究数据的潜在机制和不同部分之间的关系具有重要意义。