基于均匀设计的最小二乘支持向量机改进算法
最小二乘支持向量机的两点改进

s p a t i a l i n f o r ma t i o n o f a t e r r e s t r i a l o b j e c t .wh i c h i n l f u e n c e s c l a s s i i f c a t i o n p r e c i s i o n .T h e r e f o ri o n
( C o l l e g e o f I n f o r ma t i o n a n d C o m mu n i c a t i o n E n g i n e e i r n g , Ha r b i n E n g i n e e i r n g U n i v e r s i t y , H a r b i n 1 5 0 0 0 1 ,C h i n a )
s t r a t e g y b a s e d o n t h e Co u l o mb g r a v i t y mo d e l i s p r o p o s e d .An d o n t hi s b a s i s ,a ne w c l a s s i ie f r mo d e l wh i c h me r g e s t h e a b o v e c l a s s i ic f a t i o n r e s u l t wi t h t h e c l a s s i ie f r b a s e d o n s p a t i a l i nf o m a r t i o n c a n s o l v e t h e t wo p r o b l e ms me n t i o n e d
On t h e o t h e r h a n d,L S S VM ma i n l y u s e s s p e c t r a l d a t a f o r t r a i n i n g a n d c l a s s i i f c a t i o n,a n d i t i g n o r e s mi n i n g o n t h e
最小二乘配置的一种改进解法
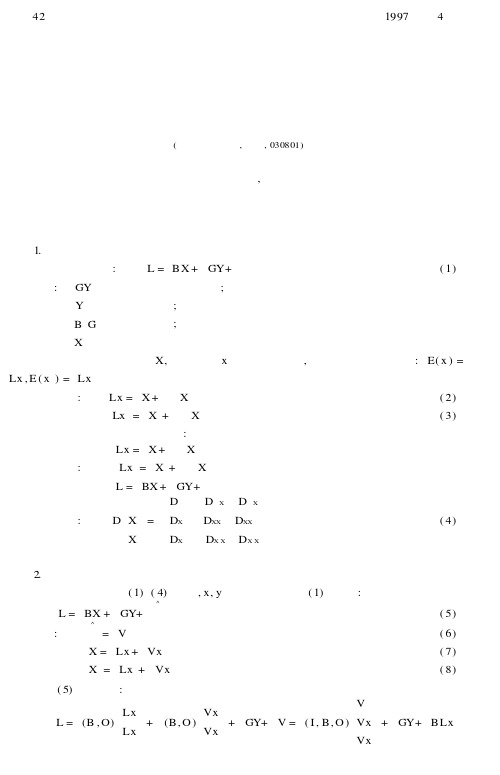
将 ( 22)式代入 ( 17)可得 V = P - 1 AT N - 1 ( f - GY) = P - 1 A- 1 N 取 e = 1, 则 P ∵ N = AD A
T 2 0 - 1
( 23)
= Q= D D△△ D△ X DX X D△ X′ I DXX′ B T ( 24 )
∴ N = (I , B , O ) D X△
= ( GT N - 1 G) - 1 GN - 1 (L - B LX ) V= P =
- 1
A N ( f - G(G N D△△ + D△ X BT DX△ + DXX BT
T
T
- 1
T
-
1
G)
- 1
G N
T
T
- 1
f) G)
- 1
N
- 1
[I - G( G N
- 1
GN
T
- 1
(L - B LX ) ]
- 1
[I - G(G N
-
1
G)
- 1
GN
- 1
把 N 代入 ( 25)、 ( 29)、 ( 30)便与参考文献一样的结果。 4. 结论与讨论 文献以间接平差原理求解 , 设虚拟观测值 , 在求解过程中 : L = BX + GY+ V , X 是当 作非随机量来求解 ,实际上 X 是随机量 , 文献作了一个转换。 而本文提出的解法中把 X 当作 随机量来看待 ,没有这个转换过程 ,但两者有着相同的结果 ,且较之间捷。
^
( 1)
为倾向参数 ; 为已知矩阵 ; 表示信号。
文献的解法以滤波信号 X, 推估信息 x ′ 为虚拟观测值 , 根据它们的先验信息: E( x ) = ( 2) ( 3)
基于改进的最大后验概率矢量量化和最小二乘支持向量机集成算法
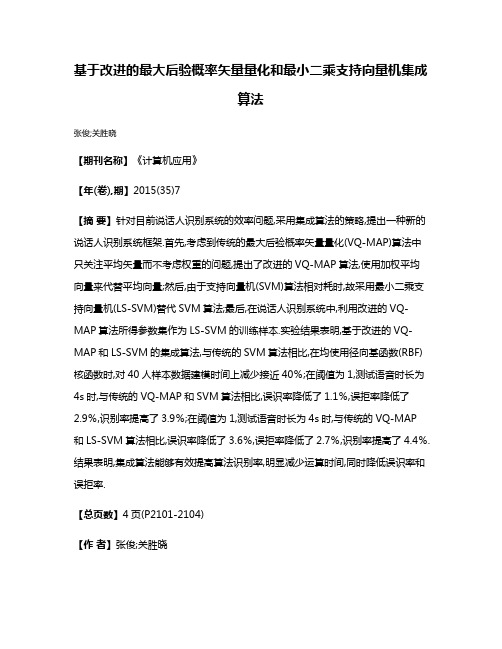
基于改进的最大后验概率矢量量化和最小二乘支持向量机集成算法张俊;关胜晓【期刊名称】《计算机应用》【年(卷),期】2015(35)7【摘要】针对目前说话人识别系统的效率问题,采用集成算法的策略,提出一种新的说话人识别系统框架.首先,考虑到传统的最大后验概率矢量量化(VQ-MAP)算法中只关注平均矢量而不考虑权重的问题,提出了改进的VQ-MAP算法,使用加权平均向量来代替平均向量;然后,由于支持向量机(SVM)算法相对耗时,故采用最小二乘支持向量机(LS-SVM)替代SVM算法;最后,在说话人识别系统中,利用改进的VQ-MAP算法所得参数集作为LS-SVM的训练样本.实验结果表明,基于改进的VQ-MAP和LS-SVM的集成算法,与传统的SVM算法相比,在均使用径向基函数(RBF)核函数时,对40人样本数据建模时间上减少接近40%;在阈值为1,测试语音时长为4s时,与传统的VQ-MAP和SVM算法相比,误识率降低了1.1%,误拒率降低了2.9%,识别率提高了3.9%;在阈值为1,测试语音时长为4s时,与传统的VQ-MAP 和LS-SVM算法相比,误识率降低了3.6%,误拒率降低了2.7%,识别率提高了4.4%.结果表明,集成算法能够有效提高算法识别率,明显减少运算时间,同时降低误识率和误拒率.【总页数】4页(P2101-2104)【作者】张俊;关胜晓【作者单位】中国科学技术大学信息科学技术学院,合肥230027;中国科学技术大学信息科学技术学院,合肥230027【正文语种】中文【中图分类】TN391.4;TP181;TN912.34【相关文献】1.一种改进的基于矩形的位图矢量化方法研究 [J], 桑伟泉;张小平2.基于改进人工蜂群的图像矢量量化码书设计算法 [J], 郭艳菊;陈雷;陈国鹰3.基于改进Loop细分的二维图像矢量化方法 [J], 邓重阳; 熊玉丹; 郑乐豪; 曹晨妤; 朱明超4.基于改进学习矢量量化神经网络输电线路故障识别技术 [J], 宋亮亮;杨毅;范栋琛;朱诚5.工程图矢量化中基于直线HOUGH变换的局部参数曲线矢量化方法 [J], 邹荣金;蔡士杰;张福炎因版权原因,仅展示原文概要,查看原文内容请购买。
