共同基金绩效的持续性分析
基金管理外文文献翻译
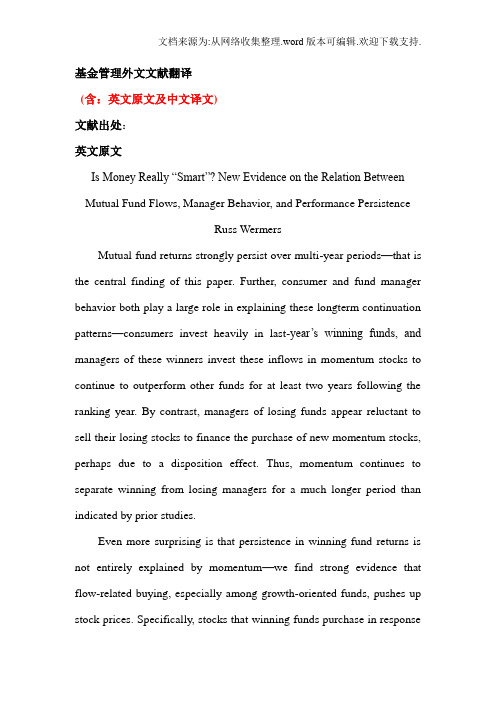
基金管理外文文献翻译(含:英文原文及中文译文)文献出处:英文原文Is Money Really “Smart”? New Evidence on the Relation Between Mutual Fund Flows, Manager Behavior, and Performance PersistenceRuss WermersMutual fund returns strongly persist over multi-year periods—that is the central finding of this paper. Further, consumer and fund manager behavior both play a large role in explaining these longterm continuation patterns—consumers invest heavily in last-year’s winning funds, and managers of these winners invest these inflows in momentum stocks to continue to outperform other funds for at least two years following the ranking year. By contrast, managers of losing funds appear reluctant to sell their losing stocks to finance the purchase of new momentum stocks, perhaps due to a disposition effect. Thus, momentum continues to separate winning from losing managers for a much longer period than indicated by prior studies.Even more surprising is that persistence in winning fund returns is not entirely explained by momentum—we find strong evidence that flow-related buying, especially among growth-oriented funds, pushes up stock prices. Specifically, stocks that winning funds purchase in responseto persistent flows have returns that beat their size, book-to-market, and momentum benchmarks by two to three percent per year over a four-year period. Cross-sectional regressions indicate that these abnormal returns are strongly related to fund inflows, but not to the past performance of the funds—thus, casting some doubt on prior findings of persistent manager talent in picking stocks. Finally, at the style-adjusted net returns level, we find no persistence, consistent with the results of prior studies. On balance, we confirm that money is smart in chasing winning managers, but that a “copycat” s trategy of mimicking winning fund stock trades to take advantage of flow-related returns appears to be the smartest strategy.Eighty-eight million individuals now hold investments in U.S. mutual funds, with over 90 percent of the value of these investments being held in actively managed funds. Further, actively managed equity funds gain the lion’s share of consumer inflows—flows of net new money to equity funds (inflows minus outflows) totalled $309 billion in 2000, pushing the aggregate value of investments held by these funds to almost $4 trillion at year-end 2000. While the majority of individual investors