基于Matlab的最小二乘支持向量机的工具箱及其应用
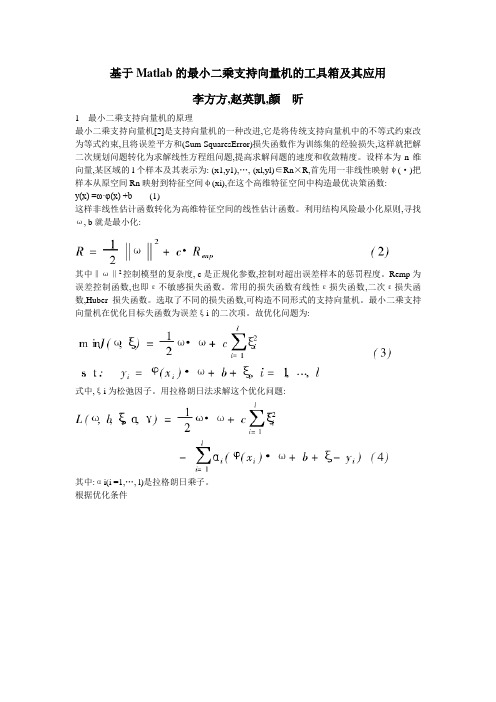
基于Matlab的最小二乘支持向量机的工具箱及其应用李方方,赵英凯,颜昕1最小二乘支持向量机的原理最小二乘支持向量机[2]是支持向量机的一种改进,它是将传统支持向量机中的不等式约束改为等式约束,且将误差平方和(Sum SquaresError)损失函数作为训练集的经验损失,这样就把解二次规划问题转化为求解线性方程组问题,提高求解问题的速度和收敛精度。
设样本为n维向量,某区域的l个样本及其表示为: (x1,y1),…, (xl,yl)∈Rn×R,首先用一非线性映射ψ(·)把样本从原空间Rn映射到特征空间φ(xi),在这个高维特征空间中构造最优决策函数:y(x) =ω·φ(x) +b(1)这样非线性估计函数转化为高维特征空间的线性估计函数。
利用结构风险最小化原则,寻找ω, b就是最小化:其中‖ω‖2控制模型的复杂度, c是正规化参数,控制对超出误差样本的惩罚程度。
Remp为误差控制函数,也即ε不敏感损失函数。
常用的损失函数有线性ε损失函数,二次ε损失函数,Huber损失函数。
选取了不同的损失函数,可构造不同形式的支持向量机。
最小二乘支持向量机在优化目标失函数为误差ξi的二次项。
故优化问题为:式中,ξi为松弛因子。
用拉格朗日法求解这个优化问题:其中:αi(i =1,…, l)是拉格朗日乘子。
根据优化条件可得到:其中:αi= c·ξi,ω·φ(xi) +b +ξi-yi=0。
定义核函数K(xi,yi) =φ(xi)·φ(xj)是满足条件的对称函数。
根据文献[6],优化问题转化为求解线性方程:最后用最小二乘法求出a与b,最小二乘支持向量机也由此得名,并且得到非线性预测模型:K(xi,x) =Φ(xi)·Φ(x)称为核函数,它是满足Mercer条件的任何对称的核函数对应于特征空间的点积。
核函数的种类较多,常用的有:(1)多项式函数:(2)RBF函数:(3)Sigmoid函数:k(xi,x) =tanh(v(x·xi) +c)(4)B样条函数:K(x,y) =B2n(x-y)2LS-SVMlab工具箱基于Matlab的LS-SVMlab工具箱[3]主要包括分类、回归、时间序列预测和无监督学习。
改进最小二乘支持向量机电量预测算法

改进最小二乘支持向量机电量预测算法杨柳;吴延琳;张超;刘超;蒋勃;张鹏【摘要】Basing on the inherent law of electric data and the relationship of external environment,theelectricity monthseason ratio (EMSR) is defined.Proposing the improved power prediction algorithm that combines least squares support vector machine algorithm and the quarterly EMSR forecast algorithm.The monthly power data of province A is used to do the verification.By comparing the forecast error of Elman neural network,BP neural network with the forecast error of proposed algorithm.The accuracy of the proposed algorithm is verified,which shows that the accuracy has been improved.%基于电量数据内在规律及与外界环境变量关系的深度挖掘,定义了占季比指标,提出了最小二乘支持向量机算法与基于占季比预测相结合的电量预测混合算法;根据国内A省历史分月电量进行实际算例分析,通过Elman神经网络、BP神经网络预测方法与文中所提方法的预测误差对比,验证了所提算法的预测精度,证明所提算法对预测精度有较大提高.【期刊名称】《电网与清洁能源》【年(卷),期】2017(033)003【总页数】6页(P71-76)【关键词】电量预测;Elman神经网络;最小二乘支持向量机;占季比【作者】杨柳;吴延琳;张超;刘超;蒋勃;张鹏【作者单位】国网陕西省电力公司,陕西西安710048;国网陕西省电力公司经济技术研究院,陕西西安710065;国网陕西省电力公司,陕西西安710048;国网陕西省电力公司经济技术研究院,陕西西安710065;国网陕西省电力公司,陕西西安710048;国网陕西省电力公司经济技术研究院,陕西西安710065【正文语种】中文【中图分类】TM715负荷电量预测是电网规划、营销与调度运行的基础,伴随着“互联网+”与智能电网建设,电网规划、智能营销、智能调度等都对电量预测精度提出了更高的要求。
基于遗传算法参数优化的最小二乘支持向量机财务困境预测

基于遗传算法参数优化的最小二乘支持向量机财务困境预测赵冠华;李玥;赵娟【摘要】When using traditional support vector machine to make financial distress prediction, we need to solve the complex quadratic programming problems, which are quite difficult. At the same time, the least squares support vector machine (LS-SVM) can solve the quadratic programming problems by transferring them into linear equations, effectively reducing the difficulty. Especially when applying genetic algorithm to optimize parameters and kernel parameters of LSSVM, the prediction accuracy is significantly improved. We randomly selected 252 A-share listed companies during 2002-2007 from Shanghai and Shenzhen Stock Exchanges as the research samples and divided them into two Sample I and Sample II. Then we carried out short-term and long-term predictions of these two sets of samples res The empirical results showed that the prediction effects of LS-SVM model based on genetic algorithm was better of traditional statistical Logit Model as well as the traditional support vector machine. higher accuracy rate compared with long-term prediction. groups - pectively. than that Besides, short-term prediction had a In addition, the number of training samples directly affected the prediction accuracy and they were positively correlated.%传统支持向量机应用于财务困境预测时,需要求解复杂的二次规划问题,求解难度大。
基于改进最小二乘支持向量机和预测误差校正的短期风电负荷预测

Short-term wind load forecasting based on improved LSSVM and error forecasting correction
LI Xiao1, WANG Xin1, ZHENG Yihui1, LI Lixue1, SHENG Xikui2, WU Hao2 (1. Center of Electrical & Electronic Technology, Shanghai Jiao Tong University, Shanghai 200240, China; 2. Yanbian Power Supply Company, Jilin Electric Power Co., Ltd., State Grid Corporation of China, Yanbian 133000, China) Abstract: In order to improve the wind load forecasting precision and ensure the effective use of wind power resources, a method based on improved least square support vector machine (LSSVM) combined with error forecasting is proposed. Firstly, lifting wavelet transform (LWT) decomposition of the original data is introduced to effectively extract the main features, with which the randomness of the wind is overcome; secondly LSSVM is used for the prediction of decomposed signals to ensure accuracy; then, error