apparently believe in the virtues of active management in general, many appear to hold even stronger beliefs concerning the talents of subgroups of fund managers—they appear to believe that, among the field of active managers, superior managers exist that can “beat the market” for long periods of time. In particular, Morningstar and Lipper compete vigorouslyfor the attention of these true believers by providing regular fund performance rankings, while popular publications such as Money Magazine routinely profile “star” mutual fund managers. In addition, investor dollars, while not very quick to abandon past losing funds, aggressively chase past winners (see, for example, Sirri and Tufano (1998)).Are these “performance-chasers” wasting their money and time, or is money “smart”? Several past papers have attempted to tackle this issue, with somewhat differing results. For example, Grinblatt and Titman (1989a, 1993) find that some mutual fund managers are able to consistently earn positive abnormal returns before fees and expenses, while Brown and Goetzmann (1995; BG) attribute persistence to inferior funds consistently earning negative abnormal returns. Gruber (1996) and Zheng (1999) examine persistence from the viewpoint of consumer money flows to funds, and find that money is “smart”—that is, money flows disproportionately to funds exhibiting superior future returns. However, the exact source of the smart money effect remains a puzzle—does smart money capture manager talent or, perhaps, simply momentum in stock returns?1 More recently, Carhart (1997) examines the persistence in net returns of U.S. mutual funds, controlling for the continuation attributable to priced equity styles (see, for example, Fama and French (1992, 1993, 1996), Jegadeesh and Titman (1993), Daniel andTitman (1997), and Moskowitz and Grinblatt (1999)). Carhart finds little evidence of superior funds that consistently outperform their style benchmarks—specifically, Carhart finds that funds in the highest net return decile (of the CRSP mutual fund database) during one year beat funds in the lowest decile by about 3.5 percent during the following year, almost all due to the one-year momentum effect documented by Jegadeesh and Titman (1993) and to the unexplained poor performance of funds in the lowest prior-year return decile.2 Thus, Carhart (1997) suggests that money is not very smart. Recent studies find somewhat more promising results than Carhart (1997). Chen, Jegadeesh, and Wermers (1999) find that stocks most actively purchased by funds beat those most actively sold by over two percent per year, while Bollen and Busse (2002) find evidence of persistence in quarterly fund performance. Wermers (2000) finds that, although the average style-adjusted net return of the average mutual fund is negative (consistent with Carhart’s study), high-turnover funds exhibit a net return that is significantly higher than low-turnover funds. In addition, these highturnover funds pick stocks well enough to cover their costs, even adjusting for style-based returns. This finding suggests that fund managers who trade more frequently have persistent stockpicking talents. All of these papers provide a more favorable view of the average actively managed fund than prior research, although none focus on the persistence issue with portfolio holdings data.This study examines the mutual fund persistence issue using both portfolio holdings and net returns data, allowing a more complete analysis of the issue than past studies. With these data, we develop measures that allow us to examine the roles of consumer inflows and fund manager behavior in the persistence of fund performance. Specifically, we decompose the returns and costs of each mutual fund into that attributable to (1) manager skills in picking stocks having returns that beat their style-based benchmarks (selectivity), (2) returns that are attributable to the characteristics (or style) of stockholdings, (3) trading costs, (4) expenses, and (5) costs that are associated with the daily liquidity offered by funds to the investing public (as documented by Edelen (1999)). Further, we construct holdings-based measures of momentum-investing behavior by the fund managers. Together, these measures allow an examination of the relation between flows, manager behavior, and performance persistence.In related work, Sirri and Tufano (1998) find that consumer flows react about as strongly to one-year lagged net returns as to any other fund characteristic. In addition, the model of Lynch and Musto (2002) predicts that performance repeats among winners (but not losers), while the model of Berk and Green (2002) predicts no persistence (or weak persistence) as consumer flows compete away any managerial talent. Consistent with Sirri and Tufano (1998), and to test the competing viewpoints of Lynchand Musto (2002) and Berk and Green (2002), we sort funds on their