forecasting (EF) is added to reduce the large error points and improve the stability of the results. Finally, experimental results using real wind farm data show that the forecasting model is better in both generalization performance and predictive accuracy, and may provide an effective and practical way for the short-term wind load forecasting. This work is supported by National Natural Science Foundation of China (No. 60504010) and National High-tech R & D Program of China (863 Program) (No. 2008AA04Z129). Key words: lifting wavelet transform (LWT); least square support vector machine (LSSVM); error forecasting (EF); wind load forecasting 中图分类号: TM714 文献标识码:A 文章编号: 1674-3415(2015)11-0063-07
改进的最小二乘支持向量机算法及应用

改进的最小二乘支持向量机算法及应用张吉斌(兰州交通大学自动化与电气工程学院,兰州 730070)摘要:针对粒子群优化算法(PSO)的局部搜索能力差和存在早熟收敛的问题,将禁忌搜索(TS)思想融入到粒子群算法中,提出一种新的禁忌搜索的粒子群优化算法。
该新算法结合了PSO和TS各自的优点,在寻优前期利用粒子群算法得到较好的初始值,同时将个体极值pbest放入禁忌表,在寻优后期,当粒子的搜索能力减弱时,利用禁忌搜索算法中禁忌表的短期记忆功能,使其跳出局部最优解,并且在搜索过程中允许接受劣解。
将改进的算法应用于函数的优化,仿真结果表明,该算法计算精度和稳定性都有所提高,验证了改进算法的有效性。
关键词:粒子群算法;禁忌搜索;函数优化中图分类号:文献标识码:A文章编号:An Improved Tabu Search-Particle Swarm Optimization Algorithm and Its ApplicationZHANG Ji-bin(School of Automation and Electrical Engineering, Lanzhou Jiaotong University, Lanzhou 730070, China) Abstract:Considering the poor local search capability and the premature convergence of the Particle Swarm Optimization (PSO), combining Tabu Search(TS) ,a new PSO with TS is proposed .The new algorithm combines the PSO and TS respective advantages, in the early period use particle swarm optimization algorithm to get some good initial values, and at the same time, put individual optimal solution pbest into tabu list, in the later stage of search process, use tabu list’s short term memory function makes particles jump out of local optimal solution and allow accept bad solutions in the search process.With the application of function optimization, simulation results show that the improved algorithm has high precision and stability, and it proves that the improvement is efficient. Key words: Particle Swarm Optimization;tabu search;function optimization0 引言近年来,许多优化算法被提出应用于解决最优化问题,其中启发式方法越来越受到关注。
- 1、下载文档前请自行甄别文档内容的完整性,平台不提供额外的编辑、内容补充、找答案等附加服务。
- 2、"仅部分预览"的文档,不可在线预览部分如存在完整性等问题,可反馈申请退款(可完整预览的文档不适用该条件!)。
- 3、如文档侵犯您的权益,请联系客服反馈,我们会尽快为您处理(人工客服工作时间:9:00-18:30)。
摘要: 针对最小二乘支持向量机模型的参数选取耗时长, 容易陷入局部最优而导致过拟合的问题 , 提出了一种基于均匀设计
的将 大 样 本 搜 索转 化 为 小样 本 搜 索 技 术 的参 数 寻 优 方法 。把 支 持 向量 机 算 法 的每 一 次 训 练 过程 作 为 一 个 试 验 考 虑 , sl so sta tcn b t ov hspo lm f e s su rssp o e trmahn sa d hn s h i ai eut h w h ti a oh sleti rbe o at q ae u p r vco c ie n mu o l t
2. Te c rAdv nc d Stdy S h o fCh n i a he a e u c o lo e x ,Che i Hu a 41 5 nx , n n, 9 00;
3 no ai c neadT cnl o ee f u a g cl r n esy hnsaH nn4 02 ,C ia .If m t nSi c n ehoo C l g nnA r ut a U i r t,C agh ua 1 18 h ) r o e y g l oH i ul v i n
XI ANG a g s e g , Ch n - h n ZHOU -yn Z Zi i g , HANG i — e g L n fn
( .O e t c n e& T c n l yC l g f u a gi l rl nvr t , h n s aH n n4 0 2 , hn ; 1 r n Si c i e e h oo ol eo H n nA r u ua U i s y C a gh u a 1 1 8 C ia g e ct ei
第2卷 第3 8 期
文 章 编号 :0 6 94 ( 0 1 0 — 14 0 10 — 3 8 2 1 ) 3 09 — 4
计
算
机
仿
真
21年3 01 月
基 于均 匀设 计 的最小 二 乘支 持 向量 机 改进算 法
向 昌盛 周子英 张林峰 , ,
( .湖 南农 业 大学 东 方科 技 学 院 , 1 湖南 长 沙 40 2 ;.湖 南 辰 溪县 进 修 学 校 , 1 182 湖南 辰 溪 ,15 0 49 0 ; 3 湖 南农 业 大 学 信息 科 学 技 术 学 院 , 南 长 沙 40 2 ) . 湖 118
A S R C T e aa t s e c o es S urs u pr V c rM cie L S M)t e n meadi B T A T:h r e l t ni L at q ae po et ahns( SV p me r s e i n S t o a sl gt n k o i s
中 图分 类 号 :P 8 T 11 文 献 标 识码 : A
I pr v d a tS u r sSu po tVe t r M a h n m o e Le s q a e p r c o c ie
Al o ihm s d o io m sg g rt Ba e n Un f r De i n
响因子为算法的参数 , 运用均匀设计的手段进行方案设计 , 采用统计 的方法对结果进行分析和选择 。最后把该方法应用于 3 个大样本数据集建模 中的参数优化 , 仿真结果表明方法 大幅度减小 了时 间复杂度 , 较好地解决 了最小二乘支持 向量机 的参
数优 化 问题 , 时获 得 精 确 的建 模 效 果 。 同 关键 词 : 小二 乘 支 持 向 量机 ; 数 选 择 ; 匀 设 计 ; 化 最 参 均 优
e s o f l it o a p i m n e dst h v f tn r b e . A e m eh d i r p s d b s d o io e a y t al n o lc lo tmu a d l a o t e o e ti g p o lm i n w t o s p o o e a e n unf r d — m sg in,i ih te lr e c l e rh i ha g d i o t m al c l e r h. Ea h tanig po e st he s p r n whc h ag -sa e s a c s c n e nt he s l -s ae s ac c r i n rc s he t up o t v co c nea g rt m s c nsd r d a io ot s h mp c a t r ft e ag rt e trma hi l o h i o ie e s a pltt e tt e i a tf co so h o hm a a tr ,t n unfr i l i p mee r s he i m o de in i s d o de in p r mee s,a d saitc lme h d r e o a l z h e ut a s lc a a ee s sg s u e t sg a a tr n t tsia t o s a e us d t nay e t e r s ls nd ee tp m tr . r Th e a g aa s t r r d ce sn h smeho o e e tn h a a tr fla ts uae u o tv co r e l e d t esa e p e it d u ig t i t d frs l cig t e p r mee so e s q r s s pp r e trma r