one-year lagged net returns for most tests in this paper. While other ways of sorting funds are attempted.DataWe merge two major mutual fund databases for our analysis of mutual fund performance. Details on the process of merging these databases is available in Wermers (2000). The first database contains quarterly portfolio holdings for all U.S. equity mutual funds existing at any time between January 1, 1975 and December 31, 1994; these data were purchased from Thomson/CDA of Rockville, Maryland. The CDA dataset lists the equity portion of each fund’s holdings (i.e., the shareholdings of each stock held by that fund) along with a listing of the total net assets under management and the self-declared investment objective at the beginning of each calendar quarter. CDA began collecting investment-objective information on June 30, 1980; we supplement these data with hand-collected investment objective data from January 1, 1975.The second mutual fund database is available from the Center for Research in Security Prices (CRSP) and is used by Carhart (1997). The CRSP database contains monthly data on net returns, as well as annual data on portfolio turnover and expense ratios for all mutual funds existing at any time between January 1, 1962 and December 31, 2000. Further details on the CRSP mutual fund database are available from CRSP.These two databases were merged to provide a complete record of the stockholdings of a given fund, along with the fund’s turnover, expense ratio, net returns, investment objective, and total net assets under management during the entire time that the fund existed during our the period of 1975 to 1994 (inclusive).5 Finally, stock prices and returns were obtained from the CRSP stock files.Performance-Decomposition Methodology In this study, we use several measures that quantify the ability of a mutual fund manager to choose stocks, as well as to generate superior performance at the net return level. These measures, in general, decompose the return of the stocks held by a mutual fund into several components in order to both benchmark the stock portfolio and to provide a performance attribution for the fund. The measures used to decompose fund returns include:1. the portfolio-weighted return on stocks currently held by the fund, in excess of returns (during the same time period) on matched control portfolios having the same style characteristics (selectivity)2. the portfolio-weighted return on control portfolios having the same characteristics as stocks currently held by the fund, in excess of time-series average returns on those control portfolios (style timing)3. the time-series average returns on control portfolios having the same characteristics as stocks currently held (style-based returns)4. the execution costs incurred by the fund5. the expense ratio charged by the fund6. the net returns to investors in the fund, in excess of the returns to an appropriate benchmark portfolio.The first three components of performance, which decompose the return on the stocks held by a given mutual fund before any trading costs or expenses are considered, are briefly described next. We estimate the execution costs of each mutual fund during each quarter by applying recent research on institutional trading costs to our stockholdings data—we also describe this procedure below. Data on expense ratios and net returns are obtained directly from the merged mutual fund database. Finally, we describe the Carhart (1997) regression-based performance measure, which we use to benchmark-adjust net returns.The Ferson-Schadt Measure Ferson and Schadt (FS, 1996) develop a conditional performance measure at the net returns level. In essence, this measure identifies a fund manager as providing value if the manager provides excess net returns that are significantly higher than the fund’s matched factor benchmarks, both unconditional and conditional. The conditional benchmarks control for any predictability of the factor return premia that is due to evolving public information. Managers, therefore, are only labeled as superior if they possess superior private information on stock prices, and not if they change factor loadings over time in response to public information. FS also find that these conditionalbenchmarks help to control for the response of consumer cashflows to mutual funds. For example, when public information indicates that the market return will be unusually high, consumers invest unusually high amounts of cash into mutual funds, which reduces the performance measure, “alpha,” from an unconditional model (such as the Carhart model). This reduction in alpha occurs because the unconditional model does not control for the negative market timing induced by the flows. Edelen (1999) provides further evidence of a negative impact of flows on measured fund performance. Using the FS model mitigates this flow-timing effect. The version of the FS model used in this paper starts with the unconditional Carhart four-factor model and adds a market factor that is conditioned on the five FS economic variables.Decomposing the Persistence in Mutual Fund ReturnsSirri and Tufano (1998) find that consumer flows react about as strongly to one-year lagged net returns as to any other fund characteristic. In addition, the model of Lynch and Musto (2002) predicts that performance repeats among winners (but not losers), while the model of Berk and Green (2002) predicts no persistence (or weak persistence) as consumer flows compete away any managerial talent. Consistent with Sirri and Tufano (1998), and to test the competing viewpoints of Lynch and Musto (2002) and Berk and Green (2002), we sort funds on their one-year lagged net returns for the majority of tests in the remainder ofthis paper. When appropriate, we provide results for other sorting approaches as well.中文译文资金真的是“聪明”吗?关于共同基金流动,经理行为和绩效持续性关系的新证据作者:Russ Wermers此外,基金的复苏在多年期间强烈持续- 这是本文的核心发现。
如何评估基金的绩效

如何评估基金的绩效基金作为一种投资工具,受到越来越多投资者的关注。
而对于投资者来说,评估基金的绩效是非常重要的,因为绩效将直接影响到投资者的收益。
本文将介绍几种评估基金绩效的常用方法,并分析其优缺点。
一、评估基金绩效的指标在评估基金绩效时,投资者可以参考以下几个常用的指标:1. 收益率收益率是评估基金绩效的最直观指标之一。
投资者可以通过计算基金的累计或年化收益率来了解基金的投资回报情况。
通过对比不同基金的收益率,投资者可以判断基金的盈利能力。
2. 风险指标除了收益率,投资者还需要考虑基金的风险情况。
常见的风险指标包括波动率、下行风险等。
波动率可以反映基金净值的波动情况,下行风险则主要关注基金在市场下跌时的表现。
通过分析基金的风险指标,投资者可以评估基金在不同市场环境下的表现能力。
3. 基金经理能力基金经理的能力是影响基金绩效的一个重要因素。
投资者可以通过研究基金经理的投资策略、经验以及过往的业绩来评估其能力。
一般来说,经验丰富、投资策略稳健的基金经理更容易取得良好的绩效。
二、评估方法在评估基金绩效时,投资者可以采用以下几种常用的方法:1. 基准比较法基准比较法是一种常见且直观的评估方法。
投资者可以选择一个或多个与基金运作策略相匹配的基准,在同一时间对比其收益率、风险等指标。
若基金的绩效明显超过基准,则可以认为其绩效较好。
2. 同类排名法同类排名法是一种通过比较基金在同一类别中的排名来评估绩效的方法。
投资者可以通过查阅报表或咨询专业机构,了解基金在同类别中的排名情况。
但需要注意的是,同类排名法只能反映基金相对于同一类别基金的表现,并不能全面评估基金的绩效。
3. 历史回溯法历史回溯法是一种通过分析基金过去的绩效来预测其未来表现的方法。
投资者可以研究基金的历史收益率、波动率等指标,以及基金经理的投资决策,来评估基金未来的发展潜力。
然而,需要注意的是,过去的业绩并不代表未来的表现,投资者应该结合其他因素进行综合分析。
基金报告分析基金绩效评估的核心指标与方法解析
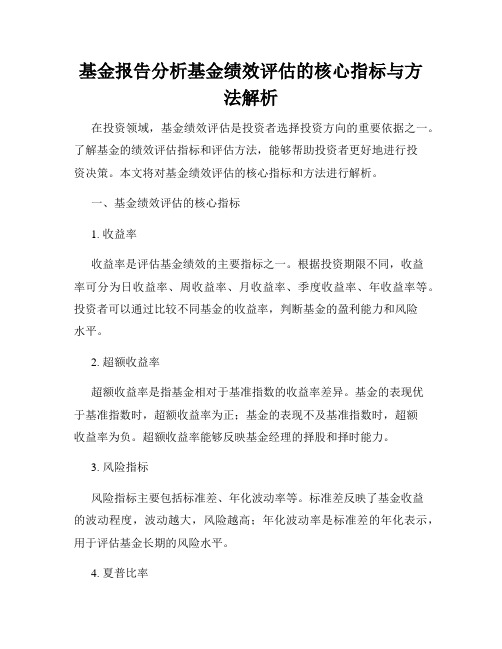
基金报告分析基金绩效评估的核心指标与方法解析在投资领域,基金绩效评估是投资者选择投资方向的重要依据之一。
了解基金的绩效评估指标和评估方法,能够帮助投资者更好地进行投资决策。
本文将对基金绩效评估的核心指标和方法进行解析。
一、基金绩效评估的核心指标1. 收益率收益率是评估基金绩效的主要指标之一。
根据投资期限不同,收益率可分为日收益率、周收益率、月收益率、季度收益率、年收益率等。
投资者可以通过比较不同基金的收益率,判断基金的盈利能力和风险水平。
2. 超额收益率超额收益率是指基金相对于基准指数的收益率差异。
基金的表现优于基准指数时,超额收益率为正;基金的表现不及基准指数时,超额收益率为负。
超额收益率能够反映基金经理的择股和择时能力。
3. 风险指标风险指标主要包括标准差、年化波动率等。
标准差反映了基金收益的波动程度,波动越大,风险越高;年化波动率是标准差的年化表示,用于评估基金长期的风险水平。
4. 夏普比率夏普比率是用于衡量基金的风险调整后的收益能力。
夏普比率越高,说明投资者每承担一单位的风险能获取到的超额收益越多。
5. 信息比率信息比率是用于衡量基金经理的主动管理能力。
信息比率越高,说明基金经理通过择股和择时等主动管理操作可以获得更多超额收益。
二、基金绩效评估的方法1. 基准指数法基准指数法是最常用的基金绩效评估方法之一。
该方法将基金的收益与一个相关的基准指数进行比较,以判断基金的表现。
若基金的超额收益率为正,说明基金的表现优于基准指数。
2. 指标系统法指标系统法是综合考虑多个评价指标来评估基金绩效的方法。
其中,可以利用TOPSIS法(技术协同最优解与最劣解法)将基金按照不同指标加权排名,得出综合评价结果。
3. 因子模型法因子模型法是根据统计模型和回归分析,利用多个因子(如市场因子、规模因子等)来解释基金绩效的方法。
通过分析基金与各种因子之间的关系,判断基金管理者的投资能力。
4. 经验法经验法是基于基金经理的经验和投资风格进行评估的方法。
共同基金绩效归因模型研究

共同基金绩效归因模型研究共同基金绩效归因模型是用来解析和评估共同基金的投资绩效的一种常用方法。
它通过对基金投资组合中各种因素的贡献进行分析,帮助投资者了解基金经理的择股能力和择时能力。
本文将对共同基金绩效归因模型的研究进行探讨,并介绍其中两种常见的归因模型。
一、共同基金绩效归因模型概述共同基金绩效归因模型是一种用于分析共同基金投资绩效的定量方法。
它通过解析基金的收益来源,分析投资经理在股票选择、行业配置、择时等方面的表现,从而评估基金的绩效。
归因模型通常基于资本资产定价模型(CAPM)或测度风险模型(MFM),采用多元线性回归的方法进行分析。
二、CAPM模型CAPM模型是一种常见的共同基金绩效归因模型,它基于资本市场有效理论,对基金的收益进行归因分析。
CAPM模型认为,股票的收益率可以被市场风险和个股的系统风险来解释。
在共同基金绩效归因中,CAPM模型用来衡量基金经理的选股能力。
CAPM模型的基本公式为:Ri = Rf + βi × (Rm - Rf) + αi其中,Ri表示基金的预期收益率,Rf表示无风险利率,βi表示基金与市场收益率的相关性,Rm表示市场的预期收益率,αi表示基金的超额收益。
通过对基金的历史收益数据进行回归分析,可以得到基金的β值和α值。
β值反映了基金对市场收益率的敏感度,α值则衡量了基金的超额收益。
三、MFM模型MFM模型是另一种常见的共同基金绩效归因模型,它基于多因子模型对基金的收益进行解释。
MFM模型考虑了多个因素对基金收益的影响,包括股票选择能力、行业配置能力、择时能力等。
MFM模型的基本公式为:Ri = αi + β1i × F1 + β2i × F2 + ... + βni × Fn + εi其中,Ri表示基金的预期收益率,αi表示基金的超额收益,β1i - βni表示基金在不同因子上的暴露程度,F1 - Fn表示不同的风险因子,εi表示残差项。
投资学ch04共同基金分析解析

4.3 共同基金
共同基金( Mutual fund )简称基金,是指通过 发售基金份额,将众多投资者的资金集中起来, 形成独立财产,由基金托管人托管,基金管理人 管理,以投资组合的方法进行证券投资的一种利 益共享、风险共担的集合投资方式。 注 意 : 基 金 当 事 人 有 三 方 : 基 金 持 有 人 (Fund Share Holders) 、基金管理人(Fund Manager)、 基金托管人(Fund Trustee)。
4-25
4.4 共同基金的投资成本(续)
4.4.2费用与共同基金的收益 收益率=( NAV1-NAV0+收入和资 本利得的分配) / NAV0 NAV0:期初资产净值 NAV1:期末资产净值 这里我们在计算收益率时忽略了佣金 ,如购买基金的前端费用。
4-26
基金净值的估计
估值:就是确定资产净值(或单位净值) (1)目的
4-13
集合投资 基金管理人 投资者甲
组合投资
证券A
投资者乙
基
金
证券B
投资者丙
证券C
基金托管人
4-14
4.3
4.3.1 4.3.2
共同基金
投资策略 如何出售基金
4-15
4.3 共同基金—分类
根据不同标准,基金可分不同种类: 1.封闭式基金和开放式基金(根据基金单位是否可增加或 赎回); 2.契约型和公司型(根据组织形态不同) 3.股票基金、债券基金、货币市场基金、混合基金(根据 投资对象或投资策略的不同分类); 4.指数基金; 5.保本基金; 6.ETF和LOF; 7.国际基金、离岸基金、国家基金等; 8.美元基金、日元基金、欧元基金; 9.伞型基金和基金中基金。
第4章
共同基金和其他投资公司
共同基金常识
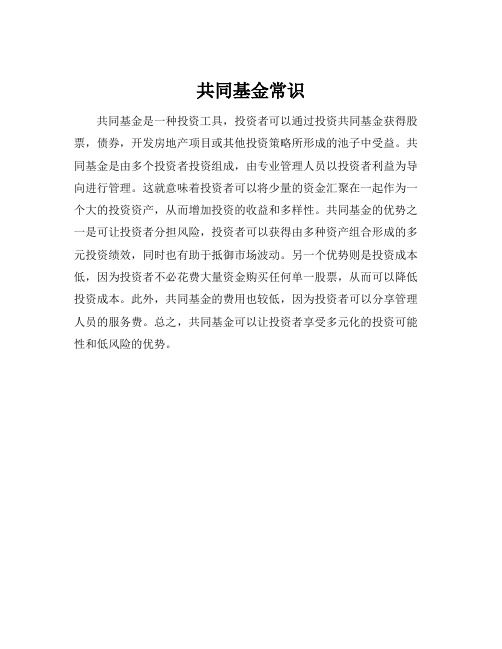
共同基金常识
共同基金是一种投资工具,投资者可以通过投资共同基金获得股票,债券,开发房地产项目或其他投资策略所形成的池子中受益。
共同基金是由多个投资者投资组成,由专业管理人员以投资者利益为导向进行管理。
这就意味着投资者可以将少量的资金汇聚在一起作为一个大的投资资产,从而增加投资的收益和多样性。
共同基金的优势之一是可让投资者分担风险,投资者可以获得由多种资产组合形成的多元投资绩效,同时也有助于抵御市场波动。
另一个优势则是投资成本低,因为投资者不必花费大量资金购买任何单一股票,从而可以降低投资成本。
此外,共同基金的费用也较低,因为投资者可以分享管理人员的服务费。
总之,共同基金可以让投资者享受多元化的投资可能性和低风险的优势。
分析基金的业绩与绩效评价指标
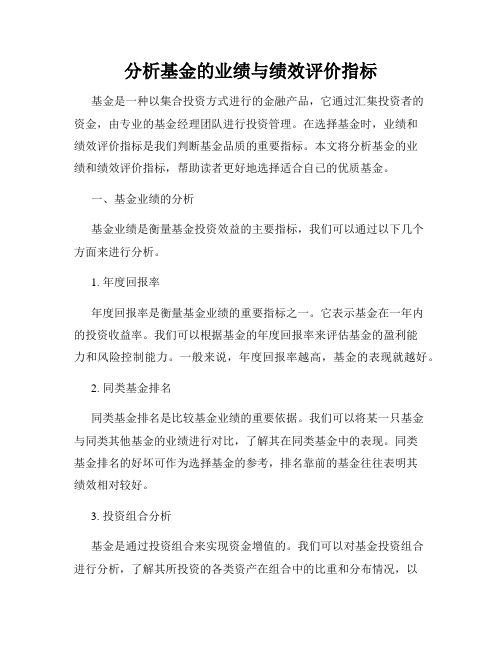
分析基金的业绩与绩效评价指标基金是一种以集合投资方式进行的金融产品,它通过汇集投资者的资金,由专业的基金经理团队进行投资管理。
在选择基金时,业绩和绩效评价指标是我们判断基金品质的重要指标。
本文将分析基金的业绩和绩效评价指标,帮助读者更好地选择适合自己的优质基金。
一、基金业绩的分析基金业绩是衡量基金投资效益的主要指标,我们可以通过以下几个方面来进行分析。
1. 年度回报率年度回报率是衡量基金业绩的重要指标之一。
它表示基金在一年内的投资收益率。
我们可以根据基金的年度回报率来评估基金的盈利能力和风险控制能力。
一般来说,年度回报率越高,基金的表现就越好。
2. 同类基金排名同类基金排名是比较基金业绩的重要依据。
我们可以将某一只基金与同类其他基金的业绩进行对比,了解其在同类基金中的表现。
同类基金排名的好坏可作为选择基金的参考,排名靠前的基金往往表明其绩效相对较好。
3. 投资组合分析基金是通过投资组合来实现资金增值的。
我们可以对基金投资组合进行分析,了解其所投资的各类资产在组合中的比重和分布情况,以及所投资证券的风险分散度。
一个良好的投资组合应该具备分散风险、收益稳定的特点。
二、基金绩效评价指标在分析基金绩效时,绩效评价指标起着关键作用。
下面介绍几个常用的绩效评价指标。
1. 夏普比率夏普比率是衡量基金业绩相对风险的指标。
它表示单位风险下的超额收益。
夏普比率越高,基金的收益越有吸引力。
2. 收益风险比收益风险比是衡量基金盈利能力与风险承受能力的比例关系。
它体现了基金在获得收益的同时,所承担的风险程度。
收益风险比越高,基金的绩效越理想。
3. 基金回撤率基金回撤率是衡量基金抗跌能力的指标。
它表示在某个时期内,基金净值高点到低点的最大跌幅。
较低的回撤率表明基金在市场下跌时能够保持相对稳定的表现。
4. 手续费率手续费率是指投资基金所产生的费用比例。
投资者在选择基金时应重点关注手续费率,以避免手续费过高对收益的影响。
总结:在选择基金时,我们应该综合考虑基金的业绩和绩效评价指标。
证券投资基金绩效评估模型分析

证券投资基金绩效评估模型分析【发布时间】2002-1-10本文依据衡量指标的不同,将目前国际上较为流行的证券投资基金绩效评估模型分为五大类,并评述了这五大类模型的运用方式、作用和区别,以及这些模型运用的相关检验,最后进行了简要的评论并提出了几点建议。
证券投资基金绩效的评估主要是针对一只基金的实际运作成果进行评价。
在绩效评估中,主要包括:(1)对基金的整体收益进行评估,判断其是否超过市场平均收益;(2)超过市场平均收益的部分中有多少可归结为基金经理的投资才能;(3)采用什么因素或指标对基金绩效进行评估,并判断不同因素或指标对绩效评估结果的影响;(4)选择什么类型的评估模型,评估模型的选择应根据一国的基金市场状况等因素而确定。
根据以上内容及不同管理风格的基金,评估基金绩效的因素或指标主要分为两类:对于采用消极管理风格的基金,主要是评估其在证券市场的一般收益水平和风险水平;对于采用积极管理风格的基金,除了以上两个指标外,还包括基金经理的选股能力、市场运作中的时间选择(或定时)能力以及基金组合的分散化程度等指标。
这些指标分别衡量了基金经理预测市场的发展趋势、识别证券价格是否被低估或高估及控制风险的能力。
本文的分析是根据积极管理风格基金类型来进行的。
一、理论模型分析(一)Markowits均值一方差模型证券投资基金投资及其他风险资产投资首先需要解决的是预期收益与预期风险这两个核心问题。
如何测定组合投资的预期收益与预期风险、如何以这两项指标进行资产分配,是市场投资者迫切需要解决的问题。
正是在这样的背景下,Markowits(1952、1958)的理论应运而生。
该理论依据以下4个假设:1.投资者在考虑每一次投资选择时,其依据是某一持仓时间内的证券收益的概率分布。
2.投资者是根据证券的预期收益率估测证券组合的风险。
3.投资者的投资决定仅仅是依据证券的预期收益和预期风险。
4.在一定的风险水平上,投资者希望收益最大,相应的是在一定的收益水平上,投资者希望风险最小。
- 1、下载文档前请自行甄别文档内容的完整性,平台不提供额外的编辑、内容补充、找答案等附加服务。
- 2、"仅部分预览"的文档,不可在线预览部分如存在完整性等问题,可反馈申请退款(可完整预览的文档不适用该条件!)。
- 3、如文档侵犯您的权益,请联系客服反馈,我们会尽快为您处理(人工客服工作时间:9:00-18:30)。
共同基金绩效的持续性
共同基金(Mutual Fund)是一种利益共享、风险共担的集合投资方式,即通过发行基金单位,集中投资者的资金,从事股票、债券、外汇、货币等投资,以获得投资收益和资本增值。
热手效应(hot-hand effect),来源于篮球运动中。
指比赛时如果某队员连续命中,其他队员一般相信他“手感好”,下次进攻时还会选择他来投篮,可他并不一定能投进。
仅凭一时的直觉,缺乏必要的分析判断就采取措施就叫做热手效应。
动量交易策略,即预先对股票收益和交易量设定过滤准则,当股票收益或股票收益和交易量同时满足过滤准则就买入或卖出股票的投资策略。
普通股票回报的共同因素和共同基金的费用和交易成本的持续性差异共同基金回报的可预测性也就是共同基金绩效具有持续性
股票回报一年期动量解释了共同基金绩效的热手效应
交易成本会消耗实施动量投资策略的股票的收益
费用对基金业绩产生负面影响,而营业额也对绩效产生负面影响。
交易降低绩效——贸易市场价值约0.95%。
整个共同基金每笔交易成本的变化也解释了绩效持久性的一部分。
基金绩效和负载费用有很强的负相关
短期互惠基金回报持续强烈,最持久的解释是共同因素的敏感性、费用、交易成本。
购买等分等分失败者过去的获奖者和销售的净收益回报是每年的8%。
我解释4.6%,与股票回报率的大小,书市场,一年的势头,费用率0.7% ;与交易成本的1 %。
然而,十分位数1和9之间传播的5.4%,四因子模型解释了4.4 %,说明0.9%的费用率和交易成本,使每年只有0.1 %的传播原因不明。
最低十等分的两倍的费用比率估计交易成本,性能表现不佳后,这些解释仍然是异常。
因此,横截面共同基金的平均回报不能解释变量几乎全部集中在底部的两个过去回报排序等分的投资组合之间的价差。